In this episode of Robots in Depth, Per Sjöborg speaks with Federico Pecora about AI and robotics. Federico Pecora is Associate Professor in Computer Science at the Center for Applied Autonomous Sensor Systems at Örebro University, Sweden.
Professor Emeritus Fernando Corbató, MIT computing pioneer, dies at 93
Image: Computer History Museum
By Adam Conner-Simons | Rachel Gordon
Fernando “Corby” Corbató, an MIT professor emeritus whose work in the 1960s on time-sharing systems broke important ground in democratizing the use of computers, died on Friday, July 12, at his home in Newburyport, Massachusetts. He was 93.
Decades before the existence of concepts like cybersecurity and the cloud, Corbató led the development of one of the world’s first operating systems. His “Compatible Time-Sharing System” (CTSS) allowed multiple people to use a computer at the same time, greatly increasing the speed at which programmers could work. It’s also widely credited as the first computer system to use passwords.
After CTSS Corbató led a time-sharing effort called Multics, which directly inspired operating systems like Linux and laid the foundation for many aspects of modern computing. Multics doubled as a fertile training ground for an emerging generation of programmers that included C programming language creator Dennis Ritchie, Unix developer Ken Thompson, and spreadsheet inventors Dan Bricklin and Bob Frankston.
Before time-sharing, using a computer was tedious and required detailed knowledge. Users would create programs on cards and submit them in batches to an operator, who would enter them to be run one at a time over a series of hours. Minor errors would require repeating this sequence, often more than once.
But with CTSS, which was first demonstrated in 1961, answers came back in mere seconds, forever changing the model of program development. Decades before the PC revolution, Corbató and his colleagues also opened up communication between users with early versions of email, instant messaging, and word processing.
“Corby was one of the most important researchers for making computing available to many people for many purposes,” says long-time colleague Tom Van Vleck. “He saw that these concepts don’t just make things more efficient; they fundamentally change the way people use information.”
Besides making computing more efficient, CTSS also inadvertently helped establish the very concept of digital privacy itself. With different users wanting to keep their own files private, CTSS introduced the idea of having people create individual accounts with personal passwords. Corbató’s vision of making high-performance computers available to more people also foreshadowed trends in cloud computing, in which tech giants like Amazon and Microsoft rent out shared servers to companies around the world.
“Other people had proposed the idea of time-sharing before,” says Jerry Saltzer, who worked on CTSS with Corbató after starting out as his teaching assistant. “But what he brought to the table was the vision and the persistence to get it done.”
CTSS was also the spark that convinced MIT to launch “Project MAC,” the precursor to the Laboratory for Computer Science (LCS). LCS later merged with the Artificial Intelligence Lab to become MIT’s largest research lab, the Computer Science and Artificial Intelligence Laboratory (CSAIL), which is now home to more than 600 researchers.
“It’s no overstatement to say that Corby’s work on time-sharing fundamentally transformed computers as we know them today,” says CSAIL Director Daniela Rus. “From PCs to smartphones, the digital revolution can directly trace its roots back to the work that he led at MIT nearly 60 years ago.”
In 1990 Corbató was honored for his work with the Association of Computing Machinery’s Turing Award, often described as “the Nobel Prize for computing.”
From sonar to CTSS
Corbató was born on July 1, 1926 in Oakland, California. At 17 he enlisted as a technician in the U.S. Navy, where he first got the engineering bug working on a range of radar and sonar systems. After World War II he earned his bachelor’s degree at Caltech before heading to MIT to complete a PhD in physics.
As a PhD student, Corbató met Professor Philip Morse, who recruited him to work with his team on Project Whirlwind, the first computer capable of real-time computation. After graduating, Corbató joined MIT’s Computation Center as a research assistant, soon moving up to become deputy director of the entire center.
It was there that he started thinking about ways to make computing more efficient. For all its innovation, Whirlwind was still a rather clunky machine. Researchers often had trouble getting much work done on it, since they had to take turns using it for half-hour chunks of time. (Corbató said that it had a habit of crashing every 20 minutes or so.)
