The Perfect Storm of Automation, Autonomous Machines and Artificial Intelligence
XPRIZE and California Governor Gavin Newsom Partner To Design an Incentive Prize For Innovation To Battle Wildfires
#286: Halodi Robotic’s EVEr3: A Full-size Humanoid Robot, with Bernt Børnich
In this episode, Audrow Nash interviews Bernt Børnich, CEO, CTO, and Co-founder of Halodi Robotics, about Eve (EVEr3), a general purpose full-size humanoid robot, capable of a wide variety of tasks. Børnich discusses how Eve can be used in research, how Eve’s motors have been designed to be safe around humans (including why they use a low gear ratio), how they do direct force control and the benefits of this approach, and how they use machine learning to reduce cogging in their motors. Børnich also discusses the longterm goal of Halodi Robotics and how they plan to support researchers using Eve.
Below are two videos of Eve. The first is a video of how Eve can be used as a platform to address several research questions. The second shows Eve moving a box and dancing.
Bernt Børnich
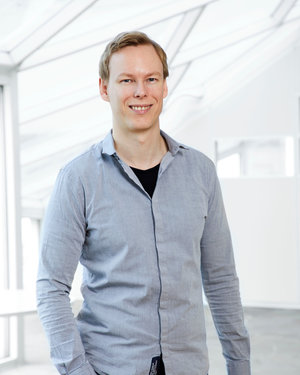
Bernt Børnich is the CEO and CTO of Halodi Robotics, and had the main responsibility for designing the motors, electronics and CAD-models for Eve. He holds a bachelor of robotics and nano-electronics from the University of Oslo.
Links
YOLO – You only look once (Single shot detectors)
The social animals that are inspiring new behaviours for robot swarms
By Edmund Hunt, University of Bristol
From flocks of birds to fish schools in the sea, or towering termite mounds, many social groups in nature exist together to survive and thrive. This cooperative behaviour can be used by engineers as “bio-inspiration” to solve practical human problems, and by computer scientists studying swarm intelligence.
“Swarm robotics” took off in the early 2000s, an early example being the “s-bot” (short for swarm-bot). This is a fully autonomous robot that can perform basic tasks including navigation and the grasping of objects, and which can self-assemble into chains to cross gaps or pull heavy loads. More recently, “TERMES” robots have been developed as a concept in construction, and the “CoCoRo” project has developed an underwater robot swarm that functions like a school of fish that exchanges information to monitor the environment. So far, we’ve only just begun to explore the vast possibilities that animal collectives and their behaviour can offer as inspiration to robot swarm design.
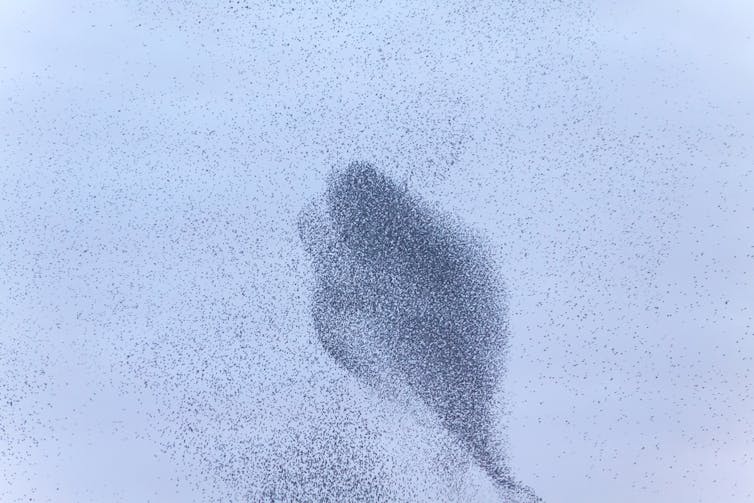
EyeSeeMicrostock/Shutterstock
Robots that can cooperate in large numbers could achieve things that would be difficult or even impossible for a single entity. Following an earthquake, for example, a swarm of search and rescue robots could quickly explore multiple collapsed buildings looking for signs of life. Threatened by a large wildfire, a swarm of drones could help emergency services track and predict the fire’s spread. Or a swarm of floating robots (“Row-bots”) could nibble away at oceanic garbage patches, powered by plastic-eating bacteria.
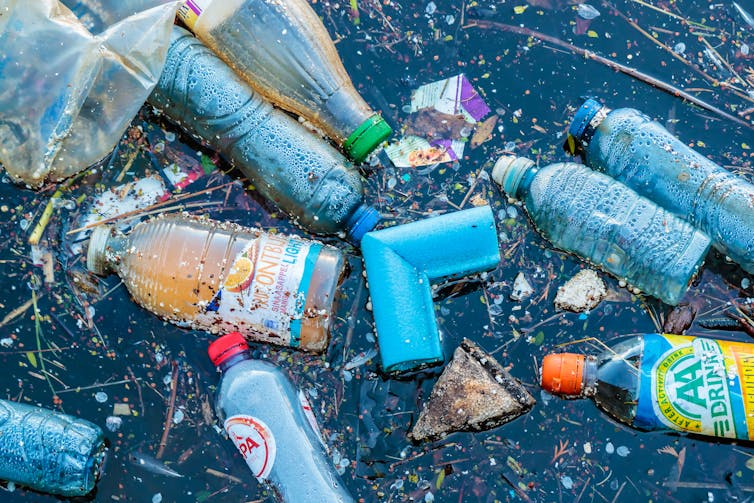
Shutterstock
Bio-inspiration in swarm robotics usually starts with social insects – ants, bees and termites – because colony members are highly related, which favours impressive cooperation. Three further characteristics appeal to researchers: robustness, because individuals can be lost without affecting performance; flexibility, because social insect workers are able to respond to changing work needs; and scalability, because a colony’s decentralised organisation is sustainable with 100 workers or 100,000. These characteristics could be especially useful for doing jobs such as environmental monitoring, which requires coverage of huge, varied and sometimes hazardous areas.
Social learning
Beyond social insects, other species and behavioural phenomena in the animal kingdom offer inspiration to engineers. A growing area of biological research is in animal cultures, where animals engage in social learning to pick up behaviours that they are unlikely to innovate alone. For example, whales and dolphins can have distinctive foraging methods that are passed down through the generations. This includes forms of tool use – dolphins have been observed breaking off marine sponges to protect their beaks as they go rooting around for fish, like a person might put a glove over a hand.
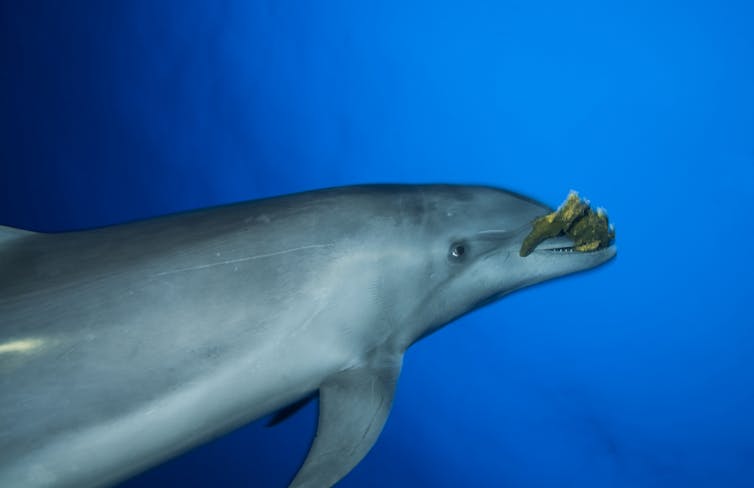
Yann Hubert/Shutterstock
Forms of social learning and artificial robotic cultures, perhaps using forms of artificial intelligence, could be very powerful in adapting robots to their environment over time. For example, assistive robots for home care could adapt to human behavioural differences in different communities and countries over time.
