It is Robotics part of AI? Is AI part of robotics? What is the difference between the two terms? We answer this fundamental question.
Robotics and artificial intelligence (AI) serve very different purposes. However, people often get them mixed up.
A lot of people wonder if robotics is a subset of artificial intelligence. Others wonder if they are the same thing.
Since the first version of this article, which we published back in 2017, the question has gotten even more confusing. The rise in the use of the word "robot" in recent years to mean any sort of automation has cast even more doubt on how robotics and AI fit together (more on this at the end of the article).
It's time to put things straight once and for all.
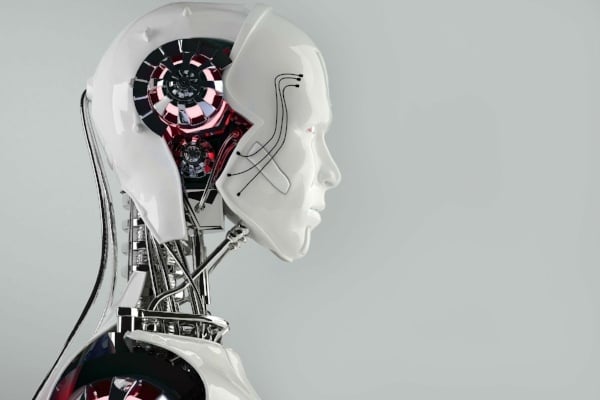
Are robotics and artificial intelligence the same thing?
The first thing to clarify is that robotics and artificial intelligence are not the same things at all. In fact, the two fields are almost entirely separate.
A Venn diagram of the two fields would look like this:
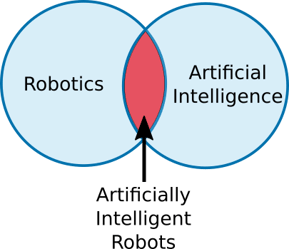
As you can see, there is one area small where the two fields overlap: Artificially Intelligent Robots. It is within this overlap that people sometimes confuse the two concepts.
To understand how these three terms relate to each other, let's look at each of them individually.
What is robotics?
Robotics is a branch of technology that deals with physical robots. Robots are programmable machines that are usually able to carry out a series of actions autonomously, or semi-autonomously.
In my opinion, there are three important factors which constitute a robot:
- Robots interact with the physical world via sensors and actuators.
- Robots are programmable.
- Robots are usually autonomous or semi-autonomous.
I say that robots are "usually" autonomous because some robots aren't. Telerobots, for example, are entirely controlled by a human operator but telerobotics is still classed as a branch of robotics. This is one example where the definition of robotics is not very clear.
It is surprisingly difficult to get experts to agree on exactly what constitutes a "robot." Some people say that a robot must be able to "think" and make decisions. However, there is no standard definition of "robot thinking." Requiring a robot to "think" suggests that it has some level of artificial intelligence but the many non-intelligent robots that exist show that thinking cannot be a requirement for a robot.
However you choose to define a robot, robotics involves designing, building and programming physical robots which are able to interact with the physical world. Only a small part of robotics involves artificial intelligence.
Example of a robot: Basic cobot
A simple collaborative robot (cobot) is a perfect example of a non-intelligent robot.
For example, you can easily program a cobot to pick up an object and place it elsewhere. The cobot will then continue to pick and place objects in exactly the same way until you turn it off. This is an autonomous function because the robot does not require any human input after it has been programmed. The task does not require any intelligence because the cobot will never change what it is doing.
Most industrial robots are non-intelligent.
What is artificial intelligence?
Artificial intelligence (AI) is a branch of computer science. It involves developing computer programs to complete tasks that would otherwise require human intelligence. AI algorithms can tackle learning, perception, problem-solving, language-understanding and/or logical reasoning.
AI is used in many ways within the modern world. For example, AI algorithms are used in Google searches, Amazon's recommendation engine, and GPS route finders. Most AI programs are not used to control robots.
Even when AI is used to control robots, the AI algorithms are only part of the larger robotic system, which also includes sensors, actuators, and non-AI programming.
Often — but not always — AI involves some level of machine learning, where an algorithm is "trained" to respond to a particular input in a certain way by using known inputs and outputs. We discuss machine learning in our article Robot Vision vs Computer Vision: What's the Difference?
The key aspect that differentiates AI from more conventional programming is the word "intelligence." Non-AI programs simply carry out a defined sequence of instructions. AI programs mimic some level of human intelligence.
Example of a pure AI: AlphaGo
One of the most common examples of pure AI can be found in games. The classic example of this is chess, where the AI Deep Blue beat world champion, Gary Kasparov, in 1997.
A more recent example is AlphaGo, an AI which beat Lee Sedol the world champion Go player, in 2016. There were no robotic elements to AlphaGo. The playing pieces were moved by a human who watched the robot's moves on a screen.
What are Artificially Intelligent Robots?
Artificially intelligent robots are the bridge between robotics and AI. These are robots that are controlled by AI programs.
Most robots are not artificially intelligent. Up until quite recently, all industrial robots could only be programmed to carry out a repetitive series of movements which, as we have discussed, do not require artificial intelligence. However, non-intelligent robots are quite limited in their functionality.
AI algorithms are necessary when you want to allow the robot to perform more complex tasks.
A warehousing robot might use a path-finding algorithm to navigate around the warehouse. A drone might use autonomous navigation to return home when it is about to run out of battery. A self-driving car might use a combination of AI algorithms to detect and avoid potential hazards on the road. These are all examples of artificially intelligent robots.
Example: Artificially intelligent cobot
You could extend the capabilities of a collaborative robot by using AI.
Imagine you wanted to add a camera to your cobot. Robot vision comes under the category of "perception" and usually requires AI algorithms.
Say that you wanted the cobot to detect the object it was picking up and place it in a different location depending on the type of object. This would involve training a specialized vision program to recognize the different types of objects. One way to do this is by using an AI algorithm called Template Matching, which we discuss in our article How Template Matching Works in Robot Vision.
In general, most artificially intelligent robots only use AI in one particular aspect of their operation. In our example, AI is only used in object detection. The robot's movements are not really controlled by AI (though the output of the object detector does influence its movements).
Where it all gets confusing…
As you can see, robotics and artificial intelligence are really two separate things.
Robotics involves building robots physical whereas AI involves programming intelligence.
However, there is one area where everything has got rather confusing since I first wrote this article: software robots.
Why software robots are not robots
The term "software robot" refers to a type of computer program which autonomously operates to complete a virtual task. Examples include:
- Search engine "bots" — aka "web crawlers." These roam the internet, scanning websites and categorizing them for search.
- Robotic Process Automation (RPA) — These have somewhat hijacked the word "robot" in the past few years, as I explained in this article.
- Chatbots — These are the programs that pop up on websites talk to you with a set of pre-written responses.
Software bots are not physical robots they only exist within a computer. Therefore, they are not real robots.
Some advanced software robots may even include AI algorithms. However, software robots are not part of robotics.
Hopefully, this has clarified everything for you. But, if you have any questions at all please ask them in the comments.