Robot swarms neutralize harmful Byzantine robots using a blockchain-based token economy
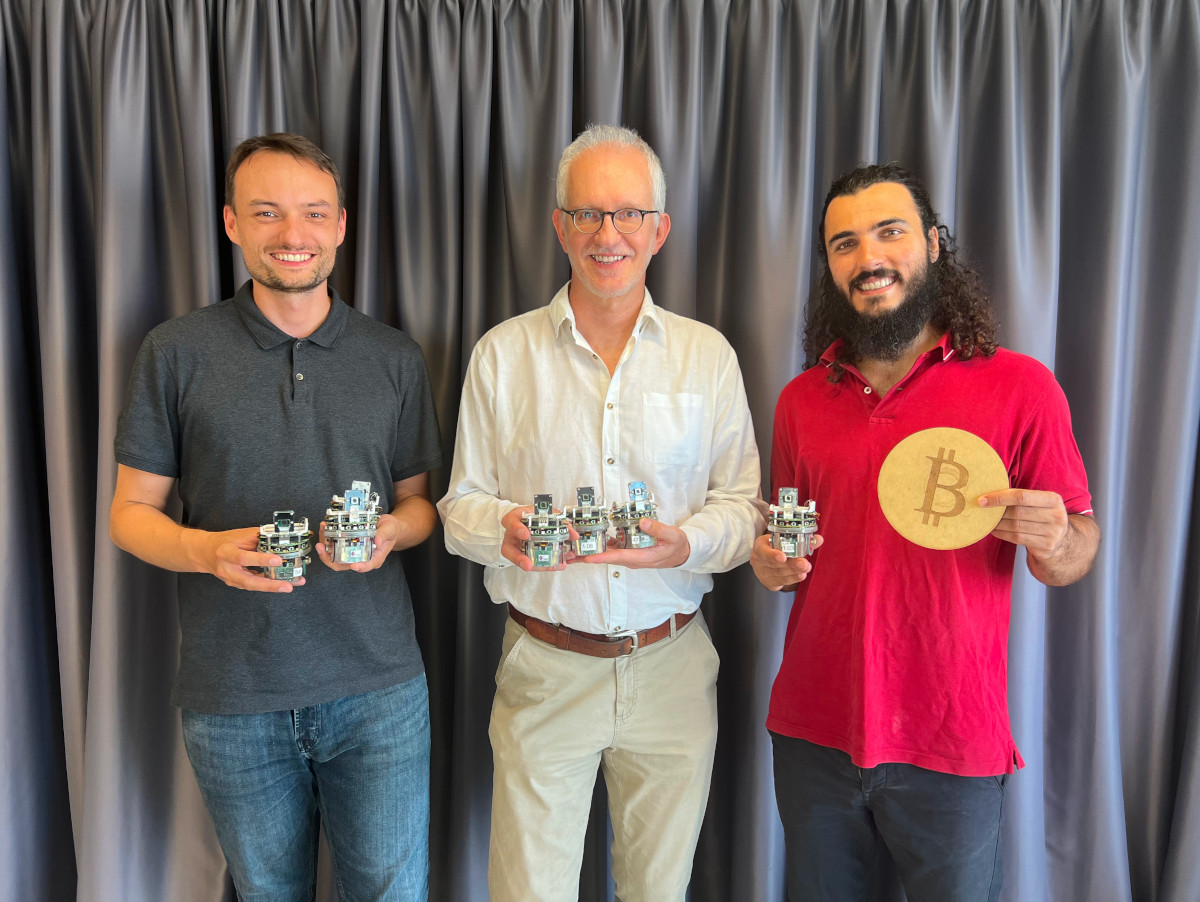
Dr. Volker Strobel, postdoctoral researcher; Prof. Marco Dorigo, research director of the F.R.S.-FNRS; and Alexandre Pacheco, doctoral student. The researchers from the Université Libre de Bruxelles, Belgium. Credit: IRIDIA, Université Libre de Bruxelles
In a new study, we demonstrate the potential of blockchain technology, known from cryptocurrencies such as Bitcoin and Ethereum, to secure the coordination of robot swarms. In experiments conducted with both real and simulated robots, we show how blockchain technology enables a robot swarm to neutralize harmful robots without human intervention, thus enabling the deployment of autonomous and safe robot swarms.
Robot swarms are multi-robot systems that consist of many robots that collaborate in order to perform a task. They do not need a central control unit but the collective behavior of the swarm is rather a result of local interactions among robots. Thanks to this decentralization, robot swarms can work independently of external infrastructure, such as the Internet. This makes them particularly suitable for applications in a wide range of different environments such as underground, underwater, at sea, and in space.
Even though current swarm robotics applications are exclusively demonstrated in research environments, experts anticipate that in the non-distant future, robot swarms will support us in our everyday life. Robot swarms might perform environmental monitoring, underwater exploration, infrastructure inspection, and waste management—and thus make significant contributions to the transition into a fossil-free future with low pollution and high quality of life. In some of these activities, robot swarms will even outperform humans, leading to higher-quality results while ensuring our safety.
Once robot swarms are deployed in the real world, however, it is very likely that some robots in a swarm will break down (for example, due to harsh weather conditions) or might even be hacked. Such robots will not behave as intended and are called “Byzantine” robots. Recent research has shown that the actions of a very small minority of such Byzantine robots in a swarm can—similar to a virus—spread in the swarm and thus break down the whole system. Although security issues are crucial for the real-world deployment of robot swarms, security research in swarm robotics is lacking behind.
