Why AMRs Are Being Adopted On Factory Floors
Exploring ROS2 using wheeled Robot – #3 – Moving the robot
By Marco Arruda
In this post you’ll learn how to publish to a ROS2 topic using ROS2 C++. Up to the end of the video, we are moving the robot Dolly robot, simulated using Gazebo 11.
You’ll learn:
- How to create a node with ROS2 and C++
- How to public to a topic with ROS2 and C++
1 – Setup environment – Launch simulation
Before anything else, make sure you have the rosject from the previous post, you can copy it from here.
Launch the simulation in one webshell and in a different tab, checkout the topics we have available. You must get something similar to the image below:
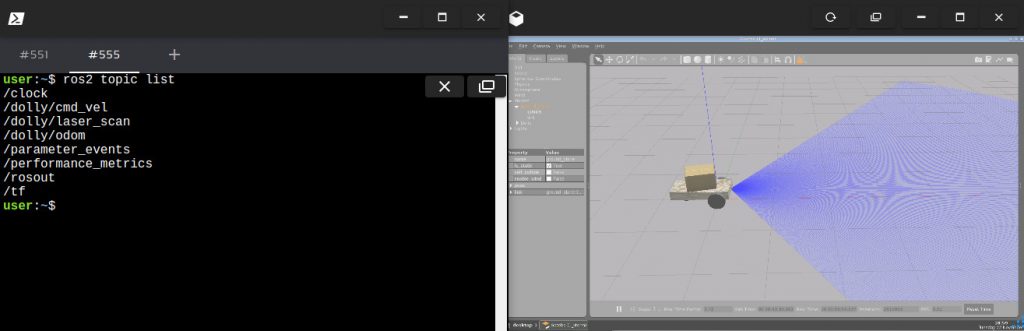
2 – Create a topic publisher
Create a new file to container the publisher node: moving_robot.cpp and paste the following content:
#include <chrono> #include <functional> #include <memory> #include "rclcpp/rclcpp.hpp" #include "geometry_msgs/msg/twist.hpp" using namespace std::chrono_literals; /* This example creates a subclass of Node and uses std::bind() to register a * member function as a callback from the timer. */ class MovingRobot : public rclcpp::Node { public: MovingRobot() : Node("moving_robot"), count_(0) { publisher_ = this->create_publisher("/dolly/cmd_vel", 10); timer_ = this->create_wall_timer( 500ms, std::bind(&MovingRobot::timer_callback, this)); } private: void timer_callback() { auto message = geometry_msgs::msg::Twist(); message.linear.x = 0.5; message.angular.z = 0.3; RCLCPP_INFO(this->get_logger(), "Publishing: '%f.2' and %f.2", message.linear.x, message.angular.z); publisher_->publish(message); } rclcpp::TimerBase::SharedPtr timer_; rclcpp::Publisher::SharedPtr publisher_; size_t count_; }; int main(int argc, char *argv[]) { rclcpp::init(argc, argv); rclcpp::spin(std::make_shared()); rclcpp::shutdown(); return 0; }QoS (Quality of Service)
Similar to the subscriber it is created a class that inherits Node. A publisher_ is setup and also a callback, although this time is not a callback that receives messages, but a timer_callback called in a frequency defined by the timer_ variable. This callback is used to publish messages to the robot.
The create_publisher method needs two arguments:
- topic name
- QoS (Quality of Service) – This is the policy of data saved in the queue. You can make use of different middlewares or even use some provided by default. We are just setting up a queue of 10. By default, it keeps the last 10 messages sent to the topic.
The message published must be created using the class imported:
message = geometry_msgs::msg::Twist();
We ensure the callback methods on the subscribers side will always recognize the message. This is the way it has to be published by using the publisher method publish.
