Underwater robot connects humans’ sight and touch to deep sea
The Next Big Thing in Manufacturing: XaaS to Boost Productivity and Revenue
Robots learn household tasks by watching humans
Using artificial intelligence to train teams of robots to work together
Emergency-response drones to save lives in the digital skies
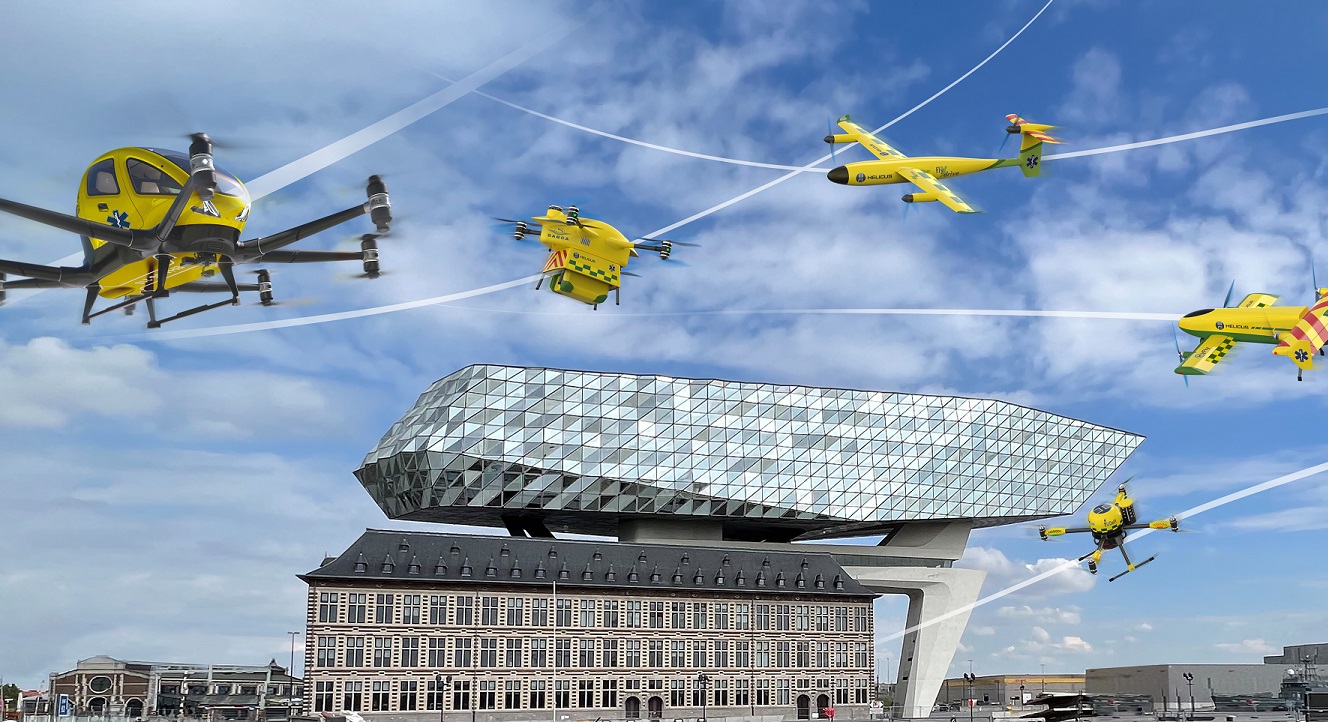
Uncrewed aircraft in the sky above the headquarters of the Port of Antwerp-Bruges. © Helicus – Geert Vanhandenhove, Rik Van Boxem, 2022
By Gareth Willmer
In a city in the future, a fire breaks out in a skyscraper. An alarm is triggered and a swarm of drones swoops in, surrounds the building and uses antennas to locate people inside, enabling firefighters to go straight to the stricken individuals. Just in the nick of time – no deaths are recorded.
Elsewhere in the city, drones fly back and forth delivering tissue samples from hospitals to specialist labs for analysis, while another rushes a defibrillator to someone who has suffered a suspected cardiac arrest on a football pitch. The patient lives, with the saved minutes proving critical.
At the time of writing, drones have already been used in search-and-rescue situations to save more than 880 people worldwide, according to drone company DJI. Drones are also being used for medical purposes, such as to transport medicines and samples, and take vaccines to remote areas.
Drones for such uses are still a relatively new development, meaning there is plenty of room to make them more effective and improve supporting infrastructure. This is particularly true when it comes to urban environments, where navigation is complex and requires safety regulations.
