CES 2019 Proves AI and 5G Will Transform the Future
Trust Region and Proximal policy optimization (TRPO and PPO)
Advancements in Robotics: Predictions for 2019
Kindred Hospital Rehabilitation Services Purchasing Robots for Stroke Therapy
Velodyne Lidar Discusses the Future of Self-Driving Cars with Next-Generation Engineers
Autonomous robot that interacts with humans using natural language and vision processing
#277: Presented work at IROS 2018 (Part 3 of 3), with Pauline Pounds, Philippe Morere and Yujung Liu
In this episode, Audrow Nash interviews Pauline Pound, Philippe Morere, and Yujung Liu about the work they presented at the 2018 International Conference on Intelligent Robots and Systems (IROS) in Madrid, Spain.
Pauline Pounds, Associate Professor at the University of Queensland, speaks about building robots that can endure children. She discusses the tradeoffs of designing a robot that can survive children and cannot harm the children. Pounds talks about how the robot has performed with children so far, the hardware design, and her future direction with this work.
Philippe Morere, a PhD student at University of Sydney, speaks about reinforcement learning in partially observable environments. Morere discusses the intuition behind this work and the problems that he solved using this approach.
Yujung Liu, a master’s student from the National Taiwan University, speaks about a painting robot. He discusses how the portrait is constructed, including how colors are chosen, how the features are made cartoon-like, and his future direction.
Links
- Download mp3 (11.3 MB)
- Subscribe to Robots using iTunes
- Subscribe to Robots using RSS
- Support us on Patreon
Hundreds of Tiny Robots Grow Bio-inspired Shapes
Robot Sensor Market Outlook
How Utilities can use Drones in Planning a Resilient Power Supply
OMRON Showcases The Harmony Between Humans And Machines With The Largest, Most Advanced Interactive Exhibit Of AI, Robotics & Automation At CES 2019
Breadmaking robot startup eyes fresh connections
A safe, wearable soft sensor
By Leah Burrows
Children born prematurely often develop neuromotor and cognitive developmental disabilities. The best way to reduce the impacts of those disabilities is to catch them early through a series of cognitive and motor tests. But accurately measuring and recording the motor functions of small children is tricky. As any parent will tell you, toddlers tend to dislike wearing bulky devices on their hands and have a predilection for ingesting things they shouldn’t.
Harvard University researchers have developed a soft, non-toxic wearable sensor that unobtrusively attaches to the hand and measures the force of a grasp and the motion of the hand and fingers.
The research was published in Advanced Functional Materials and is a collaboration between the Wyss Institute for Biologically Inspired Engineering, The Harvard John A. Paulson School of Engineering and Applied Sciences (SEAS), Beth Israel Deaconess Medical Center, and Boston Children’s Hospital.
One novel element of the sensor is a non-toxic, highly conductive liquid solution.
“We have developed a new type of conductive liquid that is no more dangerous than a small drop of salt water,” said Siyi Xu, a graduate student at SEAS and first author of the paper. “It is four times more conductive than previous biocompatible solutions, leading to cleaner, less noisy data.”
The sensing solution is made from potassium iodide, which is a common dietary supplement, and glycerol, which is a common food additive. After a short mixing period, the glycerol breaks the crystal structure of potassium iodide and forms potassium cations (K+) and iodide ions (I-), making the liquid conductive. Because glycerol has a lower evaporation rate than water, and the potassium iodide is highly soluble, the liquid is both stable across a range of temperatures and humidity levels, and highly conductive.
“Previous biocompatible soft sensors have been made using sodium chloride-glycerol solutions but these solutions have low conductivities, which makes the sensor data very noisy, and it also takes about 10 hours to prepare,” said Xu. “We’ve shortened that down to about 20 minutes and get very clean data.”
