Researchers expand ability of robots to learn from videos
Robots with tact
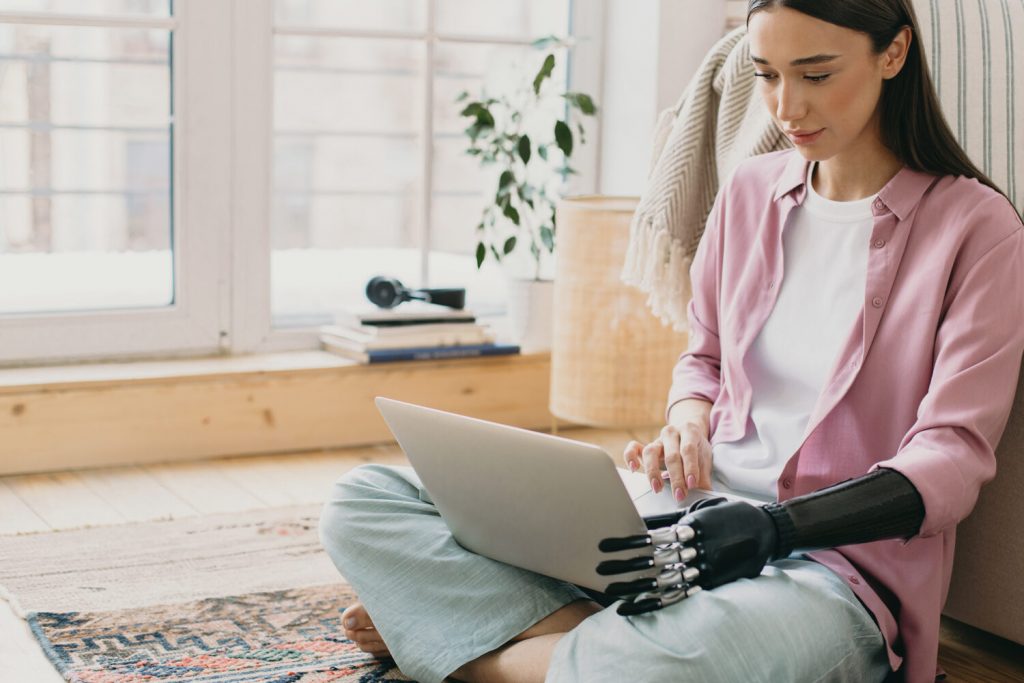
Picture: Adobe Stock/shurkin_son
Artificial hands, even the most sophisticated prostheses, are still by far inferior to human hands. What they lack are the tactile abilities crucial for dexterity. Other challenges include linking sensing to action within the robotic system – and effectively linking it to the human user. Prof. Dr. Philipp Beckerle from FAU has joined with international colleagues to summarize the latest findings in this field of Robotics – and establish an agenda for future research. Their piece in the research journal Science Robotics suggests a sensorimotor control framework for haptically enabled robotic hands, inspired by principles of the human’s central nervous system. Their aim is to link tactile sensing to movement in human-centred, haptically enabled artificial hands. According to the European and American team of researchers, this approach promises improved dexterity for humans controlling robotic hands.
Tactile sensing needs to play a bigger role
“Human manual dexterity relies critically on touch”, explains Prof. Dr. Philipp Beckerle, head of FAU’s Chair of Autonomous Systems and Mechatronics (ASM). “Humans with intact motor function but insensate fingertips can find it very difficult to grasp or manipulate things.” This, he says, indicates that tactile sensing is necessary for human dexterity. “Bioinspired design suggests that lessons from human haptics could enhance the currently limited dexterity of artificial hands. But robotic and prosthetic hands make little use of the many tactile sensors nowadays available and are hence much less dexterous.”