Since computer input and output devices were much slower than the computer itself, in the late 1950s a scheme called multiprogramming was developed to allow a second program to run whenever the first program was waiting for some device to finish. Time-sharing built on this idea, allowing other programs to run while the first program was waiting for a human user to type a request, thus allowing the user to interact directly with the first program.
Saltzer says that Corbató pioneered a programming approach that would be described today as agile design.
“It’s a buzzword now, but back then it was just this iterative approach to coding that Corby encouraged and that seemed to work especially well,” he says.
In 1962 Corbató published a paper about CTSS that quickly became the talk of the slowly-growing computer science community. The following year MIT invited several hundred programmers to campus to try out the system, spurring a flurry of further research on time-sharing.
Foreshadowing future technological innovation, Corbató was amazed — and amused — by how quickly people got habituated to CTSS’ efficiency.
“Once a user gets accustomed to [immediate] computer response, delays of even a fraction of a minute are exasperatingly long,” he presciently wrote in his 1962 paper. “First indications are that programmers would readily use such a system if it were generally available.”
Multics, meanwhile, expanded on CTSS’ more ad hoc design with a hierarchical file system, better interfaces to email and instant messaging, and more precise privacy controls. Peter Neumann, who worked at Bell Labs when they were collaborating with MIT on Multics, says that its design prevented the possibility of many vulnerabilities that impact modern systems, like “buffer overflow” (which happens when a program tries to write data outside the computer’s short-term memory).
“Multics was so far ahead of the rest of the industry,” says Neumann. “It was intensely software-engineered, years before software engineering was even viewed as a discipline.”
In spearheading these time-sharing efforts, Corbató served as a soft-spoken but driven commander in chief — a logical thinker who led by example and had a distinctly systems-oriented view of the world.
“One thing I liked about working for Corby was that I knew he could do my job if he wanted to,” says Van Vleck. “His understanding of all the gory details of our work inspired intense devotion to Multics, all while still being a true gentleman to everyone on the team.”
Another legacy of the professor’s is “Corbató’s Law,” which states that the number of lines of code someone can write in a day is the same regardless of the language used. This maxim is often cited by programmers when arguing in favor of using higher-level languages.
Corbató was an active member of the MIT community, serving as associate department head for computer science and engineering from 1974 to 1978 and 1983 to 1993. He was a member of the National Academy of Engineering, and a fellow of the Institute of Electrical and Electronics Engineers and the American Association for the Advancement of Science.
Corbató is survived by his wife, Emily Corbató, from Brooklyn, New York; his stepsons, David and Jason Gish; his brother, Charles; and his daughters, Carolyn and Nancy, from his marriage to his late wife Isabel; and five grandchildren.
In lieu of flowers, gifts may be made to MIT’s Fernando Corbató Fellowship Fund via Bonny Kellermann in the Memorial Gifts Office.
CSAIL will host an event to honor and celebrate Corbató in the coming months.
Concrete Choreography
The installation Concrete Choreography presents the first robotically 3D printed concrete stage, consisting of columns fabricated without formwork and printed in full height within 2.5 hours. Robotic concrete printing allows customised fabrication of complex components that uses concrete more efficiently.
In collaboration with the Origen Festival in Riom, Switzerland, the installation consists of 9, 2.7m columns, individually designed with custom software and fabricated with a new robotic concrete 3D printing process developed at ETH Zurich. Students of the Master of Advanced Studies in Digital Fabrication and Architecture explore the unique possibilities of 3D printing with an age-old material, demonstrating the potential of computational design and digital fabrication for future construction.
This novel fabrication process allows the production of concrete elements without the need for any formwork. In addition, one-of-a-kind designs with complex geometries can be fabricated in a fully automated manner. Hollow concrete structures are printed in a way where the material can be strategically used only where needed, allowing a more sustainable approach to concrete architecture.
Computationally designed material ornament and surface texture exemplify the versatility and significant aesthetic potential of 3D concrete printing when used in large-scale structures.
