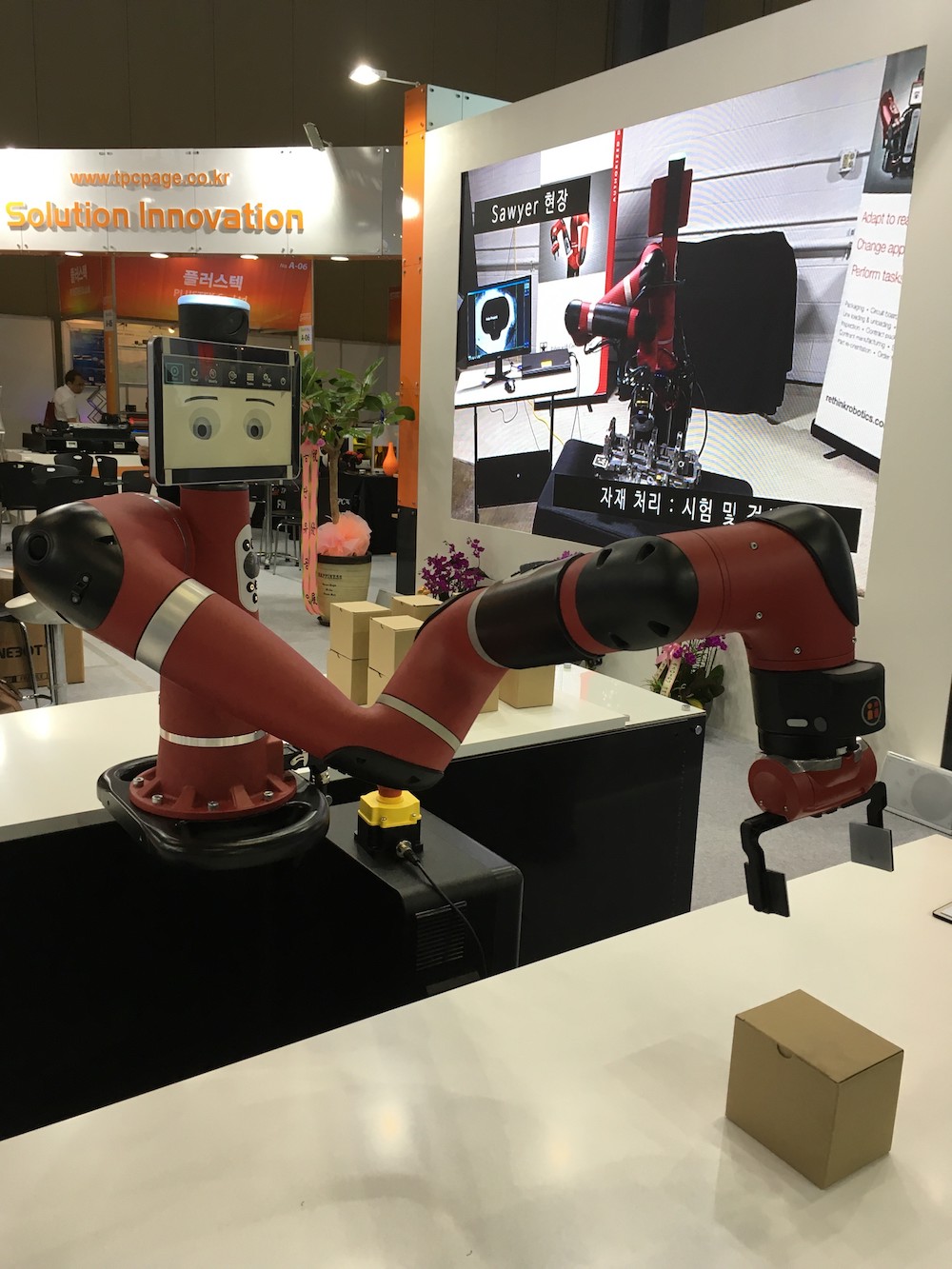
Last week I was talking to one lead engineer of a Singapore company which is building a benchmarking system for robot solutions. Having seen my presentation at ROSCON2016 about robot benchmarking, he asked me how I would benchmark solutions that are non-ROS compatible. I said that I wouldn’t dedicate time to benchmark solutions that are not ROS-based. Instead, I suggested I would use the time to polish the ROS-based benchmarking and suggest that vendors adopt that middleware in their products.