Beckerle, a Mechatronics engineer, has just had the paper “A hierarchical sensorimotor control framework for human-in-the-loop robotic hands” published in the research journal Science Robotics. In this, he unfolds with international colleagues how advanced technologies now provide not only mechatronic and computational components for anthropomorphic limbs, but also sensing ones. The scientists therefore suggest that such recently developed tactile sensing technologies could be incorporated into a general concept of “electronic skins”. “These include dense arrays of normal-force-sensing tactile elements in contrast to fingertips with a more comprehensive force perception”, the paper reads. “This would provide a directional force-distribution map over the entire sensing surface, and complex three-dimensional architectures, mimicking the mechanical properties and multimodal sensing of human fingertips.” Tactile sensing systems mounted on mechatronic limbs could therefore provide robotic systems with the complex representations needed to characterize, identify and manipulate, e.g. objects.
Human principles as inspiration for future designs
To achieve haptically informed and dexterous machines, the researchers secondly propose taking inspiration from the principles of the hierarchically organised human central nervous system (CNS). The CNS controls, which signals the brain receives from tactile senses and sends back to the body. The authors propose a conceptual framework in which a bioinspired touch-enabled robot shares control with the human – to a degree that the human sets. Principals of the framework include parallel processing of tasks, integration of feedforward and feedback control as well as a dynamic balance between subconscious and conscious processing. These could not only be applied in the design of bionic limbs, but also that of virtual avatars or remotely navigated telerobots.
It remains yet another challenge though to effectively interface a human user with touch-enabled robotic hands. “Enhancing haptic robots with high-density tactile sensing can substantially improve their capabilities but raises questions about how best to transmit these signals to a human controller, how to navigate shared perception and action in human-machine systems”, the paper reads. It remains largely unclear how to manage agency and task assignment, to maximize utility and user experience in human-in-the-loop systems. “Particularly challenging is how to exploit the varied and abundant tactile data generated by haptic devices. Yet, human principles provide inspiration for the future design of mechatronic systems that can function like humans, alongside humans, and even as replacement parts for humans.”
Philipp Beckerle’s Chair is part of the FAU’s Departments of Electrical Engineering, Electronics and Information Technology as well as the Department of Artificial Intelligence in Biomedical Engineering. “Our mission at ASM is to research human-centric mechatronics and robotics and strive for solutions that combine the desired performance with user-friendly interaction properties”, Beckerle explains. “Our focus is on wearable systems such as prostheses or exoskeletons, cognitive systems such as collaborative or humanoid robots and generally on tasks with close human-robot interaction. The human factors are crucial in such scenarios in order to meet the user’s needs and to achieve synergetic interface as well as interaction between humans and machines.”
Apart from Prof. Dr. Beckerle, scientists from the Universities of Genoa, Pisa and Rome, Aalborg, Bangor and Pittsburgh as well as the Imperial College London and the University of Southern California, Los Angeles were contributing to the paper.
RoboCat: A self-improving robotic agent
RoboCat: A self-improving robotic agent
An open-source benchmark to evaluate the manipulation and planning skills of assembly robots
Robotics Providers: Challenges and Opportunities in a Dynamic Industrial Equipment Market
Flowstate: Intrinsic’s app to simplify the creation of robotics applications
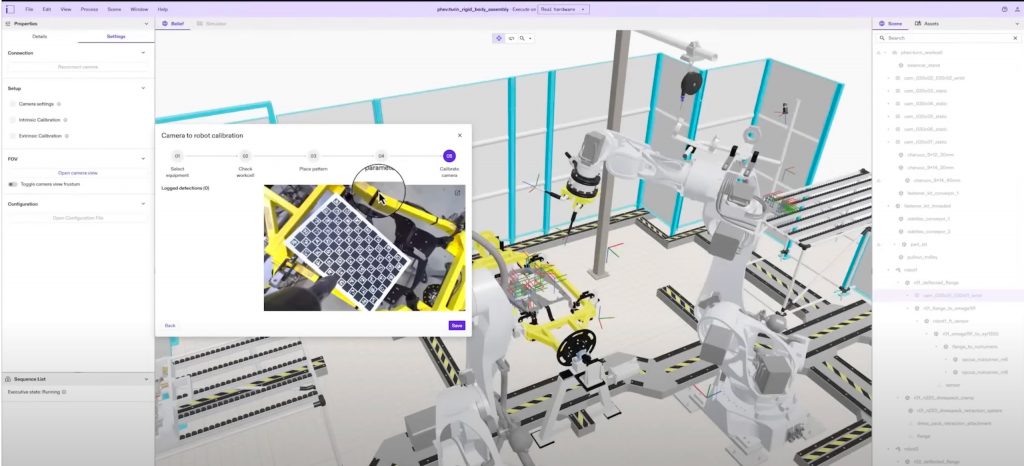
Copyright by Intrinsic.
