Artificial intelligence improves personalized cancer treatment
Inspired by water bugs, researchers create tiny swimming robots for medical, environmental uses
High-Throughput PIC Production Needs Precision Alignment Equipment for Photonics Arrays, Fibers, Lenses
New training approach could help AI agents perform better in uncertain conditions
Synthetic neurons that mimic human processes could lead to smarter robotics
Soft robots mimic mantis shrimp’s punch and flea’s leap for added power
Carving Out Your Competitive Advantage With AI
When I talk to corporate customers, there is often this idea that AI, while powerful, won’t give any company a lasting competitive edge. After all, over the past two years, large-scale LLMs have become a commodity for everyone. I’ve been thinking a lot about how companies can shape a competitive advantage using AI, and a recent article in the Harvard Business Review (AI Won’t Give You a New Sustainable Advantage) inspired me to organize my thoughts around the topic.
Indeed, maybe one day, when businesses and markets are driven by the invisible hand of AI, the equal-opportunity hypothesis might ring true. But until then, there are so many ways — big and small — for companies to differentiate themselves using AI. I like to think of it as a complex ingredient in your business recipe — the success of the final dish depends on the cook who is making it. The magic lies in how you combine AI craft with strategy, design, and execution.
In this article, I’ll focus on real-life business applications of AI and explore their key sources of competitive advantage. As we’ll see, successful AI integration goes far beyond technology, and certainly beyond having the trendiest LLM at work. It’s about finding AI’s unique sweet spot in your organization, making critical design decisions, and aligning a variety of stakeholders around the optimal design, deployment, and usage of your AI systems. In the following, I will illustrate this using the mental model we developed to structure our thinking about AI projects (cf. this article for an in-depth introduction).
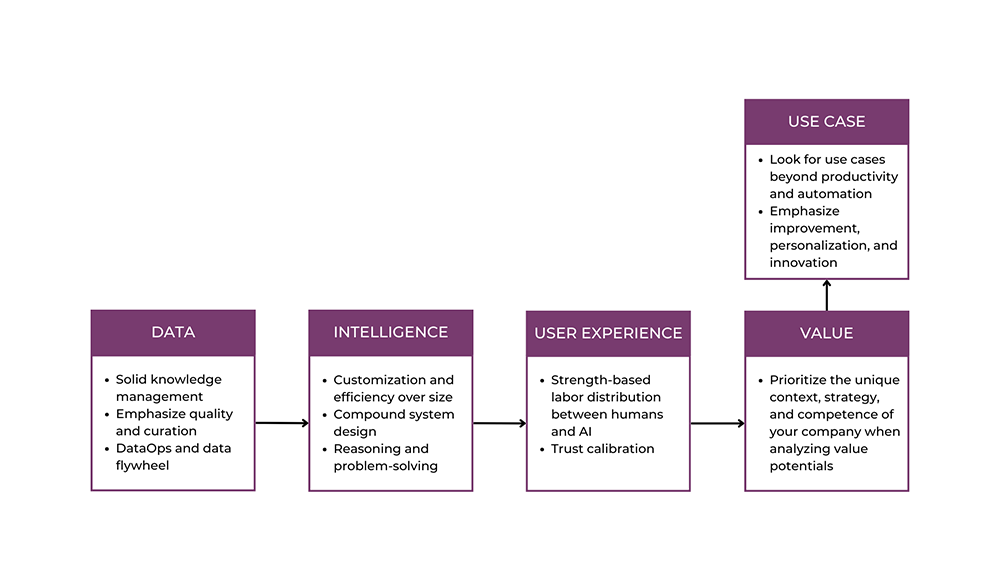
AI opportunities aren’t created equal
AI is often used to automate existing tasks, but the more space you allow for creativity and innovation when selecting your AI use cases, the more likely they will result in a competitive advantage. You should also prioritize the unique needs and strengths of your company when evaluating opportunities.