Benchmarks are necessary and they need standards
Benchmarks are necessary to improve any field. By having a benchmark, different solutions to a single problem can be compared and hence a direction for improvement can be traced. Currently, robotics lacks such benchmarking system.
I strongly believe that to create a benchmark for robotics we need a standard at the level of programming.
By having a standard at the level of programming, manufacturers can build their own hardware solutions at will, as long as they are programmable with the programming standard. That is the approach taken by devices that can be plugged into a computer. Manufacturers create the product on their own terms, and then provide a Windows driver that allows any computer in the world (that runs Windows) to communicate with the product. Once this computer-to-product communication is made, you can create programs that compare the same type of devices from different manufacturers for performance, quality, noise, whatever your benchmark is trying to compare.
You see? Different types of devices, different types of hardware. But all of them can be compared through the same benchmarking system that relies on the Windows standard.
Software development for robots also needs standards
The need for standards is not only required for comparing solutions but also to speed robotics development. By having a robotics standard, developers can concentrate on building solutions that do not have to be re-implemented whenever the robot hardware changes. Actually, given the middleware structure, developers can disassociate enough from the hardware that they can almost spend 100% of their time in the software realm, while still developing code for robots.
We need the same type of standard for robotics. We need a kind of operating system that allows us to compare different robotics solutions. We need the Windows of the PCs, the Android of the phones, the CAN of the buses…
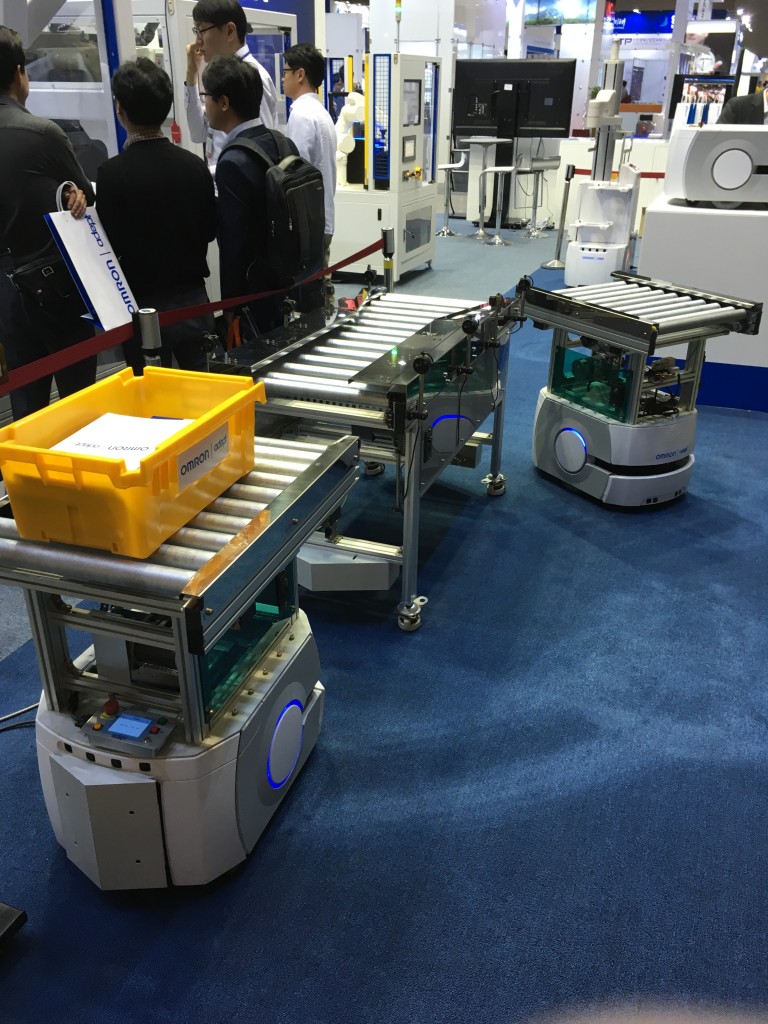
A few standard proposals and a winner
But you already know that. I’m not the first one to state this. Actually, many people have already tried to create such a standard. Some examples include Player, ROS, YARP, OROCOS, Urbi, MIRA or JdE Robot, to name a few.
Personally, I actually don’t care which standard is used. It could be ROS, it could be YARP, or it could be any other that still has not been created. The only thing I really care about is that we adopt a standard as soon as possible.
And it looks like the developers have decided. Robotics developers prefer ROS as their common middleware to program robots.
No other middleware for robotics has had such a large adoption. Some data about it:
Note 1: Only showing the current big three players.
