Data preprocessing for deep learning: Tips and tricks to optimize your data pipeline using Tensorflow
Advantages of Vacuum driven Soft Grips for Food Production
Ironhand superpower under control
Miniature antenna enables robotic teaming in complex environments
Case Study – Asparagus Picking Agricobot
New electronic skin can react to pain like human skin
When to buy and when to build AI
One of the most important questions when starting to work with and implement AI in your organization is also one of the most complicated to answer: Should you buy off-the-shelf AI products, build your own in-house or have it built custom by consultants?
There’s no one size fits all answer here, but there are some considerations that can help you to understand what is best for you. I’ll try to go through the considerations and let you decide in the end what suits your business the best.
Is AI strategic for your business?
First of all I believe you should ask yourself: Is AI development a strategic feature to my organization? That can be a bit of a vague question so I’ll boil it down to this: Will AI solutions provide you with a competitive advantage that you will try to protect and keep improving to stay a head?
If the AI is just something that is meant to make an improvement that it’s likely your competition can easily copy then you should definitely buy the solution off-the-shelf or have made from experts you hire in. Building up the needed know how and organizational capabilities to make an AI that is only here for a small tactical advantage is not necessary. That will take your focus away from the more important problems. So ask yourself the hard question: If the business would need to do cutbacks, would you keep investing in building your own AI as a strategic priority? If not, you should consider not to do it in the first place.
On the other hand if you believe that one or more AI-solutions can be a competitive advantage that your competition can not easily copy then you should try to build it in-house. In this case you have to be clear on what makes it hard for your competitors to copy. Do you have some access to data that they don’t? Do you have a better position to build the AI capability or something else? Make very sure that you are actually in a position to be competitive here. If not, your competition will copy you by buying from an experienced vendor at a lower cost than you paid to build your own AI.
Research the market
You will be surprised how many off-the-shelf AI solutions there are out there that solve all kinds of problems. People tend to in my experience not do the research and end up making expensive investments that take forever to get done and still it won’t compare to the products already on the market. You really have to have scale to make a business case for building your own solution when there’s already a lot available out there.
I actually once met someone building a solution in-house that was exactly what my AI company was doing. We needed massive scale to get anywhere near a good business case and yet these guys tried to do it themself. We had more than 14.000 business customers at the time and this one business wanted to make the same AI for their business only. They of course had to close their project since it was too big an investment but they still spent a lot of money. Once a project has been kicked off it can be hard to pull back since a lot of ego and prestige can go into corporate projects.
In for a penny in for a pound (of AI)
I have a rule of thumb that never fails me. “When an organization does something it doesn’t do regularly it will execute it poorly”. I made this rule of thumb to explain to myself why very competent organizations sometimes completely flops relatively simple endeavours. I guess the reason is that working in a new domain for an organization is not only not supported by the current processes and culture but might require the organization to work against them. Whatever the reason I see it consistently and I also see it being the case with AI. If you don’t do AI projects regularly you will see massive overhead and probably fail it. So if the frequency of your AI projects are low you should probably look to outsource as much as possible. This is not an attempt to scare anyone away from AI projects, but it takes effort to build the AI capability and that’s a conscious choice you have to make here.
Size matters
AI projects require a minimum investment that is usually larger than traditional IT projects. In AI the skills from engineers, machine learning developers, data scientists and product managers are quite unique. So as a result your organization just has to be a certain size for in-house AI projects to make sense. AI usually also is a trial and error workflow that doesn't promise revenue or profit right away.
There’s no fixed amount of employees or revenue but when the AI team has to be 4-5 people at minimum then you probably shouldn’t do it before you can handle a team of that size for a while not providing any revenue or cost saving for a good while.
Get your data straight
Data is a big part of many AI projects and I always recommend that you get your data straight before you go into the actual AI development. In my mind it’s more important(And more competitive) to get a smooth data operation with low costs and high quality data. I would always prefer to get the data operations in-house and the AI-development is second priority. Getting the data operations right is more of a competitive advantage than building the AI. It’s like supermarket chains competing - The chain with best purchasing of goods and more low cost warehouse operations can provide cheaper consumer prices and are more competitive. Data is the same way. If you can get better data at a better quality and a lower cost, your AI projects will be superior to your competitors even if their AI capabilities are superior to your businesses. So make data the priority if you have to choose.
Building AI is getting easier
One last thing I think you should take into account is that AI projects are getting easier and the barrier to get started is getting lower. AI used to be a very difficult domain to work in, requiring both Phds in data science, machine learning engineers and often thousands of hours of coding to make a useful AI. Today a lot of that can be done at a much lower buy-in with techniques such as Transfer learning and AutoML. It also seems that the bar for getting started is getting lower and lower. As a result building AI in-house is clearly becoming more accessible and with time more business should have a go at it.
That’s it. From here, the decision is yours.