Framing and informing the dance performances of the summer season in Riom, the project demonstrates how technological advancements can bring efficient and novel expressions to concrete architecture.
One column in numbers:
Column Height: 2.70 m
Print-path length: 1600 m
Print-time: 2.5 h
Print-speed: 180 mm/sec
Layer width: 25 mm
Layer height: 5 mm
Project Credits
Digital Building Technologies, ETH Zurich Prof. Benjamin Dillenburger
MAS DFAB in Architecture and Digital Fabrication | ETH Zurich
Teaching Team Ana Anton, Patrick Bedarf, Angela Yoo (Digital Building Technologies), Timothy Wangler (Physical Chemistry of Building Materials)
Students Antonio Barney, Aya Shaker Ali, Chaoyu Du, Eleni Skevaki, Jonas Van den Bulcke, Keerthana Udaykumar, Nicolas Feihl, Nik Eftekhar Olivo, Noor Khader, Rahul Girish, Sofia Michopoulou, Ying-Shiuan Chen, Yoana Taseva, Yuta Akizuki, Wenqian Yang
Origen Foundation Giovanni Netzer, Irene Gazzillo, Guido Luzio, Flavia Kistler
Research Partners Prof. Robert J. Flatt, Lex Reiter, Timothy Wangler (Physical Chemistry of Building Materials, ETH Zurich)
Technical Support Michael Lyrenmann, Philippe Fleischmann, Andreas Reusser, Heinz Richner
Supported by Debrunner Acifer Bewehrungen AG, LafargeHolcim, Elotex, Imerys Aluminates
The concrete Choreography project was recently featured on SRF’s Kulturplatz programme, and you can catch up with it online (in German). The project is also featured in Zurich’s Museum für Gestaltung as part of the Designlabor: Material und Technik exhibition.
Photo: Benjamin Hofer
Automated system generates robotic parts for novel tasks
Credit: Subramanian Sundaram
By Rob Matheson
An automated system developed by MIT researchers designs and 3-D prints complex robotic parts called actuators that are optimized according to an enormous number of specifications. In short, the system does automatically what is virtually impossible for humans to do by hand.
In a paper published today in Science Advances, the researchers demonstrate the system by fabricating actuators — devices that mechanically control robotic systems in response to electrical signals — that show different black-and-white images at different angles. One actuator, for instance, portrays a Vincent van Gogh portrait when laid flat. Tilted an angle when it’s activated, however, it portrays the famous Edvard Munch painting “The Scream.” The researchers also 3-D printed floating water lilies with petals equipped with arrays of actuators and hinges that fold up in response to magnetic fields run through conductive fluids.
The actuators are made from a patchwork of three different materials, each with a different light or dark color and a property — such as flexibility and magnetization — that controls the actuator’s angle in response to a control signal. Software first breaks down the actuator design into millions of three-dimensional pixels, or “voxels,” that can each be filled with any of the materials. Then, it runs millions of simulations, filling different voxels with different materials. Eventually, it lands on the optimal placement of each material in each voxel to generate two different images at two different angles. A custom 3-D printer then fabricates the actuator by dropping the right material into the right voxel, layer by layer.
“Our ultimate goal is to automatically find an optimal design for any problem, and then use the output of our optimized design to fabricate it,” says first author Subramanian Sundaram PhD ’18, a former graduate student in the Computer Science and Artificial Intelligence Laboratory (CSAIL). “We go from selecting the printing materials, to finding the optimal design, to fabricating the final product in almost a completely automated way.”
The shifting images demonstrates what the system can do. But actuators optimized for appearance and function could also be used for biomimicry in robotics. For instance, other researchers are designing underwater robotic skins with actuator arrays meant to mimic denticles on shark skin. Denticles collectively deform to decrease drag for faster, quieter swimming. “You can imagine underwater robots having whole arrays of actuators coating the surface of their skins, which can be optimized for drag and turning efficiently, and so on,” Sundaram says.
Joining Sundaram on the paper are: Melina Skouras, a former MIT postdoc; David S. Kim, a former researcher in the Computational Fabrication Group; Louise van den Heuvel ’14, SM ’16; and Wojciech Matusik, an MIT associate professor in electrical engineering and computer science and head of the Computational Fabrication Group.