Note 2: Very simple comparison. Difficult to compare in other terms since data is not available.
Note 3: Data measured in August 2017. May vary at the time you are reading this. Links provided on the numbers themselves, so you can check yourself.
This is not only the feeling that we, roboticists, have. The numbers also indicate that ROS is becoming the standard for robotics programming.
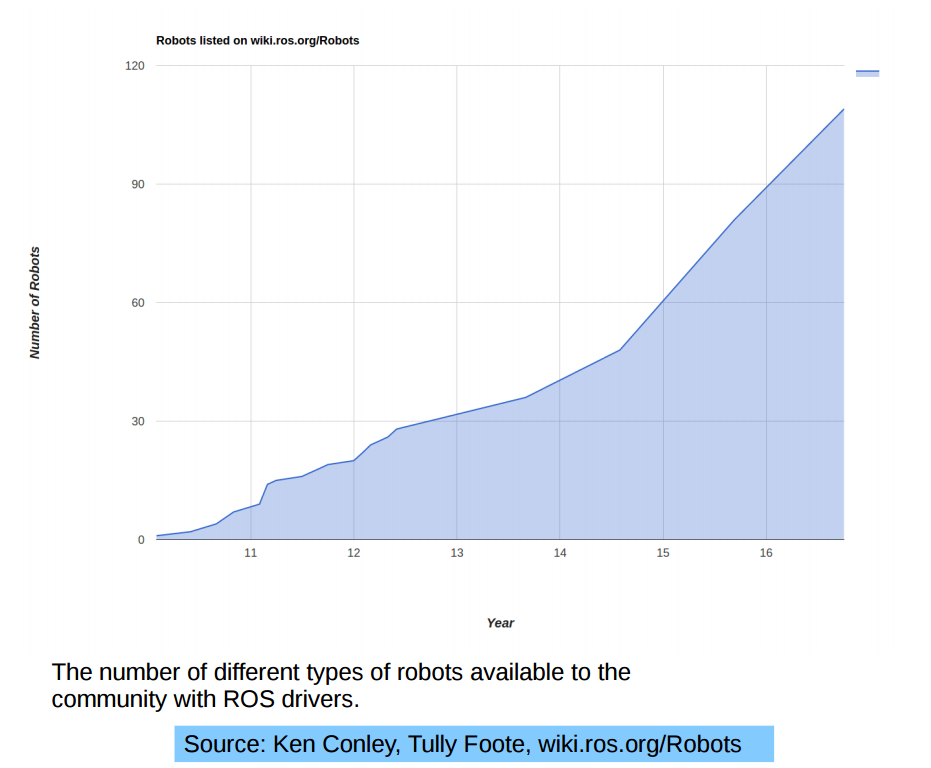
Why ROS?
The question is then, why has ROS emerged on top of all the other possible contestants. None of them is worst than ROS in terms of features. Actually you can find some feature in all the other middlewares that outperform ROS. If that is so, why or how has ROS achieved the status of becoming the standard ?
A simple answer from my point of view: excellent learning tutorials and debugging tools.
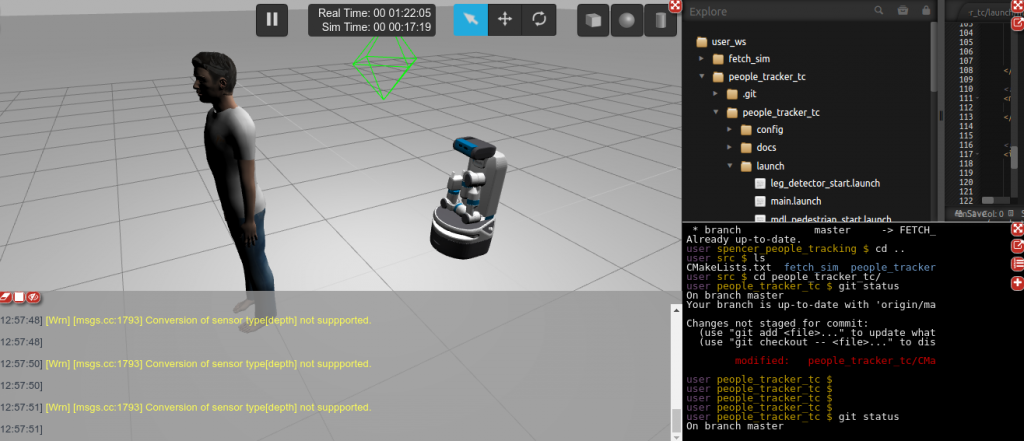
Here there is a video where Leila Takayama, early developer of ROS, explains when she realized that the key for having ROS used worldwide would be to provide tools that simplify the reuse of ROS code. None of the other projects have such a set of clear and structured tutorials. In addition, few of the other middlewares provide debugging tools for their packages. The lack of these two essential aspects is preventing new people from using their middlewares (even if I understand the developers of OROCOS and YARP for not providing it… who wants to write tutorials or build debugging tools… nobody!