Identifying use cases with differentiation potential
When we brainstorm AI use cases with customers, 90% of them typically fall into one of four buckets — productivity, improvement, personalization, and innovation. Let’s take the example of an airline business to illustrate some opportunities across these categories:
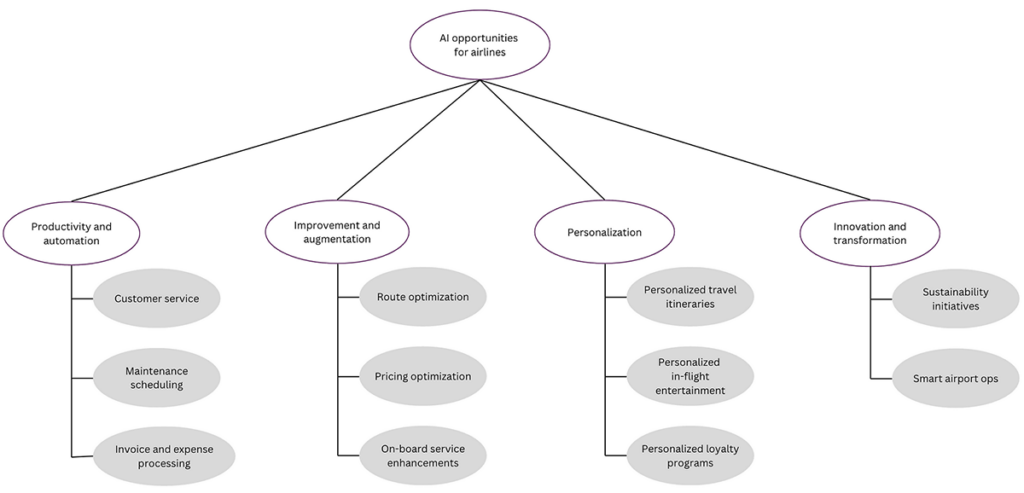
Of course, the first branch — productivity and automation — looks like the low-hanging fruit. It is the easiest one to implement, and automating boring routine tasks has an undeniable efficiency benefit. However, if you’re limiting your use of AI to basic automation, don’t be surprised when your competitors do the same. In our experience, strategic advantage is built up in the other branches. Companies that take the time to figure out how AI can help them offer something different, not just faster or cheaper, are the ones that see long-term results.
As an example, let’s look at a project we recently implemented with the Lufthansa Group. The company wanted to systematize and speed up its innovation processes. We developed an AI tool that acts as a giant sensor into the airline market, monitoring competitors, trends, and the overall market context. Based on this broad information, the tool now provides tailored innovation recommendations for Lufthansa. There are several aspects that cannot be easily imitated by potential competitors, and certainly not by just using a bigger AI model:
- Understanding which information exactly is needed to make decisions about new innovation initiatives
- Blending public data with unique company-specific knowledge
- Educating users at company scale on the right usage of the data in their assessment of new innovation initiatives
All of this is novel know-how that was developed in tight cooperation between industry experts, practitioners, and a specialized AI team, involving lots of discovery, design decisions, and stakeholder alignment. If you get all of these aspects right, I believe you are on a good path toward creating a sustainable and defensible advantage with AI.
Finding your unique sweet spot for value creation
Value creation with AI is a highly individual affair. I recently experienced this firsthand when I challenged myself to build and launch an end-to-end AI app on my own. I’m comfortable with Python and don’t massively benefit from AI help there, but other stuff like frontend? Not really my home turf. In this situation, AI-powered code generation worked like a charm. It felt like flowing through an effortless no-code tool, while having all the versatility of the underlying — and unfamiliar — programming languages under my fingertips. This was my very own, personal sweet spot — using AI where it unlocks value I wouldn’t otherwise tap into, and sparing a frontend developer on the way. Most other people would not get so much value out of this case:
- A professional front-end developer would not see such a drastic increase in speed.
- A person without programming experience would hardly ever get to the finish line. You must understand how programming works to correctly prompt an AI model and integrate its outputs.
While this is a personal example, the same principle applies at the corporate level. For good or for bad, most companies have some notion of strategy and core competence driving their business. The secret is about finding the right place for AI in that equation — a place where it will complement and amplify the existing skills.
