How to make hybrid airspace work
New prototype exoskeletons for industrial workers
LOW-MAINTENANCE PALLETISER MAGNET SAVES ROBOT AND MANPOWER
Communicating innovation: What can we do better?
Have you ever seen a bridge collapse on TV? We only care about inspection and maintenance if it does not work. However, robotics are changing the inspection & maintenance landscape, and significant societal and political implications follow the magnitude of innovation. To pay due attention to these developments, the communication on innovation in robotics for inspection & maintenance has to play a role in informing and influencing the target groups for the successful adoption of robotics technologies.
The question on what role communications play in forming the perception of innovative technology was discussed in the workshop “Communicating innovation: What can we do better?” on May 25th, 2022. Experts explained how the innovation uptake should be supported by effective communication of innovations: explaining the benefits, tackling risks and fears of the audiences, and taking innovation closer to the general public.
Innovation & communication experts Marta Palau Franco and Juan Antonio Pavón Losada presented these topics in depth. Also, the recipe for successful innovation communication in robotics in inspection and maintenance was presented by Carlos Matilla Codesal, CEO of FuVeX.
Marta Palau Franco, project officer at euRobotics, working on digital innovation, presented how social context and perception can affect the way we communicate innovative technologies. For example, mentioning the word “drone” in 2014 and in 2022 may not bring the same images to mind to a person. This is due to changes in context and perception around the technology over the years, going from a more military-surveillance use to others such as logistics (e.g. Amazon shipping), entertainment (e.g. drone lights, filming, drone races, etc.), inspection & maintenance, agriculture, etc.
This example emphasizes that intentional engagement with the audience requires a good understanding of the different communication contexts (i.e. social, physical, cultural) and their perception or future expectations of the technology. How can we change someone‘s perception or expectations? We can do that by changing their current references or their interpretation of their prior references.
The people’s perception of innovation might not necessarily correlate with the technological level of innovation. Marta introduced how good and bad communication can create different impressions on the technology. She presented 4 scenarios depending on the level of technological innovation and perception of being innovative (by people) (see the picture below).
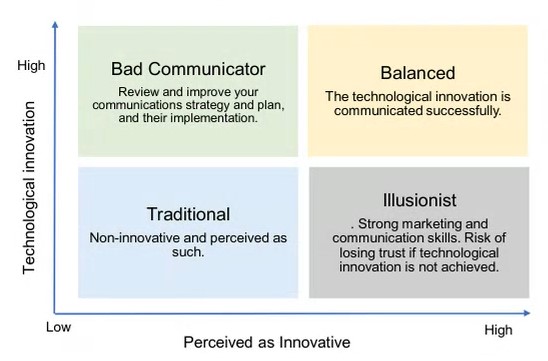
The Balanced scenario is where the company’s technological innovation is high and the company is perceived as such. When there is a high level of technological innovation, but the company is not perceived as innovative, we are in the scenario of the Bad communicator. Companies that find themselves in this group should review their communications and marketing strategy and plan. The scenario of the Illusionists presents a company with low technological innovation, but with strong communication and marketing skills.The company manages to raise audience’s expectations, but risks losing the audience’s trust if the technological innovation is not delivered.
To escape the trap of a bad communicator, whose message does not come across to the audience, Marta offered some advice:
- Be aware of your communication context(s).
- Do you know what people’s perception of your company and technology is? If the perception is negative, try to change it.
- Communicate strategically at different stages of technology development. Be flexible and adapt your communication strategy/plan when necessary. If you are in silence for a prolonged period, you are losing attention. Others will be filling in this gap.
- Listen to feedback provided by your audience and value other perspectives. (e.g., surveys). Burst your bubble, and let in the external input.
- Look for professional marketing and communications advice when needed. Take it seriously.
- By communicating about your innovative technology, you are changing the social and cultural context, contributing to smoother (and safer) adoption of your technology.
- Failure is part of the learning process.
The advice on building trust with stakeholders and communicating in a crisis was suggested by Juan Antonio Pavón Losada, a Project Manager Assistant at PRISMA Lab in the Department of Electrical Engineering and Information Technology at the University of Naples Federico II. Juan is working on the internal and external design and management of European projects’ communication and dissemination.
Juan presented what symptoms define inefficient communication. Firstly, the communication misses the point if it is not consistent and relies on minimal efforts, i.e., “checking the boxes”. In addition, the lack of a defined value proposition (communicating features and activities instead of value) and strategic positioning for the different audiences drives the communication astray from its goal.
