New jumping device achieves the tallest height of any known jumper, engineered or biological
Electronic skin anticipates and perceives touch from different directions for the first time
A newcomer’s guide to #ICRA2022: A primer
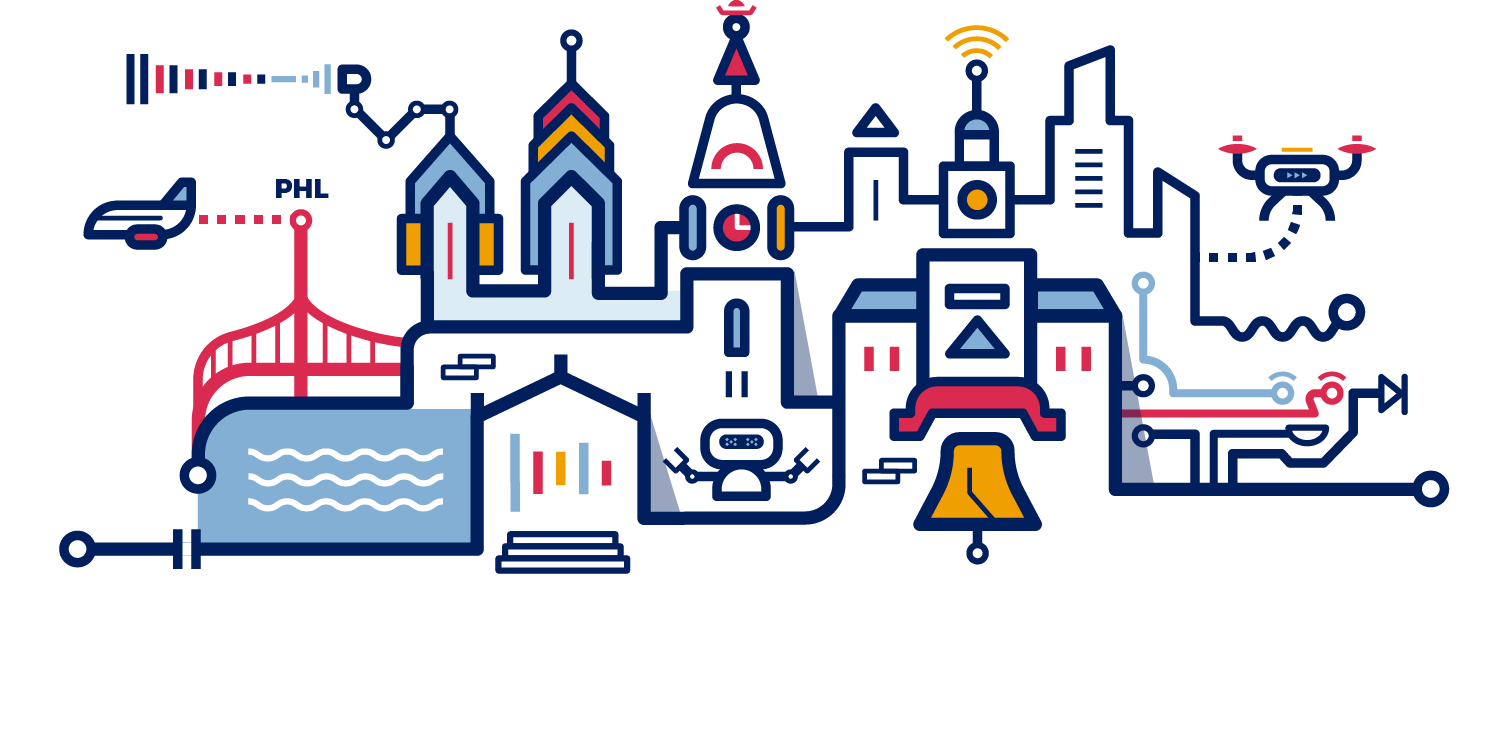
Dear robotics graduate students and newcomers to robotics,
If you are what I imagine delving into robotics to be like today, the majority of your time is spent as follows:
- navigating Slack channels while tuning into some online lectures,
- trying to figure out whether you should be reading more papers, coding more, or if you are just a slow reader/coder/etc,
- and if you are a grad student, in particular, having a never-ending cycle of self-doubt questions that seem super important, such as “what is a research question anyway? And how do you find one that no one has tackled before — and not because it’s a dumb question? Oh wait… is my question dumb? Can I find out without asking my prof?”
Much of the questions I had as a grad student stemmed from my lack of knowledge about what is considered to be normal by academia, the robotics community, and more narrowly the subdomain of robotics I belonged to. The friends/colleagues/people I met along the way helped me fill that much-needed knowledge gap about the norm because many of us were on the same journey with similar struggles/questions.
As an assistant professor who spent the majority of my professorship in the COVID-19 pandemic mode, I worry that my students’ grad school journey has not offered the same kind of shared experience and camaraderie with people in the domain that I am now seeing the huge benefit of.
The upcoming IEEE International Conference on Robotics and Automation 2022 will be the first robotics conference that many of you attend in-person since the pandemic (I’m in this category). For many of you, it may be your first time attending an academic conference. For even more of you, this may be your first virtual attendance at ICRA.