3 – Compile and run the node
In order to compile we need to adjust some things in the ~/ros2_ws/src/my_package/CMakeLists.txt. So add the following to the file:
- Add the geometry_msgs dependency
- Append the executable moving_robot
- Add install instruction for moving_robot
find_package(geometry_msgs REQUIRED) ... # moving robot add_executable(moving_robot src/moving_robot.cpp) ament_target_dependencies(moving_robot rclcpp geometry_msgs) ... install(TARGETS moving_robot reading_laser DESTINATION lib/${PROJECT_NAME}/ )
We can run the node like below:
source ~/ros2_ws/install/setup.bash
ros2 run my_package

Related courses & extra links:
The post Exploring ROS2 using wheeled Robot – #3 – Moving the Robot
appeared first on The Construct.
Exploring ROS2 using wheeled Robot – #3 – Moving the robot
By Marco Arruda
In this post you’ll learn how to publish to a ROS2 topic using ROS2 C++. Up to the end of the video, we are moving the robot Dolly robot, simulated using Gazebo 11.
You’ll learn:
- How to create a node with ROS2 and C++
- How to public to a topic with ROS2 and C++
1 – Setup environment – Launch simulation
Before anything else, make sure you have the rosject from the previous post, you can copy it from here.
Launch the simulation in one webshell and in a different tab, checkout the topics we have available. You must get something similar to the image below:
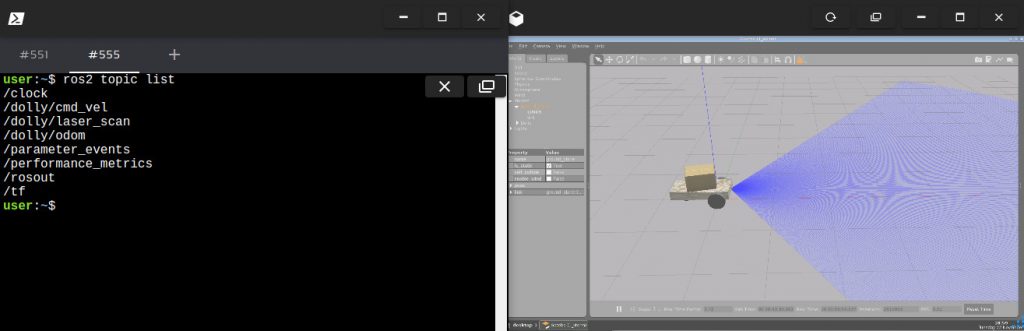
2 – Create a topic publisher
Create a new file to container the publisher node: moving_robot.cpp and paste the following content:
#include <chrono> #include <functional> #include <memory> #include "rclcpp/rclcpp.hpp" #include "geometry_msgs/msg/twist.hpp" using namespace std::chrono_literals; /* This example creates a subclass of Node and uses std::bind() to register a * member function as a callback from the timer. */ class MovingRobot : public rclcpp::Node { public: MovingRobot() : Node("moving_robot"), count_(0) { publisher_ = this->create_publisher("/dolly/cmd_vel", 10); timer_ = this->create_wall_timer( 500ms, std::bind(&MovingRobot::timer_callback, this)); } private: void timer_callback() { auto message = geometry_msgs::msg::Twist(); message.linear.x = 0.5; message.angular.z = 0.3; RCLCPP_INFO(this->get_logger(), "Publishing: '%f.2' and %f.2", message.linear.x, message.angular.z); publisher_->publish(message); } rclcpp::TimerBase::SharedPtr timer_; rclcpp::Publisher::SharedPtr publisher_; size_t count_; }; int main(int argc, char *argv[]) { rclcpp::init(argc, argv); rclcpp::spin(std::make_shared()); rclcpp::shutdown(); return 0; }QoS (Quality of Service)
Similar to the subscriber it is created a class that inherits Node. A publisher_ is setup and also a callback, although this time is not a callback that receives messages, but a timer_callback called in a frequency defined by the timer_ variable. This callback is used to publish messages to the robot.
The create_publisher method needs two arguments:
- topic name
- QoS (Quality of Service) – This is the policy of data saved in the queue. You can make use of different middlewares or even use some provided by default. We are just setting up a queue of 10. By default, it keeps the last 10 messages sent to the topic.