How can we solve these mistakes and create efficient communication for inspection and maintenance? Juan has elaborated on the key concepts that are especially relevant in the field of inspection & maintenance. First, the goal of communication is to build relationships, not explain things. Trust, reliability and good reputation are the key goals. In the worst-case scenario, even when an accident happens, if the relationship with stakeholders is firm it will endure the crisis. The trust will absorb the impact of the issue. Also, sufficient attention to communication includes preparation for crisis – identifying who is eligible to speak? What would be the best channel to communicate? What should be the outputs: press release? Press conference? What would be the messages and possible consequences of the messages? Such preparation would help to react quicker and mitigate the crisis.
But, to build trust you need time. That means that the communication about your innovative solution has to be consistent and start early in the process. Apart from this, Juan proposed other tips for effective communication of innovation:
- To be an engaged listener, know the domain and stakeholders, and learn the domain-specific language.
- Express yourself clearly & constantly. Communication is like any relationship – don’t expect people to be there to hear you when you have time. Make some time and show up more than once each 6 months.
- Connect your content to the events happening in the world simultaneously.
- Get communication professionals to do the work and develop high-quality material.
- Engage in new collaborations & break the bubble of your organization to receive new inputs.
- Make intentional language choices and find a balance between technical language and superficial claims without evidence (see the picture below).
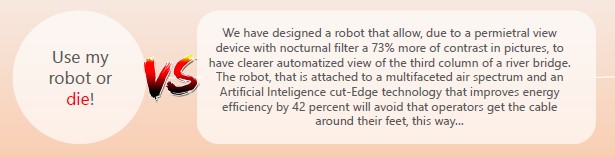
Even though robotics in inspection & maintenance seems to be a rather technical and hardly engaging field for the general audience, Juan encouraged the audience to pay substantial attention to communication in their projects. The due attention converts to allocated budget, planning efforts, strategic positioning, and consistency. However, the main idea for the communication in this field revolves around the TRUST, which is built among stakeholders, whether they are tech providers, projects, institutions, developers, politicians, citizens, etc.
FuVex is a practical example of the successful communication of innovative robotics solutions in inspection & maintenance. FuVeX is a start-up whose mission is to replace manned helicopters with long-range drones in power line inspection and other aerial data capture operations. Carlos Matilla Codesal, CEO and co-founder of FuVeX, highlighted the importance of the intent behind the communication – every word must serve to reach the goal, whether it is to raise awareness, attract investment or find clients. In addition, instead of talking about technical details, which might be the most exhilarating on earth for the developers and engineers, the communication must revolve around the problem you solve. In robotics, this problem is not obviously and easily relatable to the general public (compared to, for example, problems in medicine). However, the responsibility to identify this value proposition and story is not on the audience but the one delivering the message. Vanity is not a strategy. Do not get into the trap of doing things because it feels great but is not valuable for the company. If you are communicating to an audience that is neither your customer nor your investor, but it feels great at the end of the day, you are wasting the resources on the things that matter just to your ego.
The next workshop “Policy issues in Robotics for Infrastructure & Maintenance” will be held during the European Robotics Forum in Rotterdam. If you happen to participate in the Forum, you are welcome to join the workshop on June 29th, at 9.50am (CEST). The experts will discuss how AI regulation affects the domain of Robotics for Infrastructure and Maintenance, what direction the policy is moving to and what are the issues in the pan-European legislation.
Bridging DeepMind research with Alphabet products
Origami millirobots bring health care closer to precisely targeted drug delivery
What’s the Difference Between a Servo Motor and Motion Controller?
Shape-shifting drone flies and dives to find aquatic environmental clues
Rubbery camouflage skin exhibits smart and stretchy behaviors
An approach to produce elaborate flying maneuvers using morphing-wing drones
How to build a working AI only using synthetic data in just 5 minutes
Synthetic data is on the rise in artificial intelligence. It's going to make AI cheaper, better and less biased.
It's also very obtainable and usable. In a short while, it has gone from being an experimental technology. To something, I would not hesitate to use for production AI solutions.
To illustrate that, I will build an AI that can classify the difference between apples and bananas. I will only use images of the two classes generated by another AI - In this case, using DALL-E Mini.
