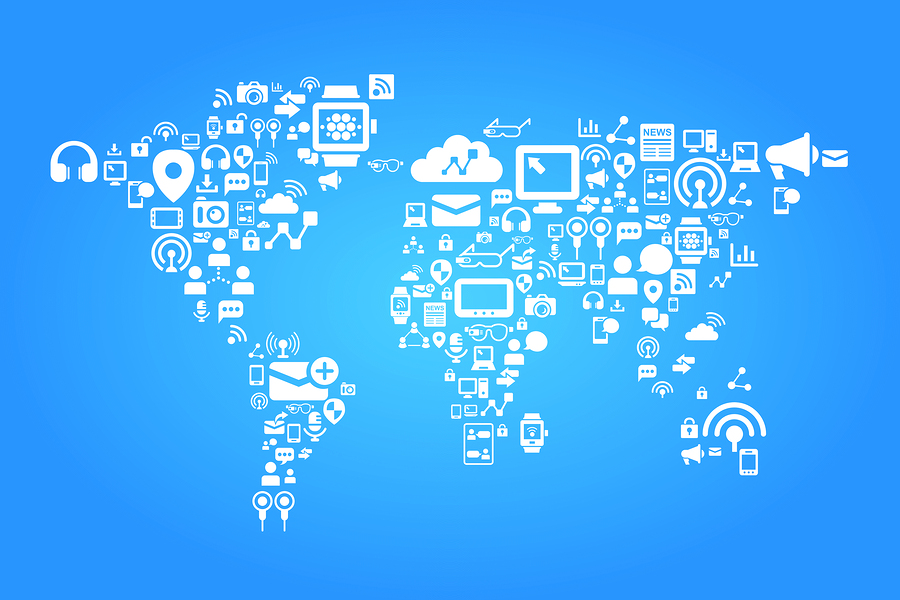
Much excellent work has been done, by many organizations, to develop ‘Roadmaps for Robotics’, in order to steer government policy, innovation investment, and the development of standards and commercialization. However, unless you took part in the roadmapping activity, it can be very hard to find these resources. Silicon Valley Robotics in partnership with the Industrial Activities Board of the IEEE Robotics and Automation Society, is compiling an up to date resource list of various robotics, AIS and AI roadmaps, national or otherwise. This initiative will allow us all to access the best robotics commercialization advice from around the world, to be able to compare and contrast various initiatives and their regional effectiveness, and to provide guidance for countries and companies without their own robotics roadmaps.
Another issue making it harder to find recent robotics roadmaps is the subsumption of robotics into the AI landscape, at least in some national directives. Or it may appear not as robotics but as ‘AIS’, standing for Autonomous Intelligent Systems, such as in the work of OCEANIS, the Open Community for Ethics in Autonomous aNd Intelligent Systems, which hosts a global standards repository. And finally there are subcategories of robotics, ie Autonomous Vehicles, or Self Driving Cars, or Drones, or Surgical Robotics, all of which may have their own roadmaps. This is not an exhaustive list, but with your help we can continue to evolve it.
-
Global Roadmaps:
- IEEE RAS 2050Robotics.org
- OCEANIS, Open Community for Ethics of Autonomous and Intelligent Systems
- OCEANIS, Global Standards Repository
- OECD.ai Policy Observatory
- OECD.ai Stakeholder Initiatives
- OECD.ai National AI Strategies
- Mapping the Evolution of the Robotics Industry: A Cross-Country Comparison
- BCG Robotics Outlook 2030
- 2021 World Bank Review of National AI Strategies and Policies
-
US Roadmaps
- 2020 US National Robotics Roadmap
- ASME: Robotics & Covid 19
- ASME: Accelerating US Robotics for American Prosperity and Security
- 2020 NASA Technology Taxonomy
- 2017 Automated Vehicles 3.0: Preparing for the Future of Transportation
- NITRD Supplement to the President’s FY2021 Budget
- American AI Initiative
-
Canada Roadmaps
-
Latam Roadmaps
- AI for Social Good in Latin America and the Caribbean
- Mexico Agenda National for Artificial Intelligence
- Brazil Industry 4.0
- Brazil E-Digital
- Colombia National Policy for Digital Transformation and Artificial Intelligence
- Chile National Artificial Intelligence Policy
- Argentina Agenda Digital 2030
- Uruguay Artificial Intelligence Strategy for the Public Administration
-
UK & EU Roadmaps
- AI in the UK: No Room for Complacency
- UK’s Industrial Strategy
- SPARC: Robotics 2020 Multi-Annual Roadmap for Robotics in Europe
- SRIDA: AI, Data and Robotics Partnership
- EC: Communication on Artificial Intelligence
- Sweden National Approach for Artificial Intelligence
- Spain RDI Strategy in Artificial Intelligence
- Strategy for the Development of Artificial Intelligence in the Republic of Serbia for the period 2020-2025
- AI Portugal 2030
- Assumptions for the AI strategy in Poland
- Norway National Strategy for Artificial Intelligence
- The Declaration on AI in the Nordic-Baltic Region
- Malta the Ultimate AI Launchpad: A Strategy and Vision for Artificial Intelligence in Malta 2030
- Artificial Intelligence: a strategic vision for Luxembourg
- Lithuanian Artificial Intelligence Strategy: A vision of the future
- Italy National Strategy on Artificial Intelligence
- Made in Germany: Artificial Intelligence Strategy
- Meaningful Artificial Intelligence: Towards a French and European Strategy
- Finland’s Age of Artificial Intelligence
- Finland Leading the Way into the Age of Artificial Intelligence
- Denmark National Strategy for Artificial Intelligence
- Czech National Artificial Intelligence Strategy
- Cyprus National Strategy AI
- AI 4 Belgium
- Austria AIM AT 2030
-
Russia Roadmaps
-
Africa & MENA Roadmaps
- PIDA Closing the Infrastructure Gap Vital for Africa’s Transformation
- Africa50
- AIDA Accelerated Industrial Development for Africa
- UAE Strategy for Artificial Intelligence
- UAE Artificial Intelligence Strategy 2031
- Saudi Arabia Vision 2030
- National AI Strategy: Unlocking Tunisia’s capabilities potential
-
Asia & SE Asia Roadmaps
-
China Roadmaps
-
Japan Roadmaps
-
Australia Roadmaps
-
New Zealand & Pacifica Roadmaps
-
Non Terrestrial Roadmaps
Do you know of robotics roadmaps not yet included? Please share them with us.