Navigating the “combinatorial explosion”
Robotic actuators today are becoming increasingly complex. Depending on the application, they must be optimized for weight, efficiency, appearance, flexibility, power consumption, and various other functions and performance metrics. Generally, experts manually calculate all those parameters to find an optimal design.
Adding to that complexity, new 3-D-printing techniques can now use multiple materials to create one product. That means the design’s dimensionality becomes incredibly high. “What you’re left with is what’s called a ‘combinatorial explosion,’ where you essentially have so many combinations of materials and properties that you don’t have a chance to evaluate every combination to create an optimal structure,” Sundaram says.
In their work, the researchers first customized three polymer materials with specific properties they needed to build their actuators: color, magnetization, and rigidity. In the end, they produced a near-transparent rigid material, an opaque flexible material used as a hinge, and a brown nanoparticle material that responds to a magnetic signal. They plugged all that characterization data into a property library.
The system takes as input grayscale image examples — such as the flat actuator that displays the Van Gogh portrait but tilts at an exact angle to show “The Scream.” It basically executes a complex form of trial and error that’s somewhat like rearranging a Rubik’s Cube, but in this case around 5.5 million voxels are iteratively reconfigured to match an image and meet a measured angle.
Initially, the system draws from the property library to randomly assign different materials to different voxels. Then, it runs a simulation to see if that arrangement portrays the two target images, straight on and at an angle. If not, it gets an error signal. That signal lets it know which voxels are on the mark and which should be changed. Adding, removing, and shifting around brown magnetic voxels, for instance, will change the actuator’s angle when a magnetic field is applied. But, the system also has to consider how aligning those brown voxels will affect the image.
Voxel by voxel
To compute the actuator’s appearances at each iteration, the researchers adopted a computer graphics technique called “ray-tracing,” which simulates the path of light interacting with objects. Simulated light beams shoot through the actuator at each column of voxels. Actuators can be fabricated with more than 100 voxel layers. Columns can contain more than 100 voxels, with different sequences of the materials that radiate a different shade of gray when flat or at an angle.
When the actuator is flat, for instance, the light beam may shine down on a column containing many brown voxels, producing a dark tone. But when the actuator tilts, the beam will shine on misaligned voxels. Brown voxels may shift away from the beam, while more clear voxels may shift into the beam, producing a lighter tone. The system uses that technique to align dark and light voxel columns where they need to be in the flat and angled image. After 100 million or more iterations, and anywhere from a few to dozens of hours, the system will find an arrangement that fits the target images.
“We’re comparing what that [voxel column] looks like when it’s flat or when it’s titled, to match the target images,” Sundaram says. “If not, you can swap, say, a clear voxel with a brown one. If that’s an improvement, we keep this new suggestion and make other changes over and over again.”
To fabricate the actuators, the researchers built a custom 3-D printer that uses a technique called “drop-on-demand.” Tubs of the three materials are connected to print heads with hundreds of nozzles that can be individually controlled. The printer fires a 30-micron-sized droplet of the designated material into its respective voxel location. Once the droplet lands on the substrate, it’s solidified. In that way, the printer builds an object, layer by layer.
The work could be used as a stepping stone for designing larger structures, such as airplane wings, Sundaram says. Researchers, for instance, have similarly started breaking down airplane wings into smaller voxel-like blocks to optimize their designs for weight and lift, and other metrics. “We’re not yet able to print wings or anything on that scale, or with those materials. But I think this is a first step toward that goal,” Sundaram says.