The message published must be created using the class imported:
message = geometry_msgs::msg::Twist();
We ensure the callback methods on the subscribers side will always recognize the message. This is the way it has to be published by using the publisher method publish.
3 – Compile and run the node
In order to compile we need to adjust some things in the ~/ros2_ws/src/my_package/CMakeLists.txt. So add the following to the file:
- Add the geometry_msgs dependency
- Append the executable moving_robot
- Add install instruction for moving_robot
find_package(geometry_msgs REQUIRED) ... # moving robot add_executable(moving_robot src/moving_robot.cpp) ament_target_dependencies(moving_robot rclcpp geometry_msgs) ... install(TARGETS moving_robot reading_laser DESTINATION lib/${PROJECT_NAME}/ )
We can run the node like below:
source ~/ros2_ws/install/setup.bash
ros2 run my_package

Related courses & extra links:
The post Exploring ROS2 using wheeled Robot – #3 – Moving the Robot
appeared first on The Construct.
NeatNet: A model that can learn people’s tidying up preferences
Honda Tests Prototype Autonomous Work Vehicle at Solar Construction Site with Black & Veatch
Xenobots: Team builds first living robots that can reproduce
Machine-learning model could enable robots to understand interactions in the way humans do
New software allows industrial robots to achieve touch sensitivity and precision close to human hands
An inventory of robotics roadmaps to better inform policy and investment
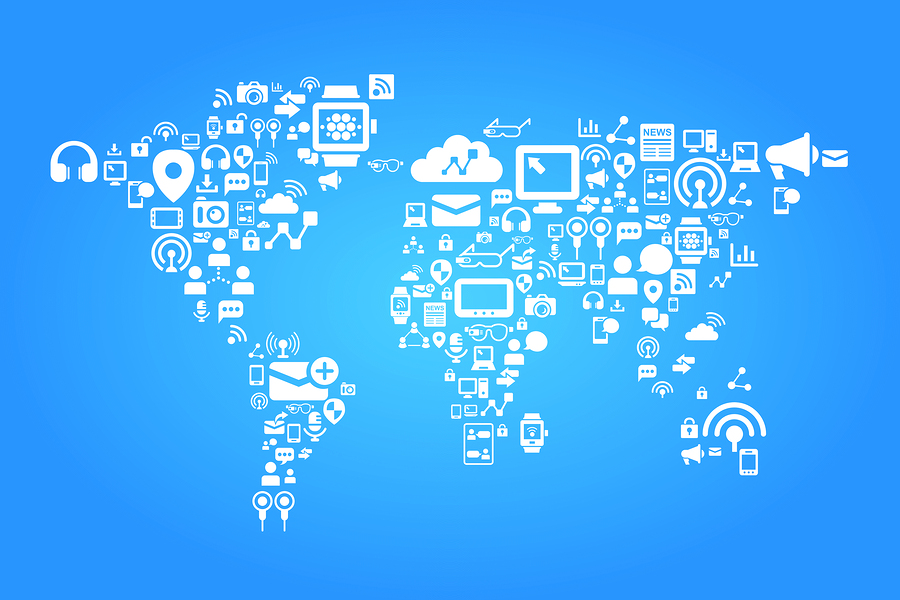
Much excellent work has been done, by many organizations, to develop ‘Roadmaps for Robotics’, in order to steer government policy, innovation investment, and the development of standards and commercialization. However, unless you took part in the roadmapping activity, it can be very hard to find these resources. Silicon Valley Robotics in partnership with the Industrial Activities Board of the IEEE Robotics and Automation Society, is compiling an up to date resource list of various robotics, AIS and AI roadmaps, national or otherwise. This initiative will allow us all to access the best robotics commercialization advice from around the world, to be able to compare and contrast various initiatives and their regional effectiveness, and to provide guidance for countries and companies without their own robotics roadmaps.
