How to lead AI projects
Artificial intelligence is becoming mainstream and many organizations, startups and big corporates alike, are now starting internal AI projects or are incooperating AI into other existing IT. There's just one problem. Leading AI projects are very different from leading in traditional IT.
As a result many AI projects either fail or ignite frustration with project participants, users of the AI and the management involved. They are unaware that they are now in a new paradigm and should have different expectations than usually have to IT. Even implementing off-the-shelf AI components or systems can cause problems that are not usual to the organization.
This is very understandable. A few years ago AI was still something a few big techs and the universities were engaged with but for the masses a distant future. So very few leaders and project managers have experience in the AI-field, that they are suddenly thrown into
So what is so different about AI projects?
The keyword here is uncertainty. The big difference between traditional IT and AI is how much certainty is available. The low certainty is due to the experimental nature of AI. The AI paradigm is experimental in the sense that you can’t predict the road to the finished product. You can't plan the inner workings of AI models before you have created it and you don't know exactly what data you will need or how much data. Lastly you don’t know how well the AI will work. So setting up expectations for the finished solution can be very difficult. In many ways AI development is very much comparable with vaccine development. It's impossible to know in advance if your project will even be successful and most of the insights you need will be acquired while developing. This is very much in contrast the IT paradigm we know. The consensus of traditional IT projects is to precise planning, estimation and achieve a preset list of business objectives as timely and accurate as possible. For that reason we have developed an expertise in for example, planning tools and estimation techniques. But suddenly with the entrance of AI a lot of these skills are no longer useful. In fact they can be downright damaging in an experimental paradigm. If management demands deadlines and accurate estimates the project is bound to fail as it will never be able to deliver.
The first step is admitting
In order to lead in the AI domain you first of all, must acknowledge that this is a new paradigm and you must speak about it openly. When working in a new paradigm the most important tool is to be vocal about the new rules of engagement. With very little certainty in AI, expectation management is already approaching an art. So if you are not even in a dialogue with the project stakeholders about how the new way of working is, expectations will never align. So be very clear about this up front. Even as you’re making the business case you have to be clear that you cannot know either the costs nor revenue generated by an AI project. Not everyone will like it and you will see resistance but it’s much better that you take the conflicts before the project starts.
It's a culture thing
The enabler to get acceptance from stakeholders to work in this kind of uncertainty is the right organizational culture. As a leader it is your responsibility to massage and try to shift the culture in a direction that works with experimental AI projects. If there’s a mismatch between the paradigm you work in and the culture, you will get in trouble in no time.
One of the important features in an experimental culture is the willingness to accept null results exactly like the scientific community does. This is not just the usual preach about accepting failure and mistakes. This is a culture that sees a lot of hard work amounting to no more than the knowledge that a specific solution is not viable.
The experimental culture that fits AI development is very much in line with the learning culture, a cultural style found in the eight distinct culture styles of corporate culture. Other cultural styles such as Results culture and Safety culture can be in sharp contrast to the learning style on crucial points when working with AI. The styles are respectively very keen on achieved results and accurate planning. With AI that offers no certainty for either results or predictability this can quickly conflict.
Be visionary
When leading under uncertainty, leading through a strong vision is very effective. Leading through a strong vision is very much in line with the Purpose culture style. I like to compare it to Columbus' journey to America. Not knowing what to expect on the way or if the journey would even see any kind of success, Columbus still managed to get funding and a reliable crew. Columbus was well known for being extremely strong in his vision and I would attribute at least some of his voyage success to a strong vision. The trick here is to be specific about what you envision on the other side of uncertainty. How will everything look and feel when the project is done?
In conclusion it’s very effective to actively use the culture to support AI development since the alternative might be that it will have that working against you. And organizational culture is definitely one of the stronger forces of the universe.
30 women in robotics you need to know about – 2020
It’s Ada Lovelace Day and once again we’re delighted to introduce you to “30 women in robotics you need to know about”! From 13 year old Avye Couloute to Bala Krishnamurthy who worked alongside the ‘Father of Robotics’ Joseph Engelberger in the 1970s & 1980s, these women showcase a wide range of roles in robotics. We hope these short bios will provide a world of inspiration, in our eighth Women in Robotics list!
In 2020, we showcase women in robotics in China, Japan, Malaysia, Israel, Australia, Canada, United States, United Kingdom, Switzerland, Israel, Norway, Spain, The Netherlands, India and Iran. There are researchers, industry leaders, and artists. Some women are at the start of their careers, while others have literally written the book, the program or the standards.