An Apple or Banana recognizer
I will build an image classifier using only easy-to-access, free AutoAI tools.
Generating data
We need around 30 images of each label, bananas and apples.
We will be using DALL-E Mini, an open-source version of NVIDIAs text-to-image model DALL-E 2.
To generate the images, you can go to https://huggingface.co/spaces/dalle-mini/dalle-mini. Here you can prompt the text-to-image model with queries such as:
"Banana on table"
"Banana on random background"
"Apple on table"
"Apple on random background"
Try to match the background you will be testing on.
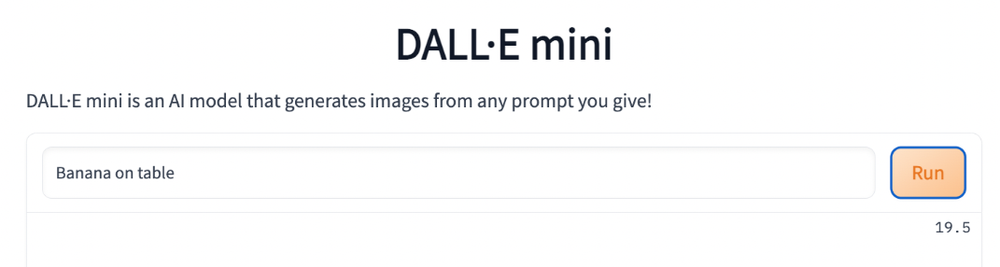
The response should look like this:
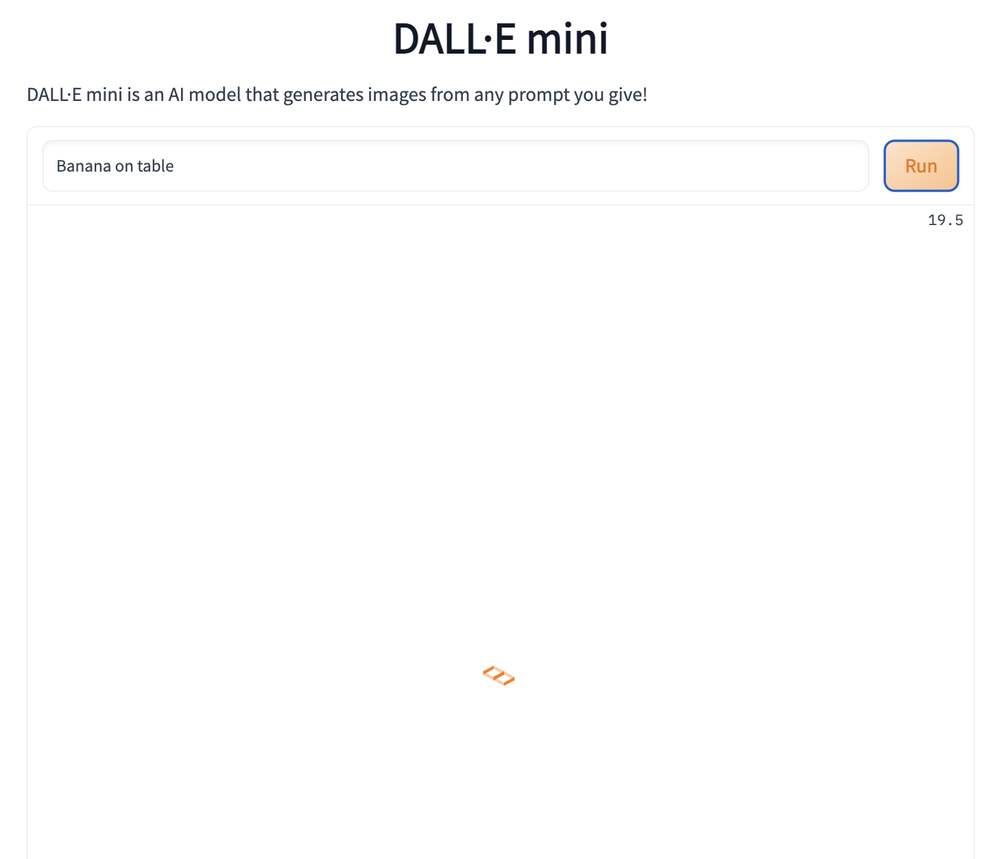
Generate around 30 images of each label and save them.
Creating the model
To create the model, we will be using the tool Teachable Machine. Teachable machine has
Open Teachable Machine and choose Image project and then Standard image model.
