Avoiding Unnecessary Downtime in Semiconductor Production
MIT robot disinfects Greater Boston Food Bank
A scheme to enhance how swarm robots search for multiple targets
3PL-Provider Romark Logistics Automates Inventory Counts With Drones
RoboBusiness Direct Presents: The Future of Robotics: Research to Commercialization
AI and Robots Redefining the Construction Industry
#313: Solid State Lidar – the 3D Camera, with Erin Bishop
In this episode, Abate interviews Erin Bishop from Sense Photonics about the technology in their “Solid State” LiDAR sensors that allows them to detect objects more accurately and over a larger field of view than traditional scanning LiDAR. Erin dives into the technical details of Solid State Lidar, discusses the applications and industries of the technology.
Erin Bishop
Erin operates at the intersection of product management, project engineering, customer development, and product-market fit within 3D camera company Sense Photonics. Over the past several years, she has worked to initiate the market appetite for telepresence robots, indoor mobile robots, and robotic picking in warehouses. Erin has made appearances at CES, UX Week, RoboBusiness, HardwareCon, and Mobile Future Forward.
Erin creates partnerships that result in large-scale deployment of the company’s revolutionary solid-state FLASH architecture. Prior to joining Sense, she held product manager, marketing, and research roles with several robotics companies, including Industrial Perception, Inc. – which was acquired by Google in 2013 – Adept Technology, iRobot, and DEKA. Erin earned her Master’s in Mechanical Engineering from UMass Lowell, where she performed extensive research in Human-Robot Interaction.
Links
‘Robotics for Infectious Diseases’ and other resources
In times of crisis, we all want to know where the robots are! And young roboticists just starting their careers, or simply thinking about robotics as a career, ask us ‘How can robotics help?’ and ‘What can I do to help?’. Cluster organizations like Silicon Valley Robotics can serve as connection points between industry and academia, between undergrads and experts, between startups and investors, which is why we rapidly organized a weekly discussion with experts about “COVID-19, robots and us” (video playlist).
During our online series, we heard from roboticists directly helping with all sorts of COVID-19 response, like Gui Cavalcanti of Open Source Medical Supplies and Alder Riley of Helpful Engineering. Both groups are great examples of the incredible power of people working together.
Open Source Medical Supplies (OSMS) was formed in March 2020 to research and disseminate open source plans for medical supplies used to treat and reduce the spread of COVID-19 that could be fabricated locally. Additionally, Open Source Medical Supplies supports, mentors, and guides local communities as they self-organize hospital systems, essential services, professional fabricators, makerspaces, and local governments into resilient, self-supporting supply units.
In its first two months of operation, Open Source Medical Supplies helped organize over 73,000 people in its Facebook group, produced 6 iterations of its Open Source COVID-19 Medical Supply Guide featuring 20 design categories and 90+ curated open source designs, engaged over 200 Local Response groups in 50 countries. OSMS materials are being translated into 40 languages, and OSMS guidance and collaboration platforms have catalyzed volunteers around the world to produce and deliver over 11 million medical supply items to their local communities (as of May 30).
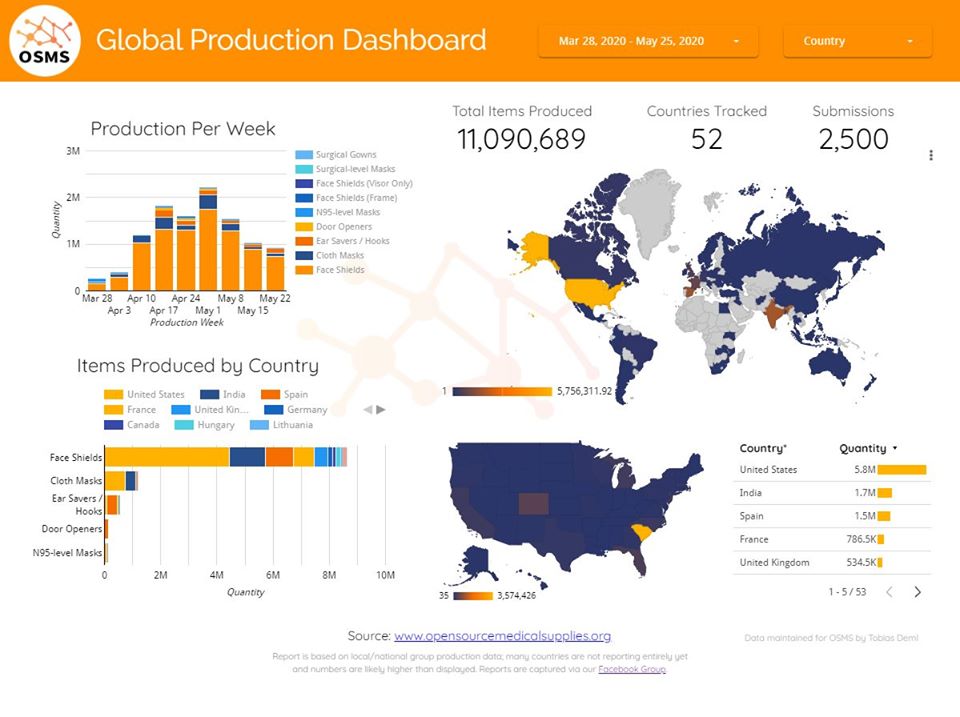
Speakers like Mark Martin from California Community Colleges who started the Bay Area Manufacturers Discussion Forum and Rich Stump from Studio Fathom shared how the manufacturing community was coping with pivoting to making PPE instead of other products, and some of the issues with regulations and the supply chain.