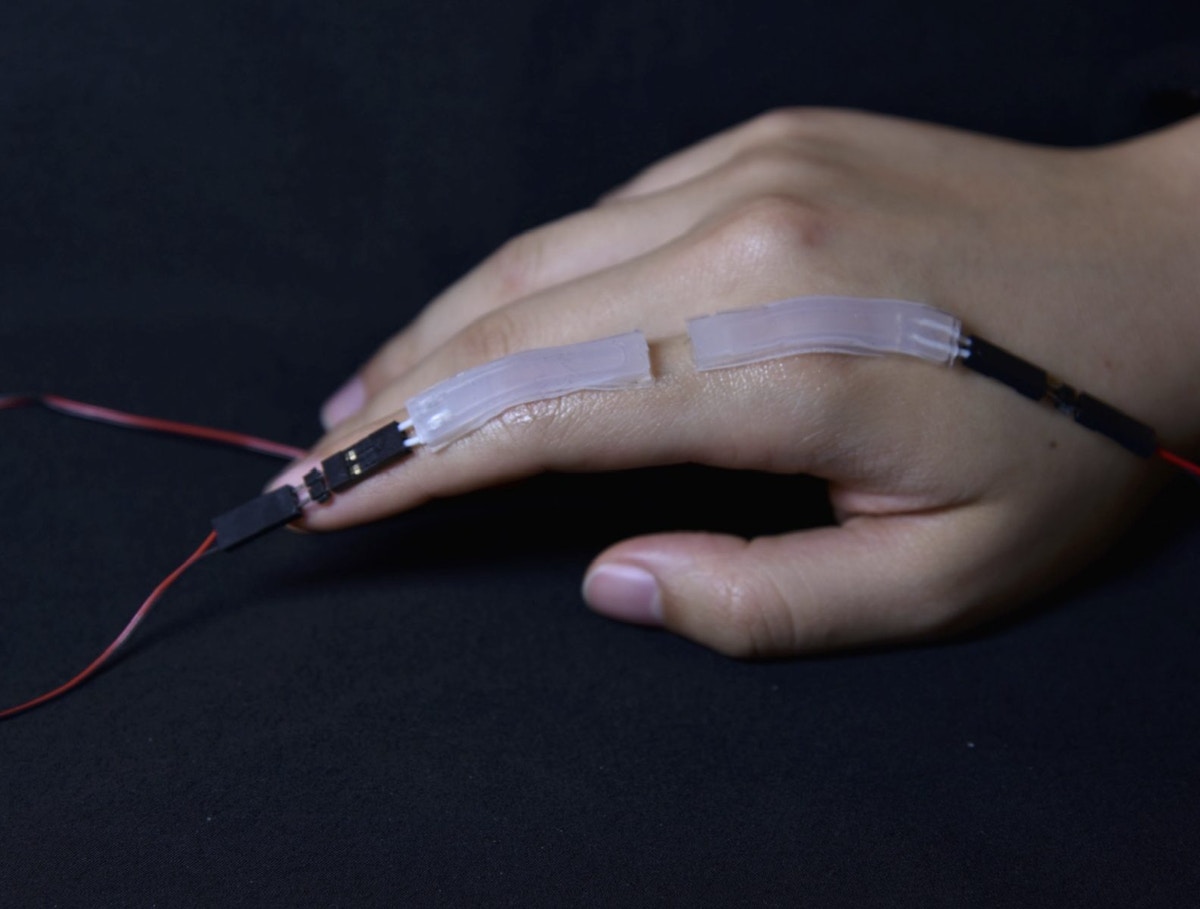
Safe, soft sensors on the top and tip of the index finger detect the movements, strain and force of the finger while performing different activities, such as flexing and extending the finger and picking up weights and boxes. Credit: Harvard SEAS
The design of the sensors also takes the need of children into account. Rather than a bulky glove, the silicon-rubber sensor sits on top of the finger and on the finger pad.
“We often see that children who are born early or who have been diagnosed with early developmental disorders have highly sensitive skin,” said Eugene Goldfield, Ph.D., coauthor of the study, and Associate Faculty Member of the Wyss Institute at Harvard University and an Associate Professor in the Program in Behavioral Sciences at Boston Children’s Hospital and Harvard Medical School. “By sticking to the top of the finger, this device gives accurate information while getting around the sensitively of the child’s hand.”
Goldfield is the Principal investigator of the Flexible Electronics for Toddlers project at the Wyss Institute, which designs modular robotic systems for toddlers born prematurely and at risk for cerebral palsy.
Goldfield and his colleagues currently study motor function using the Motion Capture Lab at SEAS and Wyss. While motion capture can tell a lot about movement, it cannot measure force, which it critical to diagnosing neuromotor and cognitive developmental disabilities.
“Early diagnosis is the name of the game when it comes to treating these developmental disabilities and this wearable sensor can give us a lot of advantages not currently available,” said Goldfield.
This paper only tested the device on adult hands. Next, the researchers plan to scale down the device and test it on the hands of children.