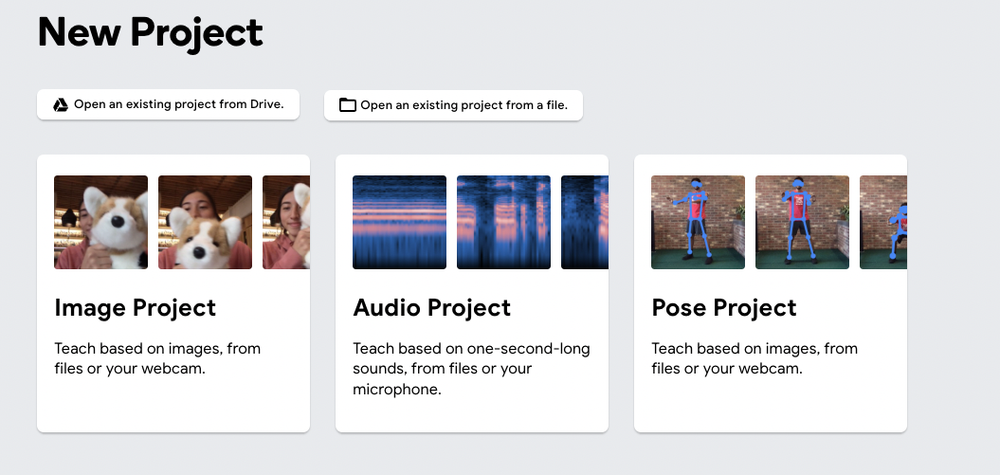
Names your classes Banana and Apple.
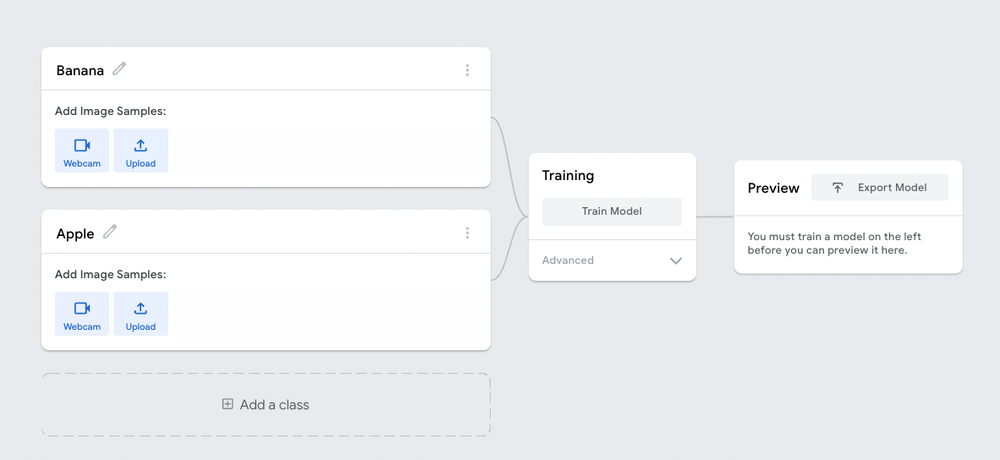
Upload your images from DALL-E Mini to the respective classes.
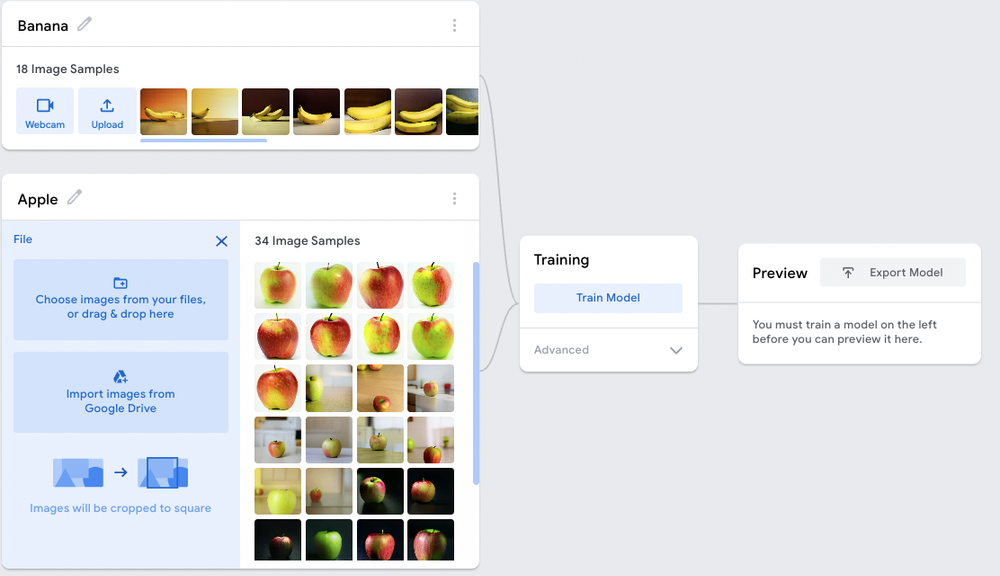
Press Train. Training will only take a few seconds.