Speakers like Tom Low and Roy Kornbluh from SRI International and Eric Bennett from Frontier Bio talked about redesigning ventilators, including designing robots to teleoperate ventilators. Ventilators are critical in treating COVID-19 but there is also a lack of trained operators, and FDA regulations don’t allow devices to be adapted or changed. And Rachel McCrafty Sadd (aka The Crafty Avenger) from Ace Monster Toys talked about making hundreds thousands of cloth masks and what sort of robots would have been useful.
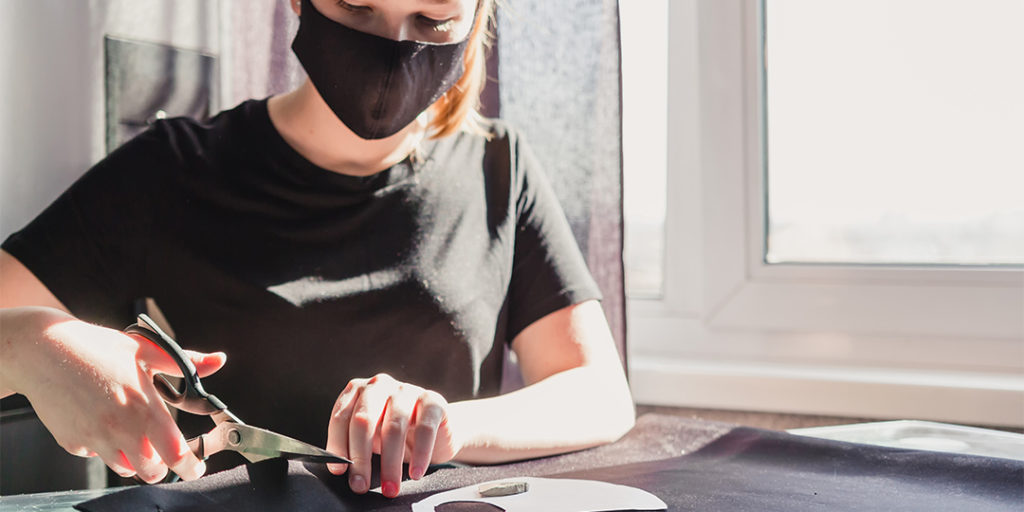
In general, teleoperation is the trojan horse for adopting robots in workplaces in new ways. People trust a robot being remotely operated much more readily than a fully autonomous one. We spoke to Tra Vu from OhmniLabs and David Crawley of Ubiquity Robotics both of whom produce very affordable mobile bases for telepresence and other use cases, including disinfecting. The demand for both robots is rapidly increasing and people are asking for add-on abilities, like the ability to push a button, or open a door. Not all of these tasks are going to be simple to add but clearly once a hospital, facility or household has successfully used one robot, they are very willing to add more robots.