Finally, Intrinsic (a spin-off of Google-X) has revealed the product they have been working with the help of the Open Source Robotics Corporation team (among others): Flowstate!
What is Flowstate?
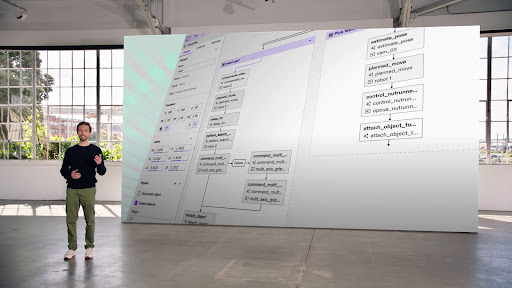
Introducing Intrinsic Flowstate | Intrinsic (image copyright by Intrinsic)
Flowstate is a web-based software designed to simplify the creation of software applications for industrial robots. The application provides a user-friendly desktop environment where blocks can be combined to define the desired behavior of an industrial robot for specific tasks.
Good points
- Flowstate offers a range of features, including simulation testing, debugging tools, and seamless deployment to real robots.
- It is based on ROS, so we should be able to use our favorite framework and all the existing software to program on it, including Gazebo simulations.
- It has a behavior tree based system to graphically control the flow of the program, which simplifies the way to create programs by just moving blocks around. But it is also possible to switch to expert mode to manually touch the code.
- It has a library of already existing robot models and hardware ready to be added, but you can also add your own.
- Additionally, the application provides pre-built AI skills that can be utilized as modules to achieve complex AI results without the need for manual coding.
- One limitiation (but I actually consider a good point) is that the tool is thought for industrial robots not for service robots in general. This is good because it provides a focus for the product, specially for this initial release
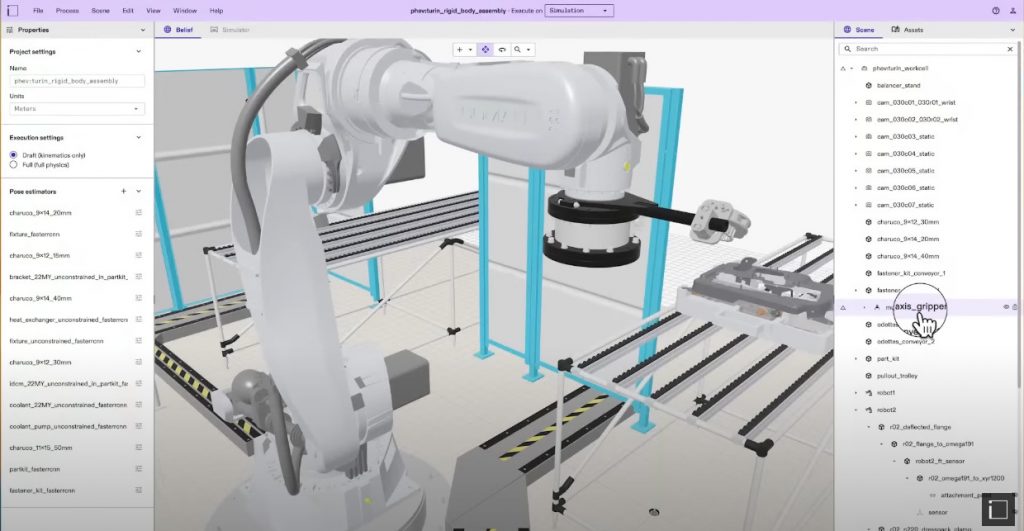
Flowstate | Intrinsic (image copyright by Intrinsic)
Based on the official post and the keynote released on Monday, May 15, 2023 (available here), this is the information we have gathered so far. However, we currently lack a comprehensive understanding of how the software works, its complete feature set, and any potential limitations. To gain more insights, we must wait until July of this year, hoping that I will be among the lucky participants selected for the private beta (open call to the beta still available here).