Testing the model
Now you can try the model showing a banana or an apple via the webcam view.
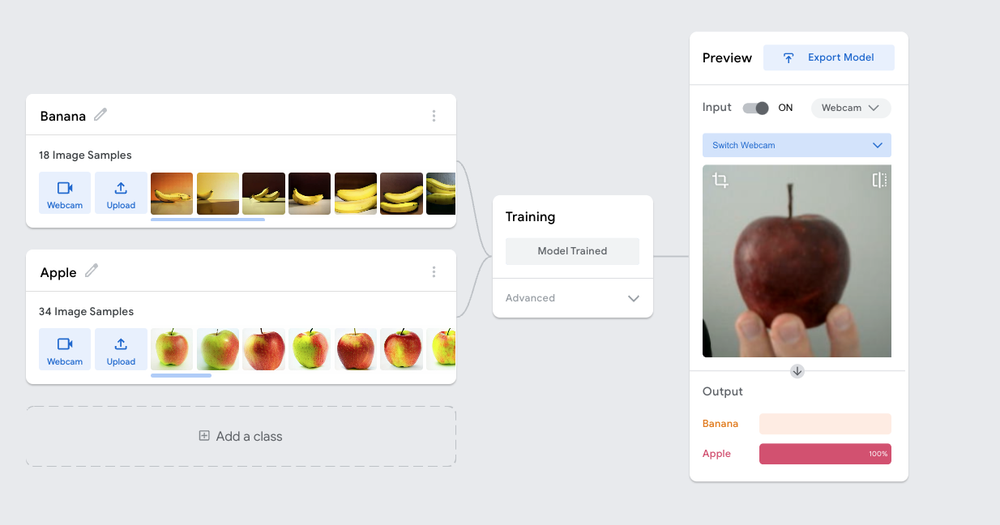
As you can see, it works. The model has been trained only using synthetic data. I think it's remarkable that training models work with only synthetic data.
It suggests a future with significantly lower costs of building AI solutions. That means more people can build AI and utilize the possibilities of the technology.
A fascinating future!
For more tips, sign up for the book here: https://www.danrose.ai/book.
What is synthetic data for artificial intelligence?
This article is a cutout of my forthcoming book that you can sign up for here: https://www.danrose.ai/book.
Synthetic data in AI is probably the subject I think the most about currently, to be honest. It has enormous potential to improve privacy, lower bias and improve model accuracy simultaneously in a giant technological leap in the coming years. Gartner even stated, "By 2024, 60% of the data used for the development of AI and analytics projects will be synthetically generated.". That is a game-changer considering that many people working with AI today haven't even started to adopt this technology.
Synthetic data is data but not actual observations of the world. It is fake data either created by humans or algorithms. It is created artificially or synthetically, but the goal is the same as real data - To represent the world in which the AI is supposed to function. The idea that data for training AI models should accurately represent the world is still a means to the end. Ultimately, the goal of building AI is models that accurately predict to provide a good user experience.
Types of synthetic data
Depending on the data type, text, images and tabular data, there are different approaches and use cases.
Synthetic texts
For language and text AI, you can generate synthetic texts that look like those you would find in the real world. It might even look like gibberish to a human, but if it does the job of representing the world when used for training data, that's good enough.
I have implemented that approach before in a text classification case. I chose this approach because the data could only be stored for three months, making it hard to keep up with seasonal-specific signals. I took the real data that I fed to a language model and fine-tuned the model so that it could produce similar data to real data. We could then generate unlimited data for each label without personal data to train the AI models.
