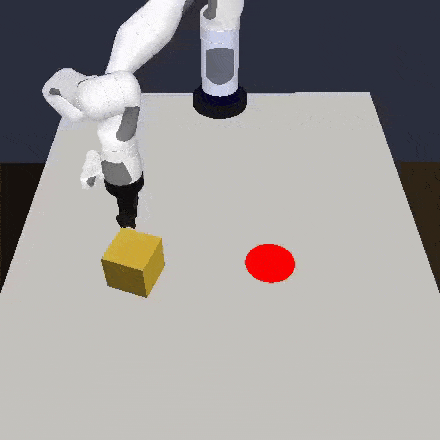
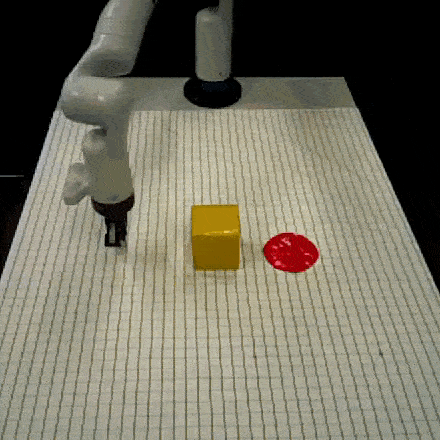
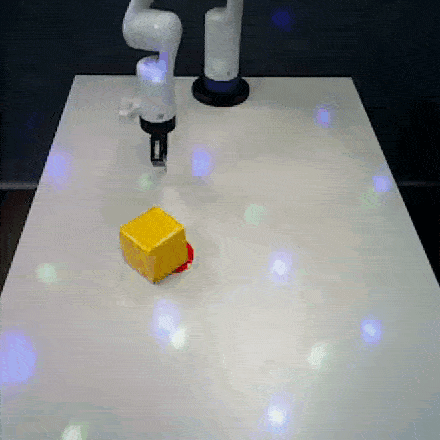
Our method learns a task in a fixed, simulated environment and quickly adapts
to new environments (e.g. the real world) solely from online interaction during
deployment.
The ability for humans to generalize their knowledge and experiences to new situations is remarkable, yet poorly understood. For example, imagine a human driver that has only ever driven around their city in clear weather. Even though they never encountered true diversity in driving conditions, they have acquired the fundamental skill of driving, and can adapt reasonably fast to driving in neighboring cities, in rainy or windy weather, or even driving a different car, without much practice nor additional driver’s lessons. While humans excel at adaptation, building intelligent systems with common-sense knowledge and the ability to quickly adapt to new situations is a long-standing problem in artificial intelligence.
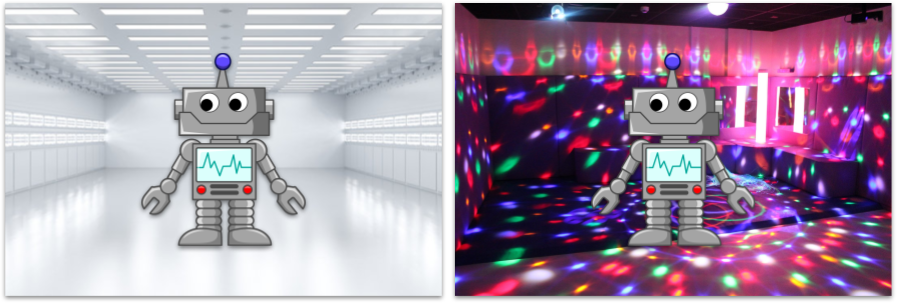
A robot trained to perform a given task in a lab environment may not generalize
to other environments, e.g. an environment with moving disco lights, even
though the task itself remains the same.
In recent years, learning both perception and behavioral policies in an end-to-end framework by deep Reinforcement Learning (RL) has been widely successful, and has achieved impressive results such as superhuman performance on Atari games played directly from screen pixels. Although impressive, it has become commonly understood that such policies fail to generalize to even subtle changes in the environment – changes that humans are easily able to adapt to. For this reason, RL has shown limited success beyond the game or environment in which it was originally trained, which presents a significant challenge in deployment of policies trained by RL in our diverse and unstructured real world.