Professor Patrick Winston, former director of MIT’s Artificial Intelligence Laboratory, dies at 76
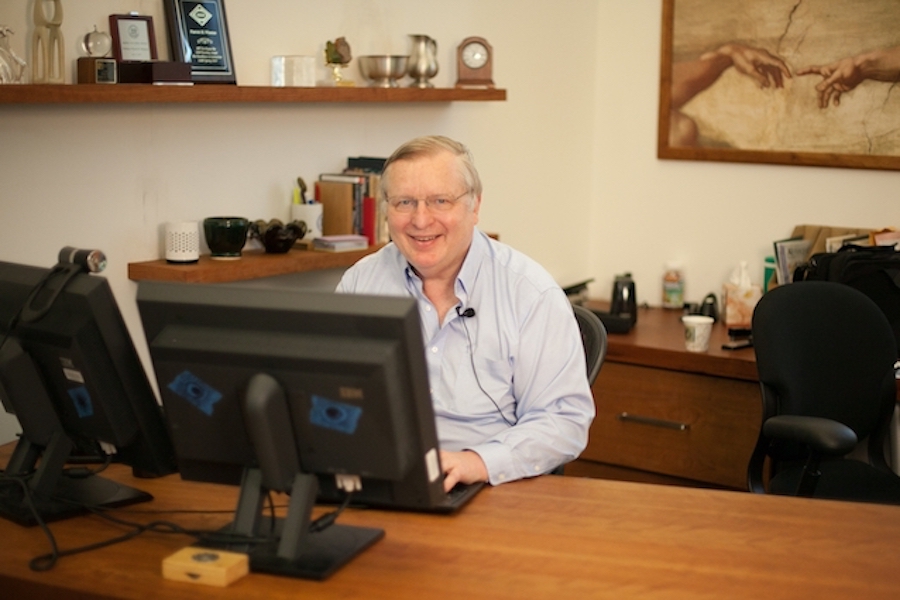
Photo: Jason Dorfman/MIT CSAIL
By Adam Conner-Simons and Rachel Gordon
Patrick Winston, a beloved professor and computer scientist at MIT, died on July 19 at Massachusetts General Hospital in Boston. He was 76.
A professor at MIT for almost 50 years, Winston was director of MIT’s Artificial Intelligence Laboratory from 1972 to 1997 before it merged with the Laboratory for Computer Science to become MIT’s Computer Science and Artificial Intelligence Laboratory (CSAIL).
A devoted teacher and cherished colleague, Winston led CSAIL’s Genesis Group, which focused on developing AI systems that have human-like intelligence, including the ability to tell, perceive, and comprehend stories. He believed that such work could help illuminate aspects of human intelligence that scientists don’t yet understand.
“My principal interest is in figuring out what’s going on inside our heads, and I’m convinced that one of the defining features of human intelligence is that we can understand stories,’” said Winston, the Ford Professor of Artificial Intelligence and Computer Science, in a 2011 interview for CSAIL. “Believing as I do that stories are important, it was natural for me to try to build systems that understand stories, and that shed light on what the story-understanding process is all about.”
He was renowned for his accessible and informative lectures, and gave a hugely popular talk every year during the Independent Activities Period called “How to Speak.”
“As a speaker he always had his audience in the palm of his hand,” says MIT Professor Peter Szolovits. “He put a tremendous amount of work into his lectures, and yet managed to make them feel loose and spontaneous. He wasn’t flashy, but he was compelling and direct. ”
Winston’s dedication to teaching earned him many accolades over the years, including the Baker Award, the Eta Kappa Nu Teaching Award, and the Graduate Student Council Teaching Award.
“Patrick’s humanity and his commitment to the highest principles made him the soul of EECS,” MIT President L. Rafael Reif wrote in a letter to the MIT community. “I called on him often for advice and feedback, and he always responded with kindness, candor, wisdom and integrity. I will be forever grateful for his counsel, his objectivity, and his tremendous inspiration and dedication to our students.”
Teaching computers to think
Born Feb. 5, 1943 in Peoria, Illinois, Winston was always exceptionally curious about science, technology and how to use such tools to explore what it means to be human. He was an MIT-lifer starting in 1961, earning his bachelor’s, master’s and doctoral degrees from the Institute before joining the faculty of the Department of Electrical Engineering and Computer Science in 1970.
His thesis work with Marvin Minsky centered on the difficulty of learning, setting off a trajectory of work where he put a playful, yet laser-sharp focus on fine-tuning AI systems to better understand stories.
