Scientists create the next generation of living robots
Case Study: Grippers from Zimmer Group Automate a Cleaning Machine for Sterile Glass Vials
Roboreptile climbs like a real lizard
Ready for duty: Healthcare robots get good prognosis for next pandemic
Redefining Communications for Today’s Mobile Workforces
Boston Dynamics’ new robot Stretch can help move boxes in warehouses
#331: Multi-Robot Learning, with Amanda Prorok
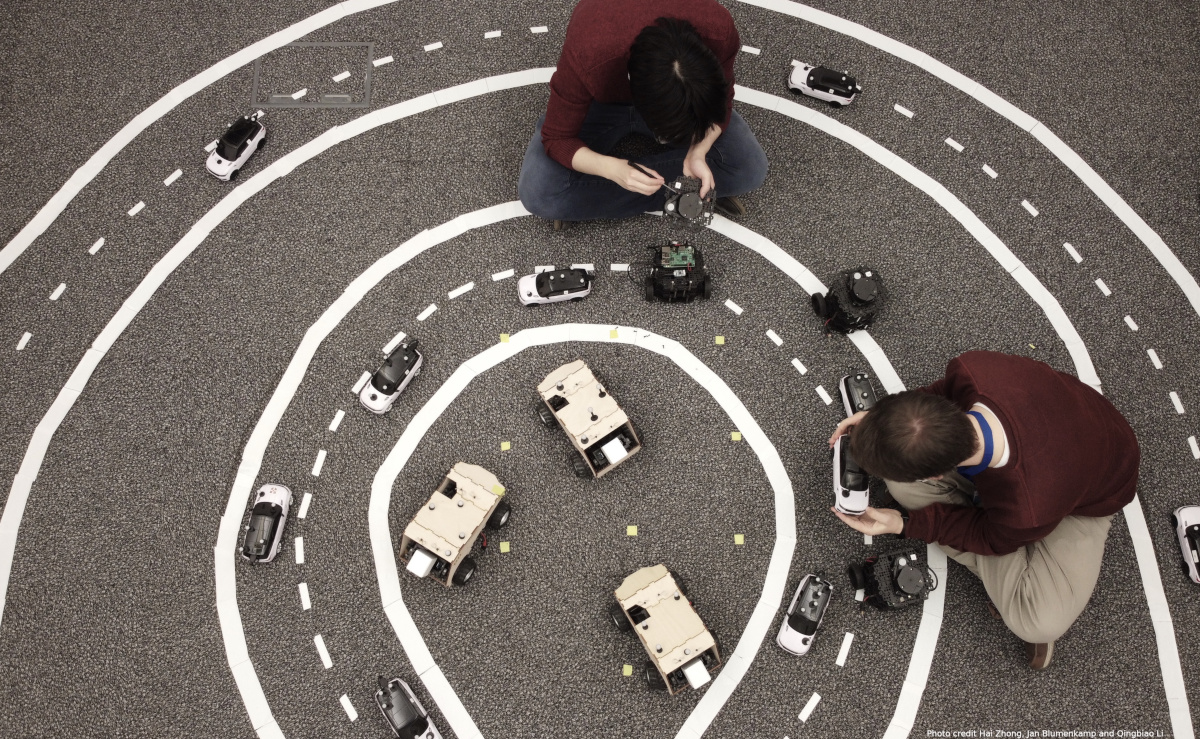
In this episode, Lilly interviews Amanda Prorok, Professor of Computer Science and Technology at the University of Cambridge. Prorok discusses her research on multi-robot and multi-agent systems and learning coordination policies via Graph Neural Networks. They dig into her recent work on self-interested robots and finding explainability in emergent behavior.
Amanda Prorok
Amanda Prorok is an Assistant Professor (University Lecturer) in the Department of Computer Science and Technology, at Cambridge University, and a Fellow of Pembroke College. She serves as Associate Editor for IEEE Robotics and Automation Letters (R-AL) and Associate Editor for Autonomous Robots (AURO). Prior to joining Cambridge, Prorok was a postdoctoral researcher at the General Robotics, Automation, Sensing and Perception (GRASP) Laboratory at the University of Pennsylvania, USA, where she worked with Prof. Vijay Kumar. She completed her PhD at EPFL, Switzerland, with Prof. Alcherio Martinoli.