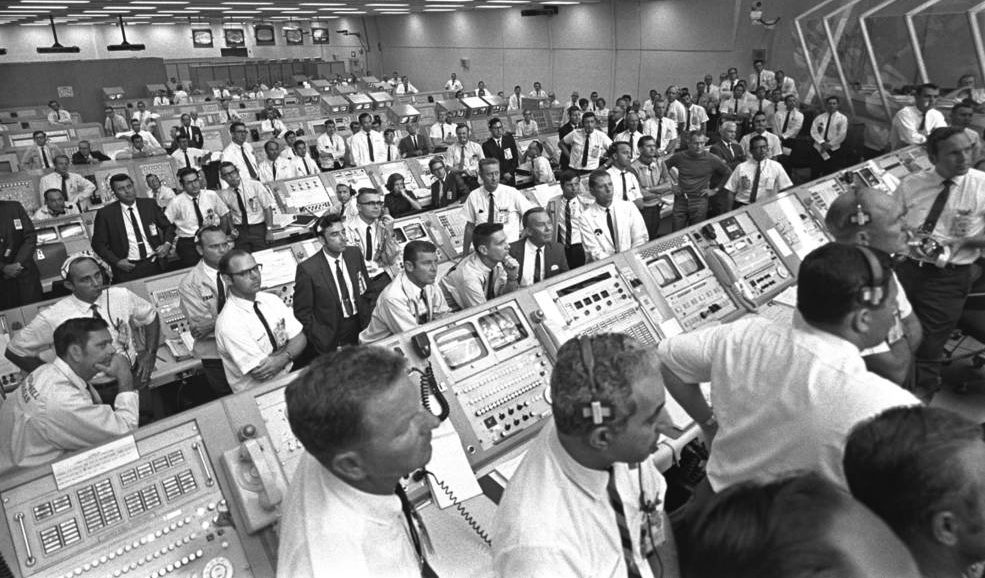
We publish this list because the lack of visibility of women in robotics leads to the unconscious perception that women aren’t making newsworthy contributions. We encourage you to use our lists to help find women for keynotes, panels, interviews etc. Sadly, the daily experience of most women in robotics still looks like this famous NASA control room shot from the 1969 Apollo 11 moon landing, with one solitary woman in the team. It has taken sixty years for the trailblazers like Joann Morgan, Katherine G. Johnson, Dorothy Vaughan, Mary Jackson and Poppy Northcutt to become well known. And finally now we have a woman, Charlie Blackwell-Thompson, serving as Launch Director for the upcoming Artemis Mission, and another, Gwynne Shotwell, serving as President and COO of SpaceX.
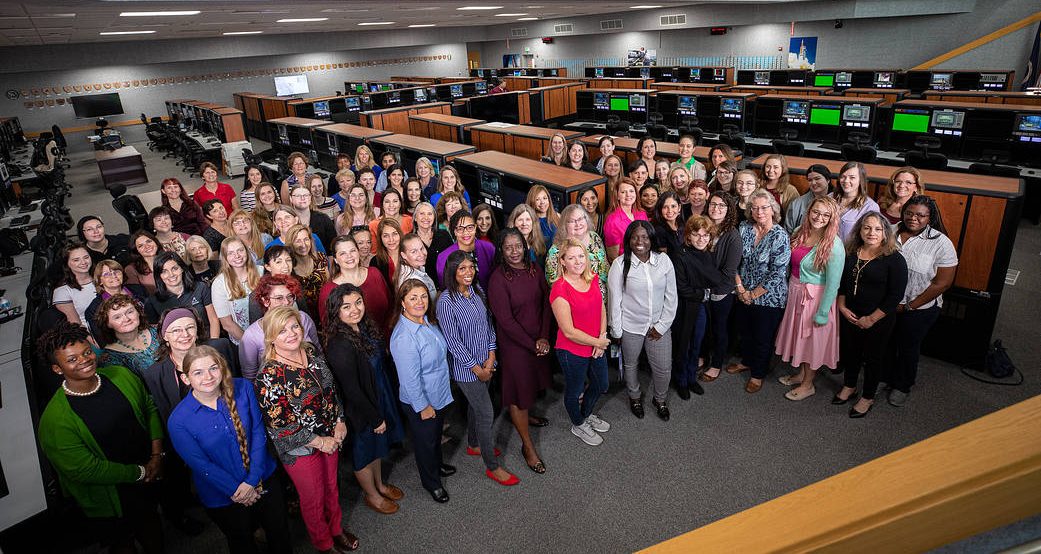
In 2019, women still accounted for less than a quarter (23.6%) of those working in natural and applied sciences and related occupations. In these occupations, women earned, on average, \$0.76 to every \$1.00 earned by men in annual wages, salaries, and commissions in 2018. [ref Catalyst.org ]
This issue is even more pervasive and devastating if you are a person of color. We have always strived to showcase a wide range of origins and ethnicities in our annual list, and this year, as well as four African American roboticists, our list includes the first African American female CEO of a company valued over $1Billion USD. This is just a small step forward, but we’re pleased to announce the recent launch of the Black in Robotics organization, as well as greater recognition of the citation problem.