Flying firefighters
The IDEAL DRONE project developed a system to aid in firefighting and other emergencies to demonstrate the potential for using swarms of uncrewed aerial vehicles (UAVs) in such situations. Equipped with antennas, the drones use a radio-frequency system to detect the location of ‘nodes’ – or tags – worn by people inside a building.
“By knowing how many people are inside the building and where they are located, it will optimise the search-and-rescue operation.”
– Prof Gian Paolo Cimellaro, IDEAL DRONE
Making use of an Italian aircraft hangar, the tests involved pilots on the ground flying three drones around the outside of a building. The idea is that the drones triangulate the position of people inside where their signals intersect, as well as detecting information about their health condition. The details can then be mapped to optimise and accelerate rescue operations, and enhance safety for firefighters by allowing them to avoid searching all over a burning building without knowing where people are.
‘You create a sort of temporary network from outside the building through which you can detect the people inside,’ said Professor Gian Paolo Cimellaro, an engineer at the Polytechnic University of Turin and project lead on IDEAL DRONE.
‘By knowing how many people are inside the building and where they are located, it will optimise the search-and-rescue operation.’
He added: ‘A unique characteristic of this project is that it allows indoor tracking without communication networks such as Wi-Fi or GPS, which might not be available if you are in an emergency like a disaster or post-earthquake situation.’
There are some challenges in terms of accuracy and battery life, while another obvious drawback is that people in the building need to already be wearing trackers.
However, said Prof Cimellaro, current thinking is that this can be unintrusive if tags are incorporated in existing technology that people often already carry such as smartwatches, mobile phones or ID cards. They can also be used by organisations that mandate their use for staff working in hazardous environments, such as factories or offshore oil rigs.
Looking beyond the challenges, Prof Cimellaro thinks such systems could be a reality within five years, with drones holding significant future promise for avoiding ‘putting human lives in danger’.
Medical networks
Another area in which drones can be used to save lives is medical emergencies. This is the focus of the SAFIR-Med project.
Belgian medical drone operator Helicus has established a command-and-control (C2C) centre in Antwerp to coordinate drone flights. The idea is that the C2C automatically creates flight plans using artificial intelligence, navigating within a digital twin – or virtual representation – of the real world. These plans are then relayed to the relevant air traffic authorities for flight authorisation.
‘We foresee drone cargo ports on the rooftops of hospitals, integrated as much as possible with the hospital’s logistical system so that transport can be on demand,’ added Geert Vanhandenhove, manager of flight operations at Helicus.
So far, SAFIR-Med has successfully carried out remote virtual demonstrations, simulations, flights controlled from the C2C at test sites, and other tests such as that of a ‘detect-and-avoid’ system to help drones take evasive action when others are flying in the vicinity.
The next step will be to validate the concepts in real-life demonstrations in several countries, including Belgium, Germany and the Netherlands. The trials envisage scenarios including transfers of medical equipment and tissue samples between hospitals and labs, delivery of a defibrillator to treat a cardiac patient outside a hospital, and transport of a physician to an emergency site by passenger drone.
“We foresee drone cargo ports on the rooftops of hospitals.”
– Geert Vanhandenhove, SAFIR-Med
Additional simulations in Greece and the Czech Republic will show the potential for extending such systems across Europe.
SAFIR-Med is part of a wider initiative known as U-space. It’s co-funded by the Single European Sky Air Traffic Management Research (SESAR) Joint Undertaking which is a public-private effort for safer drone operations under the Digital European Sky.
Making rules
Much of the technology is already there for such uses of drones, says Vanhandenhove. However, he highlights that there are regulatory challenges involved in drone flights in cities, especially with larger models flying beyond visual line of sight (BVLOS). This includes authorisations for demonstrations within SAFIR-Med itself.
‘The fact that this is the first time this is being done is posing significant hurdles,’ he said. ‘It will depend on the authorisations granted as to which scenarios can be executed.’
But regulations are set to open up over time, with European Commission rules facilitating a framework for use of BVLOS UAVs in low-level airspace due to come into force next January.
Vanhandenhove emphasises that the development of more robust drone infrastructure will be a gradual process of learning and improvement. Eventually, he hopes that through well-coordinated systems with authorities, emergency flights can be mobilised in seconds in smart cities of the future. ‘For us, it’s very important that we can get an authorisation in sub-minute time,’ he said.