Data — a game of effort
Data is the fuel for any AI system. Here, success comes from curating high-quality, focused datasets and continuously adapting them to evolving needs. By blending AI with your unique expertise and treating data as a dynamic resource, you can transform information into long-term strategic value.
Managing knowledge and domain expertise
To illustrate the importance of proper knowledge management, let’s do a thought experiment and travel to the 16th century. Antonio and Bartolomeo are the best shoemakers in Florence (which means they’re probably the best in the world). Antonio’s family has meticulously recorded their craft for generations, with shelves of notes on leather treatments, perfect fits, and small adjustments learned from years of experience. On the other hand, Bartolomeo’s family has kept their secrets more closely guarded. They don’t write anything down; their shoemaking expertise has been passed down verbally, from father to son.
Now, a visionary named Leonardo comes along, offering both families a groundbreaking technology that can automate their whole shoemaking business — if it can learn from their data. Antonio comes with his wagon of detailed documentation, and the technology can directly learn from those centuries of know-how. Bartolomeo is in trouble — without written records, there’s nothing explicit for the AI to chew on. His family’s expertise is trapped in oral tradition, intuition, and muscle memory. Should he try to write all of it down now — is it even possible, given that most of his work is governed intuitively? Or should he just let it be and go on with his manual business-as-usual? Succumbing to inertia and uncertainty, he goes for the latter option, while Antonio’s business strives and grows with the help of the new technology. Freed from daily routine tasks, he can get creative and invent new ways to make and improve shoes.
Beyond explicit documentation, valuable domain expertise is also hidden across other data assets such as transactional data, customer interactions, and market insights. AI thrives on this kind of information, extracting meaning and patterns that would otherwise go unnoticed by humans.
Quality over quantity
Data doesn’t need to be big — on the contrary, today, big often means noisy. What’s critical is the quality of the data you’re feeding into your AI system. As models become more sample-efficient — i.e., able to learn from smaller, more focused datasets — the kind of data you use is far more important than how much of it you have.
In my experience, the companies that succeed with AI treat their data — be it for training, fine-tuning, or evaluation — like a craft. They don’t just gather information passively; they curate and edit it, refining and selecting data that reflects a deep understanding of their specific industry. This careful approach gives their AI sharper insights and a more nuanced understanding than any competitor using a generic dataset. I’ve seen firsthand how even small improvements in data quality can lead to significant leaps in AI performance.
Capturing the dynamics with the data flywheel
Data needs to evolve along with the real world. That’s where DataOps comes in, ensuring data is continuously adapted and doesn’t drift apart from reality. The most successful companies understand this and regularly update their datasets to reflect changing environments and market dynamics. A power mechanism to achieve this is the data flywheel. The more your AI generates insights, the better your data becomes, creating a self-reinforcing feedback loop because users will come back to your system more often. With every cycle, your data sharpens and your AI improves, building an advantage that competitors will struggle to match. To kick off the data flywheel, your system needs to demonstrate some initial value to start with — and then, you can bake in some additional incentives to nudge your users into using your system on a regular basis.
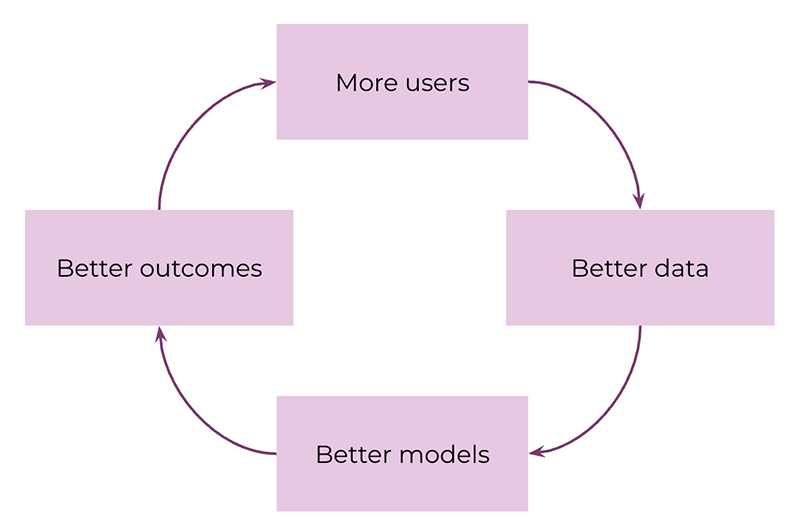
Intelligence: Sharpening your AI tools
Now, let’s dive into the “intelligence” component. This component isn’t just about AI models in isolation — it’s about how you integrate them into larger intelligent systems. Big Tech is working hard to make us believe that AI success hinges on the use of massive LLMs such as the GPT models. Good for them — bad for those of us who want to use AI in real-life applications. Overrelying on these heavyweights can bloat your system and quickly become a costly liability, while smart system design and tailored models are important sources for differentiation and competitive advantage.