ICRA is a multi-track, full-week of robotics festivity that draws in thousands. It can pass by you in a blink.
So, in a series of short blog posts (because, who has the time these days), I am going to highlight a few things in the form of a millennial’s guide to ICRA.
I’m assuming that you, the reader, may be as impatient a reader as I am, who likes information presented in a short, snappy, and organized way. The more bullet points the better.
So let’s get started.
** Full disclosure, I’m one of the two publicity co-chairs for the ICRA conference. If you want to be on the grounds of ICRA as a student science communicator, reach out to us. **
Why Are You Still Manually Programming Your Robot?
The ALLOMAN hexapod robot is a novel multifunctional platform with leg-arm integration
Scientists to develop electronic noses to track down body odors
A robot called Lyra is helping transform nuclear infrastructure inspection
Microrobot collectives display versatile movement patterns
A model to improve robots’ ability to hand over objects to humans
Medical Exoskeleton for Rehabilitation
An easier way to teach robots new skills
Working With Robots With Different Levels of Collaboration
DeepMind’s latest research at ICLR 2022
MimicEducationalRobots teach robotics for the future
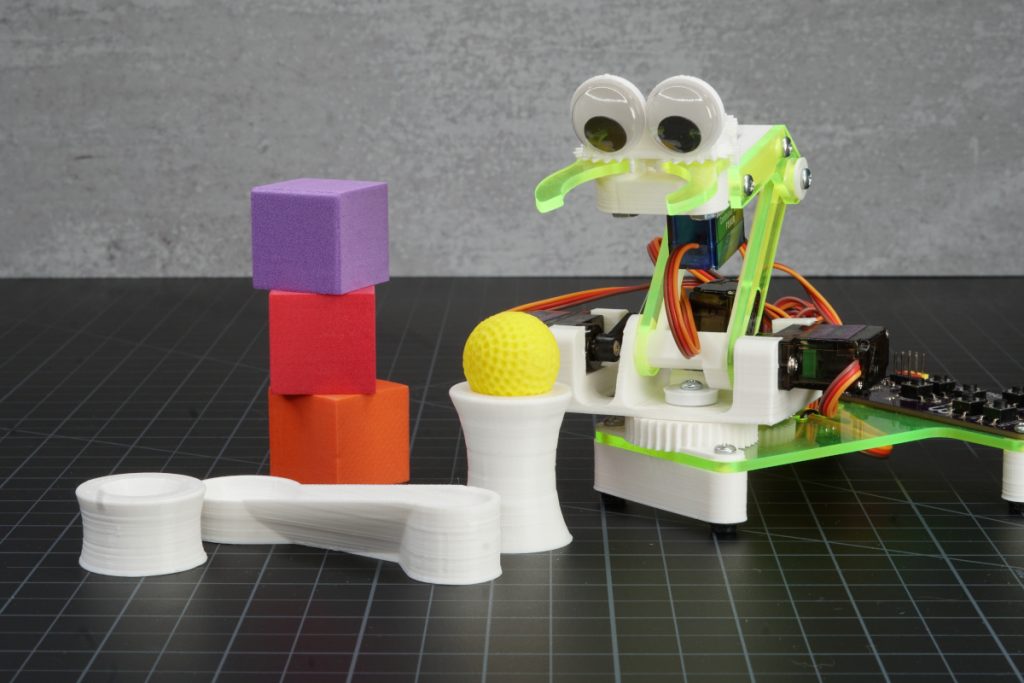
The robotics industry is changing. The days of industrial robot arms working behind enclosures, performing pre-programmed identical tasks are coming to an end. Robots that can interact with each other and other equipment are becoming standard and robots are expanding to more aspects of our lives. My name is Brett Pipitone, and I am the founder, CEO, and sole employee of mimicEducationalRobots. I believe that robots will soon become an inescapable part of modern life, and I seek to prepare today’s students to work with these emerging technologies.
The mimicEducationalRobots product line consists of a family of three robots. The largest and most sophisticated is mimicArm. The adorable tinyBot is small and capable. The newest robot, bitsyBot (currently on Kickstarter) is perfect for those taking the first steps into the robotics world. Despite having different features, all three robots are designed to communicate with each other and use special sensors to make decisions about their environment. Interfaces are simple but powerful, allowing users to learn quickly and without frustration.
mimicEducationalRobots believes that every student will encounter robots in their everyday life, no matter their career path. Learning robotics allows students to become comfortable and familiar with technology that is rapidly becoming commonplace in day-to-day life. Through their combinations of features, the mimicEducationalRobots products introduce technology that’s still in its infancy, such as human/robot interaction and cooperative robotics, at a level students can understand. This is why every mimicEducationalRobots robot starts with manual control, allowing students to get the feel of their robot and what it can and can’t do. Once they’ve mastered manual control programming is a smaller leap. The mimicEducationalRobots programming software simplifies this transition by reflecting the same motions the students have been making manually with simple commands like “robotMove” and “robotGrab”.
