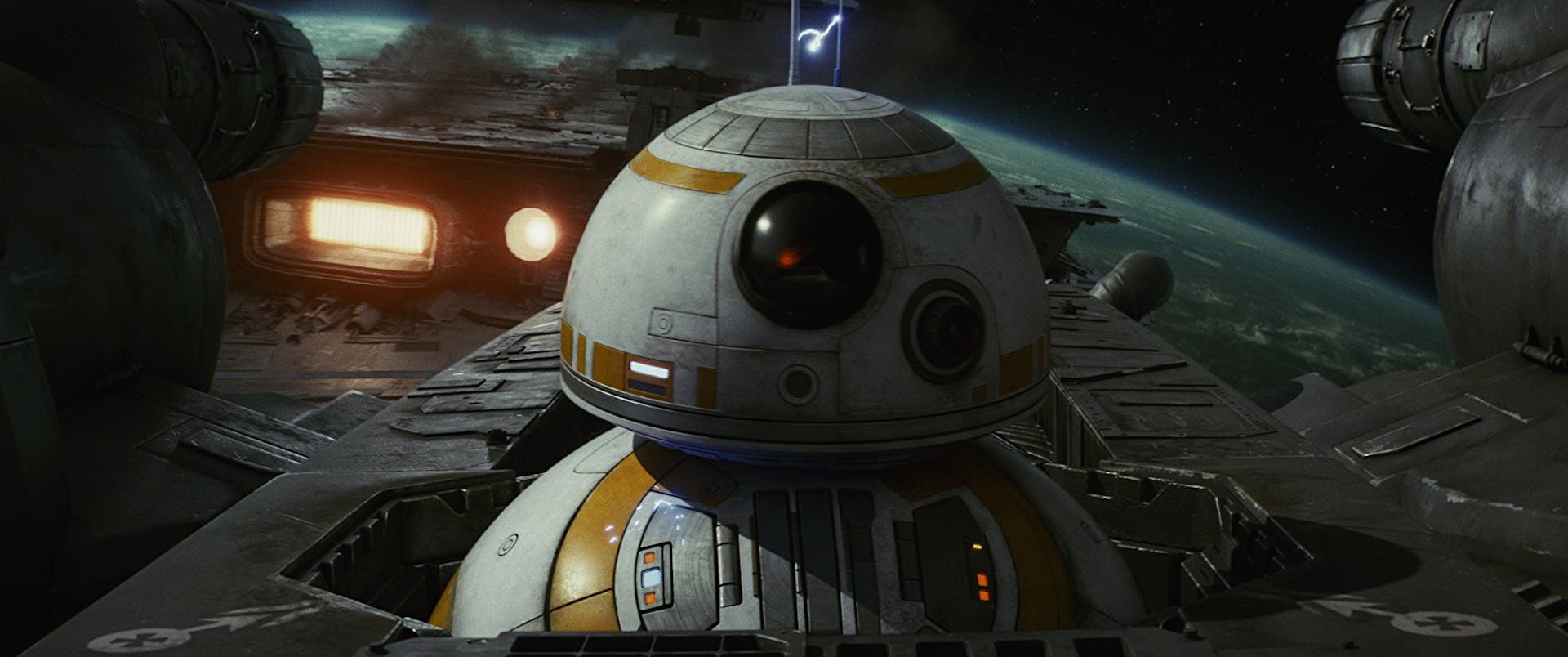
Lucasfilm/IMDB
By Paul Salmon, University of the Sunshine Coast
Millions of fans all over the world eagerly anticipated this week’s release of Star Wars: The Last Jedi, the eighth in the series. At last we will get some answers to questions that have been vexing us since 2015’s The Force Awakens.
Throughout the franchise, the core characters have been accompanied by a number of much-loved robots, including C-3PO, R2-D2 and more recently, BB-8 and K2-SO. While often fulfilling the role of wise-cracking sidekicks, these and other robots also play an integral role in events.
Interestingly, they can also tell us useful things about automation, such as whether it poses dangers to us and whether robots will ever replace human workers entirely. In these films, we see the good, bad and ugly of robots – and can thus glean clues about what our technological future might look like.
The fear of replacement
One major fear is that robots and automation will replace us, despite work design principles that tell us technology should be used as a tool to assist, rather than replace, humans. In the world of Star Wars, robots (or droids as they are known) mostly assist organic lifeforms, rather than completely replace them.
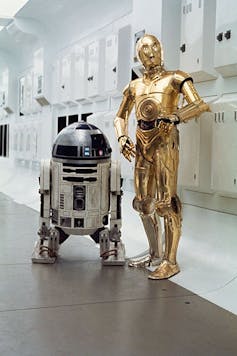
Lucasfilms/IMDB
So for instance, C-3PO is a protocol droid who was designed to assist in translation, customs and etiquette. R2-D2 and the franchise’s new darling, BB-8, are both “astromech droids” designed to assist in starship maintenance.