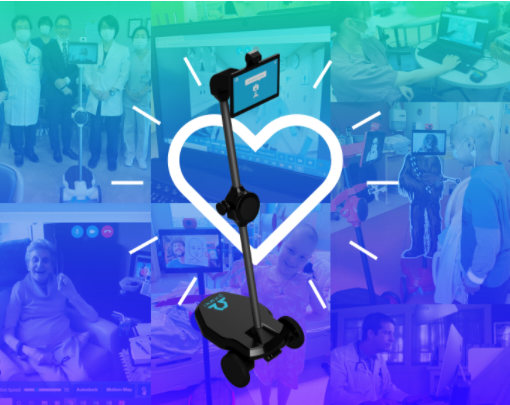
Rescue robotics expert Robin Murphy, who is Raytheon Professor of Computer Science and Engineering at Texas A&M, IEEE Fellow, ACM Fellow and Chair of the new Robotics for Infectious Diseases Organization joined us on several evenings to share a global tally of robot use cases around the world. Not only did the facts get the discussion going but she shared tips and tricks for how to design and deploy robots successfully in pandemic conditions.
Robotics for Infectious Diseases has launched two new interview series: Series 1 provides information from Public Health, Public Safety and Emergency Management Experts that answer many questions about what and how to deploy technology in a disaster response scenario (like COVID-19). The interviews are intended to give roboticists and robotics startups insights into the problems and requirements for technology in the health sector. Series 2 follows roboticists like Antonio Bicchi and Gangtie Zheng working on COVID-19 applications and describes the lessons learned.
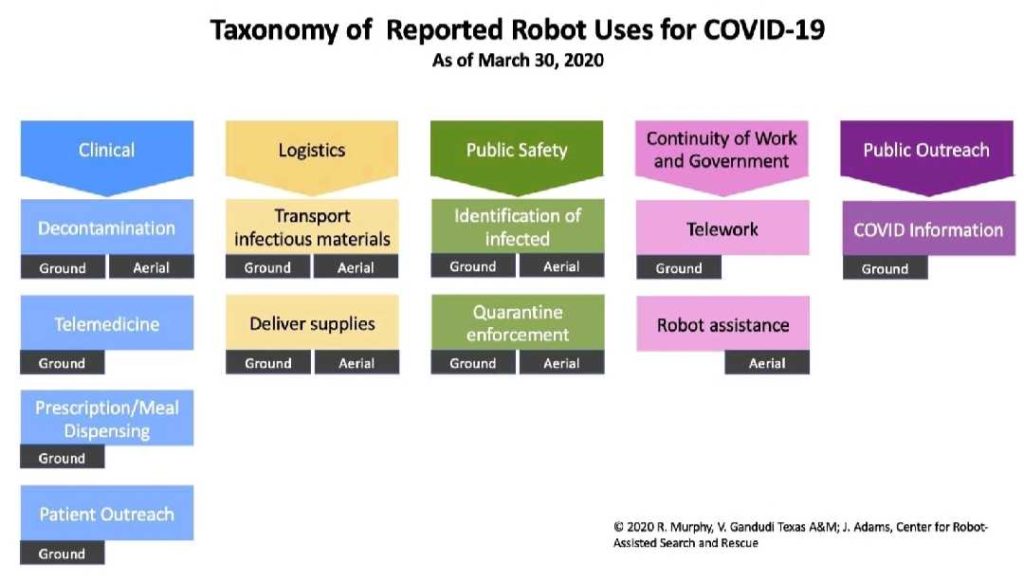
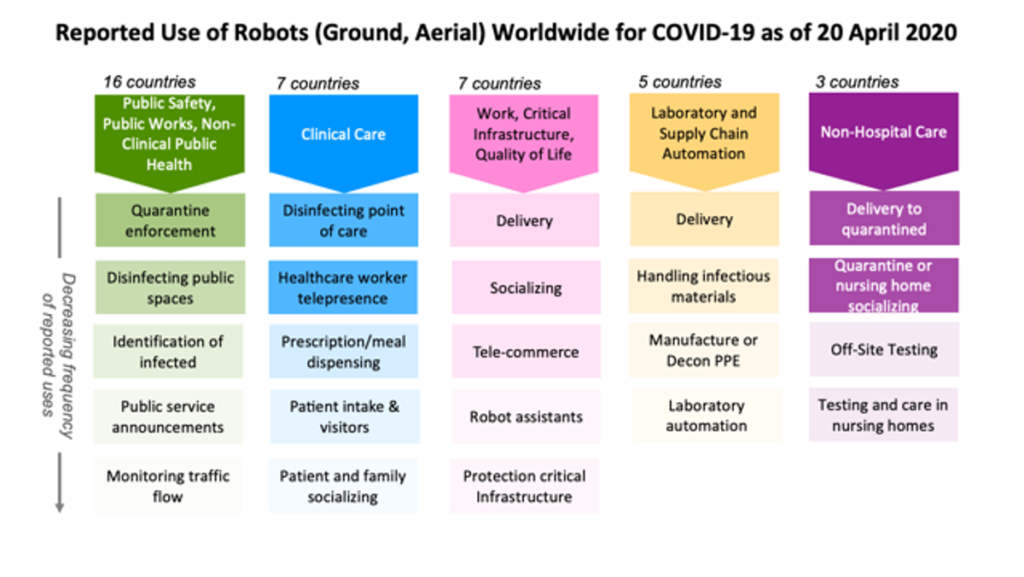
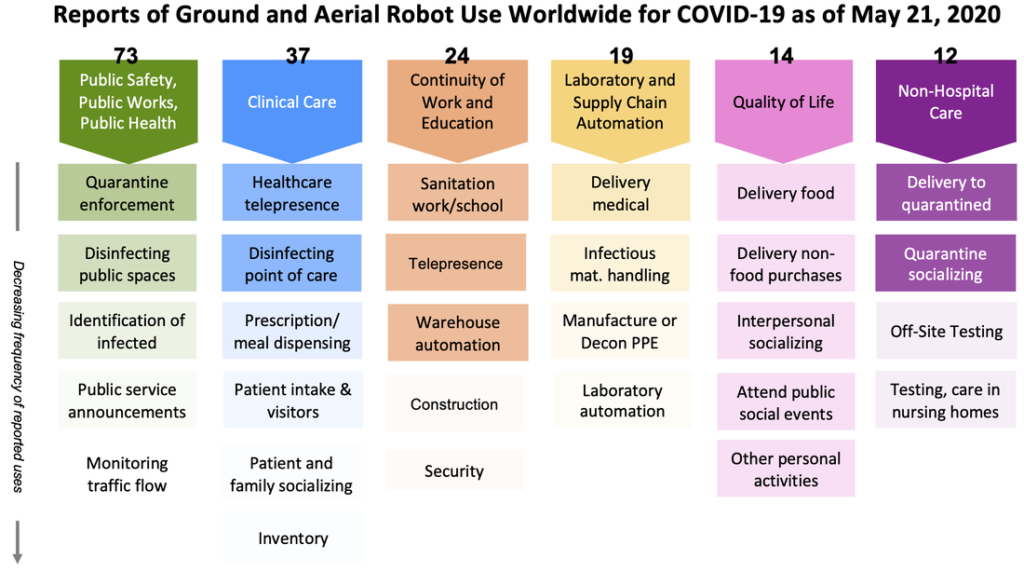
Murphy’s primary research is in artificial intelligence for mobile robots as applied to disaster robotics. She has literally written the book about “Disaster Robotics”. Her analyses have shown that 50% of the terminal failures in disaster robotics are due to human error, so a significant portion of her work is in human-robot interaction. Murphy works with responders and agency stakeholders to determine gaps that lead to the formulation of applied and fundamental research thrusts with her non-profit Center for Robot-Assisted Search and Rescue (CRASAR). Her research group has participated in 27 disasters or incidents and over 35 exercises gathering data spanning urban search and rescue, structural inspection, hurricanes, flooding, mudslides, mine disasters, radiological events, and wilderness search and rescue.
In our COVID-19, robots and us series, we also heard from Sue Keay, the head of Australia’s robotics research cluster and organizer of the Australian Robotics Roadmap about some successful deployments for COVID response, and also disaster scenarios of other sorts, including their 4th place finish in the recent DARPA Subterranean Robotics Challenge.