Robot (or animal) cultures, however, depend on learning abilities that are costly to develop, requiring a larger brain – or, in the case of robots, a more advanced computer. But the value of the “swarm” approach is to deploy robots that are simple, cheap and disposable. Swarm robotics exploits the reality of emergence (“more is different”) to create social complexity from individual simplicity. A more fundamental form of “learning” about the environment is seen in nature – in sensitive developmental processes – which do not require a big brain.
‘Phenotypic plasticity’
Some animals can change behavioural type, or even develop different forms, shapes or internal functions, within the same species, despite having the same initial “programming”. This is known as “phenotypic plasticity” – where the genes of an organism produce different observable results depending on environmental conditions. Such flexibility can be seen in the social insects, but sometimes even more dramatically in other animals.
Most spiders are decidedly solitary, but in about 20 of 45,000 spider species, individuals live in a shared nest and capture food on a shared web. These social spiders benefit from having a mixture of “personality” types in their group, for example bold and shy.
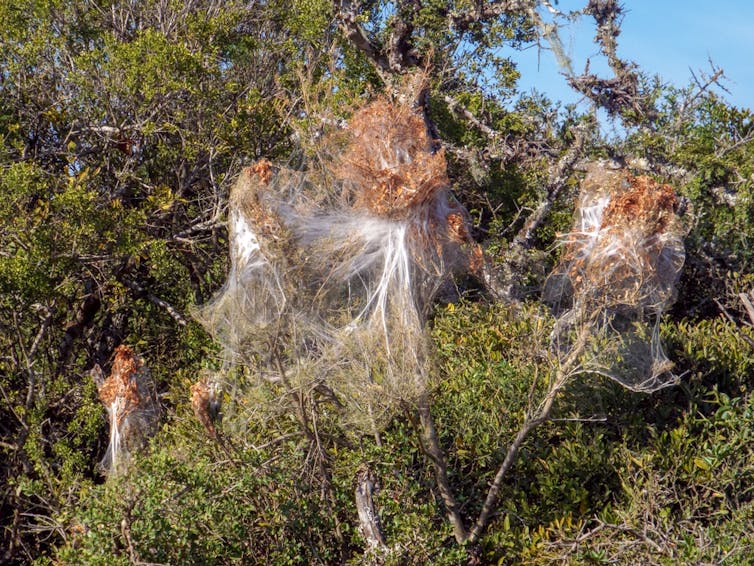
PicturesofThings/Shutterstock
My research identified a flexibility in behaviour where shy spiders would step into a role vacated by absent bold nestmates. This is necessary because the spider colony needs a balance of bold individuals to encourage collective predation, and shyer ones to focus on nest maintenance and parental care. Robots could be programmed with adjustable risk-taking behaviour, sensitive to group composition, with bolder robots entering into hazardous environments while shyer ones know to hold back. This could be very helpful in mapping a disaster area such as Fukushima, including its most dangerous parts, while avoiding too many robots in the swarm being damaged at once.
The ability to adapt
Cane toads were introduced in Australia in the 1930s as a pest control, and have since become an invasive species themselves. In new areas cane toads are seen to be somewhat social. One reason for their growth in numbers is that they are able to adapt to a wide temperature range, a form of physiological plasticity. Swarms of robots with the capability to switch power consumption mode, depending on environmental conditions such as ambient temperature, could be considerably more durable if we want them to function autonomously for the long term. For example, if we want to send robots off to map Mars then they will need to cope with temperatures that can swing from -150°C at the poles to 20°C at the equator.
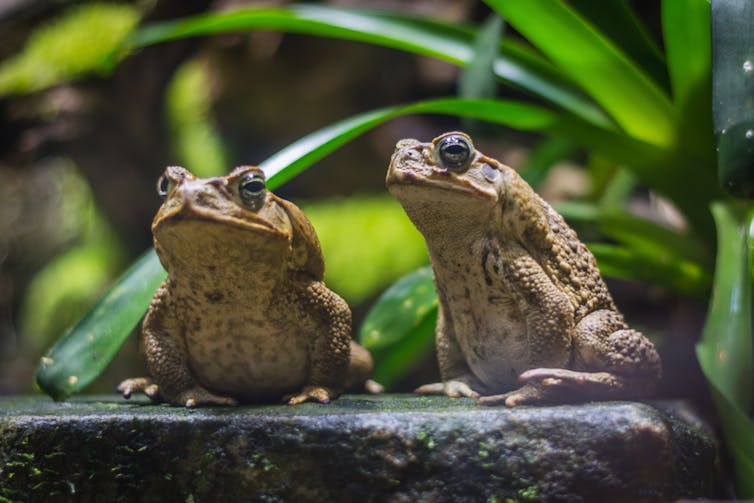
Radek Ziemniewicz/Shutterstock
In addition to behavioural and physiological plasticity, some organisms show morphological (shape) plasticity. For example, some bacteria change their shape in response to stress, becoming elongated and so more resilient to being “eaten” by other organisms. If swarms of robots can combine together in a modular fashion and (re)assemble into more suitable structures this could be very helpful in unpredictable environments. For example, groups of robots could aggregate together for safety when the weather takes a challenging turn.
Whether it’s the “cultures” developed by animal groups that are reliant on learning abilities, or the more fundamental ability to change “personality”, internal function or shape, swarm robotics still has plenty of mileage left when it comes to drawing inspiration from nature. We might even wish to mix and match behaviours from different species, to create robot “hybrids” of our own. Humanity faces challenges ranging from climate change affecting ocean currents, to a growing need for food production, to space exploration – and swarm robotics can play a decisive part given the right bio-inspiration.
Edmund Hunt, EPSRC Doctoral Prize Fellow, University of Bristol
This article is republished from The Conversation under a Creative Commons license. Read the original article.
An updated round up of ethical principles of robotics and AI
This blogpost is an updated round up of the various sets of ethical principles of robotics and AI that have been proposed to date, ordered by date of first publication.
I previously listed principles published before December 2017 here; this blogpost appends those principles drafted since January 2018 (plus one in October 2017 I had missed). The principles are listed here (in full or abridged) with links, notes and references but without critique.
Scroll down to the next horizontal line for the updates.
If there any (prominent) ones I’ve missed please let me know.
Asimov’s three laws of Robotics (1950)
- A robot may not injure a human being or, through inaction, allow a human being to come to harm.
- A robot must obey the orders given it by human beings except where such orders would conflict with the First Law.
- A robot must protect its own existence as long as such protection does not conflict with the First or Second Laws.
I have included these to explicitly acknowledge, firstly, that Asimov undoubtedly established the principle that robots (and by extension AIs) should be governed by principles, and secondly that many subsequent principles have been drafted as a direct response. The three laws first appeared in Asimov’s short story Runaround [1]. This wikipedia article provides a very good account of the three laws and their many (fictional) extensions.
Murphy and Wood’s three laws of Responsible Robotics (2009)
- A human may not deploy a robot without the human-robot work system meeting the highest legal and professional standards of safety and ethics.
- A robot must respond to humans as appropriate for their roles.
- A robot must be endowed with sufficient situated autonomy to protect its own existence as long as such protection provides smooth transfer of control which does not conflict with the First and Second Laws.
These were proposed in Robin Murphy and David Wood’s paper Beyond Asimov: The Three Laws of Responsible Robotics [2].
EPSRC Principles of Robotics (2010)
- Robots are multi-use tools. Robots should not be designed solely or primarily to kill or harm humans, except in the interests of national security.
- Humans, not Robots, are responsible agents. Robots should be designed and operated as far as practicable to comply with existing laws, fundamental rights and freedoms, including privacy.
- Robots are products. They should be designed using processes which assure their safety and security.
- Robots are manufactured artefacts. They should not be designed in a deceptive way to exploit vulnerable users; instead their machine nature should be transparent.