Robot takes contact-free measurements of patients’ vital signs
Year-to-Date Numbers Show Decline in Robotics (18%), Machine Vision (8%), and Motion Control (6%) Orders Compared to 2019
The human touch – Robots for completing tasks that require human senses
Amateur drone videos could aid in natural disaster damage assessment
Simplifying Motion Control through Integration of All System Components
AI and Decision Science – A forced marriage that is largely ignored
You might not be aware or do this unconsciously but, if you work with AI you also work in the decision science space.
Imagine this: You have made an AI model that can take in support tickets and classify them into different subjects and sentiments. With that you can prioritize support tickets by how critical they are and have them directed to the appropriate support team. Sounds great right? But is it really that simple? No. With the AI model in place we are really only halfway to the finish line. If you decided to make an AI like the one I just described you must have had the goal of optimizing the support ticket workflow. Either for happier customers or to lower costs or maybe some other business objective. Either way, the way we choose to act on the data we get as a result of the AI is equally important to the actual AI, if not more. When we take a stand on how to act on the data we get we actually make a decision model. The science that goes into these models are not as simple as it might sound. Look at this example:
The support ticket AI suggests that with 60% likelihood a new ticket is about termination, 30% about a new feature and scores medium critical on the sentiment analysis. Now it doesn’t seem so easy anymore does it? How do we handle this information? Who should get this ticket? And isn’t a termination critical no matter the sentiment score?
I’m in no way a decision scientist and cannot teach anyone much here. But what I can tell you for certain is that the decision models on top of AI are way too often left to be a secondary priority with no conscience or strategic approach. And even worse - The decision model is only discussed after we are finished with the AI models. I would argue that it is in the making of the decision model that we actually get to understand what data we really need, so making the AI first rarely makes sense since we don’t know what we actually need.
There’s also a lot of traps to be aware of in decision making such as survival bias(Thinking you made the right decision because you got the right result) and many of us think we are better decision makers than we really are.
If you want to learn more about decision science my best advice is to follow the Chief Decision Scientist at Google Cassie Kozyrkov. She really succeeds at taking decision science to an understandable level.
So to sum up. If we want to have better results with our AI solutions we should pay more attention to decision making and in many cases start with that before we go modelling.
Cutting surgical robots down to size
By Lindsay Brownell
Minimally invasive laparoscopic surgery, in which a surgeon uses tools and a tiny camera inserted into small incisions to perform operations, has made surgical procedures safer for both patients and doctors over the last half-century. Recently, surgical robots have started to appear in operating rooms to further assist surgeons by allowing them to manipulate multiple tools at once with greater precision, flexibility, and control than is possible with traditional techniques. However, these robotic systems are extremely large, often taking up an entire room, and their tools can be much larger than the delicate tissues and structures on which they operate.
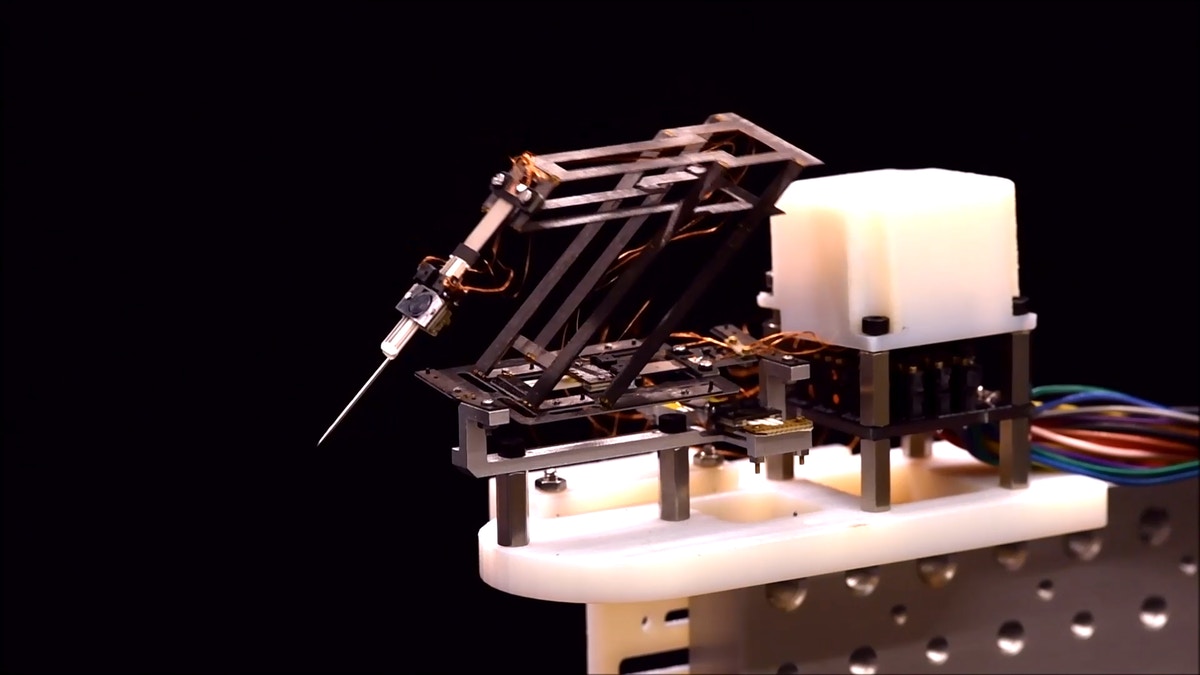
A collaboration between Wyss Associate Faculty member Robert Wood, Ph.D. and Robotics Engineer Hiroyuki Suzuki of Sony Corporation has brought surgical robotics down to the microscale by creating a new, origami-inspired miniature remote center of motion manipulator (the “mini-RCM”). The robot is the size of a tennis ball, weighs about as much as a penny, and successfully performed a difficult mock surgical task, as described in a recent issue of Nature Machine Intelligence.