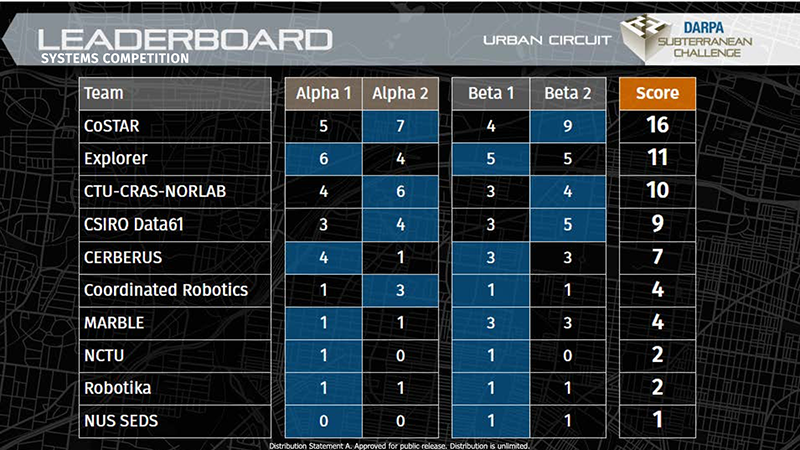
In the Systems competition of the Urban Circuit, 10 teams navigated two courses winding through an unfinished nuclear power plant in Elma, Washington, Feb. 18-27, 2020. DARPA designed the courses to represent complex urban underground infrastructure. The Virtual competition with eight teams took place Jan. 23-30, with results announced Feb. 27. Teams from eleven countries participated across the Virtual and Systems competitions in the Urban Circuit.
“Teams are under tremendous pressure in the SubT Challenge, not just because of the prize money at stake, but because of the significance of winning a DARPA Grand Challenge, events that have a history of jumpstarting innovation,” said Dr. Timothy Chung, program manager for the Subterranean Challenge in DARPA’s Tactical Technology Office. “At the core of the SubT Challenge is the mission to face an unknown environment and respond to changing situations.”
The focus turns now to the Cave Circuit, set for August 2020. DARPA will announce the location about three months prior to the start of the event. DARPA-funded and self-funded teams compete side-by-side throughout the Subterranean Challenge. Only self-funded teams are eligible for prizes in the Circuit Events, but they must finish in the top six overall for the Systems competition and top five overall for the Virtual competition. All qualified teams are eligible for prizes in the Final Event.
“We knew heading into the Urban Circuit that verticality would be one of the significant obstacles. Teams that traveled between floors, either flying, walking, or rolling, found more artifacts,” said Dr. Chung. “Teams designed their approaches to tackle uncertainty up front, and then toward the end of the Urban Circuit, we saw them put their platforms out there and take more risks, I look forward to seeing how they adapt for the Cave Circuit.”
Finally, if you have experiences to share deploying robots for COVID-19 applications, there is a Call for Papers for a Special Issue of IEEE RAM.
This special issue, edited by the IEEE RAS Special Interest Group on Humanitarian Technologies (SIGHT), aims to present up-to-date results and innovative advanced solutions on how robotics and automation technologies are used to fight the outbreak, giving particular emphasis to works involving the actual deployments of robots with meaningful analysis and lessons learned for the robotics community. The editors will accept both conventional full length contributions and short contributions reporting practical solutions to the problem that have proven effective in the field. The topics of interest for paper submissions include, but are not limited to:
- autonomous or teleoperated robots for hospital disinfection and disinfection of public spaces.
- telehealth and physical human-robot interaction systems enabling healthcare workers to remotely diagnose and treat patients.
- hospital and laboratory supply chain robots for handling and transportation of samples and contaminated materials.
- robots use by public safety and public health departments for quarantine enforcement and public service announcements.
- social robots for families interacting with patients or with relatives in nursing homes.
- robots enabling or assisting humans to return to work or companies to continue to function.
- case studies of experimental use of robots in the COVID-19 pandemic.
Important Dates:
May 2020 – Call for papers
31 July 2020 – Submission deadline
15 September 2020 – First decisions on manuscripts
30 October 2020 – Resubmission
30 November 2020 – Final decisions
10 December 2020 – Final manuscripts uploaded
March 2021 – Publication
Click here for more details.
The Increased Role of Robots Post Pandemic
A Lot of Power in a Very Small Space
How to Unit Test Deep Learning: Tests in TensorFlow, mocking and test coverage
Conversation on racism and robotics
Talking about racism and it’s impact on robotics and roboticists was the first conversation in our new biweekly online discussion series “Society, Robots and Us” on alternate Tuesdays at 6pm PDT. It was a generous, honest and painful discussion that I hope has left a lasting impact on everyone who listened. There is systemic racism in America, and this does have an impact on robotics and roboticists in many many ways.
The US Senator Elizabeth Warren in conversation today with Alicia Garza from Black Futures Lab said, “America was founded on principles of liberty and freedom, but it was built on the backs of enslaved people. This is a truth we must not ignore. Racism and white supremacy have shaped every crucial aspect of our economy, and our political system for generations now.”