- The person with legal responsibility for a robot should be attributed.
These principles were drafted in 2010 and published online in 2011, but not formally published until 2017 [3] as part of a two-part special issue of Connection Science on the principles, edited by Tony Prescott & Michael Szollosy [4]. An accessible introduction to the EPSRC principles was published in New Scientist in 2011.
Future of Life Institute Asilomar principles for beneficial AI (Jan 2017)
I will not list all 23 principles but extract just a few to compare and contrast with the others listed here:
6. Safety: AI systems should be safe and secure throughout their operational lifetime, and verifiably so where applicable and feasible.
7. Failure Transparency: If an AI system causes harm, it should be possible to ascertain why.
8. Judicial Transparency: Any involvement by an autonomous system in judicial decision-making should provide a satisfactory explanation auditable by a competent human authority.
9. Responsibility: Designers and builders of advanced AI systems are stakeholders in the moral implications of their use, misuse, and actions, with a responsibility and opportunity to shape those implications.
10. Value Alignment: Highly autonomous AI systems should be designed so that their goals and behaviors can be assured to align with human values throughout their operation.
11. Human Values: AI systems should be designed and operated so as to be compatible with ideals of human dignity, rights, freedoms, and cultural diversity.
12. Personal Privacy: People should have the right to access, manage and control the data they generate, given AI systems’ power to analyze and utilize that data.
13. Liberty and Privacy: The application of AI to personal data must not unreasonably curtail people’s real or perceived liberty.
14. Shared Benefit: AI technologies should benefit and empower as many people as possible.
15. Shared Prosperity: The economic prosperity created by AI should be shared broadly, to benefit all of humanity.
An account of the development of the Asilomar principles can be found here.
The ACM US Public Policy Council Principles for Algorithmic Transparency and Accountability (Jan 2017)
- Awareness: Owners, designers, builders, users, and other stakeholders of analytic systems should be aware of the possible biases involved in their design, implementation, and use and the potential harm that biases can cause to individuals and society.
- Access and redress: Regulators should encourage the adoption of mechanisms that enable questioning and redress for individuals and groups that are adversely affected by algorithmically informed decisions.
- Accountability: Institutions should be held responsible for decisions made by the algorithms that they use, even if it is not feasible to explain in detail how the algorithms produce their results.
- Explanation: Systems and institutions that use algorithmic decision-making are encouraged to produce explanations regarding both the procedures followed by the algorithm and the specific decisions that are made. This is particularly important in public policy contexts.
- Data Provenance: A description of the way in which the training data was collected should be maintained by the builders of the algorithms, accompanied by an exploration of the potential biases induced by the human or algorithmic data-gathering process.
- Auditability: Models, algorithms, data, and decisions should be recorded so that they can be audited in cases where harm is suspected.
- Validation and Testing: Institutions should use rigorous methods to validate their models and document those methods and results.
See the ACM announcement of these principles here. The principles form part of the ACM’s updated code of ethics.
Japanese Society for Artificial Intelligence (JSAI) Ethical Guidelines (Feb 2017)
- Contribution to humanity Members of the JSAI will contribute to the peace, safety, welfare, and public interest of humanity.
- Abidance of laws and regulations Members of the JSAI must respect laws and regulations relating to research and development, intellectual property, as well as any other relevant contractual agreements. Members of the JSAI must not use AI with the intention of harming others, be it directly or indirectly.
- Respect for the privacy of others Members of the JSAI will respect the privacy of others with regards to their research and development of AI. Members of the JSAI have the duty to treat personal information appropriately and in accordance with relevant laws and regulations.
- Fairness Members of the JSAI will always be fair. Members of the JSAI will acknowledge that the use of AI may bring about additional inequality and discrimination in society which did not exist before, and will not be biased when developing AI.
- Security As specialists, members of the JSAI shall recognize the need for AI to be safe and acknowledge their responsibility in keeping AI under control.
- Act with integrity Members of the JSAI are to acknowledge the significant impact which AI can have on society.
- Accountability and Social Responsibility Members of the JSAI must verify the performance and resulting impact of AI technologies they have researched and developed.
- Communication with society and self-development Members of the JSAI must aim to improve and enhance society’s understanding of AI.
- Abidance of ethics guidelines by AI AI must abide by the policies described above in the same manner as the members of the JSAI in order to become a member or a quasi-member of society.
An explanation of the background and aims of these ethical guidelines can be found here, together with a link to the full principles (which are shown abridged above).
Draft principles of The Future Society’s Science, Law and Society Initiative (Oct 2017)
- AI should advance the well-being of humanity, its societies, and its natural environment.
- AI should be transparent.
- Manufacturers and operators of AI should be accountable.
- AI’s effectiveness should be measurable in the real-world applications for which it is intended.
- Operators of AI systems should have appropriate competencies.
- The norms of delegation of decisions to AI systems should be codified through thoughtful, inclusive dialogue with civil society.
This article by Nicolas Economou explains the 6 principles with a full commentary on each one.
Montréal Declaration for Responsible AI draft principles (Nov 2017)
- Well-being The development of AI should ultimately promote the well-being of all sentient creatures.
- Autonomy The development of AI should promote the autonomy of all human beings and control, in a responsible way, the autonomy of computer systems.
- Justice The development of AI should promote justice and seek to eliminate all types of discrimination, notably those linked to gender, age, mental / physical abilities, sexual orientation, ethnic/social origins and religious beliefs.
- Privacy The development of AI should offer guarantees respecting personal privacy and allowing people who use it to access their personal data as well as the kinds of information that any algorithm might use.
- Knowledge The development of AI should promote critical thinking and protect us from propaganda and manipulation.
- Democracy The development of AI should promote informed participation in public life, cooperation and democratic debate.
- Responsibility The various players in the development of AI should assume their responsibility by working against the risks arising from their technological innovations.
The Montréal Declaration for Responsible AI proposes the 7 values and draft principles above (here in full with preamble, questions and definitions).
IEEE General Principles of Ethical Autonomous and Intelligent Systems (Dec 2017)
- How can we ensure that A/IS do not infringe human rights?
- Traditional metrics of prosperity do not take into account the full effect of A/IS technologies on human well-being.
- How can we assure that designers, manufacturers, owners and operators of A/IS are responsible and accountable?
- How can we ensure that A/IS are transparent?
- How can we extend the benefits and minimize the risks of AI/AS technology being misused?
These 5 general principles appear in Ethically Aligned Design v2, a discussion document drafted and published by the IEEE Standards Association Global Initiative on Ethics of Autonomous and Intelligent Systems. The principles are expressed not as rules but instead as questions, or concerns, together with background and candidate recommendations.
A short article co-authored with IEEE general principles co-chair Mark Halverson Why Principles Matter explains the link between principles and standards, together with further commentary and references.
Note that these principles have been revised and extended, in March 2019 (see below).
UNI Global Union Top 10 Principles for Ethical AI (Dec 2017)
- Demand That AI Systems Are Transparent
- Equip AI Systems With an Ethical Black Box
- Make AI Serve People and Planet
- Adopt a Human-In-Command Approach
- Ensure a Genderless, Unbiased AI
- Share the Benefits of AI Systems
- Secure a Just Transition and Ensuring Support for Fundamental Freedoms and Rights
- Establish Global Governance Mechanisms
- Ban the Attribution of Responsibility to Robots
- Ban AI Arms Race
Drafted by UNI Global Union‘s Future World of Work these 10 principles for Ethical AI (set out here with full commentary) “provide unions, shop stewards and workers with a set of concrete demands to the transparency, and application of AI”.
Updated principles
Intel’s recommendation for Public Policy Principles on AI (October 2017)
- Foster Innovation and Open Development – To better understand the impact of AI and explore the broad diversity of AI implementations, public policy should encourage investment in AI R&D. Governments should support the controlled testing of AI systems to help industry, academia, and other stakeholders improve the technology.