He believes commercial flights could even begin within a couple of years, though it may not be until post-2025 that widely integrated, robust uncrewed medical systems come into play in cities. ‘It’s about making the logistics of delivering whatever medical treatment faster and more efficient, and taking out as much as possible the constraints and limitations that we have on the route,’ said Vanhandenhove.
Research in this article was funded via the EU’s European Research Council.
This article was originally published in Horizon, the EU Research and Innovation magazine.
Controlled Environment Agriculture: A Fertile Landscape for Robot Suppliers
Agricultural engineers design early step for robotic, green-fruit thinning
Following the Path to AGV Safety
The virtuous cycle of AI research
The virtuous cycle of AI research
Roboticists go off-road to compile data that could train self-driving ATVs
Robot dog learns to walk in one hour
Bees’ ‘waggle dance’ may revolutionize how robots talk to each other in disaster zones
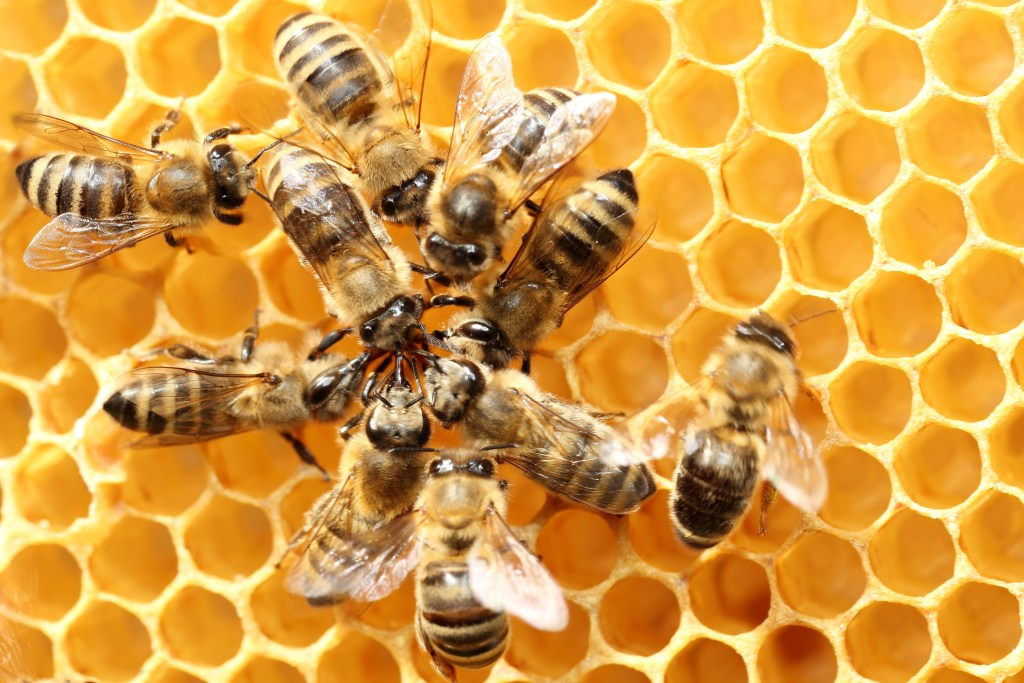
Image credit: rtbilder / Shutterstock.com
By Conn Hastings, science writer
Honeybees use a sophisticated dance to tell their sisters about the location of nearby flowers. This phenomenon forms the inspiration for a form of robot-robot communication that does not rely on digital networks. A recent study presents a simple technique whereby robots view and interpret each other’s movements or a gesture from a human to communicate a geographical location. This approach could prove invaluable when network coverage is unreliable or absent, such as in disaster zones.
Where are those flowers and how far away are they? This is the crux of the ‘waggle dance’ performed by honeybees to alert others to the location of nectar-rich flowers. A new study in Frontiers in Robotics and AI has taken inspiration from this technique to devise a way for robots to communicate. The first robot traces a shape on the floor, and the shape’s orientation and the time it takes to trace it tell the second robot the required direction and distance of travel. The technique could prove invaluable in situations where robot labor is required but network communications are unreliable, such as in a disaster zone or in space.
Honeybees excel at non-verbal communication
If you have ever found yourself in a noisy environment, such as a factory floor, you may have noticed that humans are adept at communicating using gestures. Well, we aren’t the only ones. In fact, honeybees take non-verbal communication to a whole new level.
By wiggling their backside while parading through the hive, they can let other honeybees know about the location of food. The direction of this ‘waggle dance’ lets other bees know the direction of the food with respect to the hive and the sun, and the duration of the dance lets them know how far away it is. It is a simple but effective way to convey complex geographical coordinates.