Links
- Download mp3 (19.6 MB)
- Subscribe to Robohub using iTunes, RSS, or Spotify
- Support us on Patreon
Elmo Motion Control – Gold Solo Triple Twitter digital servo drive
Boston Dynamics Unveils New Robot for Warehouse Automation
The three AI adoption strategies
AI comes in many different shapes and sizes. That applies to the use cases, the underlying technologies as well as the approaches to adopting AI in your organization. As many organizations are looking to adopt AI, an increasing need for tangible frameworks to understand the technology in a business perspective is requested by leaders in all industries.
Some of the key questions asked by leaders are simple. How much time and money is required to adopt AI and solve business problems via AI and what returns do we get for those efforts? That is more than reasonable questions but answering these questions have been an issue in two parts. Firstly the answers have been a moving target with the technology being in an exponential development and as a result the answers of yesterday seem antique today. Secondly the intangible and explorative nature of AI has made it hard to provide such answers at all.
But as AI has matured as a technology, and been packaged into products and ready-to-use solutions, these questions are ready to be answered. The products and solutions might come in different levels of abstractions but they are nevertheless ready for being applied to business problems without much hassle.
The three main AI approaches
To make it easy to understand the efforts and the outcomes of AI it can be divided into three core approaches; Off-the-shelf-AI, AutoAI and Custom AI. The idea is simple. AI has reached a point where some solutions are ready to use out of the box and others need a lot of work before being applied. All approaches come with their own benefits and drawbacks so the trick is to understand these properties and know when to apply what kind. These core AI adoption strategies provide a more concrete foundation for predicting costs, risks and returns when applying AI.
Off-the-shelf-AI

Some AI solutions are ready to use out of the box and need little to no adjustment. Examples can be the Siri in your iPhone, an invoice capture software or speech-to-text solutions. These solutions take minutes to get started and the business models are often AI-as-a-Service making the initial investments low. Often these services are pay-per-use models and consequently implies low risk. The challenge of course is that you get what you get and you shouldn’t get upset. The options for adjusting and making necessary changes for the business problem are usually as low as the costs. More and more of these AI-services are blooming in the AI ecosystem with the large cloud providers as Google and Microsoft taking the lead.
AutoAI

Also known as AutoML, a more technical name, this solution is the hybrid solution giving both freedom to shape the AI as one wishes to a certain extent but also not having to invent the wheel once again. With AutoAI a business can take it’s own data such as documents, customer data or even pictures of products. This data is then used to train AI’s in pre-made environments that have the ability to pick the right algorithms for the job and deploy the AI ready to use in the cloud. As it can be costly to acquire data so there are some efforts required with AutoAI but at least it rarely requires a small army of data scientists. The drawback is also the inflexibility that is inherent in standardized tools. AutoAI also will be challenged when aiming for an AI with the highest possible accuracy.
Custom AI

With Custom AI almost everything is built from scratch. It is a job for data scientists, machine learning engineers and more of a task for R&D than any other place in the organization. The Custom AI approached is usually the weapon of choice when extremely high accuracy is required. Everything can be built and the possibilities are endless. This also usually means at least months or even years of work and experiments. Costly and time consuming. As the AutoAI and Off-the-shelf-AI is becoming more and more available and advanced, Custom AI is more suitable for companies building AI solutions that compete with other AI solutions. With all these extra efforts you might get the small edge that will win you the market.
A final note
For solving problems with AI the most usual approach is advancing towards off-the-shelf and AutoAI. An even more likely future is that a combination of these will be the favorite choice for many organizations adopting AI.
The concept of these approaches is not unique to AI. Almost all other technologies have been through the same natural progression and now the time has come to AI. It is a sign of maturity and that AI is in a state of public property and no longer hidden behind the ivy walls of the top universities.