Toward customization and efficiency
Mainstream LLMs are generalists. Like high-school graduates, they have a mediocre-to-decent performance across a wide range of tasks. However, in business, decent isn’t enough. You need to send your AI model to university so it can specialize, respond to your specific business needs, and excel in your domain. This is where fine-tuning comes into play. However, it’s important to recognize that mainstream LLMs, while powerful, can quickly become slow and expensive if not managed efficiently. As Big Tech boasts about larger model sizes and longer context windows — i.e., how much information you can feed into one prompt — smart tech is quietly moving towards efficiency. Techniques like prompt compression reduce prompt size, making interactions faster and more cost-effective. Small language models (SLMs) are another trend (Figure 4). With up to a couple of billions of parameters, they allow companies to safely deploy task- and domain-specific intelligence on their internal infrastructure (Anacode).
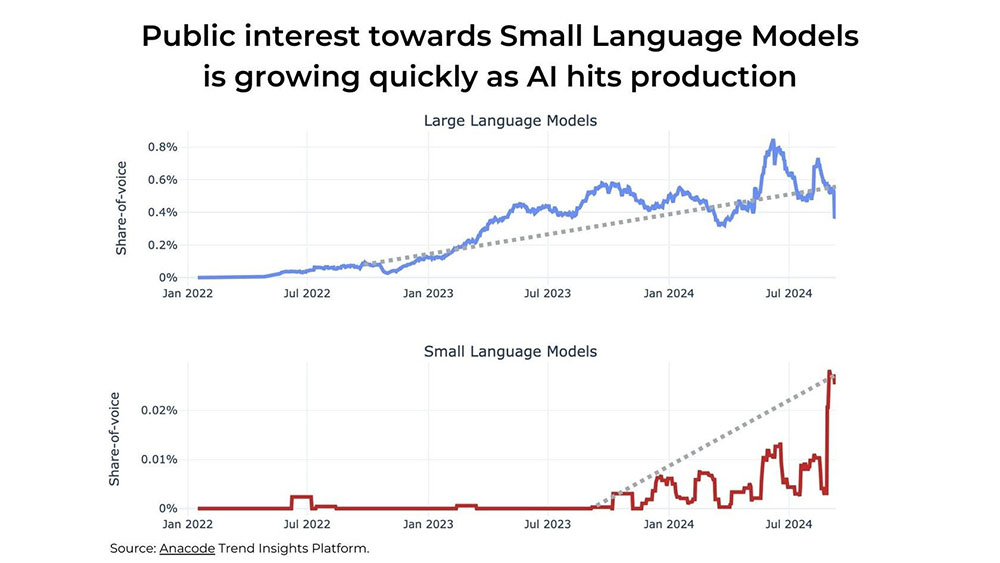
But before fine-tuning an LLM, ask yourself whether generative AI is even the right solution for your specific challenge. In many cases, predictive AI models — those that focus on forecasting outcomes rather than generating content — are more effective, cheaper, and easier to defend from a competitive standpoint. And while this might sound like old news, most of AI value creation in businesses actually happens with predictive AI.
Crafting compound AI systems
AI models don’t operate in isolation. Just as the human brain consists of multiple regions, each responsible for specific capabilities like reasoning, vision, and language, a truly intelligent AI system often involves multiple components. This is also called a “compound AI system” (BAIR). Compound systems can accommodate different models, databases, and software tools and allow you to optimize for cost and transparency. They also enable faster iteration and extension — modular components are easier to test and rearrange than a huge monolithic LLM.