Another issue making it harder to find recent robotics roadmaps is the subsumption of robotics into the AI landscape, at least in some national directives. Or it may appear not as robotics but as ‘AIS’, standing for Autonomous Intelligent Systems, such as in the work of OCEANIS, the Open Community for Ethics in Autonomous aNd Intelligent Systems, which hosts a global standards repository. And finally there are subcategories of robotics, ie Autonomous Vehicles, or Self Driving Cars, or Drones, or Surgical Robotics, all of which may have their own roadmaps. This is not an exhaustive list, but with your help we can continue to evolve it.
-
Global Roadmaps:
- IEEE RAS 2050Robotics.org
- OCEANIS, Open Community for Ethics of Autonomous and Intelligent Systems
- OCEANIS, Global Standards Repository
- OECD.ai Policy Observatory
- OECD.ai Stakeholder Initiatives
- OECD.ai National AI Strategies
- Mapping the Evolution of the Robotics Industry: A Cross-Country Comparison
- BCG Robotics Outlook 2030
- 2021 World Bank Review of National AI Strategies and Policies
-
US Roadmaps
- 2020 US National Robotics Roadmap
- ASME: Robotics & Covid 19
- ASME: Accelerating US Robotics for American Prosperity and Security
- 2020 NASA Technology Taxonomy
- 2017 Automated Vehicles 3.0: Preparing for the Future of Transportation
- NITRD Supplement to the President’s FY2021 Budget
- American AI Initiative
-
Canada Roadmaps
-
Latam Roadmaps
- AI for Social Good in Latin America and the Caribbean
- Mexico Agenda National for Artificial Intelligence
- Brazil Industry 4.0
- Brazil E-Digital
- Colombia National Policy for Digital Transformation and Artificial Intelligence
- Chile National Artificial Intelligence Policy
- Argentina Agenda Digital 2030
- Uruguay Artificial Intelligence Strategy for the Public Administration
-
UK & EU Roadmaps
- AI in the UK: No Room for Complacency
- UK’s Industrial Strategy
- SPARC: Robotics 2020 Multi-Annual Roadmap for Robotics in Europe
- SRIDA: AI, Data and Robotics Partnership
- EC: Communication on Artificial Intelligence
- Sweden National Approach for Artificial Intelligence
- Spain RDI Strategy in Artificial Intelligence
- Strategy for the Development of Artificial Intelligence in the Republic of Serbia for the period 2020-2025
- AI Portugal 2030
- Assumptions for the AI strategy in Poland
- Norway National Strategy for Artificial Intelligence
- The Declaration on AI in the Nordic-Baltic Region
- Malta the Ultimate AI Launchpad: A Strategy and Vision for Artificial Intelligence in Malta 2030
- Artificial Intelligence: a strategic vision for Luxembourg
- Lithuanian Artificial Intelligence Strategy: A vision of the future
- Italy National Strategy on Artificial Intelligence
- Made in Germany: Artificial Intelligence Strategy
- Meaningful Artificial Intelligence: Towards a French and European Strategy
- Finland’s Age of Artificial Intelligence
- Finland Leading the Way into the Age of Artificial Intelligence
- Denmark National Strategy for Artificial Intelligence
- Czech National Artificial Intelligence Strategy
- Cyprus National Strategy AI
- AI 4 Belgium
- Austria AIM AT 2030
-
Russia Roadmaps
-
Africa & MENA Roadmaps
- PIDA Closing the Infrastructure Gap Vital for Africa’s Transformation
- Africa50
- AIDA Accelerated Industrial Development for Africa
- UAE Strategy for Artificial Intelligence
- UAE Artificial Intelligence Strategy 2031
- Saudi Arabia Vision 2030
- National AI Strategy: Unlocking Tunisia’s capabilities potential
-
Asia & SE Asia Roadmaps
-
China Roadmaps
-
Japan Roadmaps
-
Australia Roadmaps
-
New Zealand & Pacifica Roadmaps
-
Non Terrestrial Roadmaps
Do you know of robotics roadmaps not yet included? Please share them with us.