Unclear points
Even if I find interesting the proposal of Intrinsic, I have identified three potential concerns regarding it:
- Interoperability across different hardware and software platforms poses a challenge. The recruitment of the full OSRC team by Intrinsic appears to address this issue, given that ROS is currently the closest system in the market to achieve such interoperability. However, widespread adoption of ROS by industrial robot manufacturers is still limited, with only a few companies embracing it.
Ensuring hardware interoperability necessitates the adoption of a common framework by robot manufacturers, which is currently a distant reality. What we, ROS developers, aim right now is to be able to have somebody build the ROS drivers for the robotic arm we want to use (like for example the manufacturers of the robot, or the team of ROS Industrial). However, manufacturers generally hesitate to develop ROS drivers due to potential business limitations and their aims for customer lock-in. Unless a platform dedicates substantial resources to developing and maintaining drivers for supported robots, the challenge of hardware interoperability cannot be solved by a platform alone (actually, that is one of the goals that ROS-Industrial is trying to achieve).
Google possesses the potential to unite hardware companies towards this goal, as Wendy Tan White, the CEO of Intrinsic mentioned, “This is an ecosystem effort” However, it is crucial for the industrial community to perceive tangible benefits and value in supporting this initiative beyond merely assisting others in building their businesses. The specific benefits that the ecosystem stands to gain by supporting this initiative remain unclear.
- The availability of pre-made AI skills for robots is a complex task. Consider the widely used skills in ROS, such as navigation or arm path planning, exemplified by Nav2 and MoveIt, which offer excellent functionality. However, integrating these skills into new robots is not as simple as plug-and-play. In fact, dedicated courses exist to teach users how to effectively utilize the different components of navigation within a robot. This highlights the challenges associated with implementing such skills for robots in general. Thus, it is reasonable to anticipate similar difficulties in developing pre-made skills within Flowstate.
- A final point that I don’t see clear (because it was not addressed in the presentation) is how the company is going to do business with Flowstate. This is a very important point for every robotics developer because we don’t want to be locked into proprietary systems. We understand that companies must have a business, but we want to understand clearly what the business is so we can decide if that is convenient or not for us, both in the short and the long run. For instance, Robomaker from Amazon did not gain much traction because forced the developers to pay for the cloud while running Robomaker, when they could do the same thing (with less fancy stuff) in their own local computers for free
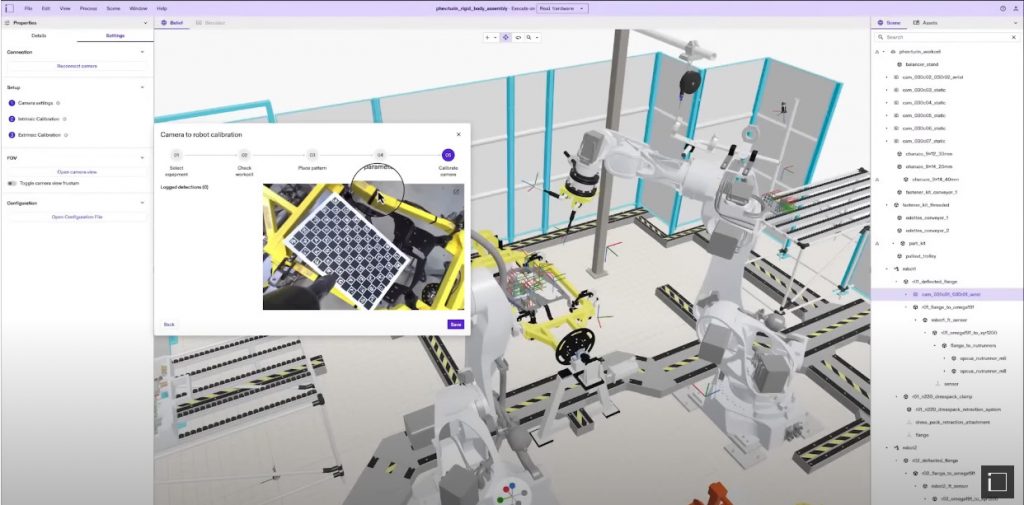
Flowstate | Intrinsic (image copyright by Intrinsic)
Conclusion
Overall, while Flowstate shows promising, further information and hands-on experience are required to assess its effectiveness and address potential challenges.