For more complex programs, mimicEducationalRobots believes that their robots should mimic industry as closely as possible. This means doing as much as possible with the simplest possible sensor. Things start small, with a great big tempting pushable button called, incidentally, “greatBigButton”. This is the students’ first introduction to human interaction as they program their robot to react to a button press. From there things get much more exciting without getting very much more complicated. For example, an array of non-contact IR thermometers called Grid-EYE allows mimicArm to detect faces using nothing but body heat. A simple IR proximity sensor allows tinyBot or bitsyBot to react when offered a block before the block touches any part of the robot. There’s even a cable that allows robots to communicate with each other and react to what the other is doing. These simple capabilities allow students to create a wide range of robotic behaviors.
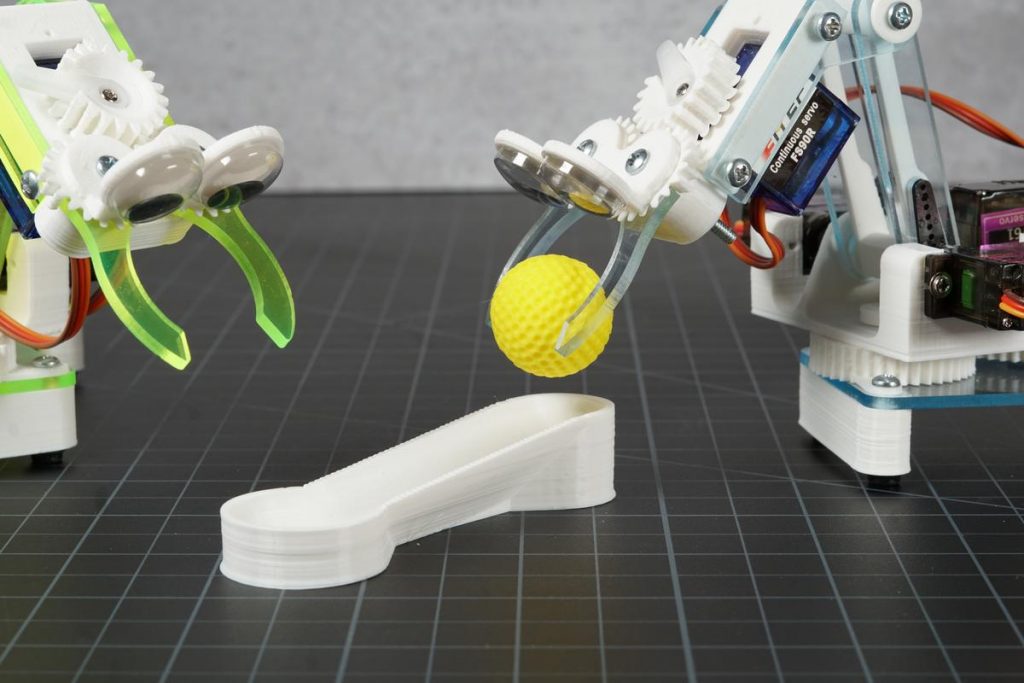
mimicEducationalRobots is a homegrown business designed and built by an engineer and dad passionate about teaching people of all ages about robotics. I created the robots’ brains using a bare circuit board, template, some solder paste, and tweezers. Every component is added by hand and the board is soldered together with a toaster oven I modified. Once cooled the boards are programmed using a modified Arduino UNO R3, one of the best technological tools for beginners.
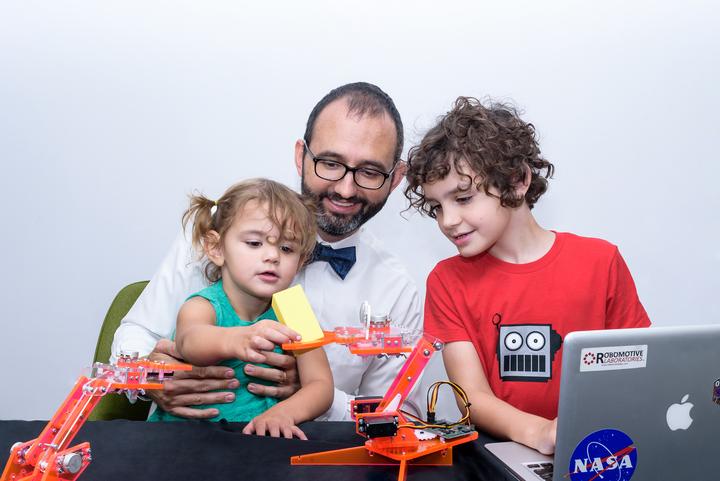
Other physical robot parts are designed using 3D modeling software and made either on a 3D printer or CNC router. I have two 3D printers in his basement running 24 hours a day, producing at least 20 robot kits a week. The CNC router requires a great deal more supervision but is capable of turning out four sets of beautiful neon plastic parts every 30 minutes.
mimicEducationalRobots is a new kind of company, producing a new kind of product, for a new kind of consumer. Their products demonstrate just how fundamental technology, and in particular open source technology, has changed our world. I hope students learning on mimicArm, tinyBot, or bitsyBot will help create the next life-changing technological leap.
To learn more about the family of mimicEducationalRobots visit www.mimicRobots.com