Applying the dance to robots
This ingenious method of communication inspired the researchers behind this latest study to apply it to the world of robotics. Robot cooperation allows multiple robots to coordinate and complete complex tasks. Typically, robots communicate using digital networks, but what happens when these are unreliable, such as during an emergency or in remote locations? Moreover, how can humans communicate with robots in such a scenario?
To address this, the researchers designed a visual communication system for robots with on-board cameras, using algorithms that allow the robots to interpret what they see. They tested the system using a simple task, where a package in a warehouse needs to be moved. The system allows a human to communicate with a ‘messenger robot’, which supervises and instructs a ‘handling robot’ that performs the task.
Robot dancing in practice
In this situation, the human can communicate with the messenger robot using gestures, such as a raised hand with a closed fist. The robot can recognize the gesture using its on-board camera and skeletal tracking algorithms. Once the human has shown the messenger robot where the package is, it conveys this information to the handling robot.
This involves positioning itself in front of the handling robot and tracing a specific shape on the ground. The orientation of the shape indicates the required direction of travel, while the length of time it takes to trace it indicates the distance. This robot dance would make a worker bee proud, but did it work?
The researchers put it to the test using a computer simulation, and with real robots and human volunteers. The robots interpreted the gestures correctly 90% and 93.3% of the time, respectively, highlighting the potential of the technique.
“This technique could be useful in places where communication network coverage is insufficient and intermittent, such as robot search-and-rescue operations in disaster zones or in robots that undertake space walks,” said Prof Abhra Roy Chowdhury of the Indian Institute of Science, senior author on the study. “This method depends on robot vision through a simple camera, and therefore it is compatible with robots of various sizes and configurations and is scalable,” added Kaustubh Joshi of the University of Maryland, first author on the study.
Video credit: K Joshi and AR Chowdury
This article was originally published on the Frontiers blog.
Why do Policy Gradient Methods work so well in Cooperative MARL? Evidence from Policy Representation
In cooperative multi-agent reinforcement learning (MARL), due to its on-policy nature, policy gradient (PG) methods are typically believed to be less sample efficient than value decomposition (VD) methods, which are off-policy. However, some recent empirical studies demonstrate that with proper input representation and hyper-parameter tuning, multi-agent PG can achieve surprisingly strong performance compared to off-policy VD methods.
Why could PG methods work so well? In this post, we will present concrete analysis to show that in certain scenarios, e.g., environments with a highly multi-modal reward landscape, VD can be problematic and lead to undesired outcomes. By contrast, PG methods with individual policies can converge to an optimal policy in these cases. In addition, PG methods with auto-regressive (AR) policies can learn multi-modal policies.
Figure 1: different policy representation for the 4-player permutation game.
CTDE in Cooperative MARL: VD and PG methods
Centralized training and decentralized execution (CTDE) is a popular framework in cooperative MARL. It leverages global information for more effective training while keeping the representation of individual policies for testing. CTDE can be implemented via value decomposition (VD) or policy gradient (PG), leading to two different types of algorithms.
VD methods learn local Q networks and a mixing function that mixes the local Q networks to a global Q function. The mixing function is usually enforced to satisfy the Individual-Global-Max (IGM) principle, which guarantees the optimal joint action can be computed by greedily choosing the optimal action locally for each agent.
By contrast, PG methods directly apply policy gradient to learn an individual policy and a centralized value function for each agent. The value function takes as its input the global state (e.g., MAPPO) or the concatenation of all the local observations (e.g., MADDPG), for an accurate global value estimate.
The permutation game: a simple counterexample where VD fails
We start our analysis by considering a stateless cooperative game, namely the permutation game. In an -player permutation game, each agent can output
actions
. Agents receive
reward if their actions are mutually different, i.e., the joint action is a permutation over
; otherwise, they receive
reward. Note that there are
symmetric optimal strategies in this game.
Figure 2: the 4-player permutation game.
Let us focus on the 2-player permutation game for our discussion. In this setting, if we apply VD to the game, the global Q-value will factorize to
where and
are local Q-functions,
is the global Q-function, and
is the mixing function that, as required by VD methods, satisfies the IGM principle.
Figure 3: high-level intuition on why VD fails in the 2-player permutation game.
We formally prove that VD cannot represent the payoff of the 2-player permutation game by contradiction. If VD methods were able to represent the payoff, we would have
However, if either of these two agents have different local Q values, e.g. , then according to the IGM principle, we must have
Otherwise, if and
, then
As a result, value decomposition cannot represent the payoff matrix of the 2-player permutation game.