- Create New Human Employment Opportunities and Protect People’s Welfare – AI will change the way people work. Public policy in support of adding skills to the workforce and promoting employment across different sectors should enhance employment opportunities while also protecting people’s welfare.
- Liberate Data Responsibly – AI is powered by access to data. Machine learning algorithms improve by analyzing more data over time; data access is imperative to achieve more enhanced AI model development and training. Removing barriers to the access of data will help machine learning and deep learning reach their full potential.
- Rethink Privacy – Privacy approaches like The Fair Information Practice Principles and Privacy by Design have withstood the test of time and the evolution of new technology. But with innovation, we have had to “rethink” how we apply these models to new technology.
- Require Accountability for Ethical Design and Implementation – The social implications of computing have grown and will continue to expand as more people have access to implementations of AI. Public policy should work to identify and mitigate discrimination caused by the use of AI and encourage designing in protections against these harms.
These principles were announced in a blog post by Naveen Rao (Intel VP AI) here.
Lords Select Committee 5 core principles to keep AI ethical (April 2018)
- Artificial intelligence should be developed for the common good and
benefit of humanity. - Artificial intelligence should operate on principles of intelligibility and
fairness. - Artificial intelligence should not be used to diminish the data rights or
privacy of individuals, families or communities. - All citizens have the right to be educated to enable them to flourish
mentally, emotionally and economically alongside artificial intelligence. - The autonomous power to hurt, destroy or deceive human beings
should never be vested in artificial intelligence.
These principles appear in the UK House of Lords Select Committee on Artificial Intelligence report AI in the UK: ready, willing and able? published in April 2019. The WEF published a summary and commentary here.
AI UX: 7 Principles of Designing Good AI Products (April 2018)
- Differentiate AI content visually – let people know if an algorithm has generated a piece of content so they can decide for themselves whether to trust it or not.
- Explain how machines think – helping people understand how machines work so they can use them better
- Set the right expectations – especially in a world full of sensational, superficial news about new AI technologies.
- Find and handle weird edge cases – spend more time testing and finding weird, funny, or even disturbing or unpleasant edge cases.
- User testing for AI products (default methods won’t work here).
- Provide an opportunity to give feedback.
These principles, focussed on the design of the User Interface (UI) and User Experience (UX), are from Budapest based company UX Studio.
The Toronto Declaration on equality and non-discrimination in machine learning systems (May 2018)
The Toronto Declaration: Protecting the right to equality and non-discrimination in machine learning systems does not succinctly articulate ethical principles but instead presents arguments under the following headings to address concerns “about the capability of [machine learning] systems to facilitate intentional or inadvertent discrimination against certain individuals or groups of people”.
- Using the framework of international human rights law The right to equality and non-discrimination; Preventing discrimination, and Protecting the rights of all individuals and groups: promoting diversity and inclusion
- Duties of states: human rights obligations State use of machine learning systems; Promoting equality, and Holding private sector actors to account
- Responsibilities of private sector actors human rights due diligence
- The right to an effective remedy
Google AI Principles (June 2018)
- Be socially beneficial.
- Avoid creating or reinforcing unfair bias.
- Be built and tested for safety.
- Be accountable to people.
- Incorporate privacy design principles.
- Uphold high standards of scientific excellence.
- Be made available for uses that accord with these principles.
These principles were launched with a blog post and commentary by Google CEO Sundar Pichai here.
IBM’s 5 ethical AI principles (September 2018)
- Accountability: AI designers and developers are responsible for considering AI design, development, decision processes, and outcomes.
- Value alignment: AI should be designed to align with the norms and values of your user group in mind.
- Explainability: AI should be designed for humans to easily perceive, detect, and understand its decision process, and the predictions/recommendations. This is also, at times, referred to as interpretability of AI. Simply speaking, users have all rights to ask the details on the predictions made by AI models such as which features contributed to the predictions by what extent. Each of the predictions made by AI models should be able to be reviewed.
- Fairness: AI must be designed to minimize bias and promote inclusive representation.
- User data rights: AI must be designed to protect user data and preserve the user’s power over access and uses
For a full account read IBM’s Everyday Ethics for Artificial Intelligence here.
Microsoft Responsible bots: 10 guidelines for developers of
conversational AI (November 2018)
- Articulate the purpose of your bot and take special care if your bot will support consequential use cases.
- Be transparent about the fact that you use bots as part of your product or service.
- Ensure a seamless hand-off to a human where the human-bot exchange leads to interactions that exceed the bot’s competence.
- Design your bot so that it respects relevant cultural norms and guards against misuse.
- Ensure your bot is reliable.
- Ensure your bot treats people fairly.
- Ensure your bot respects user privacy.
- Ensure your bot handles data securely.
- Ensure your bot is accessible.
- Accept responsibility.
Microsoft’s guidelines for the ethical design of ‘bots’ (chatbots or conversational AIs) are fully described here.
CEPEJ European Ethical Charter on the use of artificial
intelligence (AI) in judicial systems and their environment, 5 principles (February 2019)
- Principle of respect of fundamental rights: ensuring that the design and implementation of artificial intelligence tools and services are compatible with fundamental rights.
- Principle of non-discrimination: specifically preventing the development or intensification of any discrimination between individuals or groups of individuals.
- Principle of quality and security: with regard to the processing of judicial decisions and data, using certified sources and intangible data with models conceived in a multi-disciplinary manner, in a secure technological environment.
- Principle of transparency, impartiality and fairness: making data processing methods accessible and understandable, authorising external audits.
- Principle “under user control”: precluding a prescriptive approach and ensuring that users are informed actors and in control of their choices.
The Council of Europe ethical charter principles are outlined here, with a link to the ethical charter istelf.
Women Leading in AI (WLinAI) 10 recommendations (February 2019)
- Introduce a regulatory approach governing the deployment of AI which mirrors that used for the pharmaceutical sector.
- Establish an AI regulatory function working alongside the Information Commissioner’s Office and Centre for Data Ethics – to audit algorithms, investigate complaints by individuals,issue notices and fines for breaches of GDPR and equality and human rights law, give wider guidance, spread best practice and ensure algorithms must be fully explained to users and open to public scrutiny.
- Introduce a new Certificate of Fairness for AI systems alongside a ‘kite mark’ type scheme to display it. Criteria to be defined at industry level, similarly to food labelling regulations.
- Introduce mandatory AIAs (Algorithm Impact Assessments) for organisations employing AI systems that have a significant effect on individuals.
- Introduce a mandatory requirement for public sector organisations using AI for particular purposes to inform citizens that decisions are made by machines, explain how the decision is reached and what would need to change for individuals to get a different outcome.
- Introduce a ‘reduced liability’ incentive for companies that have obtained a Certificate of Fairness to foster innovation and competitiveness.
- To compel companies and other organisations to bring their workforce with them – by publishing the impact of AI on their workforce and offering retraining programmes for employees whose jobs are being automated.
- Where no redeployment is possible, to compel companies to make a contribution towards a digital skills fund for those employees
- To carry out a skills audit to identify the wide range of skills required to embrace the AI revolution.
- To establish an education and training programme to meet the needs identified by the skills audit, including content on data ethics and social responsibility. As part of that, we recommend the set up of a solid, courageous and rigorous programme to encourage young women and other underrepresented groups into technology.
Presented by the Women Leading in AI group at a meeting in parliament in February 2019, this report in Forbes by Noel Sharkey outlines both the group, their recommendations, and the meeting.
The NHS’s 10 Principles for AI + Data (February 2019)
- Understand users, their needs and the context
- Define the outcome and how the technology will contribute to it
- Use data that is in line with appropriate guidelines for the purpose for which it is being used
- Be fair, transparent and accountable about what data is being used
- Make use of open standards
- Be transparent about the limitations of the data used and algorithms deployed
- Show what type of algorithm is being developed or deployed, the ethical examination of how the data is used, how its performance will be validated and how it will be integrated into health and care provision
- Generate evidence of effectiveness for the intended use and value for money
- Make security integral to the design
- Define the commercial strategy
These principles are set out with full commentary and elaboration on Artificial Lawyer here.
