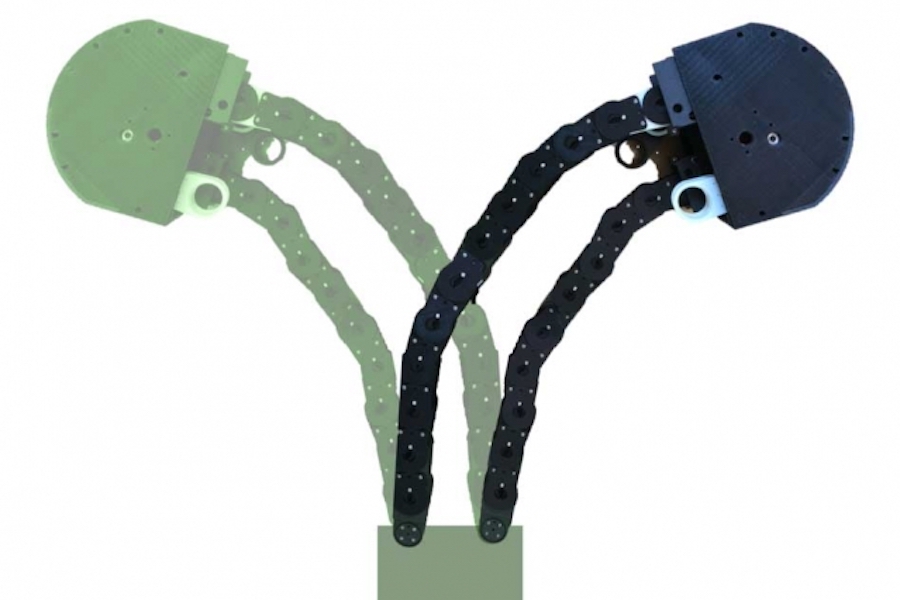
The new “growing robot” can be programmed to grow, or extend, in different directions, based on the sequence of chain units that are locked and fed out from the “growing tip,” or gearbox.
Image courtesy of researchers, edited by MIT News
In today’s factories and warehouses, it’s not uncommon to see robots whizzing about, shuttling items or tools from one station to another. For the most part, robots navigate pretty easily across open layouts. But they have a much harder time winding through narrow spaces to carry out tasks such as reaching for a product at the back of a cluttered shelf, or snaking around a car’s engine parts to unscrew an oil cap.
Now MIT engineers have developed a robot designed to extend a chain-like appendage flexible enough to twist and turn in any necessary configuration, yet rigid enough to support heavy loads or apply torque to assemble parts in tight spaces. When the task is complete, the robot can retract the appendage and extend it again, at a different length and shape, to suit the next task.
The appendage design is inspired by the way plants grow, which involves the transport of nutrients, in a fluidized form, up to the plant’s tip. There, they are converted into solid material to produce, bit by bit, a supportive stem.
Likewise, the robot consists of a “growing point,” or gearbox, that pulls a loose chain of interlocking blocks into the box. Gears in the box then lock the chain units together and feed the chain out, unit by unit, as a rigid appendage.
The researchers presented the plant-inspired “growing robot” this week at the IEEE International Conference on Intelligent Robots and Systems (IROS) in Macau. They envision that grippers, cameras, and other sensors could be mounted onto the robot’s gearbox, enabling it to meander through an aircraft’s propulsion system and tighten a loose screw, or to reach into a shelf and grab a product without disturbing the organization of surrounding inventory, among other tasks.
“Think about changing the oil in your car,” says Harry Asada, professor of mechanical engineering at MIT. “After you open the engine roof, you have to be flexible enough to make sharp turns, left and right, to get to the oil filter, and then you have to be strong enough to twist the oil filter cap to remove it.”
“Now we have a robot that can potentially accomplish such tasks,” says Tongxi Yan, a former graduate student in Asada’s lab, who led the work. “It can grow, retract, and grow again to a different shape, to adapt to its environment.”
The team also includes MIT graduate student Emily Kamienski and visiting scholar Seiichi Teshigawara, who presented the results at the conference.
The last foot
The design of the new robot is an offshoot of Asada’s work in addressing the “last one-foot problem” — an engineering term referring to the last step, or foot, of a robot’s task or exploratory mission. While a robot may spend most of its time traversing open space, the last foot of its mission may involve more nimble navigation through tighter, more complex spaces to complete a task.
Engineers have devised various concepts and prototypes to address the last one-foot problem, including robots made from soft, balloon-like materials that grow like vines to squeeze through narrow crevices. But Asada says such soft extendable robots aren’t sturdy enough to support “end effectors,” or add-ons such as grippers, cameras, and other sensors that would be necessary in carrying out a task, once the robot has wormed its way to its destination.
“Our solution is not actually soft, but a clever use of rigid materials,” says Asada, who is the Ford Foundation Professor of Engineering.
Chain links
Once the team defined the general functional elements of plant growth, they looked to mimic this in a general sense, in an extendable robot.
“The realization of the robot is totally different from a real plant, but it exhibits the same kind of functionality, at a certain abstract level,” Asada says.
The researchers designed a gearbox to represent the robot’s “growing tip,” akin to the bud of a plant, where, as more nutrients flow up to the site, the tip feeds out more rigid stem. Within the box, they fit a system of gears and motors, which works to pull up a fluidized material — in this case, a bendy sequence of 3-D-printed plastic units interlocked with each other, similar to a bicycle chain.
As the chain is fed into the box, it turns around a winch, which feeds it through a second set of motors programmed to lock certain units in the chain to their neighboring units, creating a rigid appendage as it is fed out of the box.
The researchers can program the robot to lock certain units together while leaving others unlocked, to form specific shapes, or to “grow” in certain directions. In experiments, they were able to program the robot to turn around an obstacle as it extended or grew out from its base.
“It can be locked in different places to be curved in different ways, and have a wide range of motions,” Yan says.
When the chain is locked and rigid, it is strong enough to support a heavy, one-pound weight. If a gripper were attached to the robot’s growing tip, or gearbox, the researchers say the robot could potentially grow long enough to meander through a narrow space, then apply enough torque to loosen a bolt or unscrew a cap.
Auto maintenance is a good example of tasks the robot could assist with, according to Kamienski. “The space under the hood is relatively open, but it’s that last bit where you have to navigate around an engine block or something to get to the oil filter, that a fixed arm wouldn’t be able to navigate around. This robot could do something like that.”
This research was funded, in part, by NSK Ltd.