“The ability to quantify complex human motions gives us an unprecedented diagnostic tool,” says senior author Robert Wood, Ph.D., Founding Core Faculty Member of the Wyss Institute and the Charles River Professor of Engineering and Applied Sciences at SEAS. “The focus on the development of motor skills in toddlers presents unique challenges for how to integrate many sensors into a small, lightweight, and unobtrusive wearable device. These new sensors solve these challenges – and if we can create wearable sensors for such a demanding task, we believe that this will also open up applications in diagnostics, therapeutics, human-computer interfaces, and virtual reality.”
This research was also authored by SEAS researcher Daniel M. Vogt; Wyss Institute researchers Wen-Hao Hsu, John Osborne, Timothy Walsh, Jonathan R. Foster, Sarah K. Sullivan, and Andreas Rousing; and Beth Israel Deaconess Medical Center researcher Vincent C. Smith. It was supported by the National Institutes of Health.
PUBLICATION – Advanced Functional Materials : Biocompatible Soft Fluidic Strain and Force Sensors for Wearable Devices
WYSS TECHNOLOGY – “Flexi-mitts”for tracking neurodevelopment in very low birth weight premature infants
How robots are helping doctors save lives in the Canadian North
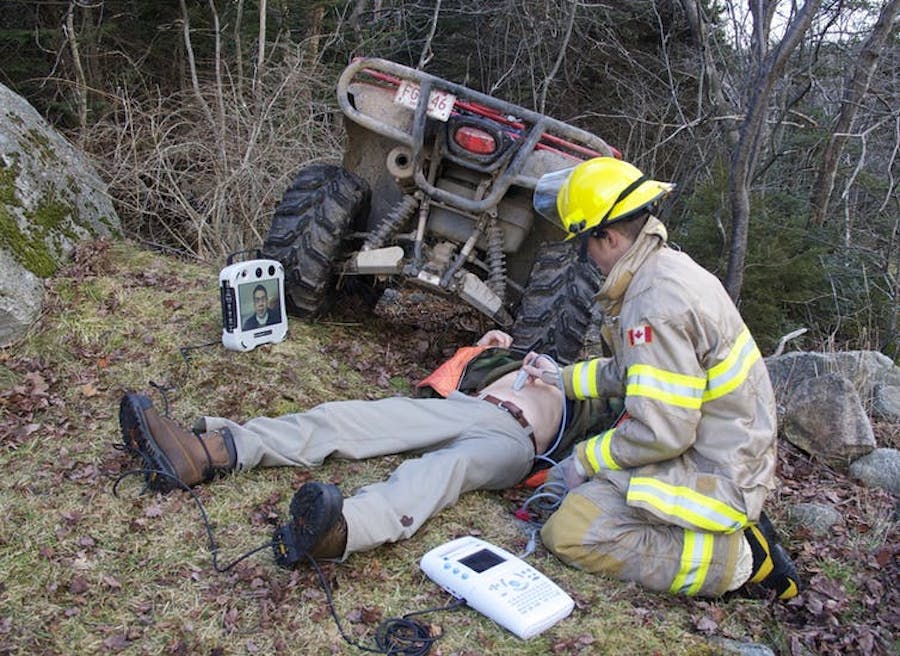
(University of Saskatchewan), Author provided
Ivar Mendez, University of Saskatchewan
It is the middle of the winter and a six-month-old child is brought with acute respiratory distress to a nursing station in a remote community in the Canadian North.
The nurse realizes that the child is seriously ill and contacts a pediatric intensivist located in a tertiary care centre 900 kilometres away. The intensivist uses her tablet to activate a remote presence robot installed in the nursing station and asks the robot to go to the assessment room.
The robot autonomously navigates the nursing station corridors and arrives at the assessment room two minutes later. With the help of the robot’s powerful cameras, the doctor “sees” the child and talks to the nurse and the parents to obtain the medical history. She uses the robot’s stethoscope to listen to the child’s chest, measures the child’s oxygen blood saturation with a pulse oximeter and performs an electrocardiogram.
With the robot’s telestrator (an electronic device which enables the user to write and draw freehand over a video image) she helps the nurse to start an intravenous line and commences therapy to treat the child’s life-threatening condition.
This is not science fiction. This remote presence technology is currently in use in Saskatchewan, Canada — to provide care to acutely ill children living in remote Northern communities.
Treating acutely ill children
Advances in telecommunication, robotics, medical sensor technology and artificial intelligence (AI) have opened the door for solutions to the challenge of delivering remote, real-time health care to underserviced rural and remote populations.
(University of Saskatchewan), Author provided
In Saskatchewan, we have established a remote medicine program that focuses on the care of the most vulnerable populations — such as acutely ill children, pregnant women and the elderly.