In the most recent movie, Rogue One, an offshoot of the main franchise, we were introduced to K2-SO, a wisecracking advanced autonomous military robot who was caught and reprogrammed to switch allegiance to the rebels. K2-SO mainly acts as a co-pilot, for example when flying a U-Wing with the pilot Cassian Andor to the planet of Eadu.
In most cases then, the Star Wars droids provide assistance – co-piloting ships, helping to fix things, and even serving drinks. In the world of these films, organic lifeforms are still relied upon for most skilled work.
When organic lifeforms are completely replaced, it is generally when the work is highly dangerous. For instance, during the duel between Annakin and Obi Wan on the planet Mustafar in Revenge of the Sith, DLC-13 mining droids can be seen going about their work in the planet’s hostile lava rivers.
Further, droid armies act as the frontline in various battles throughout the films. Perhaps, in the future, we will be OK with losing our jobs if the work in question poses a significant risk to our health.
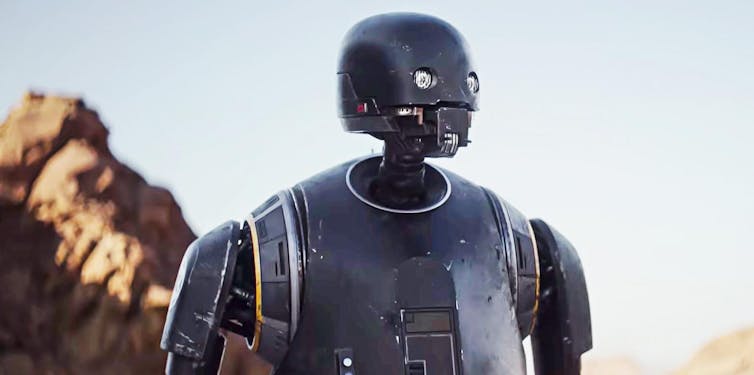
Lucasfilm/IMDB
However, there are some exceptions to this trend in the Star Wars universe. In the realm of healthcare, for instance, droids have fully replaced organic lifeforms. In The Empire Strikes Back a medical droid treats Luke Skywalker after his encounter with a Wampa, a yeti-like snow beast on the planet Hoth. The droid also replaces his hand following his battle with Darth Vadar on the planet Bespin.
Likewise, in Revenge of the Sith, a midwife droid is seen delivering the siblings Luke and Leia on Polis Massa.
Perhaps this is one area in which Star Wars has it wrong: here on earth, full automation is a long way off in healthcare. Assistance from robots in healthcare is the more realistic prospect and is in fact, already here. Indeed, robots have been assisting surgeons in operating theatres for some time now.
Automated vehicles
Driverless vehicles are currently flavour of the month – but will we actually use them? In Star Wars, despite the capacity for spacecraft and star ships to be fully automated, organic lifeforms still take the controls. The spaceship Millenium Falcon, for example, is mostly flown by the smuggler Han Solo and his companion Chewbacca.
Most of the Star Wars starship fleet (A-Wings, X-Wings, Y-Wings, Tie Fighters, Star Destroyers, Starfighters and more) ostensibly possess the capacity for fully automated flight, however, they are mostly flown by organic lifeforms. In The Phantom Menace the locals on Tatooine have even taken to building and manually racing their own “pod racers”.
It seems likely that here on earth, humans too will continue to prefer to drive, fly, sail, and ride. Despite the ability to fully automate, most people will still want to be able to take full control.
Flawless, error proof robots?
Utopian visions often depict a future where sophisticated robots will perform highly skilled tasks, all but eradicating the costly errors that humans make. This is unlikely to be true.
A final message from the Star Wars universe is that the droids and advanced technologies are often far from perfect. In our own future, costly human errors may simply be replaced by robot designer errors.
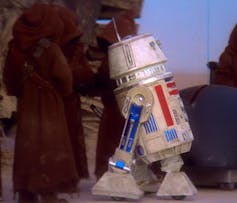
Lucasfilms/IMDB
The B1 Battle Droids seen in the first and second Star Wars films lack intelligence and frequently malfunction. C-3PO is notoriously error prone and his probability-based estimates are often wide of the mark.
In the fourth film, A New Hope, R5-D4 (another astromech droid) malfunctions and explodes just as the farmer Owen Lars is about to buy it. Other droids are slow and clunky, such as the GNK Power droid and HURID-327, the groundskeeper at the castle of Maz Kanata in The Force Awakens.
The much feared scenario, whereby robots become so intelligent that they eventually take over, is hard to imagine with this lot.
Perhaps the message from the Star Wars films is that we need to lower our expectations of robot capabilities, in the short term at least. Cars will still crash, mistakes will still be made, regardless of whether humans or robots are doing the work.
Paul Salmon, Professor of Human Factors, University of the Sunshine Coast
This article was originally published on The Conversation. Read the original article.