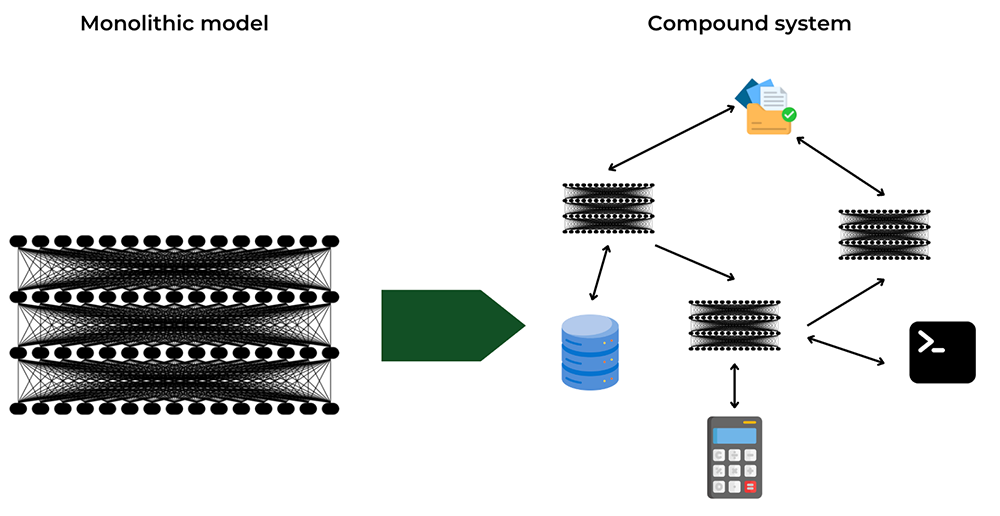
Take, for example, a customer service automation system for an SME. In its basic form — calling a commercial LLM — such a setup might cost you a significant amount — let’s say $21k/month for a “vanilla” system. This cost can easily scare away an SME, and they will not touch the opportunity at all. However, with careful engineering, optimization, and the integration of multiple models, the costs can be reduced by as much as 98% (FrugalGPT). Yes, you read it right, that’s 2% of the original cost — a staggering difference, putting a company with stronger AI and engineering skills at a clear advantage. At the moment, most businesses are not leveraging these advanced techniques, and we can only imagine how much there is yet to optimize in their AI usage.
Generative AI isn’t the finish line
While generative AI has captured everyone’s imagination with its ability to produce content, the real future of AI lies in reasoning and problem-solving. Unlike content generation, reasoning is nonlinear — it involves skills like abstraction and generalization which generative AI models aren’t trained for.
AI systems of the future will need to handle complex, multi-step activities that go far beyond what current generative models can do. We’re already seeing early demonstrations of AI’s reasoning capabilities, whether through language-based emulations or engineered add-ons. However, the limitations are apparent — past a certain threshold of complexity, these models start to hallucinate. Companies that invest in crafting AI systems designed to handle these complex, iterative processes will have a major head start. These companies will thrive as AI moves beyond its current generative phase and into a new era of smart, modular, and reasoning-driven systems.
User experience: Seamless integration into user workflows
User experience is the channel through which you can deliver the value of AI to users. It should smoothly transport the benefits users need to speed up and perfect their workflows, while inherent AI risks and issues such as erroneous outputs need to be filtered or mitigated.
Optimizing on the strengths of humans and AI
In most real-world scenarios, AI alone can’t achieve full automation. For example, at my company Equintel, we use AI to assist in the ESG reporting process, which involves multiple layers of analysis and decision-making. While AI excels at large-scale data processing, there are many subtasks that demand human judgment, creativity, and expertise. An ergonomic system design reflects this labor distribution, relieving humans from tedious data routines and giving them the space to focus on their strengths.
This strength-based approach also alleviates common fears of job replacement. When employees are empowered to focus on tasks where their skills shine, they’re more likely to view AI as a supporting tool, not a competitor. This fosters a win-win situation where both humans and AI thrive by working together.