IEEE General Principles of Ethical Autonomous and Intelligent Systems (A/IS) (March 2019)
- Human Rights: A/IS shall be created and operated to respect, promote, and protect internationally recognized human rights.
- Well-being: A/IS creators shall adopt increased human well-being as a primary success criterion for development.
- Data Agency: A/IS creators shall empower individuals with the ability to access and securely share their data to maintain people’s capacity to have control over their identity.
- Effectiveness: A/IS creators and operators shall provide evidence of the effectiveness and fitness for purpose of A/IS.
- Transparency: the basis of a particular A/IS decision should always be discoverable.
- Accountability: A/IS shall be created and operated to provide an unambiguous rationale for all decisions made.
- Awareness of Misuse: A/IS creators shall guard against all potential misuses and risks of A/IS in operation.
- Competence: A/IS creators shall specify and operators shall adhere to the knowledge and skill required for safe and effective operation.
Ethical issues arising from the police use of live facial
recognition technology (March 2019)
9 ethical principles relate to: public interest, effectiveness, the avoidance of bias and algorithmic justice, impartiality and deployment, necessity, proportionality, impartiality, accountability, oversight, and the construction of watchlists, public trust, and cost effectiveness.
Reported here the UK government’s independent Biometrics and Forensics Ethics Group (BFEG) published an interim report outlining nine ethical principles forming a framework to guide policy on police facial recognition systems.
Floridi and Clement Jones’ five principles key to any
ethical framework for AI (March 2019)
- AI must be beneficial to humanity.
- AI must also not infringe on privacy or undermine security.
- AI must protect and enhance our autonomy and ability to take decisions and choose between alternatives.
- AI must promote prosperity and solidarity, in a fight against inequality, discrimination, and unfairness
- We cannot achieve all this unless we have AI systems that are understandable in terms of how they work (transparency) and explainable in terms of how and why they reach the conclusions they do (accountability).
Luciano Floridi and Lord Tim Clement Jones set out, here in the New Statesman, these 5 general ethical principles for AI, with additional commentary.
The European Commission’s High Level Expert Group on AI Ethics Guidelines for Trustworthy AI (April 2019)
- Human agency and oversight AI systems should support human autonomy and decision-making, as prescribed by the principle of respect for human autonomy.
- Technical robustness and safety A crucial component of achieving Trustworthy AI is technical robustness, which is closely linked to the principle of prevention of harm.
- Privacy and Data governance Closely linked to the principle of prevention of harm is privacy, a fundamental right particularly affected by AI systems.
- Transparency This requirement is closely linked with the principle of explicability and encompasses transparency of elements relevant to an AI system: the data, the system and the business models.
- Diversity, non-discrimination and fairness In order to achieve Trustworthy AI, we must enable inclusion and diversity throughout the entire AI system’s life cycle.
- Societal and environmental well-being In line with the principles of fairness and prevention of harm, the broader society, other sentient beings and the environment should be also considered as stakeholders throughout the AI system’s life cycle.
- Accountability The requirement of accountability complements the above requirements, and is closely linked to the principle of fairness
For more detail on each of these principles follow the links above.
Published on 8 April 2019, the EU HLEG AI ethics guidelines for trustworthy AI are detailed in full here.
Draft core principles of Australia’s Ethics Framework for AI (April 2019)
- Generates net-benefits. The AI system must generate benefits for people that are greater than the costs.
- Do no harm. Civilian AI systems must not be designed to harm or deceive people and should be implemented in ways that minimise any negative outcomes.
- Regulatory and legal compliance. The AI system must comply with all relevant international, Australian Local, State/Territory and Federal government obligations, regulations and laws.
- Privacy protection. Any system, including AI systems, must ensure people’s private data is protected and kept confidential plus prevent data breaches which could cause reputational, psychological, financial, professional or other types of harm.
- Fairness. The development or use of the AI system must not result in unfair discrimination against individuals, communities or groups. This requires particular attention to ensure the “training data” is free from bias or characteristics which may cause the algorithm to behave unfairly.
- Transparency & Explainability. People must be informed when an algorithm is being used that impacts them and they should be provided with information about what information the algorithm uses to make decisions.
- Contestability. When an algorithm impacts a person there must be an efficient process to allow that person to challenge the use or output of the algorithm.
- Accountability. People and organisations responsible for the creation and implementation of AI algorithms should be identifiable and accountable for the impacts of that algorithm, even if the impacts are unintended.
These draft principles are detailed in Artificial Intelligence Australia’s Ethics Framework A Discussion Paper. This comprehensive paper includes detailed summaries of many of the frameworks and initiatives listed above, together with some very useful case studies.
References
[1] Asimov, Isaac (1950): Runaround, in I, Robot, (The Isaac Asimov Collection ed.) Doubleday. ISBN 0-385-42304-7.
[2] Murphy, Robin; Woods, David D. (2009): Beyond Asimov: The Three Laws of Responsible Robotics. IEEE Intelligent systems. 24 (4): 14–20.
[3] Margaret Boden et al (2017): Principles of robotics: regulating robots in the real world
Connection Science. 29 (2): 124:129.
[4] Tony Prescott and Michael Szollosy (eds.) (2017): Ethical Principles of Robotics, Connection Science. 29 (2) and 29 (3).
Robots that learn to adapt
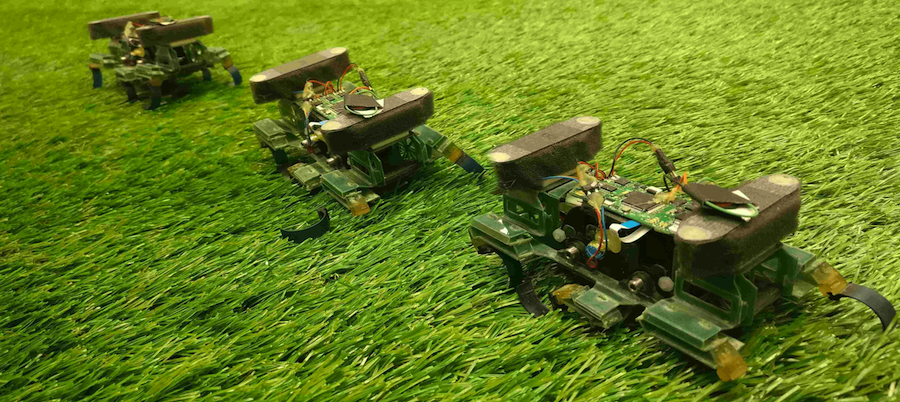
By Anusha Nagabandi and Ignasi Clavera
Humans have the ability to seamlessly adapt to changes in their environments: adults can learn to walk on crutches in just a few seconds, people can adapt almost instantaneously to picking up an object that is unexpectedly heavy, and children who can walk on flat ground can quickly adapt their gait to walk uphill without having to relearn how to walk. This adaptation is critical for functioning in the real world.
Robots, on the other hand, are typically deployed with a fixed behavior (be it hard-coded or learned), allowing them succeed in specific settings, but leading to failure in others: experiencing a system malfunction, encountering a new terrain or environment changes such as wind, or needing to cope with a payload or other unexpected perturbations. The idea behind our latest research is that the mismatch between predicted and observed recent states should inform the robot to update its model into one that more accurately describes the current situation. Noticing our car skidding on the road, for example, informs us that our actions are having a different effect than expected, and thus allows us to plan our consequent actions accordingly (Fig. 2). In order for our robots to be successful in the real world, it is critical that they have this ability to use their past experience to quickly and flexibly adapt. To this effect, we developed a model-based meta-reinforcement learning algorithm capable of fast adaptation.