We have demonstrated that with this technology about 70 per cent of acutely ill children can be successfully treated in their own communities. In similar communities without this technology, all acutely ill children need to be transported to a tertiary care centre.
We have also shown that this technology prevents delays in diagnosis and treatment and results in substantial savings to the health-care system.
Prenatal ultrasounds for Indigenous women
Remote communities often lack access to diagnostic ultrasonography services. This gap disproportionally affects Indigenous pregnant women in the Canadian North and results in increases in maternal and newborn morbidity and mortality.
We are pioneering the use of an innovative tele-robotic ultrasound system that allows an expert sonographer to perform a diagnostic ultrasound study, in real time, in a distant location.
Research shows that robotic ultrasonography is comparable to standard sonography and is accepted by most patients.
The first tele-robotic ultrasonography systems have been deployed to two northern Saskatchewan communities and are currently performing prenatal ultrasounds.
Emergency room trauma assessment
Portable remote presence devices that use available cellular networks could also be used in emergency situations, such as trauma assessment at the scene of an accident or transport of a victim to hospital.
For example, emergency physicians or trauma surgeons could perform real-time ultrasonography of the abdomen, thorax and heart in critically injured patients, identify life-threatening injuries and start life-saving treatment.
Wearable remote presence devices such a Google Glass technology are the next step in remote presence health care for underserviced populations.
For example, a local nurse and a specialist in a tertiary care centre thousand of kilometres away could assess together an acutely ill patient in an emergency room in a remote community through the nurse’s eyes.
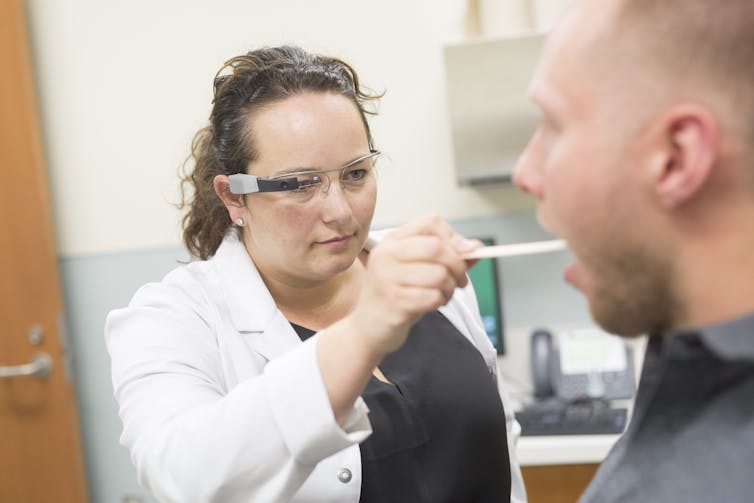
(University of Saskatchewan), Author provided
Although remote presence technology may be applied initially to emergency situations in remote locations, its major impact may be in the delivery of primary health care. We can imagine the use of mobile remote presence devices by health professionals in a wide range of scenarios — from home-care visits to follow-up mental health sessions — in which access to medical expertise in real time would be just a computer click away.
A paradigm shift in health-care delivery
The current model of centralized health care, where the patient has to go to a hospital or a clinic to receive urgent or elective medical care, is inefficient and costly. Patients have to wait many hours in emergency rooms. Hospitals run at overcapacity. Delays in diagnosis and treatment cause poor outcomes or even death.
Underserviced rural and remote communities and the most vulnerable populations such as children and the elderly are the most affected by this centralized model.
Remote presence technologies have the potential to shift this — so that we can deliver medical care to a patient anywhere. In this decentralized model, patients requiring urgent or elective medical care will be seen, diagnosed and treated in their own communities or homes and patients requiring hospitalization will be triaged without delay.
This technology could have important applications in low-resource settings. Cellular network signals around the globe and rapidly increasing bandwidth will provide the telecommunication platform for a wide range of mobile applications.
Low-cost, dedicated remote-presence devices will increase access to medical expertise for anybody living in a geographical area with a cellphone signal. This access will be especially beneficial to people in developing countries where medical expertise is insufficient or not available.
The future of medical care is not in building more or bigger hospitals but in harnessing the power of technology to monitor and reach patients wherever they are — to preserve life, ensure wellness and speed up diagnosis and treatment.
Ivar Mendez, Fred H. Wigmore Professor and Unified Head of the Department of Surgery, University of Saskatchewan
This article is republished from The Conversation under a Creative Commons license. Read the original article.