“The Wood lab’s unique technical capabilities for making micro-robots have led to a number of impressive inventions over the last few years, and I was convinced that it also had the potential to make a breakthrough in the field of medical manipulators as well,” said Suzuki, who began working with Wood on the mini-RCM in 2018 as part of a Harvard-Sony collaboration. “This project has been a great success.”
A mini robot for micro tasks
To create their miniature surgical robot, Suzuki and Wood turned to the Pop-Up MEMS manufacturing technique developed in Wood’s lab, in which materials are deposited on top of each other in layers that are bonded together, then laser-cut in a specific pattern that allows the desired three-dimensional shape to “pop up,” as in a children’s pop-up picture book. This technique greatly simplifies the mass-production of small, complex structures that would otherwise have to be painstakingly constructed by hand.
The team created a parallelogram shape to serve as the main structure of the robot, then fabricated three linear actuators (mini-LAs) to control the robot’s movement: one parallel to the bottom of the parallelogram that raises and lowers it, one perpendicular to the parallelogram that rotates it, and one at the tip of the parallelogram that extends and retracts the tool in use. The result was a robot that is much smaller and lighter than other microsurgical devices previously developed in academia.
The mini-LAs are themselves marvels in miniature, built around a piezoelectric ceramic material that changes shape when an electrical field is applied. The shape change pushes the mini-LA’s “runner unit” along its “rail unit” like a train on train tracks, and that linear motion is harnessed to move the robot. Because piezoelectric materials inherently deform as they change shape, the team also integrated LED-based optical sensors into the mini-LA to detect and correct any deviations from the desired movement, such as those caused by hand tremors.
Steadier than a surgeon’s hands
To mimic the conditions of a teleoperated surgery, the team connected the mini-RCM to a Phantom Omni device, which manipulated the mini-RCM in response to the movements of a user’s hand controlling a pen-like tool. Their first test evaluated a human’s ability to trace a tiny square smaller than the tip of a ballpoint pen, looking through a microscope and either tracing it by hand, or tracing it using the mini-RCM. The mini-RCM tests dramatically improved user accuracy, reducing error by 68% compared to manual operation – an especially important quality given the precision required to repair small and delicate structures in the human body.
After the mini-RCM’s success on the tracing test, the researchers then created a mock version of a surgical procedure called retinal vein cannulation, in which a surgeon must carefully insert a needle through the eye to inject therapeutics into the tiny veins at the back of the eyeball. They fabricated a silicone tube the same size as the retinal vein (about twice the thickness of a human hair), and successfully punctured it with a needle attached to the end of the mini-RCM without causing local damage or disruption.
In addition to its efficacy in performing delicate surgical maneuvers, the mini-RCM’s small size provides another important benefit: it is easy to set up and install and, in the case of a complication or electrical outage, the robot can be easily removed from a patient’s body by hand.
“The Pop-Up MEMS method is proving to be a valuable approach in a number of areas that require small yet sophisticated machines, and it was very satisfying to know that it has the potential to improve the safety and efficiency of surgeries to make them even less invasive for patients,” said Wood, who is also the Charles River Professor of Engineering and Applied Sciences at Harvard’s John A. Paulson School of Engineering and Applied Sciences (SEAS).
The researchers aim to increase the force of the robot’s actuators to cover the maximum forces experienced during an operation, and improve its positioning precision. They are also investigating using a laser with a shorter pulse during the machining process, to improve the mini-LAs’ sensing resolution.
“This unique collaboration between the Wood lab and Sony illustrates the benefits that can arise from combining the real-world focus of industry with the innovative spirit of academia, and we look forward to seeing the impact this work will have on surgical robotics in the near future,” said Wyss Institute Founding Director Don Ingber, M.D., Ph.D., who is also the the Judah Folkman Professor of Vascular Biology at Harvard Medical School and Boston Children’s Hospital, and Professor of Bioengineering at SEAS.