Generalization by Randomization
In applications of RL, practitioners have sought to improve the generalization ability of policies by introducing randomization into the training environment (e.g. a simulation), also known as domain randomization. By randomizing elements of the training environment that are also expected to vary at test-time, it is possible to learn policies that are invariant to certain factors of variation. For autonomous driving, we may for example want our policy to be robust to changes in lighting, weather, and road conditions, as well as car models, nearby buildings, different city layouts, and so forth. While the randomization quickly evolves into an elaborate engineering challenge as more and more factors of variation are considered, the learning problem itself also becomes harder, greatly decreasing the sample efficiency of learning algorithms. It is therefore natural to ask: rather than learning a policy robust to all conceivable environmental changes, can we instead adapt a pre-trained policy to the new environment through interaction?
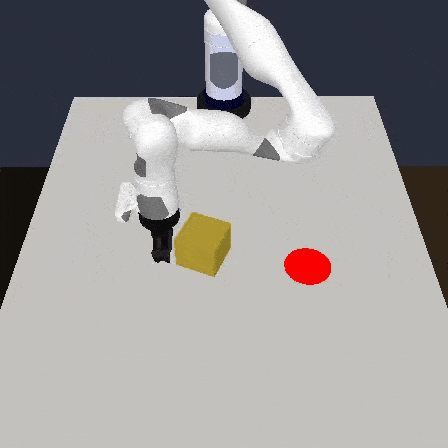
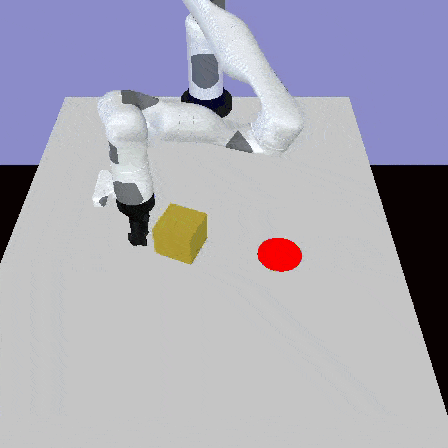
Left: training in a fixed environment. Right: training with
domain randomization.
Policy Adaptation
A naïve way to adapt a policy to new environments is by fine-tuning parameters using a reward signal. In real-world deployments, however, obtaining a reward signal often requires human feedback or careful engineering, neither of which are scalable solutions.
In recent work from our lab, we show that it is possible to adapt a pre-trained policy to unseen environments, without any reward signal or human supervision. A key insight is that, in the context of many deployments of RL, the fundamental goal of the task remains the same, even though there may be a mismatch in both visuals and underlying dynamics compared to the training environment, e.g. a simulation. When training a policy in simulation and deploying it in the real world (sim2real), there are often differences in dynamics due to imperfections in the simulation, and visual inputs captured by a camera are likely to differ from renderings of the simulation. Hence, the source of these errors often lie in an imperfect world understanding rather than misspecification of the task itself, and an agent’s interactions with a new environment can therefore provide us with valuable information about the disparity between its world understanding and reality.
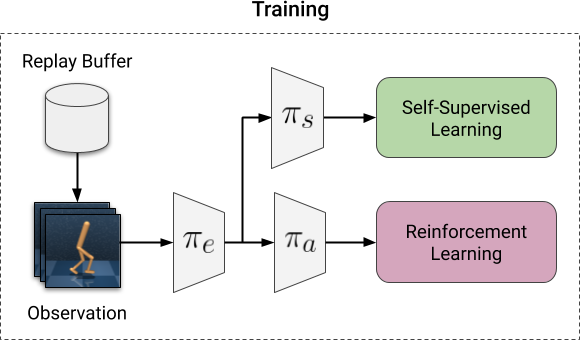
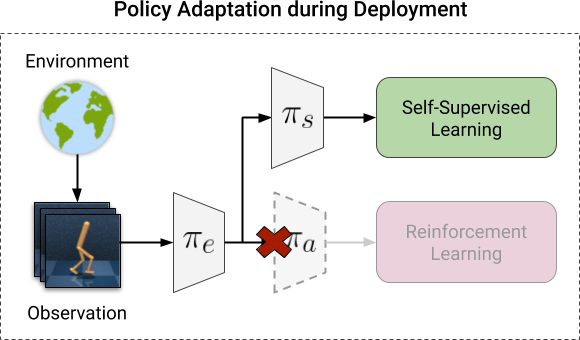
Illustration of our framework for adaptation. Left: training before
deployment. The RL objective is optimized together with a self-supervised
objective. Right: adaptation during deployment. We optimize only the
self-supervised objective, using observations collected through interaction
with the environment.
