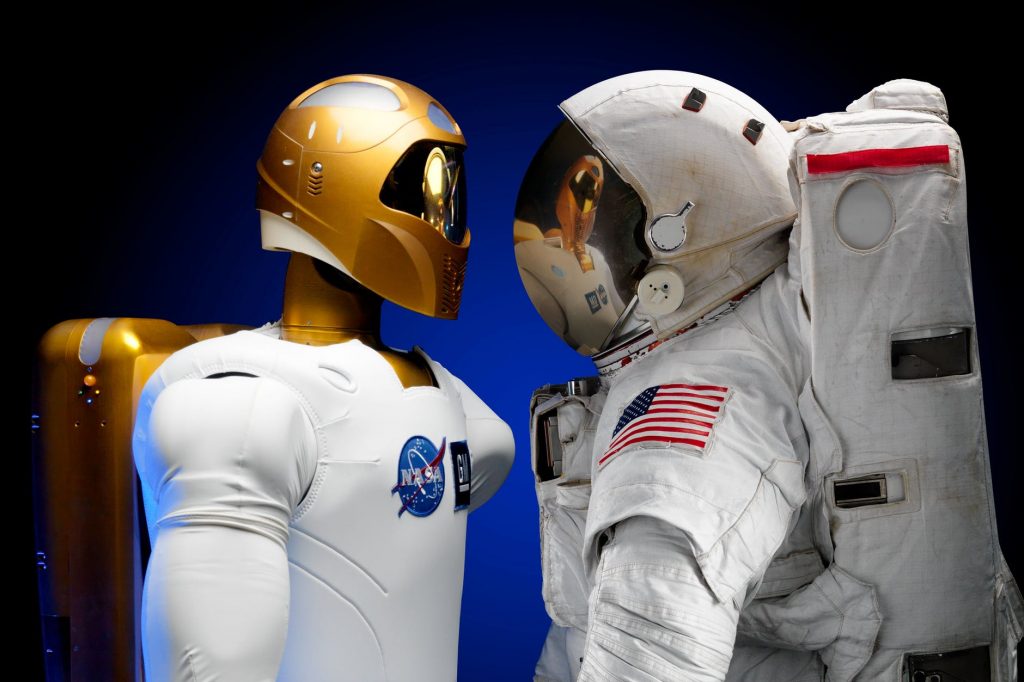
As part of her role as one of the IEEE ICRA 2022 Science Communication Awardees, Avie Ravendran sat down virtually with a few researchers from academia and industry attending the conference. Curious about what they had to say? Read their quotes below!
“I really believe that learned methods, especially imitation and transfer learning, will enable scalable robot applications in human and unstructured environments We’re on the cusp of seeing robot agents dynamically adapt and solve real world problems”
– Nicholas Nadeau, CTO, Halodi Robotics
“On one hand I think that the interplay of perception and control is quite exciting, in terms of the common underlying principles, while on the other, it’s both cool and inspiring to see more robots getting out of the lab”
– Matías Mattamala, PhD Student, Oxford Dynamic Robot Systems, Oxford Robotics Institute
“I believe that incorporating priors regarding the existing scene geometry and the temporal consistency that’s present in the context of mobile robotics, can be used to guide the learning of more robust representations”
– Kavisha Vidanapathirana, QUT & CSIRORobotics
“At the moment, I am aiming to find out what researchers need in order to take care of their motivation and wellbeing”
– Daniel Carrillo-Zapata, Founder, Scientific Agitation
“We have an immense amount of unsupervised knowledge and we’re always updating our priors. Taking advantage of large-scale unsupervised pretraining and having a lifelong learning system seems like a significant step in the right direction”
– Nitish Dashora, Researcher, Berkeley AI Research & Redwood Center for Theoretical Neuroscience
“When objects are in clutter, with various objects lying on top of one another, the robot needs to interactively and autonomously rearrange the scene in order to retrieve the pose of the target object with minimal number of actions to achieve overall efficiency. I work on pose estimation algorithms to process dense visual data as well as sparse tactile data”
– Prajval Kumar, BMW & University of Glasgow
“Thinking of why the robots or even the structures behave the way they do, and framing and answering questions in that line satisfies my curiosity as a researcher”
– Tung Ta, Postdoctoral Researcher, The University of Tokyo
“I sometimes hear that legged locomotion is a solved problem, but I disagree. I think that the standards of performance have just been raised and collectively we can now tackle more dynamic, efficient and reliable gaits”
– Kevin Green, PhD Candidate, Oregon State University
“My goal in robotics research is to bring down the cost and improve the capabilities of marine research platforms by introducing modularity and underactuation into the field. We’re working on understanding how to bring our collective swimming technology into flowing environments now”
– Gedaliah Knizhnik, PhD Candidate, GRASP Laboratory & The modular robotics laboratory, University of Pennsylvania
“I am interested in how we can develop the algorithms and representations needed to enable long-term autonomous robot navigation without human intervention, such as in the case of an autonomous underwater robot persistently mapping a marine ecosystem for an extended period of time. There are lots of challenges like how can we build a compact representation of the world, ideally grounded in human-understandable semantics? How can we deal gracefully with outliers in perception that inevitably occur in the lifelong setting? and also how can we scale robot state estimation methods in time and space while bounding memory and computation requirements?”
– Kevin Doherty, Computer Science and AI Lab, MIT & Woods Hole Oceanographic Institution
“How can robots learn to interact with and reason about themselves and the world without an intuitive feel for either? Communication is at the heart of biological and robotic systems. Inspired by control theory, information theory, and neuroscience, early work in artificial intelligence (AI) and robotics focused on a class of dynamical system known as feedback systems. These systems are characterized by recurrent mechanisms or feedback loops that govern, regulate, or ‘steer’ the behaviour of the system toward desirable stable states in the presence of disturbance in diverse environments. Feedback between sensation, prediction, decision, action, and back is a critical component of sensorimotor learning needed to realize robust intelligent robotic systems in the wild, a grand challenge of the field. Existing robots are fundamentally numb to the world, limiting their ability to sense themselves and their environment. This problem will only increase as robots grow in complexity, dexterity, and maneuverability, guided by biomimicry. Feedback control systems such as the proportional integral derivative (PID), reinforcement learning (RL), and model predictive control (MPC) are now common in robotics, as is (optimal, Bayesian) Kálmán filtering of point-based IMU-GPS signals. Lacking are the distributed multi-modal, high-dimensional sensations needed to realize general intelligent behaviour, executing complex action sequences through high-level abstractions built up from an intuitive feel or understanding of physics.While the central nervous system and biological neural networks are quantum parallel distributed processing (PDP) engines, most digital artificial neural networks are fully decoupled from sensors and provide only a passive image of the world. We are working to change that by coupling parallel distributed sensing and data processing through a neural paradigm. This involves innovations in hardware, software, and datasets. At Nervosys, we aim to make this dream a reality by building the first nervous system and platform for general robotic intelligence.”
– Adam Erickson, Founder, Nervosys