Figure 2: The driver normally makes decisions based on his/her model of the world. Suddenly encountering a slippery road, however, leads to unexpected skidding. Online adaptation of the driver’s world model based on just a few of these observations of model mismatch allows for fast recovery.
Fast Adaptation
Prior work has used (a) trial-and-error adaptation approaches (Cully et al., 2015) as well as (b) model-free meta-RL approaches (Wang et al., 2016; Finn et al., 2017) to enable agents to adapt after a handful of trials. However, our work takes this adaptation ability to the extreme. Rather than adaptation requiring a few episodes of experience under the new settings, our adaptation happens online on the scale of just a few timesteps (i.e., milliseconds): so fast that it can hardly be noticed.
We achieve this fast adaptation through the use of meta-learning (discussed below) in a model-based learning setup. In the model-based setting, rather than adapting based on the rewards that are achieved during rollouts, data for updating the model is readily available at every timestep in the form of model prediction errors on recent experiences. This model-based approach enables the robot to meaningfully update the model using only a small amount of recent data.
Method Overview
Fig 3. The agent uses recent experience to fine-tune the prior model into an adapted one, which the planner then uses to perform its action selection. Note that we omit details of the update rule in this post, but we experiment with two such options in our work.
Our method follows the general formulation shown in Fig. 3 of using observations from recent data to perform adaptation of a model, and it is analogous to the overall framework of adaptive control (Sastry and Isidori, 1989; Åström and Wittenmark, 2013). The real challenge here, however, is how to successfully enable model adaptation when the models are complex, nonlinear, high-capacity function approximators (i.e., neural networks). Naively implementing SGD on the model weights is not effective, as neural networks require much larger amounts of data in order to perform meaningful learning.
Thus, we enable fast adaptation at test time by explicitly training with this adaptation objective during (meta-)training time, as explained in the following section. Once we meta-train across data from various settings in order to get this prior model (with weights denoted as ) that is good at adaptation, the robot can then adapt from this at each time step (Fig. 3) by using this prior in conjunction with recent experience to fine-tune its model to the current setting at hand, thus allowing for fast online adaptation.
Meta-training:
At any given time step , we are in state , we take action , and we end up in some resulting state according to the underlying dynamics function . The true dynamics are unknown to us, so we instead want to fit some learned dynamics model that makes predictions as well as possible on observed data points of the form . Our planner can use this estimated dynamics model in order to perform action selection.
Assuming that any detail or setting could have changed at any time step along the rollout, we consider temporally-close time steps as being able to inform us about the “task” details of our current situation: operating in different parts of the state space, enduring disturbances, attempting new goals/reward, experiencing a system malfunction, etc. Thus, in order for our model to be the most useful for planning, we want to first update it using our recently observed data.
At training time (Fig. 4), what this amounts to is selecting a consecutive sequence of (M+K) data points, using the first M to update our model weights from to , and then optimizing for this new to be good at predicting the state transitions for the next K time steps. This newly formulated loss function represents prediction error on the future K points, after adapting the weights using information from the past K points:
where
In other words, does not need to result in good dynamics predictions. Instead, needs to be such that it can use task-specific (i.e. recent) data points to quickly adapt itself into new weights that do result in good dynamics predictions. See the MAML blog post for more intuition on this formulation.
Fig 4. Meta-training procedure for obtaining a $\theta$ such that the adaptation of $\theta$ using the past $M$ timesteps of experience produces a model that performs well for the future $K$ timesteps.
Simulation Experiments
We conducted experiments on simulated robotic systems to test the ability of our method to adapt to sudden changes in the environment, as well as to generalize beyond the training environments. Note that we meta-trained all agents on some distribution of tasks/environments (see paper for details), but we then evaluated their adaptation ability on unseen and changing environments at test time. Figure 5 shows a cheetah robot that was trained on piers of varying random buoyancy, and then tested on a pier with sections of varying buoyancy in the water. This environment demonstrates the need for not only adaptation, but for fast/online adaptation. Figure 6 also demonstrates the need for online adaptation by showing an ant robot that was trained with different crippled legs, but tested on an unseen leg failure occurring part-way through a rollout. In these qualitative results below, we compare our gradient-based adaptive learner (‘GrBAL’) to a standard model-based learner (‘MB’) that was trained on the same variation of training tasks but has no explicit mechanism for adaptation.
Fig 5. Cheetah: Both methods are trained on piers of varying buoyancy. Ours is able to perform fast online adaptation at run-time to cope with changing buoyancy over the course of a new pier.
Fig 6. Ant: Both methods are trained on different joints being crippled. Ours is able to use its recent experiences to adapt its knowledge and cope with an unexpected and new malfunction in the form of a crippled leg (for a leg that was never seen as crippled during training).
The fast adaptation capabilities of this model-based meta-RL method allow our simulated robotic systems to attain substantial improvement in performance and/or sample efficiency over prior state-of-the-art methods, as well as over ablations of this method with the choice of yes/no online adaptation, yes/no meta-training, and yes/no dynamics model. Please refer to our paper for these quantitative comparisons.
Hardware Experiments
Fig 7. Our real dynamic legged millirobot, on which we successfully employ our model-based meta-reinforcement learning algorithm to enable online adaptation to disturbances and new settings such as traversing a slippery slope, accommodating payloads, accounting for pose miscalibration errors, and adjusting to a missing leg.
To highlight not only the sample efficiency of our meta reinforcement learning approach, but also the importance of fast online adaptation in the real world, we demonstrate our approach on a real dynamic legged millirobot (see Fig 7). This small 6-legged robot presents a modeling and control challenge in the form of highly stochastic and dynamic movement. This robot is an excellent candidate for online adaptation for many reasons: the rapid manufacturing techniques and numerous custom-design steps used to construct this robot make it impossible to reproduce the same dynamics each time, its linkages and other body parts deteriorate over time, and it moves very quickly and dynamically as a function of its terrain.
We meta-train this legged robot on various terrains, and we then test the agent’s learned ability to adapt online to new tasks (at run-time) including a missing leg, novel slippery terrains and slopes, miscalibration or errors in pose estimation, and new payloads to be pulled. Our hardware experiments compare our method to (a) standard model-based learning (‘MB’), with neither adaptation nor meta-learning, and well as (b) a dynamic evaluation (‘MB+DE’) comparison having adaptation, but performing the adaptation from a non-meta-learned prior. These results (Fig. 8-10) show the need for not only adaptation, but adaptation from an explicitly meta-learned prior.
Fig 8. Missing leg.
Fig 9. Payload.
Fig 10. Miscalibrated Pose.
By effectively adapting online, our method prevents drift from a missing leg, prevents sliding sideways down a slope, accounts for pose miscalibration errors, and adjusts to pulling payloads. Note that these tasks/environments share enough commonalities with the locomotion behaviors learned during the meta-training phase such that it would be useful to draw from that prior knowledge (rather than learn from scratch), but they are different enough that they do require effective online adaptation for success.
Fig 11. The ability to draw from prior knowledge as well as to learn from recent knowledge enables GrBAL (ours) to clearly outperform both MB and MB+DE when tested on environments that (1) require online adaptation and/or (2) were never seen during training.
Future Directions
This work enables online adaptation of high-capacity neural network dynamics models, through the use of meta-learning. By allowing local fine-tuning of a model starting from a meta-learned prior, we preclude the need for an accurate global model, as well as allow for fast adaptation to new situations such as unexpected environmental changes. Although we showed results of adaptation on various tasks in both simulation and hardware, there remain numerous relevant avenues for improvement.
First, although this setup of always fine-tuning from our pre-trained prior can be powerful, one limitation of this approach is that even numerous times of seeing a new setting would result in the same performance as the 1st time of seeing it. In this follow-up work, we take steps to address precisely this issue of improving over time, while simultaneously not forgetting older skills as a consequence of experiencing new ones.