I have applied to the restricted beta. I hope to be selected so I can have a first hand experience and report about it.
Please make sure to read the original post by Wendy Tan White and the keynote presentation, both can be found at the web of Intrinsic.
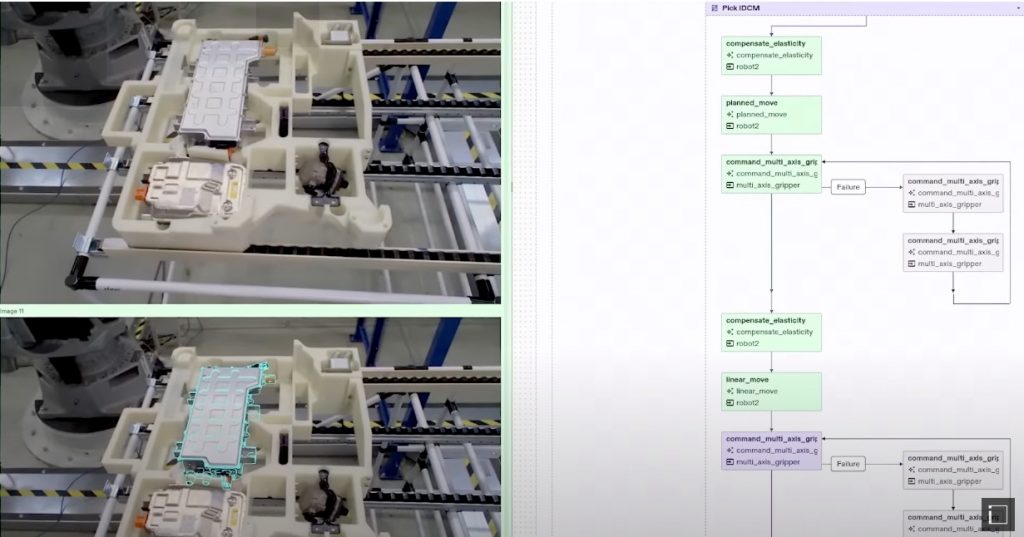
Flowstate | Intrinsic (image copyright by Intrinsic)
Machine-learning method used for self-driving cars could improve lives of type-1 diabetes patients
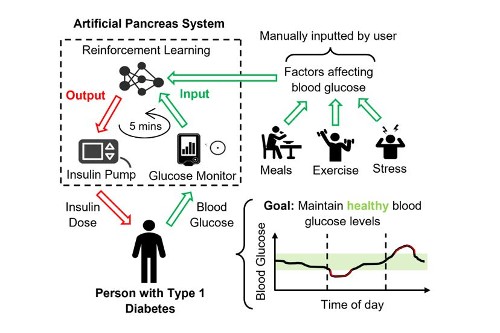
Artificial Pancreas System with Reinforcement Learning. Image credit: Harry Emerson
Scientists at the University of Bristol have shown that reinforcement learning, a type of machine learning in which a computer program learns to make decisions by trying different actions, significantly outperforms commercial blood glucose controllers in terms of safety and effectiveness. By using offline reinforcement learning, where the algorithm learns from patient records, the researchers improve on prior work, showing that good blood glucose control can be achieved by learning from the decisions of the patient rather than by trial and error.