The citation problem is expected to significantly disadvantage women and people of color due to the historical lack of women followed by the recent growth of large scientific teams, multiplying exclusion. For example, Nature recently published a paper on the impact of NumPy, a significant scientific resource. NumPy was originally developed by many contributors. But the authoritative citation is likely to belong to this description paper, which has 26 authors, all male. [ref Space Australia]
On a positive note, many individuals and organizations intentionally try to reverse this bias. For example, Tulane University just published a guide to help you calculate how much of your reading list includes female authors and a citation guide, similar to the CiteHer campaign from BlackComputeher.org. And as Bram Vanderborght, editor of IEEE Robotics and Automation magazine pointed out in the March 2020 issue, “Scientists are starting to consider how gender biases materialize in physical robots. The danger is that robot makers, consciously or not, may reinforce gender stereotypes and inadvertently create even greater deterrents for young, underrepresented people interested in joining our field.”
We hope you are inspired by these profiles, and if you want to work in robotics too, please join us at Women in Robotics. We are now a 501(c)(3) non-profit organization, but even so, this post wouldn’t be possible if not for the hard work of volunteers; Andra Keay, Hallie Siegel, Sabine Hauert, Sunita Pokharel, Ioannis Erripis, Ron Thalanki and Daniel Carrillo Zapata.
Online events to look out for on Ada Lovelace Day 2020
Tomorrow the world celebrates Ada Lovelace Day to honor the achievements of women in science, technology, engineering and maths. We’ve specially chosen a couple of online events featuring amazing women in robotics and technology. You can enjoy their talks in the comfort of your own home.
Ada Lovelace Day: The Near Future (panel discussion)
Organized by Ada Lovelace Day, this panel session will be joined by Dr Beth Singler (Junior Research Fellow in Artificial Intelligence at the University of Cambridge), Prof Praminda Caleb-Solly (Professor of Assistive Robotics and Intelligent Health Technologies at the University of the West of England), Dr Anat Caspi (director of the Taskar Center for Accessible Technology, University of Washington) and Dr Chanuki Seresinhe (visiting data science researcher at The Alan Turing Institute). The event will take place at 4pm (UTC). You can register here.
Ada Lovelace Day 2020 Celebration of Women in Robotics
Hosted by UC CITRIS CPAR and Silicon Valley Robotics, this event will be joined by Dr Ayanna Howard (Chair of Interactive Computing Georgia Tech), Dr Carlotta Berry (Professor of Electrical and Computer Engineering Rose-Hulman Institute of Technology), Angelique Taylor (PhD Candidate at the Healthcare Robotics Lab in UCSD and Facebook Research Intern), Dr Ariel Anders (First Technical Hire at Robust.ai) and Jasmine Lawrence, (Product Manager at X, the Moonshot Factory). This event will take place at 1am (UTC). You can register here.
Tomorrow we will also publish our 2020 list of women in robotics you need to know about. Stay tuned!
These Robotic Turtles are Nobody’s Prey
Can the voice of healthcare robots influence how they are perceived by humans?
AIR-Act2Act: A dataset for training social robots to interact with the elderly
Toyota Research Institute Showcases Latest Robotics Research Aimed at Amplifying Human Ability in the Home
RoboticsTomorrow – Fall Product Special – Part 2
The Future of Manufacturing: What to Expect
Don’t be data-driven in AI
Being data-driven is usually used and understood with positive connotations but when I hear the word used I get a little anxious about the “data-driven” decisions that might be about to happen. Let me explain why.
According to Wikipedia data-driven means “The adjective data-driven means that progress in an activity is compelled by data, rather than by intuition or by personal experience.” In other words - Look at the data as a primary source of information to act on. When the data gives you a reason to act, you act. It might at a glance seem like a very sound way to work and especially in the AI domain, that in so many ways rely on data. But in fact being data-driven can be very problematic when working with AI. I actually think people that say they are data-driven in general are on the wrong track. This does not mean that I’m against putting much effort into understanding your data. I’m actually a big believer that collecting, understanding and preparing data for AI projects should be the activities with the most resources allocated to it. So I’m pro good data science but against being driven by data and I see that as two very different things.