Another area for improvement includes formulating conditions or an analysis of the capabilities and limitations of this adaptation: what can or cannot be adapted to, given the knowledge contained in the prior? For example, consider two humans learning to ride a bicycle who suddenly experience a slippery road. Assume that neither of them have ridden a bike before, so they have never fallen off a bike before. Human A might fall, break their wrist, and require months of physical therapy. Human B, on the other hand, might draw from his/her prior knowledge of martial arts and thus implement a good “falling” procedure (i.e., roll onto your back instead of trying to break a fall with the wrist). This is a case when both humans are trying to execute a new task, but other experiences from their prior knowledge significantly affect the result of their adaptation attempt. Thus, having some mechanism for understanding limitations of adaptation, under the existing prior, would be interesting.
We would like to thank Sergey Levine and Chelsea Finn for their feedback during the preparation of this blog post. We would also like to thank our co-authors Simin Liu, Ronald Fearing, and Pieter Abbeel. This post is based on the following paper:
- Learning to Adapt in Dynamic, Real-World Environments Through Meta-Reinforcement Learning
A Nagabandi*, I Clavera*, S Liu, R Fearing, P Abbeel, S Levine, C Finn
International Conference on Learning Representations (ICLR) 2019
Arxiv, Code, Project Page
This article was initially published on the BAIR blog, and appears here with the authors’ permission.
Using hydraulics for robots: Introduction
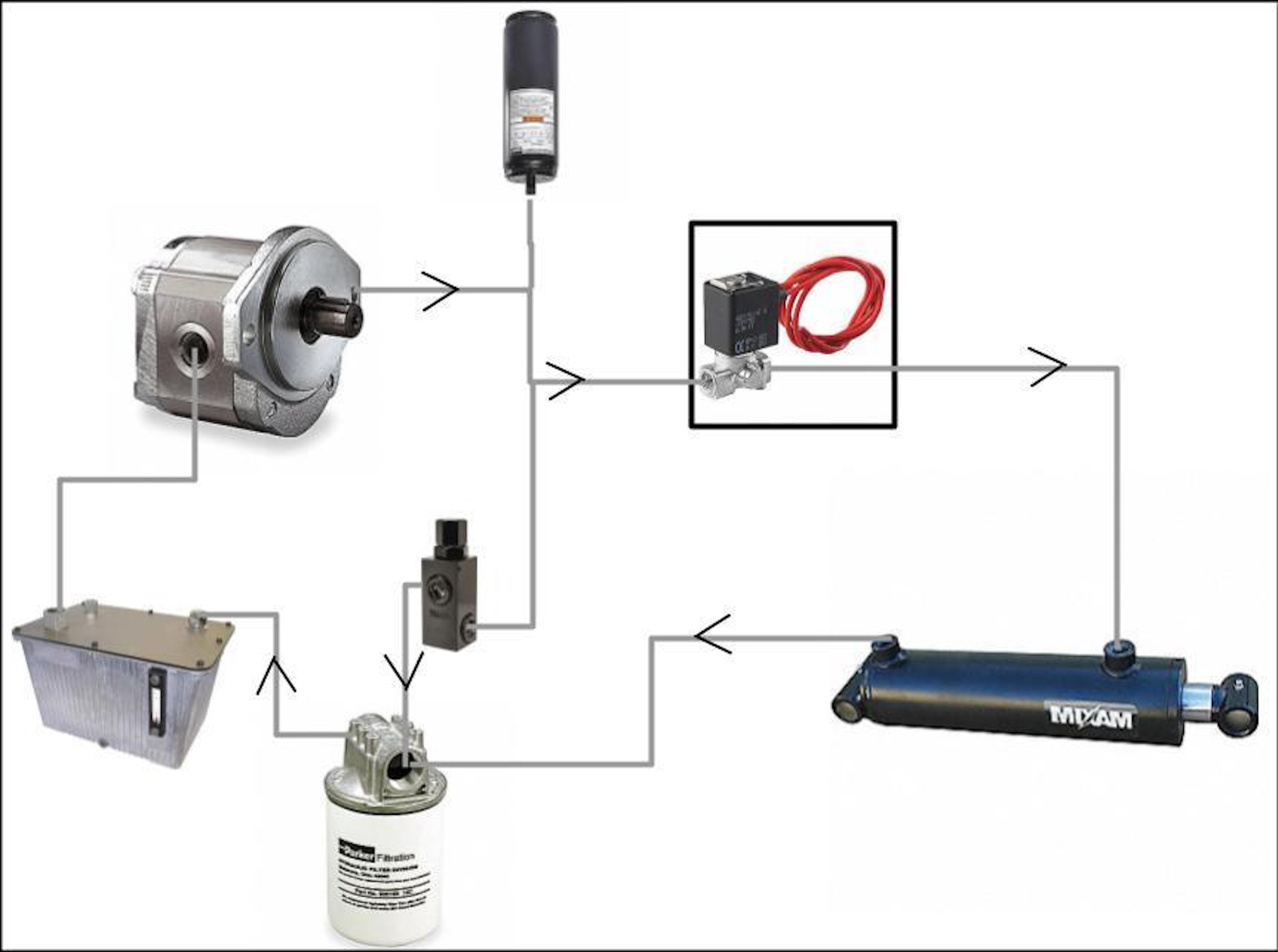
Hydraulics are sometimes looked at as an alternative to electric motors.
Some of the primary reasons for this include:
- Linear motion
- Very high torque applications
- Small package for a given torque
- Large number of motors that can share the reservoir/pump can increase volume efficiency
- You can add dampening for shock absorption
However there are also some downsides to using hydraulics including:
- More parts are required (however they can be separated from the robot in some applications)
- Less precise control (unless you use a proportional valve)
- Hydraulic fluid (mess, leaks, mess, and more mess)
Hydraulic systems use an incompressible liquid (as opposed to pneumatics that use a compressible gas) to transfer force from one place to another. Since the hydraulic system will be a closed system (ignore relief valves for now) when you apply a force to one end of the system that force is transferred to another part of that system. By manipulating the volume of fluid in different parts of the system you can change the forces in different parts of the system (Remember Pascal’s Law from high school??).
So here are some of the basic components used (or needed) to develop a hydraulic system.
Pump
The pump is the heart of your hydraulic system. The pump controls the flow and pressure of the hydraulic fluid in your system that is used for moving the actuators.
The size and speed of the pump determines the flow rate and the load at the actuator determines the pressure. For those familiar with electric motors the pressure in the system is like the voltage, and the flow rate is like the electrical current.
Pump Motor
We know what the pump is, but you need a way to “power” the pump so that it can pump the hydraulic fluid. Generally the way you power the pump is by connecting it to an electric motor or gas/diesel engine.
Hydraulic Fluid
Continuing the analogy where the pump is the heart, the hydraulic fluid is the blood of the system. The fluid is what is used to transfer the pressure from the pump to the motor.
Hydraulic Hoses (and fittings to connect things)
These are the arteries and veins of the system that allows for the transfer of hydraulic fluid.
Hydraulic Actuators – Motor/Cylinder


The actuator is generally the reason we are designing this hydraulic system. The motor is essentially the same as the pump; however instead of going from a mechanical input to generating the pressure, the motor converts the pressure into mechanical motion.
Actuators can come in the form of linear motion (referred to as a hydraulic cylinder) or rotary motion motors.
For cylinders, you generally apply a force and the cylinder end extends, and then if you release the force and the cylinder gets pushed back in (think of a car lift). This is the classic and most common use of hydraulics.
For rotary motors there are generally 3 connections on the motor.
- A – Hydraulic fluid input/output line
- B – Hydraulic fluid input/output line
- Drain – Hydraulic fluid output line (generally only on motors, not cylinders)
Depending on the motor you can either only use A as the fluid input and B as the fluid output and the motor only spins in one direction. Or some motors can spin in either direction based on if A or B is used as the input or output of the hydraulic fluid.