Type 1 diabetes is one of the most prevalent auto-immune conditions in the UK and is characterised by an insufficiency of the hormone insulin, which is responsible for blood glucose regulation.
Many factors affect a person’s blood glucose and therefore it can be a challenging and burdensome task to select the correct insulin dose for a given scenario. Current artificial pancreas devices provide automated insulin dosing but are limited by their simplistic decision-making algorithms.
However a new study, published in the Journal of Biomedical Informatics, shows offline reinforcement learning could represent an important milestone of care for people living with the condition. The largest improvement was in children, who experienced an additional one-and-a-half hours in the target glucose range per day.
Children represent a particularly important group as they are often unable to manage their diabetes without assistance and an improvement of this size would result in markedly better long-term health outcomes.
Lead author Harry Emerson from Bristol’s Department of Engineering Mathematics, explained: “My research explores whether reinforcement learning could be used to develop safer and more effective insulin dosing strategies.
“These machine learning driven algorithms have demonstrated superhuman performance in playing chess and piloting self-driving cars, and therefore could feasibly learn to perform highly personalised insulin dosing from pre-collected blood glucose data.
“This particular piece of work focuses specifically on offline reinforcement learning, in which the algorithm learns to act by observing examples of good and bad blood glucose control.
“Prior reinforcement learning methods in this area predominantly utilise a process of trial-and-error to identify good actions, which could expose a real-world patient to unsafe insulin doses.”
Due to the high risk associated with incorrect insulin dosing, experiments were performed using the FDA-approved UVA/Padova simulator, which creates a suite of virtual patients to test type 1 diabetes control algorithms. State-of-the-art offline reinforcement learning algorithms were evaluated against one of the most widely used artificial pancreas control algorithms. This comparison was conducted across 30 virtual patients (adults, adolescents and children) and considered 7,000 days of data, with performance being evaluated in accordance with current clinical guidelines. The simulator was also extended to consider realistic implementation challenges, such as measurement errors, incorrect patient information and limited quantities of available data.
This work provides a basis for continued reinforcement learning research in glucose control; demonstrating the potential of the approach to improve the health outcomes of people with type 1 diabetes, while highlighting the method’s shortcomings and areas of necessary future development.
The researchers’ ultimate goal is to deploy reinforcement learning in real-world artificial pancreas systems. These devices operate with limited patient oversight and consequently will require significant evidence of safety and effectiveness to achieve regulatory approval.
Harry added: ”This research demonstrates machine learning’s potential to learn effective insulin dosing strategies from the pre-collected type 1 diabetes data. The explored method outperforms one of the most widely used commercial artificial pancreas algorithms and demonstrates an ability to leverage a person’s habits and schedule to respond more quickly to dangerous events.”
YouTube: Enhancing the user experience
A multisensory simulation platform to train and test home robots
YouTube: Enhancing the user experience
World’s most remote robot automates Amazon reforestation project
Robot Talk Episode 53 – Robert Richardson
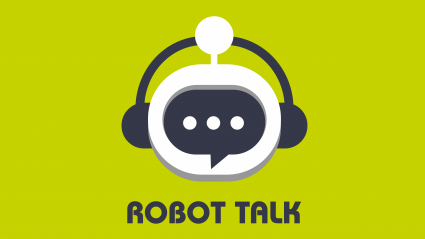
Claire chatted to Robert Richardson from the University of Leeds all about 3D printing, robot design, and infrastructure repair.
Robert Richardson is Professor of Robotics in the School of Mechanical Engineering at the University of Leeds, and executive chair of EPSRC UK-RAS network. His research interests include robotics for civil infrastructure inspection and repair, making smart bodies for smart robots, and robotics for 3D printing applications. As Innovation Director for University of Leeds spin out company Acuity robotics, he is working towards real world impact in civil inspection tasks. In 2011 he led an international team to develop and deploy robots into the Great Pyramid of Giza in Egypt.