The speakers in ‘Society, Robots and Us’ were Chad Jenkins, Monroe Kennedy III, Jasmine Lawrence, Tom Williams, Ken Goldberg and Maynard Holliday explored the impact of racism in their experiences in robotics, along with explicit information about changes that we all can make. And we discussed learnings for allies and supporters and what a difference support could make. Please listen to the full discussion but Chad Jenkin’s notes capture some of the critical insights.
“I have been in computing for nearly 30 years and a roboticist for over 20 years. Thus, I have been able to experience firsthand many of the systemic problems that face our field. Let me build on some of the recommendations from the blackincomputing.org open letter and call to action. “
In particular, I believe we can bring equal opportunity to STEM quickly by upholding Title VI of the Civil Rights Act of 1964 and Title IX of the Educational Amendments of 1972 for institutions receiving federal funding, and public funding more generally. We now incentivize systemic disparate impacts in STEM.
Like law enforcement, university faculty are asked to do too much. Given our bandwidth limits, we have to make hard choices about what gets our attention and effort.
This creates a dilemma in every faculty member about whether to bolster their own personal advancement (by gaining social acceptance in the establishments of the field that control access to funding, hiring, and publishing through peer review) or further create and extend opportunity to others (taking a professional sacrifice to provide mentorship and empathy to future generations towards broadening participation in the STEM workforce).
It is clear STEM incentivizes the former given systemic exclusion of underrepresented minorities, with disastrous results thus far.
I believe we are a vastly better society with the upholding of Title VII of the Civil Rights Act of 1964 yesterday by the Supreme Court to prohibit employment discrimination against LGBTQ+ citizens. Discrimination is wrong. My hope is that we can apply this same standard and attention for Title VI of this statue to outcomes in STEM. This is not an issue of altruism, it reflects our true values at a nation and affects the quality of our work and its impact on the world.
There are placeholder measures that can be enacted to incentivize equal opportunity. For example, universities could decline sabbatical and leave requests from faculty seeking to collaborate with companies that have failed to provide equal opportunity, such as OpenAI and Google DeepMind.
To achieve systemic fairness in robotics, however, we must go beyond token gestures to address the causal factors of inequity rooted in the core economic incentives of our universities. It is universities that are the central ladder to opportunity through the development of future leaders, innovators, and contributors to our society.
We have the tools at hand today to create equal opportunity in STEM. The question is whether we have the will.
Equal opportunity cannot be true for anyone unless equal opportunity is true for everyone.
Odeste Chadwicke Jenkins, Associate Professor University of Michigan Robotics Institute
Our next episode of “Society, Robots and Us” on June 30 is going to discuss the role and the roll out of killer robots, but we’ll be coming back to talk more about racism, diversity and inclusion in robotics because we’ve only just scratched the surface.
Conversation on racism and robotics
Talking about racism and it’s impact on robotics and roboticists was the first conversation in our new biweekly online discussion series “Society, Robots and Us” on alternate Tuesdays at 6pm PDT. It was a generous, honest and painful discussion that I hope has left a lasting impact on everyone who listened. There is systemic racism in America, and this does have an impact on robotics and roboticists in many many ways.
The US Senator Elizabeth Warren in conversation today with Alicia Garza from Black Futures Lab said, “America was founded on principles of liberty and freedom, but it was built on the backs of enslaved people. This is a truth we must not ignore. Racism and white supremacy have shaped every crucial aspect of our economy, and our political system for generations now.”
The speakers in ‘Society, Robots and Us’ were Chad Jenkins, Monroe Kennedy III, Jasmine Lawrence, Tom Williams, Ken Goldberg and Maynard Holliday explored the impact of racism in their experiences in robotics, along with explicit information about changes that we all can make. And we discussed learnings for allies and supporters and what a difference support could make. Please listen to the full discussion but Chad Jenkin’s notes capture some of the critical insights.
“I have been in computing for nearly 30 years and a roboticist for over 20 years. Thus, I have been able to experience firsthand many of the systemic problems that face our field. Let me build on some of the recommendations from the blackincomputing.org open letter and call to action. “
In particular, I believe we can bring equal opportunity to STEM quickly by upholding Title VI of the Civil Rights Act of 1964 and Title IX of the Educational Amendments of 1972 for institutions receiving federal funding, and public funding more generally. We now incentivize systemic disparate impacts in STEM.
Like law enforcement, university faculty are asked to do too much. Given our bandwidth limits, we have to make hard choices about what gets our attention and effort.