Of course these approaches are not set in stone and the boundaries between them are fluid and inconsistent. But applying this framework of approaches to the conversation when adopting AI helps the almost magic aura of artificial intelligence become closer to a tool in the toolbox business. And that is where the value starts mounting.
A robotic ball with playful intentions. What if you would use this?
Imagine a ball. When you step closer, the ball rolls away. When you try to catch him, he escapes. This is Fizzy, an autonomous, robotic ball that is programmed to play with children. He is ambiguous, does not like to be captured but does need attention. Little wheels inside the motor make sure that the movement is unconstrained and facilitate his playful character.
In this series of articles, we take robot innovations from their test-lab and bring them to a randomly selected workplace in the outside world. It turns out that Fizzy could flourish in cafés and restaurants, where cheerful attentiveness is a much sought after quality.
‘Children learn through play’. With this truism as a starting point, researcher Boudewijn Boon was asked to enhance the benefits of play for children in care at the Máxima hospital in the south east of the Netherlands. During his research, he found that one thing is essential to playing: it has to be intuitive. This means that all structured approaches to stimulate play, must eventually lead to more unstructured and spontaneous behaviour. Such a beautiful paradox. Who would have thought that the golden idea would be in robotics?
“Children are invited to chase, explore and imagine with this robotic ball, turning a sterile and quiet hospital into a world of playing”
Boudewijn Boon, researcher at the TU Delft
Boon found three core principles for initiating healthy play. First, it must spread out spatially, so kids will explore. The second imperative is for children to use their entire body. And thirdly, there is the need to introduce randomness and spontaneity in the structured environment of a hospital.