Synthetic images
For images, it's possible to use a text-to-image model that can create synthetic images simply by being prompted by a user with a text. The most famous version of this is NVIDIAs DALL-E 2 model that produces amazingly realistic pictures. An open-source version, available on HuggingFace, called DALL-E Mini, can be tried for free here: https://huggingface.co/spaces/dalle-mini/dalle-mini. You can prompt the model with a short text like "squared strawberry", and you get nine attempts from the model to produce an image of a squared strawberry.
As the model is open-source, you can also download the model and use it for your projects.
The images produced by DALL-E Mini might not be photo-realistic, but it's still good enough to train AI models.
You can try it yourself. Go to the DALL-E Mini and query the model to make images of bananas and apples. Use sentences such as "Banana on table" or "Banana on random background". Do the same with apple until you have 30 or so images of each. You can now upload these images to Teachable Machine to make a banana vs apple recogniser. I promise it will work. If it does not impress you just a tiny bit that you can build AI to recognise objects from purely synthetic images, then I don't know what does.
The use cases here are many. You can synthetically create objects you expect but have not seen in the training data. You can also bring ordinary objects to random backgrounds to make sure you cover unknown scenarios. That will also increase the quality of the models as a change in environment will matter less.
Synthetic tabular data
Tabular data is also possible to generate synthetically. That is popular in healthcare as healthcare is very vulnerable to data issues. Besides the endless combination of scenarios with different diseases and medicine interacting, there's also the privacy issue. Data from one patient's history of diagnostics and medication can be so unique that it can identify individuals. By generating synthetics versions of the actual data, the data can be extended to cover rare scenarios better and anonymise the data. That makes it easy to share between researchers and medical experts.
Models of the world
With synthetic models of the world, we can also experiment with AI solutions before we release them and teach them to become better at a fraction of the cost. Self-driving cars are a perfect use case for this. Self-driving cars can be developed faster and safer by building a synthetic model of the world close to the real world with physics and random scenarios. Many companies building self-driving cars today use models built in the engine Unity, initially intended for computer game development. Cars can try, crash and improve with no humans at risk in a virtual world millions of times before being released.
The good and the bad of synthetic data
The benefits of applying synthetic data to your solutions are many. It can provide more data at a lower price to improve the accuracy of models. It can remove bias by evening out the data by adding to the otherwise rare features or labels that would be a disadvantage for some groups. It can also improve the privacy of people whose personal data might be part of the training data. It can also let us test known and unknown scenarios.
But is it all good? No. Synthetic data is not a silver bullet. It does come with the risk of adding to bias or bringing the data further from the world it is meant to represent. The challenge is that it is difficult to identify the cause of bias as synthetic data is often used where the real data is in shortfall and, by definition, challenging to reality-check. Synthetic data is a promising solution to many problems, but use it with care. As very few have experience in synthetic data in AI, we are unaware of many of the challenges that await.
For more tips, sign up for the book here: https://www.danrose.ai/book.
Robots could help reaching UN goals of sustainable development
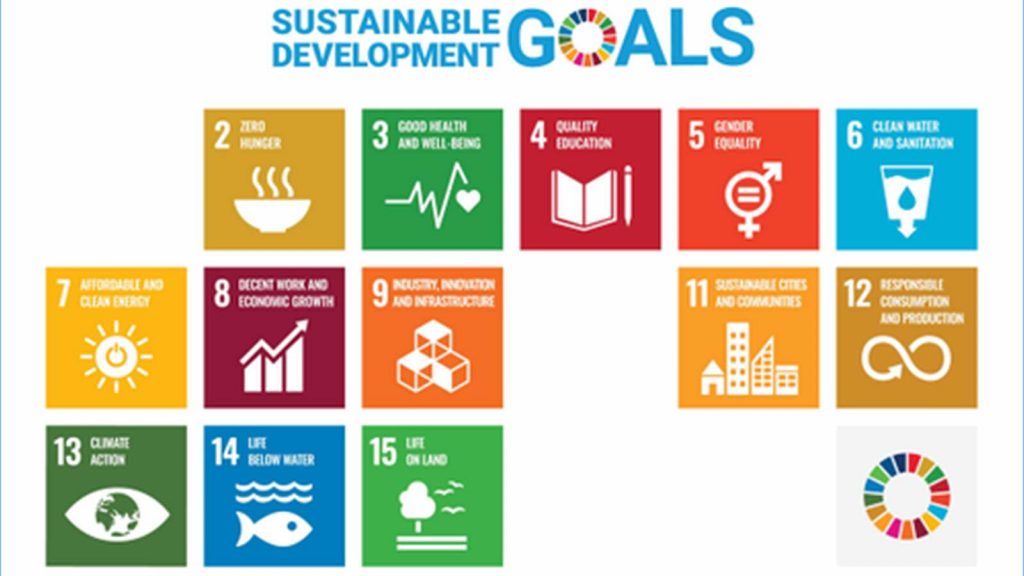
Sustainable Development Goals relevant for robotics
“The use of robots responds to the UN´s call for action,” says Milton Guerry, President of the International Federation of Robotics (IFR). “The IFR supports the United Nations´ sustainable development goals. There are fantastic new ways in which robots save resources and produce green technologies of the future.”