This creates a dilemma in every faculty member about whether to bolster their own personal advancement (by gaining social acceptance in the establishments of the field that control access to funding, hiring, and publishing through peer review) or further create and extend opportunity to others (taking a professional sacrifice to provide mentorship and empathy to future generations towards broadening participation in the STEM workforce).
It is clear STEM incentivizes the former given systemic exclusion of underrepresented minorities, with disastrous results thus far.
I believe we are a vastly better society with the upholding of Title VII of the Civil Rights Act of 1964 yesterday by the Supreme Court to prohibit employment discrimination against LGBTQ+ citizens. Discrimination is wrong. My hope is that we can apply this same standard and attention for Title VI of this statue to outcomes in STEM. This is not an issue of altruism, it reflects our true values at a nation and affects the quality of our work and its impact on the world.
There are placeholder measures that can be enacted to incentivize equal opportunity. For example, universities could decline sabbatical and leave requests from faculty seeking to collaborate with companies that have failed to provide equal opportunity, such as OpenAI and Google DeepMind.
To achieve systemic fairness in robotics, however, we must go beyond token gestures to address the causal factors of inequity rooted in the core economic incentives of our universities. It is universities that are the central ladder to opportunity through the development of future leaders, innovators, and contributors to our society.
We have the tools at hand today to create equal opportunity in STEM. The question is whether we have the will.
Equal opportunity cannot be true for anyone unless equal opportunity is true for everyone.
Odeste Chadwicke Jenkins, Associate Professor University of Michigan Robotics Institute
Our next episode of “Society, Robots and Us” on June 30 is going to discuss the role and the roll out of killer robots, but we’ll be coming back to talk more about racism, diversity and inclusion in robotics because we’ve only just scratched the surface.
Next-generation cockroach-inspired robot is small but mighty
By Leah Burrows
This itsy-bitsy robot can’t climb up the waterspout yet but it can run, jump, carry heavy payloads and turn on a dime. Dubbed HAMR-JR, this microrobot developed by researchers at the Harvard John A. Paulson School of Engineering and Applied Sciences (SEAS) and Harvard’s Wyss Institute for Biologically Inspired Engineering, is a half-scale version of the cockroach-inspired Harvard Ambulatory Microrobot or HAMR.
About the size of a penny, HAMR-JR can perform almost all of the feats of its larger-scale predecessor, making it one of the most dexterous microrobots to date.
“Most robots at this scale are pretty simple and only demonstrate basic mobility,” said Kaushik Jayaram, Ph.D., a former postdoctoral fellow at SEAS and the Wyss Institute, and first author of the paper. “We have shown that you don’t have to compromise dexterity or control for size.”
Jayaram is currently an Assistant Professor at the University of Colorado, Boulder.
The research was presented virtually at the International Conference on Robotics and Automation (ICRA 2020) this week.
One of the big questions going into this research was whether or not the pop-up manufacturing process used to build previous versions of HAMR and other microbots, including the RoboBee, could be used to build robots at multiple scales — from tiny surgical bots to large-scale industrial robots.
PC-MEMS (short for printed circuit microelectromechanical systems) is a fabrication process in which the robot’s components are etched into a 2D sheet and then popped out in its 3D structure. To build HAMR-JR, the researchers simply shrunk the 2D sheet design of the robot — along with the actuators and onboard circuitry — to recreate a smaller robot with all the same functionalities.
“The wonderful part about this exercise is that we did not have to change anything about the previous design,” said Jayaram. “We proved that this process can be applied to basically any device at a variety of sizes.”

HAMR-JR comes in at 2.25 centimeters in body length and weighs about 0.3 grams — a fraction of the weight of an actual penny. It can run about 14 body lengths per second, making it not only one of the smallest but also one of the fastest microrobots.
Scaling down does change some of the principles governing things like stride length and joint stiffness, so the researchers also developed a model that can predict locomotion metrics like running speeds, foot forces, and payload based on a target size. The model can then be used to design a system with the required specifications.
“This new robot demonstrates that we have a good grasp on the theoretical and practical aspects of scaling down complex robots using our folding-based assembly approach,” said co-author Robert Wood, Ph.D., Charles River Professor of Engineering and Applied Sciences in SEAS and Core Faculty Member of the Wyss Institute.
This research was co-authored by Jennifer Shum, Samantha Castellanos and E. Farrell Helbling, Ph.D. This research was supported by the Defense Advanced Research Projects Agency (DARPA) and the Wyss Institute.