The drain line is used so when the system is turned off, the fluid has a way to get out of the motor (to deal with internal leakage and to not blow out seals). In some motors the drain line is connected to one of the A or B lines. Also their are sometimes multiple drain lines so that you can route the hydraulic hoses from different locations.
Note: While the pump and motor are basically the same component. You usually can not switch their role due to how they are designed to handle pressure and the pumps usually not being backdrivable.
There are some actuators that are designed to be leakless and hold the fluid and pressure (using valves) so that the force from the actuator is held even without the pump. For example these are used in things like automobile carrying trucks that need to stack cars for transport.
Reservoir
This is essentially a bucket that holds the fluid. They are usually a little fancier so that they have over pressure relief valves, lids, filters, etc..
The reservoir is also often a place where the hydraulic fluid can cool down if it is getting hot within the system. As the fluid gets hotter it can get thinner which can result in increased wear of your motor and pump.
Filter
Keeps your hydraulic fluid clean before going back to the reservoir. Kind of like a persons kidneys.
Valves (and Solenoids)

Valves are things that open and close to allow the control of fluid. These can be controlled by hand (ie. manual), or more often my some other means.
One common method is to use a solenoid which is a device that when you apply a voltage can be used to open a valve. Some solenoids are latching, which means you quickly apply a voltage and it opens the valves, and then you apply a voltage again (usually switching polarity) to close the valve.
There are many types of valves, I will detail a few below.
Check Valves (One Way Valve)
These are a type of valve that can be inline to allow the flow of hydraulic fluid in only one direction.
Relief Valve
These are a type of valve that automatically opens (And lets fluid out) when the pressure gets to high. This is a safety feature so you don’t damage other components and/or cause an explosion.
Pilot Valve
These are another special class of valve that can use a small pressure to control a much larger pressure valve.
Pressure & Flow-rate Sensors/Gauges
You need to have sensors (with a gauge or computer output) to measure the pressure and/or flow-rate so you know how the system is operating and if it is operating how you expect it to operate.
Accumulator
The accumulator is essentially just a tank that holds fluid under pressure that has its own pressure source. This is used to help smooth out the pressure and take any sudden loads from the motor by having this pressure reserve. This is almost like how capacitors are used in electrical power circuits.
The pressure source in the accumulator is often a weight, springs, or a gas.
There will often be a check valve to make sure the fluid in the accumulator does not go back to the pump.
I am not an expert on hydraulic systems. But I hope this quick introduction helps people. Liked it? Take a second to support me on Patreon!
How to tell whether machine-learning systems are robust enough for the real world
Image: MIT News Office
By Rob Matheson
MIT researchers have devised a method for assessing how robust machine-learning models known as neural networks are for various tasks, by detecting when the models make mistakes they shouldn’t.
Convolutional neural networks (CNNs) are designed to process and classify images for computer vision and many other tasks. But slight modifications that are imperceptible to the human eye — say, a few darker pixels within an image — may cause a CNN to produce a drastically different classification. Such modifications are known as “adversarial examples.” Studying the effects of adversarial examples on neural networks can help researchers determine how their models could be vulnerable to unexpected inputs in the real world.
For example, driverless cars can use CNNs to process visual input and produce an appropriate response. If the car approaches a stop sign, it would recognize the sign and stop. But a 2018 paper found that placing a certain black-and-white sticker on the stop sign could, in fact, fool a driverless car’s CNN to misclassify the sign, which could potentially cause it to not stop at all.
However, there has been no way to fully evaluate a large neural network’s resilience to adversarial examples for all test inputs. In a paper they are presenting this week at the International Conference on Learning Representations, the researchers describe a technique that, for any input, either finds an adversarial example or guarantees that all perturbed inputs — that still appear similar to the original — are correctly classified. In doing so, it gives a measurement of the network’s robustness for a particular task.
Similar evaluation techniques do exist but have not been able to scale up to more complex neural networks. Compared to those methods, the researchers’ technique runs three orders of magnitude faster and can scale to more complex CNNs.
The researchers evaluated the robustness of a CNN designed to classify images in the MNIST dataset of handwritten digits, which comprises 60,000 training images and 10,000 test images. The researchers found around 4 percent of test inputs can be perturbed slightly to generate adversarial examples that would lead the model to make an incorrect classification.
“Adversarial examples fool a neural network into making mistakes that a human wouldn’t,” says first author Vincent Tjeng, a graduate student in the Computer Science and Artificial Intelligence Laboratory (CSAIL). “For a given input, we want to determine whether it is possible to introduce small perturbations that would cause a neural network to produce a drastically different output than it usually would. In that way, we can evaluate how robust different neural networks are, finding at least one adversarial example similar to the input or guaranteeing that none exist for that input.”
Joining Tjeng on the paper are CSAIL graduate student Kai Xiao and Russ Tedrake, a CSAIL researcher and a professor in the Department of Electrical Engineering and Computer Science (EECS).
CNNs process images through many computational layers containing units called neurons. For CNNs that classify images, the final layer consists of one neuron for each category. The CNN classifies an image based on the neuron with the highest output value. Consider a CNN designed to classify images into two categories: “cat” or “dog.” If it processes an image of a cat, the value for the “cat” classification neuron should be higher. An adversarial example occurs when a tiny modification to that image causes the “dog” classification neuron’s value to be higher.
The researchers’ technique checks all possible modifications to each pixel of the image. Basically, if the CNN assigns the correct classification (“cat”) to each modified image, no adversarial examples exist for that image.
Behind the technique is a modified version of “mixed-integer programming,” an optimization method where some of the variables are restricted to be integers. Essentially, mixed-integer programming is used to find a maximum of some objective function, given certain constraints on the variables, and can be designed to scale efficiently to evaluating the robustness of complex neural networks.
The researchers set the limits allowing every pixel in each input image to be brightened or darkened by up to some set value. Given the limits, the modified image will still look remarkably similar to the original input image, meaning the CNN shouldn’t be fooled. Mixed-integer programming is used to find the smallest possible modification to the pixels that could potentially cause a misclassification.
The idea is that tweaking the pixels could cause the value of an incorrect classification to rise. If cat image was fed in to the pet-classifying CNN, for instance, the algorithm would keep perturbing the pixels to see if it can raise the value for the neuron corresponding to “dog” to be higher than that for “cat.”
If the algorithm succeeds, it has found at least one adversarial example for the input image. The algorithm can continue tweaking pixels to find the minimum modification that was needed to cause that misclassification. The larger the minimum modification — called the “minimum adversarial distortion” — the more resistant the network is to adversarial examples. If, however, the correct classifying neuron fires for all different combinations of modified pixels, then the algorithm can guarantee that the image has no adversarial example.
“Given one input image, we want to know if we can modify it in a way that it triggers an incorrect classification,” Tjeng says. “If we can’t, then we have a guarantee that we searched across the whole space of allowable modifications, and found that there is no perturbed version of the original image that is misclassified.”
In the end, this generates a percentage for how many input images have at least one adversarial example, and guarantees the remainder don’t have any adversarial examples. In the real world, CNNs have many neurons and will train on massive datasets with dozens of different classifications, so the technique’s scalability is critical, Tjeng says.
“Across different networks designed for different tasks, it’s important for CNNs to be robust against adversarial examples,” he says. “The larger the fraction of test samples where we can prove that no adversarial example exists, the better the network should perform when exposed to perturbed inputs.”
“Provable bounds on robustness are important as almost all [traditional] defense mechanisms could be broken again,” says Matthias Hein, a professor of mathematics and computer science at Saarland University, who was not involved in the study but has tried the technique. “We used the exact verification framework to show that our networks are indeed robust … [and] made it also possible to verify them compared to normal training.”