“The transformation on the way to a sustainable use of resources is proving robotics and automation to be key technologies,” says Dr Susanne Bieller, General Secretary of the International Federation of Robotics. “Intelligent automation reduces production costs: This helps battery technology achieve a breakthrough in e-mobility for example or fuel cells production for hydrogen-power as an alternative to fossil energy. At the same time, highly efficient production technology reduces CO2-emissions.”
Clean energy, industrial innovation and sustainable agriculture are just three examples which show how the use of robots contribute to achieve these UN sustainable development goals:
Energies of the future – electricity and solar heat – Clean Energy (UN´s SDG 7)
Pivoting away from fossil fuel makes solar heat and electricity energies of the future: Solar panels are predicted to provide roughly a third of the world’s total electricity demand by 2050 – says the International Energy Agency. Heat usage makes up for half of the world’s energy usage. Keeping pace with this booming customer demand for solar panels and reflectors mean being able to produce units in greater quantities.
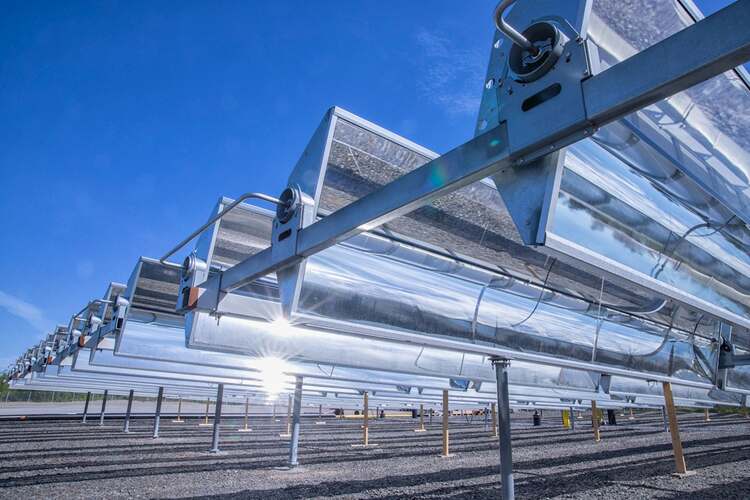
Solar collectors made by Absolicon © Absolicon
Industrial robots are now used as part of an automated factory production line in Sweden. Solar energy company Absolicon has developed what it sees as a gamechanger for the widespread adoption of solar heat as an energy source: A parabolic reflector focuses the solar irradiation on a receiver and turns it into steam with a temperature of up to 160 degrees for the use in industrial production. Almost all industries require heat for production processes. The solar collector´s emission-saving potential: every square meter of a solar thermal collector can produce the equivalent energy of 100 liters of oil.
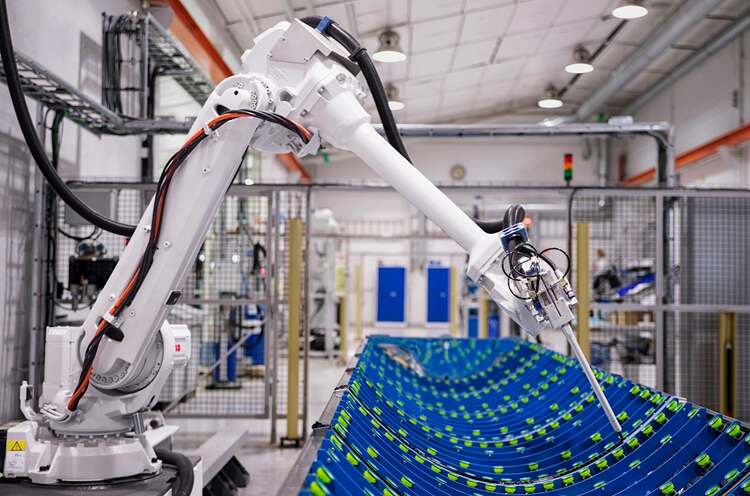
ABB robot in the production line of Absolicon © ABB Robotics
The automation at Absolicon´s factory in Härnösand using two ABB robots has increased production drastically. Where the company previously produced three solar collectors per day with manual production methods, the newly installed robotic production line now has the capacity to produce a finished collector every six minutes.