Calibrating user trust
Every AI model has an inherent failure rate. Whether generative AI hallucinations or incorrect outputs from predictive models, mistakes happen and accumulate into the dreaded “last-mile problem.” Even if your AI system performs well 90% of the time, a small error rate can quickly become a showstopper if users overtrust the system and don’t address its errors.
Consider a bank using AI for fraud detection. If the AI fails to flag a fraudulent transaction and the user doesn’t catch it, the resulting loss could be significant — let’s say $500,000 siphoned from a compromised account. Without proper trust calibration, users might lack the tools or alerts to question the AI’s decision, allowing fraud to go unnoticed.
Now, imagine another bank using the same system but with proper trust calibration in place. When the AI is uncertain about a transaction, it flags it for review, even if it doesn’t outright classify it as fraud. This additional layer of trust calibration encourages the user to investigate further, potentially catching fraud that would have slipped through. In this scenario, the bank could avoid the $500,000 loss. Multiply that across multiple transactions, and the savings — along with improved security and customer trust — are substantial.
Combining AI efficiency and human ingenuity is the new competitive frontier
Success with AI requires more than just adopting the latest technologies — it’s about identifying and nurturing the individual sweet spots where AI can drive the most value for your business. This involves:
- Pinpointing the areas where AI can create a significant impact.
- Aligning a top-tier team of engineers, domain experts, and business stakeholders to design AI systems that meet these needs.
- Ensuring effective AI adoption by educating users on how to maximize its benefits.
Finally, I believe we are moving into a time when the notion of competitive advantage itself is shaken up. While in the past, competing was all about maximizing profitability, today, businesses are expected to balance financial gains with sustainability, which adds a new layer of complexity. AI has the potential to help companies not only optimize their operations but also move toward more sustainable practices. Imagine AI helping to reduce plastic waste, streamline shared economy models, or support other initiatives that make the world a better place. The real power of AI lies not just in efficiency but in the potential it offers us to reshape whole industries and drive both profit and positive social impact.
For deep-dives into many of the topics that were touched in this article, check out my upcoming book The Art of AI Product Development.
Note: Unless noted otherwise, all images are the author’s.
This article was originally published on Towards Data Science and re-published to TOPBOTS with permission from the author.
Enjoy this article? Sign up for more AI updates.
We’ll let you know when we release more articles like this one.
The post Carving Out Your Competitive Advantage With AI appeared first on TOPBOTS.
Using AI, researchers devise a fast and precise way to teach robots complicated skills
How to strengthen collaboration across AI teams
As AI evolves, effective collaboration across project lifecycles remains a pressing challenge for AI teams.
In fact, 20% of AI leaders cite collaboration as their biggest unmet need, underscoring that building cohesive AI teams is just as essential as building the AI itself.
With AI initiatives growing in complexity and scale, organizations that foster strong, cross-functional partnerships gain a critical edge in the race for innovation.
This quick guide equips AI leaders with practical strategies to strengthen collaboration across teams, ensuring smoother workflows, faster progress, and more successful AI outcomes.
Teamwork hurdles AI leaders are facing
AI collaboration is strained by team silos, shifting work environments, misaligned objectives, and increasing business demands.
For AI teams, these challenges manifest in four key areas:
- Fragmentation: Disjointed tools, workflows, and processes make it difficult for teams to operate as a cohesive unit.
- Coordination complexity: Aligning cross-functional teams on hand-off priorities, timelines, and dependencies becomes exponentially harder as projects scale.
- Inconsistent communication: Gaps in communication lead to missed opportunities, redundancies, rework, and confusion over project status and responsibilities.
- Model integrity: Ensuring model accuracy, fairness, and security requires seamless handoffs and constant oversight, but disconnected teams often lack the shared accountability or the observability tools needed to maintain it.
Addressing these hurdles is critical for AI leaders who want to streamline operations, minimize risks, and drive meaningful results faster.
Fragmentation workflows, tools, and languages
An AI project typically passes through five teams, seven tools, and 12 programming languages before reaching its business users — and that’s just the beginning.