)
Additionally, it is not only about tutorials and debugging tools. ROS creators also provide a good system of managing packages. The result is that developers worldwide could use others packages in a (relatively) easy way. This created an explosion in ROS packages available, providing off-the-shelf almost anything for your brand new ROSified robot.
Now, the impressive rate at which contributions to the ROS ecosystem are made makes it almost unstoppable in terms of growing.
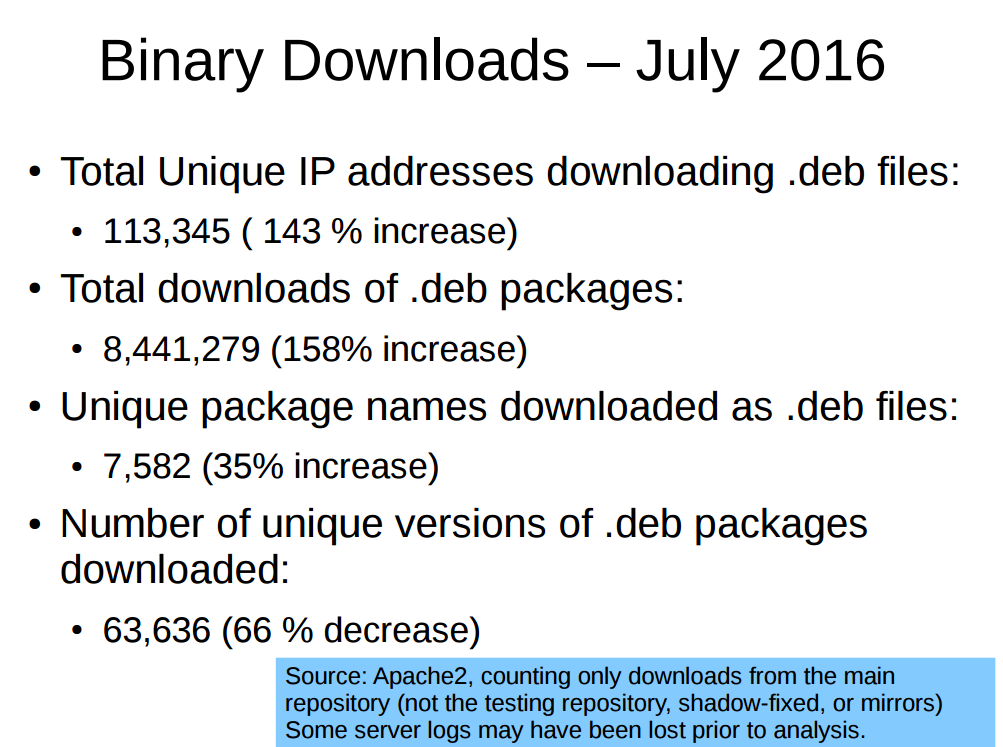
What about companies?
At the beginning, ROS was mostly used by students at Universities. However, as ROS becomes more mature and the number of packages increases, companies are realizing that adopting ROS is also good for them because they will be able to use code developed by others. On top of that, it will be easier for them to hire new engineers who already know the middleware (otherwise they would need to teach the newcomers their own middleware).
As a result, many companies have jumped onto the ROS train, developing from scratch their products to be ROS compatible. Examples include Robotnik, Fetch Robotics, Savioke, Pal Robotics, Yujin Robots, The Construct, Rapyuta Robotics, Erle Robotics, Shadow Robot or Clearpath, to name a few of the sponsors of the next ROSCON
. Creating their ROS-compatible products, they decreased their development time by several orders of magnitude.