What about PG methods? Individual policies can indeed represent an optimal policy for the permutation game. Moreover, stochastic gradient descent can guarantee PG to converge to one of these optima under mild assumptions. This suggests that, even though PG methods are less popular in MARL compared with VD methods, they can be preferable in certain cases that are common in real-world applications, e.g., games with multiple strategy modalities.
We also remark that in the permutation game, in order to represent an optimal joint policy, each agent must choose distinct actions. Consequently, a successful implementation of PG must ensure that the policies are agent-specific. This can be done by using either individual policies with unshared parameters (referred to as PG-Ind in our paper), or an agent-ID conditioned policy (PG-ID).
PG outperform best VD methods on popular MARL testbeds
Going beyond the simple illustrative example of the permutation game, we extend our study to popular and more realistic MARL benchmarks. In addition to StarCraft Multi-Agent Challenge (SMAC), where the effectiveness of PG and agent-conditioned policy input has been verified, we show new results in Google Research Football (GRF) and multi-player Hanabi Challenge.
Figure 4: (top) winning rates of PG methods on GRF; (bottom) best and average evaluation scores on Hanabi-Full.
In GRF, PG methods outperform the state-of-the-art VD baseline (CDS) in 5 scenarios. Interestingly, we also notice that individual policies (PG-Ind) without parameter sharing achieve comparable, sometimes even higher winning rates, compared to agent-specific policies (PG-ID) in all 5 scenarios. We evaluate PG-ID in the full-scale Hanabi game with varying numbers of players (2-5 players) and compare them to SAD, a strong off-policy Q-learning variant in Hanabi, and Value Decomposition Networks (VDN). As demonstrated in the above table, PG-ID is able to produce results comparable to or better than the best and average rewards achieved by SAD and VDN with varying numbers of players using the same number of environment steps.
Beyond higher rewards: learning multi-modal behavior via auto-regressive policy modeling
Besides learning higher rewards, we also study how to learn multi-modal policies in cooperative MARL. Let’s go back to the permutation game. Although we have proved that PG can effectively learn an optimal policy, the strategy mode that it finally reaches can highly depend on the policy initialization. Thus, a natural question will be:
Can we learn a single policy that can cover all the optimal modes?
In the decentralized PG formulation, the factorized representation of a joint policy can only represent one particular mode. Therefore, we propose an enhanced way to parameterize the policies for stronger expressiveness — the auto-regressive (AR) policies.
Figure 5: comparison between individual policies (PG) and auto-regressive policies (AR) in the 4-player permutation game.
Formally, we factorize the joint policy of agents into the form of
where the action produced by agent depends on its own observation
and all the actions from previous agents
. The auto-regressive factorization can represent any joint policy in a centralized MDP. The only modification to each agent’s policy is the input dimension, which is slightly enlarged by including previous actions; and the output dimension of each agent’s policy remains unchanged.
With such a minimal parameterization overhead, AR policy substantially improves the representation power of PG methods. We remark that PG with AR policy (PG-AR) can simultaneously represent all optimal policy modes in the permutation game.
Figure: the heatmaps of actions for policies learned by PG-Ind (left) and PG-AR (middle), and the heatmap for rewards (right); while PG-Ind only converge to a specific mode in the 4-player permutation game, PG-AR successfully discovers all the optimal modes.
In more complex environments, including SMAC and GRF, PG-AR can learn interesting emergent behaviors that require strong intra-agent coordination that may never be learned by PG-Ind.
Figure 6: (top) emergent behavior induced by PG-AR in SMAC and GRF. On the 2m_vs_1z map of SMAC, the marines keep standing and attack alternately while ensuring there is only one attacking marine at each timestep; (bottom) in the academy_3_vs_1_with_keeper scenario of GRF, agents learn a “Tiki-Taka” style behavior: each player keeps passing the ball to their teammates.
Discussions and Takeaways
In this post, we provide a concrete analysis of VD and PG methods in cooperative MARL. First, we reveal the limitation on the expressiveness of popular VD methods, showing that they could not represent optimal policies even in a simple permutation game. By contrast, we show that PG methods are provably more expressive. We empirically verify the expressiveness advantage of PG on popular MARL testbeds, including SMAC, GRF, and Hanabi Challenge. We hope the insights from this work could benefit the community towards more general and more powerful cooperative MARL algorithms in the future.
This post is based on our paper in joint with Zelai Xu: Revisiting Some Common Practices in Cooperative Multi-Agent Reinforcement Learning (paper, website).