“Prepare-to-repair” – Industrial Innovation (UN´s SDG 9)
Prepare-to-repair is a successful strategy for robot manufacturers and their customers to save costs and resources. This takes into account that a robot has an average service life of up to thirty years. Using less parts translating into lower risk of future failure is the first step of this approach. To offer long-term repair to customers, storage of parts is a challenge. In order to keep the large number of spare parts in stock, Japanese robot manufacturer Fanuc e.g. runs a central warehouse for Europe. It is located in Luxembourg and has the size of a football pitch with 600,000 spare parts on stock.
Since every hour of machine downtime costs the customer money, it is oftentimes more resource-efficient to transport the spare parts to the customer and repair the machine on site – rather than to manufacture and ship new machines. Manufacturers like ABB, Fanuc, KUKA or Yaskawa all run dedicated repair centers where thousands of industrial robots are refurbished and upgraded for a second life.
“Robots eliminate chemical agents” – Smart Agriculture (UN´s SDG 2)
In agriculture, new field robots eliminate the use of chemical agents. These agricultural robots travel slowly up and down the rows of crops. Equipped with cameras and artificial intelligence software, they are able to locate weeds and burn them selectively with a laser shot. The new technology does not only completely eliminate the use of herbicides. Organic farmers now have an alternative to a related process called “flaming” using propane torches to kill weeds. Flaming could only operate before crops were planted – otherwise it would also have killed the crops.
The Fraunhofer EZRT research centre and partners equipped a farming robot with navigation technology for mechanical weed control in sugar beets. The BlueBob 2.0 also does the job autonomously, so that farmers can devote their time to tasks with higher added value than manual or chemical weeding. Since manual weeding is a very tedious task for humans, the new technology also helps to improve conditions of farm work. Taking over dirty, dull and dangerous tasks is something robots excel at.
Black Swans in Artificial Intelligence
This article is a cutout of my forthcoming book that you can sign up for here: https://www.danrose.ai/book
A significant concept in understanding your data is the concept of Black Swans. The black swan theory was coined by statistician and author of Fooled by Randomness Nassim Nicholas Taleb. A book I can only recommend.
For many years it was commonly known that black swans did not exist. As black swans had never been observed, they did not exist in any data. Had you at that time put your bid on the chance that the next swan you saw would then be black, you would probably bet against such an event. It turned out that there were lots of black swans. They just had not been observed yet. They first became so when we discovered Australia, which was full of black swans. In other words, the data only represented the known and observed world and not the actual world.
That is also an excellent time to mention that data is only historical. And as the notion goes in data science, historical data is quite bad, but the best we got.
Black Swans are, on an individual level, very rare. The latest Covid pandemic is a testament to a rare black Swan event.
One could also mention the financial crisis in 2007. Historically, the housing market never crashed, so any model built on historical data could not have predicted such an event.
But Black swans are not rare on an aggregated level. They are more common than you would intuitively think. Pandemics, crashing housing markets or war in Europe seem like such unique events that they must be uncommon. But less media-attractive firsts and rare events happen all the time.
As such, you should also expect black swans events. Whatever your data shows from historical events is just history. Relying on it should be done with the knowledge that the future can be vastly different. That also translates to the accuracy of models. As AI models use parts of the historical training data to calculate their accuracy, they do so with the past in mind. As a result, you should always expect AI models to perform at least a bit worse in production than they provide as accuracy.
You should also not try to predict black swans. They are, by nature, unpredictable. Instead, make sure the processes. Especially the Decision models and decision levels consider that a black swan can appear at any minute.
For more tips, sign up for the book here: https://www.danrose.ai/book