To bring things further, two Spanish companies have revolutionised the standardization of robotics products using ROS middleware: on one side, Robotnik has created the ROS Components shop. A shop where anyone can buy ROS compatible devices, starting from mobile bases to sensors or actuators. On the other side, Erle Robotics (now Acutronic Robotics) is in the process of developing Hardware ROS. The H-ROS is a standardized software and hardware infrastructure to easily create reusable and reconfigurable robot hardware parts. ROS is enabling hardware standarization too, but this time driven by companies, not research! That must mean something…

Finally, it looks like industrial robot manufacturers have understood the value that a standard can provide to their business. Even if they do not make their industrial robots ROS-enabled from the start, they are adopting ROS Industrial a flavour of ROS, which allows them to ROSify their industrial robots and re-use all the software created for manipulators in the ROS ecosystem.
But are all companies jumping onto the ROS train? Not all of them!
Some companies like Jibo, Aldebaran or Google still do not rely on ROS for their robot programming. Some of them rely on their own middleware created before the existence of ROS (that is the case of Aldebaran). Some others, though, are creating their own middleware from scratch. Their reasons: they do not believe ROS is good, they have already created a middleware, or do not want to develop their products dependent on the middleware of others. Those companies have very fair reasons to go their way. However, will that make them competitive? (if we have to judge from history, mobiles, VCRs, the answer may be no).
So is ROS the standard for programming robots?
That question is still too soon to be answered. It looks like it is becoming the standard, but many things can change. It is unlikely that another middleware takes the current title from ROS, but it may happen. There could be a new player that wipes ROS from the map (maybe Google will release its middleware to the public – like they did with Android – and take the sector by storm?).
Still, ROS has its problems, like a lack of security or the instability of some important packages. Even if the OSRF group are working hard to build a better ROS system (for instance ROS2 is in beta phase with many root improvements), some hard work is still required for some basic things (like the ROS controllers for real robots).
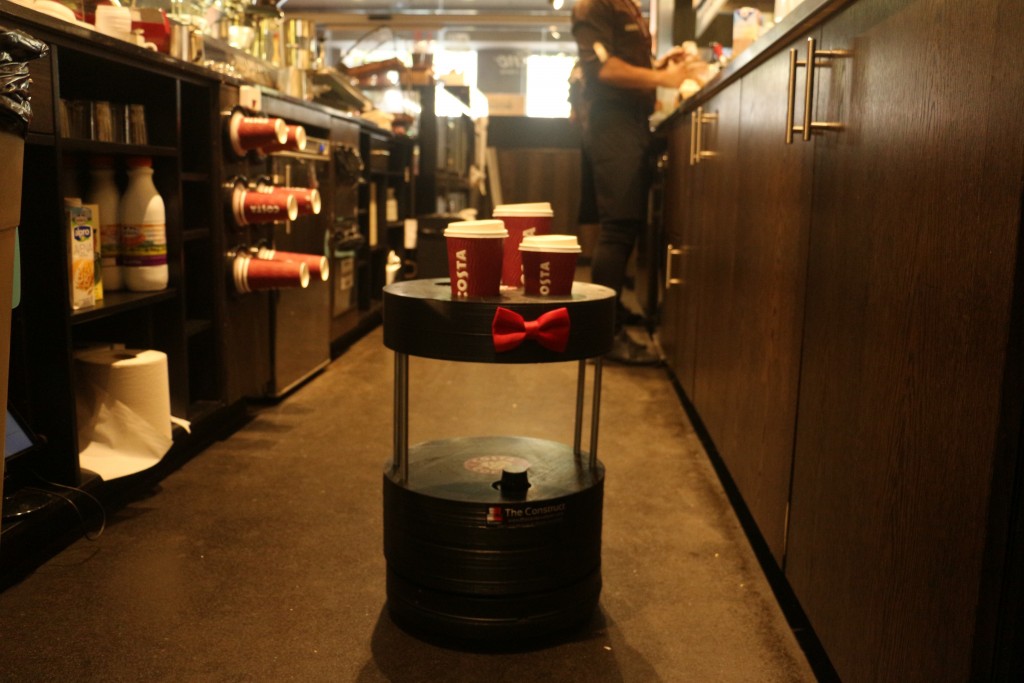
Given those numbers, at The Construct we believe that ROS IS THE STANDARD (that is why we are devoted to creating the best ROS learning tutorials of the world). Actually, it was thanks to this standardization that two Barcelona students were able to create an autonomous robot product for coffee shops in only three months with zero knowledge of robotics (see Barista robot).
This is the future, and it is good. In this future, thanks to standards, almost anyone will be able to build, design and program their own robotics product, similar to how PC stores are building computers today.
So my advice, as I said to the Singapore engineer, is to bet on ROS. Right now, it is the best option for a robotics standard.