Fizzy ticks each box almost perfectly. This autonomous robotic ball does not like to be captured, so children chase after him. Sometimes he begs for attention, which can sparks hide and seek games. All this creates freedom. A big contrast with the structured life that children know during their illness. Boon says: “Children are invited to chase, explore and imagine with this robotic ball; turning a sterile and quiet hospital into a world of play”.
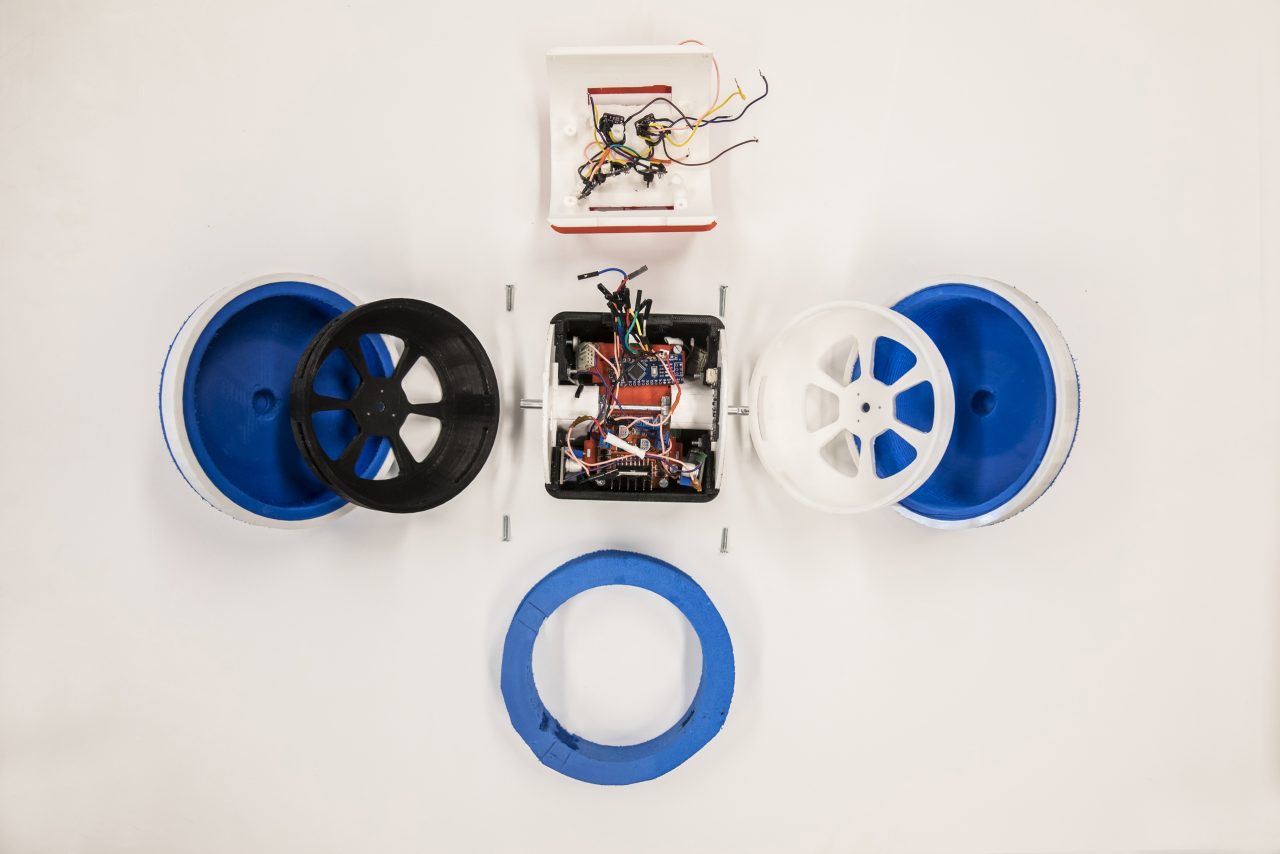
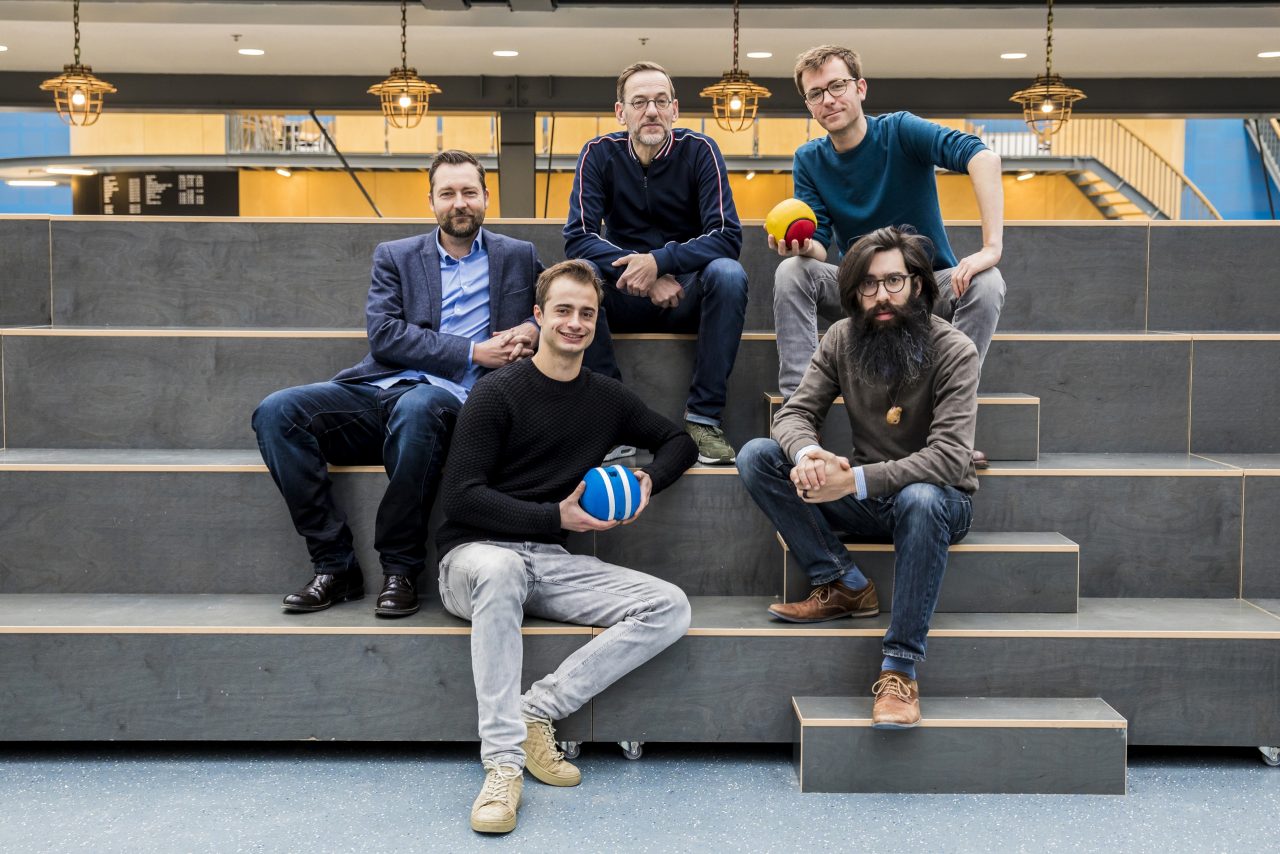
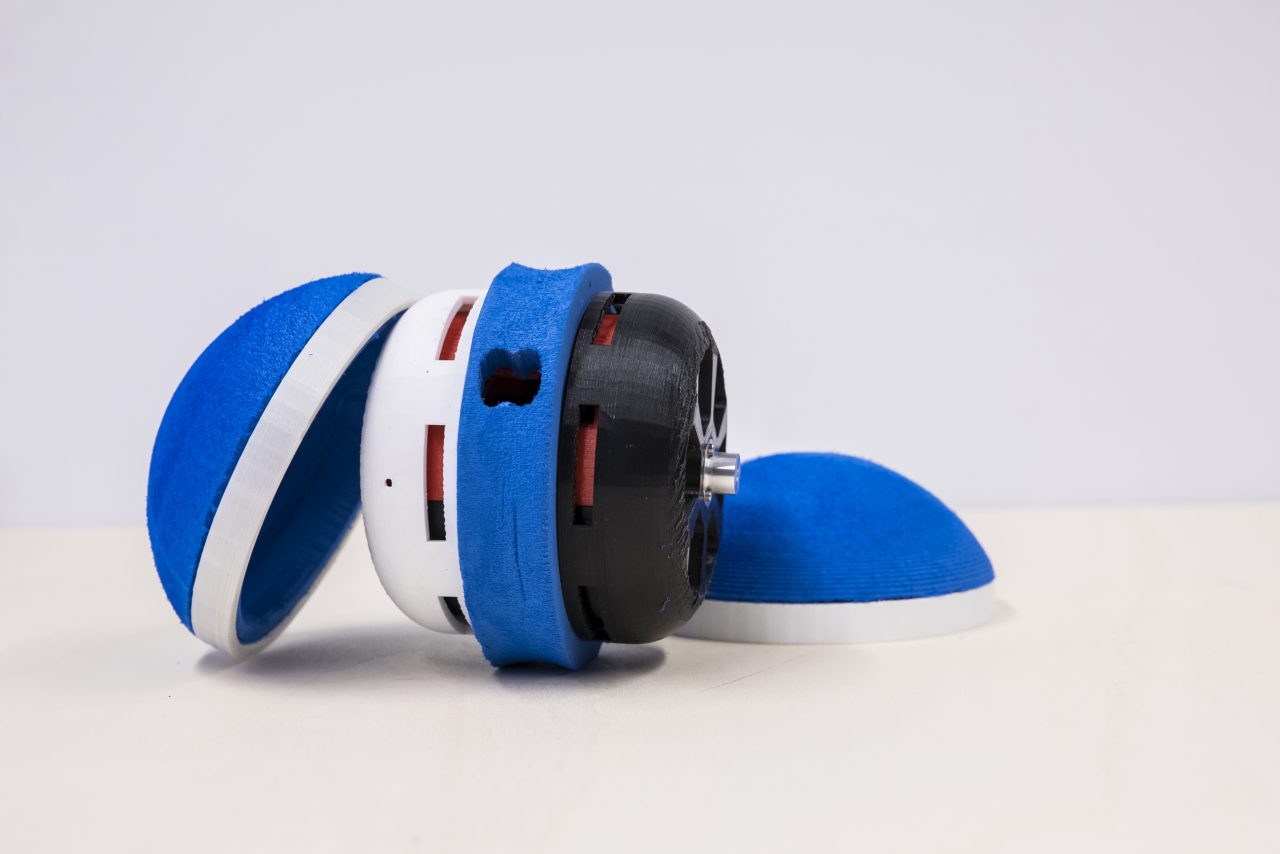
This application of robotics moves us. But at RoboHouse, we also know that innovation often moves in mysterious ways. Once an invention is sold in stores, people may buy it for purposes that researchers could never predict. So we took the Fizzy and traveled to a workplace, to seek out someone with a practical focus and an open mind. A professional, but outside of robotics. We asked her: “What would happen if you would use this?”.