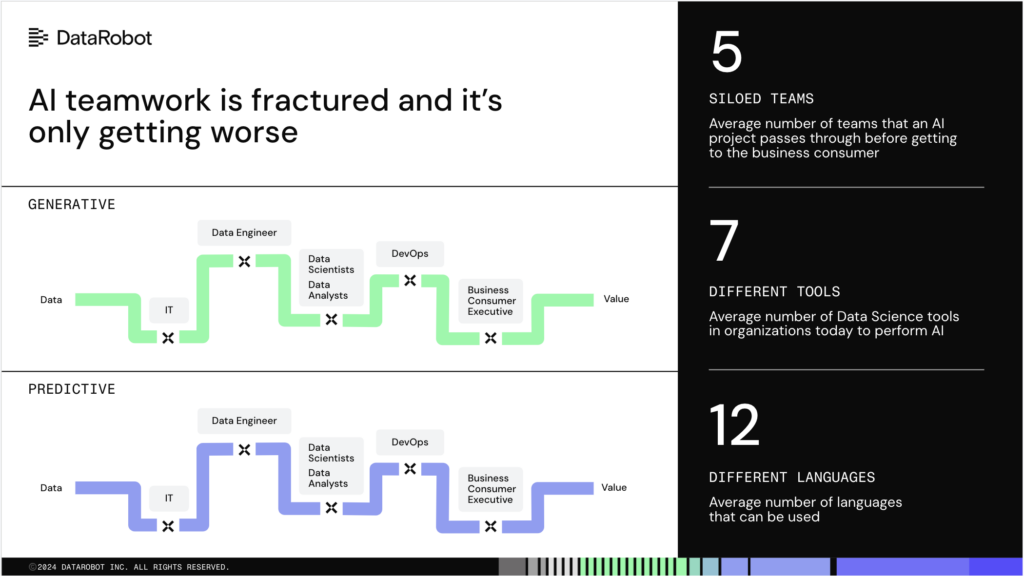
Here’s how fragmentation disrupts collaboration and what AI leaders can do to fix it:
- Disjointed projects: Silos between teams create misalignment. During the planning stage, design clear workflows and shared goals.
- Duplicated efforts: Redundant work slows progress and creates waste. Use shared documentation and centralized project tools to avoid overlap.
- Delays in completion: Poor handoffs create bottlenecks. Implement structured handoff processes and align timelines to keep projects moving.
- Tool and coding language incompatibility: Incompatible tools hinder interoperability. Standardize tools and programming languages where possible to enhance compatibility and streamline collaboration.
When the processes and teams are fragmented, it’s harder to maintain a united vision for the project. Over time, these misalignments can erode the business impact and user engagement of the final AI output.
The hidden cost of hand-offs
Each stage of an AI project presents a new hand-off – and with it, new risks to progress and performance. Here’s where things often go wrong:
- Data gaps from research to development: Incomplete or inconsistent data transfers and data duplication slow development and increases rework.
- Misaligned expectations: Unclear testing criteria lead to defects and delays during development-to-testing handoffs.
- Integration issues: Differences in technical environments can cause failures when models are moved from test to production.
- Weak monitoring: Limited oversight after deployment allows undetected issues to harm model performance and jeopardize business operations.
To mitigate these risks, AI leaders should offer solutions that synchronize cross-functional teams at each stage of development to preserve project momentum and ensure a more predictable, controlled path to deployment.
Strategic solutions
Breaking down barriers in team communications
AI leaders face a growing obstacle in uniting code-first and low-code teams while streamlining workflows to improve efficiency. This disconnect is significant, with 13% of AI leaders citing collaboration issues between teams as a major barrier when advancing AI use cases through various lifecycle stages.
To address these challenges, AI leaders can focus on two core strategies:
1. Provide context to align teams
AI leaders play a critical role in ensuring their teams understand the full project context, including the use case, business relevance, intended outcomes, and organizational policies.
Integrating these insights into approval workflows and automated guardrails maintains clarity on roles and responsibilities, protects sensitive data like personally identifiable information (PII), and ensures compliance with policies.
By prioritizing transparent communication and embedding context into workflows, leaders create an environment where teams can confidently innovate without risking sensitive information or operational integrity.