His Genesis project aimed to faithfully model computers after human intelligence in order to fully grasp the inner workings of our own motivations, rationality, and perception. Using MIT research scientist Boris Katz’s START natural language processing system and a vision system developed by former MIT PhD student Sajit Rao, Genesis can digest short, simple chunks of text, then spit out reports about how it interpreted connections between events.
While the system has processed many works, Winston chose “Macbeth” as a primary text because the tragedy offers an opportunity to take big human themes, such as greed and revenge, and map out their components.
“[Shakespeare] was pretty good at his portrayal of ‘the human condition,’ as my friends in the humanities would say,” Winston told The Boston Globe. “So there’s all kinds of stuff in there about what’s typical when we humans wander through the world.”
His deep fascination with humanity, human intelligence, and how we communicate information spilled over into what he often described as his favorite academic activity: teaching.
“He was a superb educator who introduced the field to generations of students,” says MIT Professor and longtime colleague Randall Davis. “His lectures had an uncanny ability to move in minutes from the details of an algorithm to the larger issues it illustrated, to yet larger lessons about how to be a scientist and a human being.”
A past president of the Association for the Advancement of Artificial Intelligence (AAAI), Winston also wrote and edited numerous books, including a seminal textbook on AI that’s still used in classrooms around the world. Outside of the lab he also co-founded Ascent Technology, which produces scheduling and workforce management applications for major airports.
He is survived by his wife Karen Prendergast and his daughter Sarah.
A Fossil as a Friend
RobotUnion Selects the 20 Best European Robotics Startups
SME, Stratasys Announce Winners of FANUC-Inspired SkillsUSA Additive Manufacturing Competition
World’s First Composite Concrete 7th Axis used for the First Time In Series Production at Car Manufacturer
Autonomous Mobile Manipulation Robots and e-Commerce Fulfillment
Resource-efficient soft exoskeleton for people with walking impediments
Robots in Depth with Andreas Bihlmaier
In this episode of Robots in Depth, Per Sjöborg speaks with Andreas Bihlmaier about modular robotics and starting a robotics company.
Andreas shares how he started out in computers and later felt that robotics, through its combination of software and hardware that interacts with the world, was what he found most interesting.
Andreas is one of the founders of RoboDev, a company that aims to make automation more available using modular robotics. He explains how modular systems are especially well suited for automating low volume series and how they work with customers to simplify automation.
He also discusses how a system that can easily be assembled into many different robots creates an advantage both in education and in industrial automation, by providing efficiency, flexibility and speed.
We get a personal, behind the scenes account of how the company has evolved as well as insights into the reasoning behind strategic choices made in product development.
Using artificial evolution to design bespoke surgical snakebots
In a world first, Australian Centre for Robotic Vision researchers are pushing the boundaries of evolution to create bespoke, miniaturised surgical robots, uniquely matched to individual patient anatomy.
The cutting-edge research project is the brainchild of Centre PhD researcher Andrew Razjigaev, who impressed HRH The Duke of York with the Centre’s first SnakeBot prototype designed for knee arthroscopy, last November.
Now, the young researcher, backed by the Centre’s world-leading Medical and Healthcare Robotics Group, is taking the next step in surgical SnakeBot’s design.
In place of a single robot, the new plan envisages multiple snake-like robots attached to a RAVEN II surgical robotic research platform, all working together to improve patient outcomes.
The novelty of the project extends to development of an evolutionary computational design algorithm that creates one-of-a-kind, patient-specific SnakeBots in a ‘survival-of-the-fittest’ battle.
Only the most optimal design survives, specifically suited to fit, flexibly manoeuvre and see inside a patient’s knee, doubling as a surgeon’s eyes and tools, with the added bonus of being low-cost (3D printed) and disposable.
Leading the QUT-based Medical and Healthcare Robotics Group, Centre Chief Investigator Jonathan Roberts and Associate Investigator Ross Crawford (who is also an orthopaedic surgeon) said the semi-autonomous surgical system could revolutionise keyhole surgery in ways not before imagined.