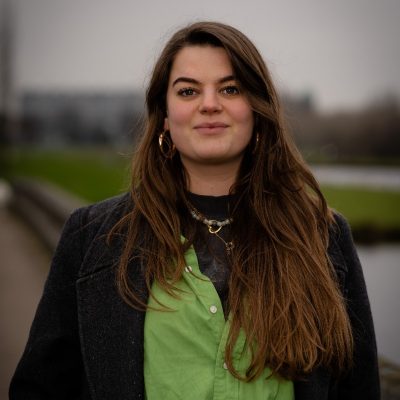
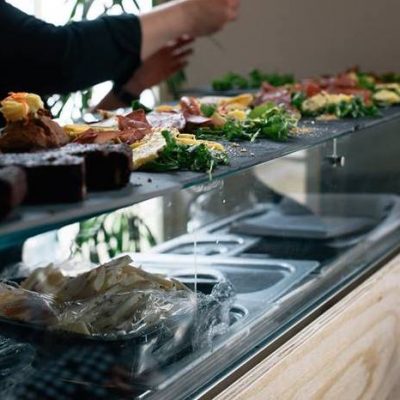
Lisan Peddemors considers our question. She has been working as a chef for several years in a cosy little brasserie in Delft, called Barbaar. We know her from making sugar sweet blondies and beautiful beetroot-hummus-sandwiches. But today, she helps us to anticipate the future.
Idea #1: Cheery and cheap kitchen cleaning
She would not trust a little robot to do any real cooking, she says: “Cooking is so subjective, it depends on thousands of variables whether you add some more salt”. Her first thoughts go out to all the things she would prefer to no longer do. Such as cleaning. The robot could be an excellent solution for that, with some minor adaptions. If you let it roll through soap and water first, Fizzy could clean the parts of the kitchen that Lisan can not get to. Or maybe when it is busy and there is a lot of trash, our little friend can take it out.
“Perhaps I could use it as a cheap kitchen assistant that’s never grumpy,” she says. “But then I wouldn’t have anyone to talk to.”
If the ball would truly have its own mind, it could also be a nuisance in the kitchen. Lisan explains that timing is everything. When it is busy, cooks dance around each other like a real tango. Could a robot ever fit in? But then it strikes her! The ball does not have to do everything right, it only has to sense when something is wrong.
Idea #2: The subtle alarm
Lisan says: “Suppose, when I’m getting something out of the cooler or have a coffee at the bar, Fizzy would give me a little notification that something is burning or a timer is done. Gently, without alarming any customers or colleagues. This cute little ball would bump against my leg and I would know I had to rush back to the kitchen!”.
Fizzy, the discreet warning system with a cute character. That’s how a restaurant chef looks at the robotic ball that Boudewijn Boon and his collaborators are developing. Where Boon sees a robot that adds spontaneity to the sterility of the hospital, Lisan sees a solution for distraction. Quite a difference, but that’s what happens when robotics enters the workplace – we are bound to be surprised, and sometimes maybe even delighted.
The post A robotic ball with playful intentions. What if you would use this? appeared first on RoboValley.