2. Use centralized platforms for collaboration
AI teams need a centralized communication platform to collaborate across model development, testing, and deployment stages.
An integrated AI suite can streamline workflows by allowing teams to tag assets, add comments, and share resources through central registries and use case hubs.
Key features like automated versioning and comprehensive documentation ensure work integrity while providing a clear historical record, simplify handoffs, and keep projects on track.
By combining clear context-setting with centralized tools, AI leaders can bridge team communication gaps, eliminate redundancies, and maintain efficiency across the entire AI lifecycle.
Protecting model integrity from development to deployment
For many organizations, models take more than seven months to reach production – regardless of AI maturity. This lengthy timeline introduces more opportunities for errors, inconsistencies, and misaligned goals.
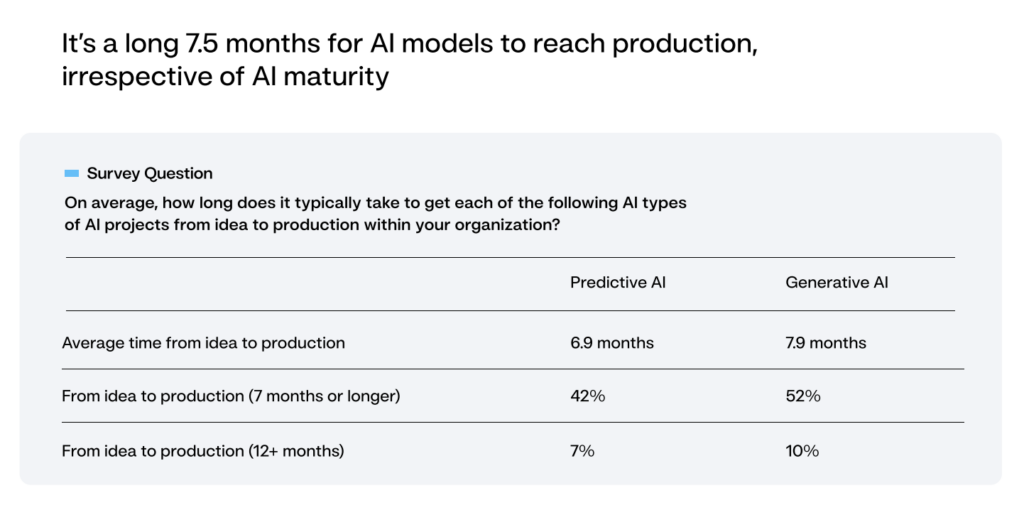
To safeguard model integrity, AI leaders should:
- Automate documentation, versioning, and history tracking.
- Invest in technologies with customizable guards and deep observability at every step.
- Empower AI teams to easily and consistently test, validate, and compare models.
- Provide collaborative workspaces and centralized hubs for seamless communication and handoffs.
- Establish well-monitored data pipelines to prevent drift, and maintain data quality and consistency.
- Emphasize the importance of model documentation and conduct regular audits to meet compliance standards.
- Establish clear criteria for when to update or maintain models, and develop a rollback strategy to quickly revert to previous versions if needed.
By adopting these practices, AI leaders can ensure high standards of model integrity, reduce risk, and deliver impactful results.
Lead the way in AI collaboration and innovation
As an AI leader, you have the power to create environments where collaboration and innovation thrive.
By promoting shared knowledge, clear communication, and collective problem-solving, you can keep your teams motivated and focused on high-impact outcomes.
For deeper insights and actionable guidance, explore our Unmet AI Needs report, and uncover how to strengthen your AI strategy and team performance.
The post How to strengthen collaboration across AI teams appeared first on DataRobot.
The most sensitive and durable robot hand yet created
Adapting to Industry 4.0: Key Tech Innovations and Data Security for Today’s Businesses
The rise of Industry 4.0 marks a turning point for businesses. It blends artificial intelligence (AI), the Internet of Things (IoT), and data science to transform operations and company growth. These technologies let businesses make smarter and faster decisions and […]
The post Adapting to Industry 4.0: Key Tech Innovations and Data Security for Today’s Businesses appeared first on TechSpective.