Professor Crawford stressed the aim of the robotic system – expected to incorporate surgical dual-arm telemanipulation and autonomous vision-based control – was to assist, not replace surgeons, ultimately improving patient outcomes.
“At the moment surgeons use what are best described as rigid ‘one-size-fits-all’ tools for knee arthroscopy procedures, even though patients and their anatomy can vary significantly,” Professor Crawford said.
He said the surgical system being explored had the potential to vastly surpass capabilities of current state-of-the-art surgical tools.
“The research project aims to design snake-like robots as miniaturised and highly dexterous surgical tools, fitted with computer vision capabilities and the ability to navigate around obstacles in confined spaces such as the anatomy of the human body,” Professor Crawford said.
“Dexterity is incredibly important as the robots are not only required to reach surgical sites but perform complicated surgical procedures via telemanipulation.”
Professor Roberts said the research project was a world-first for surgical robotics targeting knee arthroscopy and would not be possible without the multi-disciplinary expertise of researchers at the Australian Centre for Robotic Vision.
“One of the most exciting things about this project is that it is bringing many ideas from the robotics community together to form a practical solution to a real-world problem,” he said.
“The project has been proceeding at a rapid pace, mainly due to the hard work and brilliance of Andrew, supported by a team of advisors with backgrounds in mechanical engineering, mechatronics, aerospace, medicine, biology, physics and chemistry.”
Due to complete his PhD research project by early 2021, Andrew Razjigaev graduated as a mechatronics engineer at QUT in 2017 and has been a part of the Centre’s Medical and Healthcare Robotics Group since 2016.
The 23-year-old said: “Robotics is all about helping people in some way and what I’m most excited about is that this project may lead to improved health outcomes, fewer complications and faster patient recovery.
“That’s what really drives my research – being able to help people and make a positive difference. Knee arthroscopy is one of most common orthopaedic procedures in the world, with around four million procedures a year, so this project could have a huge impact.”
Andrew said he hoped his work would lead to real-world development of new surgical tools.
“Surgeons want to do the best they can and face a lot of challenges,” he said. “Our objective is to provide surgeons with new tools to be able to perform existing surgery, like knee arthroscopy, more efficiently and safely and to perhaps perform surgery that is simply too difficult to attempt with today’s tools.
“It’s also incredibly cool to use evolution in my work! There’s no question we’re witnessing the age-old process – the only difference being it’s happening inside a computer instead of nature.”
- The process starts with a scan of a patient’s knee. With the supervision of a doctor, the computer classifies the regions for the SnakeBots to reach in the knee (green area) and regions to avoid (red area).
- The resulting geometry makes a 3D environment for the SnakeBots to compete in the simulated evolution. It enables a number of standard SnakeBot designs to be tested and scored on how well they perform – namely how well they manoeuvre to sites inside a patient’s knee. The black lines in the test show some of the trajectories a SnakeBot took to manoeuvre to those sites.
- The evolutionary computational design algorithm kicks in, continually creating new generations of SnakeBots, re-testing and killing off weaker variants until one survives, uniquely matched to an individual patient’s anatomy. The SnakeBot that can safely reach those targets with more dexterity wins the battle of evolution and claims the optimal design.
- The optimal SnakeBots are generated into 3D models to be 3D printed as low-cost, disposable surgical tools unique to each patient.
- They are now ready to be deployed for surgery! The micro SnakeBots are attached to a larger, table-top robotic platform (like the RAVEN II) that positions them for entry into surgical incision sites.
- It is expected that two SnakeBots are fitted with surgical instruments at their tips to enable a surgeon to perform dual-arm teleoperated surgical procedures.
- A third SnakeBot in the multi-bot system will have a camera installed at its tip. This camera system will be used by a robotic vision system to map a patient’s body cavity so that the robot can be steered towards the areas of interest and away from delicate areas that should be avoided. It will track the two arms and surgical area simultaneously, working as the eyes of the surgeon.
Find out more about the work of the Centre’s Medical and Healthcare Robotics Group in our latest annual report.