To take advantage of this information we turn to the literature of self-supervised learning. We propose PAD, a general framework for adaptation of policies during deployment, by using self-supervision as a proxy for the absent reward signal. A given policy network $\pi$ parameterized by a collection of parameters $\theta$ is split sequentially into an encoder $\pi_{e}$ and a policy head $\pi_{a}$ such that $a_{t} = \pi(s_{t}; \theta) = \pi_{a} (\pi_{e}(s_{t}; \theta_{e}) ;\theta_{a})$ for a state $s_{t}$ and action $a_{t}$ at time $t$. We then let $\pi_{s}$ be a self-supervised task head and similarly let $\pi_{s}$ share the encoder $\pi_{e}$ with the policy head. During training, we optimize a self-supervised objective jointly together with the RL task, where the two tasks share part of a neural network. During deployment, we can no longer assume access to a reward signal and are unable to optimize the RL objective. However, we can still continue to optimize the self-supervised objective using observations collected through interaction with the new environment. At every step in the new environment, we update the policy through self-supervision, using only the most recently collected observation:
$$s_t \sim p(s_t | a_{t-1}, s_{t-1}) \\
\theta_{e}(t) = \theta_{e}(t-1) – \nabla_{\theta_{e}}L(s_{t}; \theta_{s}(t-1), \theta_{e}(t-1))$$
where L is a self-supervised objective. Assuming that gradients of the self-supervised objective are sufficiently correlated with those of the RL objective, any adaptation in the self-supervised task may also influence and correct errors in the perception and decision-making of the policy.
In practice, we use an inverse dynamics model $a_{t} = \pi_{s}( \pi_e(s_{t}), \pi_e(s_{t+1}))$, predicting the action taken in between two consecutive observations. Because an inverse dynamics model connects observations directly to actions, the policy can be adjusted for disparities both in visuals and dynamics (e.g. lighting conditions or friction) between training and test environments, solely through interaction with the new environment.
Adapting policies to the real world
We demonstrate the effectiveness of self-supervised policy adaptation (PAD) by training policies for robotic manipulation tasks in simulation and adapting them to the real world during deployment on a physical robot, taking observations directly from an uncalibrated camera. We evaluate generalization to a real robot environment that resembles the simulation, as well as two more challenging settings: a table cloth with increased friction, and continuously moving disco lights. In the demonstration below, we consider a Soft Actor-Critic (SAC) agent trained with an Inverse Dynamics Model (IDM), with and without the PAD adaptation mechanism.
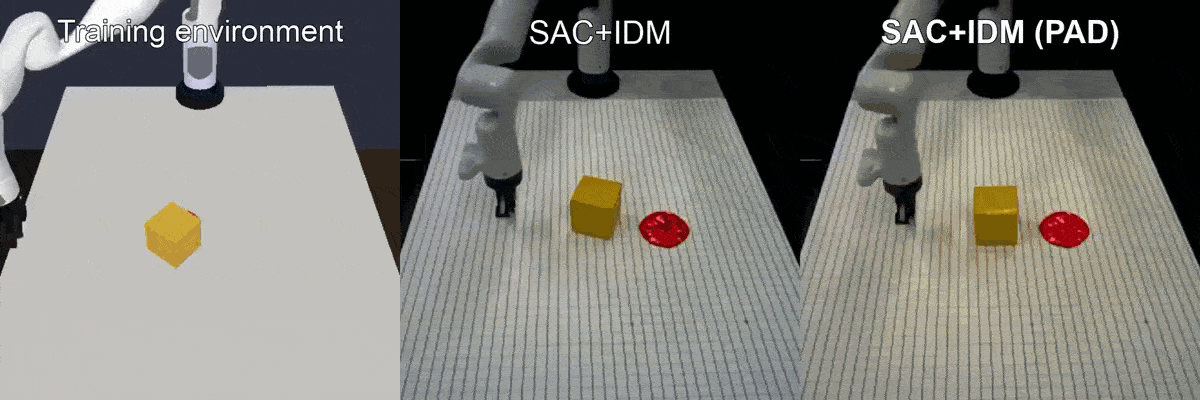
Transferring a policy from simulation to the real world. SAC+IDM is a
Soft Actor-Critic (SAC) policy trained with an Inverse Dynamics Model (IDM),
and SAC+IDM (PAD) is the same policy but with the addition of policy
adaptation during deployment on the robot.