But then why is it so problematic to be data-driven?
My primary argument is that the driver behind decision making and activities should not be the data you have, but rather curiosity on the problem and the world around it. In a sense that would mean being driven by data you don't have. The final goal of AI projects often is to solve a problem or improve a process and the solutions to that do not always exist in the data you have generated or are being generated by the current world's solutions. So instead you should be curiosity-driven or at least problem-driven. This means that you should not approach problems by looking at your data and making a conclusion. You should look at your data and look for the blindspots and from there be curious. What is it that you don’t know? I’ll get back to curiosity later. First I have some more arguments against being data-driven.
You will extremely rarely have all relevant data to a problem. Even after exhausting all potential data sources. So when you make conclusions from the data you have, the conclusion will at least always be a bit off. This doesn’t mean that data is not useful and that the conclusion is not useful, but you will always be at least a bit wrong. As statisticians would say:"all models are wrong but some are useful".
Another problem with being data-driven is that there's a narrative that decisions made on data is better than decisions made on gut feeling. And while that might be true sometimes, data is not one-sized and can be very helpful at times and very misleading at others.
An example is the father of modern statistics Ronald Fischer that also in hindsight was a little too data-driven. He stubbornly held to his conclusion that data showed that lung cancer was not a result from smoking. The correlation he said must be the other way around and people with lung cancer or higher risk of lung cancer was just more likely to be smokers. He argued that it was either a genetic relation or that cancer patients would use smoking to soothe pain in lungs.So even the best statisticians can be told stories that are far from the truth by data.
The last problem with data is its ability to tell you the story you want it to tell. That can be done consciously or unconsciously. A famous quote by the economist Ronald Case goes “If you torture the data long enough, it will confess to anything” so there no certainty that the conclusion you get from data is correct. The interpretation can be very biased and sometimes we torture the data even without being aware of it ourself.
About curiosity
So as promised I’m getting back to being curious. If I had to choose one keyword to succeed with AI it would be curiosity. AI projects usually start with a process to optimize or a problem to solve and before training a model on data you have to be curious about the problem. In that way the data comes subsequently to the problem and will as a result be more relevant and more specific to the problem.
Curiosity to me means exploring with as little preconception as possible. The best example for me is when children lift up rocks on the ground just to see what is under the rock. If you ever saw a child doing that you will have seen that there is no expectations, only excitement both before and after the rock and been lifted. And that is exactly what curiosity does to the practitioner. It leads to excitement that in turn leads to passion. Passion makes everything much easier and even the tedious parts of a project will feel effortless.
AI is also explorative in its nature and that’s why it suits so well to be curious. If there's specific expectations in an explorative process then disappointed is almost given.
As a result you must let curiosity be the primary driver behind the decisions and activities you make. Being data-driven is reactive in nature and if you want to be innovative in solving problems you must be proactive. Being proactive requires you to be curious about your blind and be driven by the unknown.
#320: Planetary Drilling, with Kris Zacny
In this episode, Lilly Clark interviews Kris Zacny, the Vice President of Exploration Technologies at Honeybee Robotics. They discuss two focuses of development at Honeybee: technology for finding extinct extraterrestrial life and technology for in-situ resource utilization. Zacny speaks about the design and operation of current flight projects, and why drilling technology is key to these missions.
Kris Zacny
Dr. Kris Zacny is VP and Senior Research Scientist at Honeybee Robotics. His interests include space mining, sample handling, soil and rock mechanics, extraterrestrial drilling, and In Situ Resource Utilization (ISRU). He co-founded Exploration Technology Division (EX), based in Altadena, CA. EX, with over 100 employees, focuses on developing space mining robots.
In his previous capacity as an engineer in South African underground gold, diamond, and coal mines, Dr. Zacny managed numerous mining projects and production divisions. This hands-on experience related to drilling and mining became invaluable in developing such technologies for space. Dr. Zacny received his PhD (UC Berkeley, 2005) in Geotechnical Engineering with an emphasis on Extraterrestrial Drilling and Mining, ME (UC Berkeley, 2001) in Petroleum Engineering with emphasis on Drilling and Materials Science, and BSc cum laude (U. Cape Town, 1997) in Mechanical Engineering. He participated in several Antarctic, Arctic, Atacama, and Greenland expeditions. Dr Zacny has approx. 200 publications, he has been a PI/Co-I on approx. 50 projects, he has over 40 NASA New Technology Records and four NASA Group Achievement Awards.
Links