PAD adapts to changes in both visuals and dynamics, and nearly recovers the original success rate of the simulated environment. Policy adaptation is especially effective when the test environment differs from the training environment in multiple ways, e.g. where both visuals and physical properties such as object dimensionality and friction differ. Because it is often difficult to formally specify the elements that vary between a simulation and the real world, policy adaptation may be a promising alternative to domain randomization techniques in such settings.
Benchmarking generalization
Simulations provide a good platform for more comprehensive evaluation of RL algorithms. Together with PAD, we release DMControl Generalization Benchmark, a new benchmark for generalization in RL based on the DeepMind Control Suite, a popular benchmark for continuous control from images. In the DMControl Generalization Benchmark, agents are trained in a fixed environment and deployed in new environments with e.g. randomized colors or continuously changing video backgrounds. We consider an SAC agent trained with an IDM, with and without adaptation, and compare to CURL, a contrastive method discussed in a previous post. We compare the generalization ability of methods in the visualization below, and generally find that PAD can adapt even in non-stationary environments, a challenging problem setting where non-adaptive methods tend to fail. While CURL is found to generalize no better than the non-adaptive SAC trained with an IDM, agents can still benefit from the training signal that CURL provides during the training phase. Algorithms that learn both during training and deployment, and from multiple training signals, may therefore be preferred.
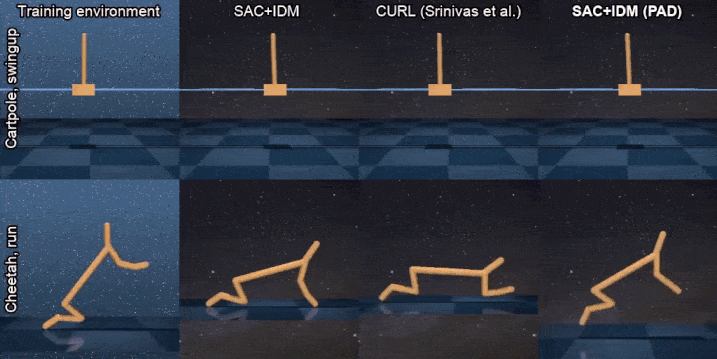
Generalization to an environment with video background. CURL is a
contrastive method, SAC+IDM is a Soft Actor-Critic (SAC) policy trained
with an Inverse Dynamics Model (IDM), and SAC+IDM (PAD) is the same
policy but with the addition of policy adaptation during deployment.
Summary
Previous work addresses the problem of generalization in RL by randomization, which requires anticipation of environmental changes and is known to not scale well. We formulate an alternative problem setting in vision-based RL: can we instead adapt a pre-trained policy to unseen environments, without any rewards or human feedback? We find that adapting policies through a self-supervised objective – solely from interactions in the new environment – is a promising alternative to domain randomization when the target environment is truly unknown. In the future, we ultimately envision agents that continuously learn and adapt to their surroundings, and are capable of learning both from explicit human feedback and through unsupervised interaction with the environment.
This post is based on the following paper:
- Self-Supervised Policy Adaptation during Deployment
Nicklas Hansen, Rishabh Jangir, Yu Sun, Guillem Alenyá, Pieter Abbeel, Alexei A. Efros, Lerrel Pinto, Xiaolong Wang
Ninth International Conference on Learning Representations (ICLR), 2021
arXiv, Project Website, Code