In Internet networks, Byzantine users such as hackers, have been successfully prevented from manipulating information by using blockchain technology. Blockchain technology is the technology behind Bitcoin: it enables users to agree on `who owns what’ without requiring a trusted third party such as a bank. Originally, blockchain technology was only meant to exchange units of a digital currency, such as Bitcoin. However, some years after Bitcoin’s release, blockchain-based smart contracts were introduced by the Ethereum framework: these smart contracts are programming code executed in a blockchain network. As no one can manipulate or stop this code, smart contracts enable “code is law”: contracts are automatically executed and do not need a trusted third party, such as a court, to be enforced.
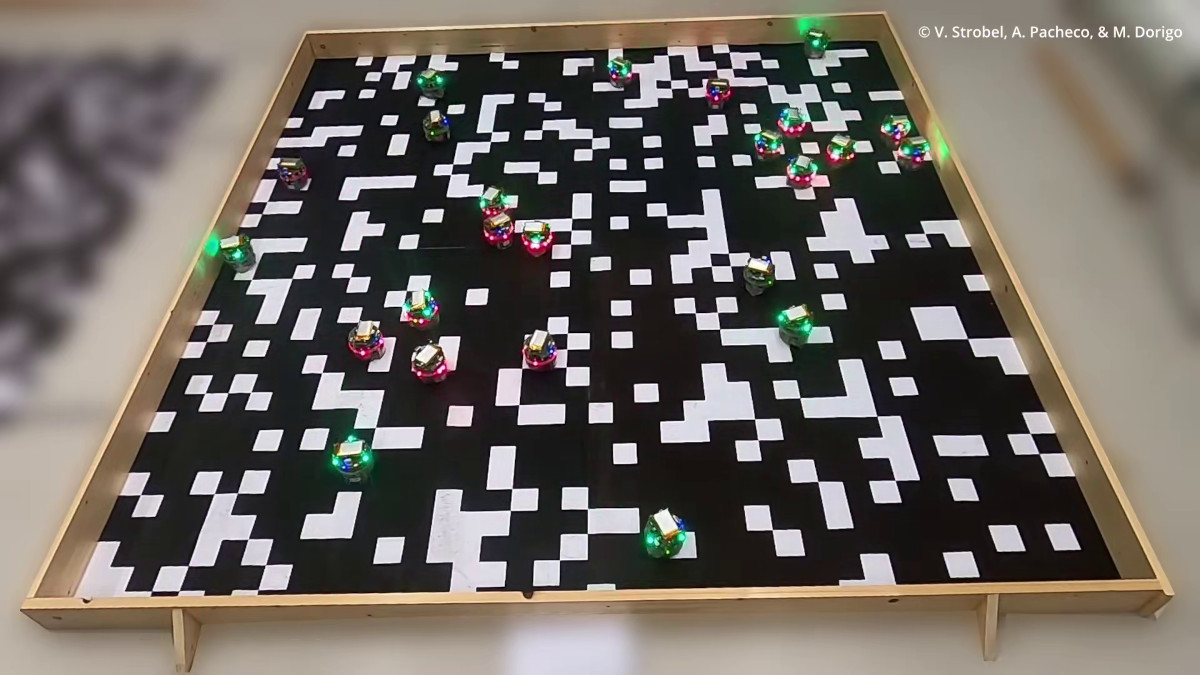
So far, it was not clear whether large robot swarms could be controlled using blockchain and smart contracts. To address this open question, we presented a comprehensive study with both real and simulated robots in a collective-sensing scenario: the goal of the robot swarm is to provide an estimate of an environmental feature. To do so the robots need to sample the environment and then agree on the feature value. In our experiments, each robot is a member of a blockchain network maintained by the robots themselves. The robots send their estimates of environmental features to a smart contract that is shared by all the robots in the swarm. These estimates are aggregated by the smart contract that uses them to generate the requested estimate of the environmental feature. In this smart contract, we implemented economic mechanisms that ensure that good (non-Byzantine) robots are rewarded for sending useful information, whereas harmful Byzantine robots are penalized. The resulting robot economy prevents the Byzantine robots from participating in the swarm activities and influencing the swarm behavior.
Adding a blockchain to a robot swarm increases the robots’ computational requirements, such as CPU, RAM, and disk space usage. In fact, it was an open question whether running blockchain software on real robot swarms was possible at all. Our experiments have demonstrated that this is indeed possible as the computational requirements are manageable: the additional CPU, RAM, and disk space usage have a minor impact on the robot performance. This successful integration of blockchain technology into robot swarms paves the way for a wide range of secure robotic applications. To favor these future developments, we have released our software frameworks as open-source.
Google DeepMind’s latest research at ICML 2023
Google DeepMind’s latest research at ICML 2023
Google DeepMind’s latest research at ICML 2023
Teaching robots to teach other robots
Enabling autonomous exploration in robots
How to give AI-based robots empathy so they won’t want to kill us
7 Reasons Robots Make a Huge Difference in Food Processing
Scientists develop stretchable robotic fabrics that enable error-prone robotic modules to march in formation
Bot inspired by baby turtles can swim under the sand
A faster way to teach a robot
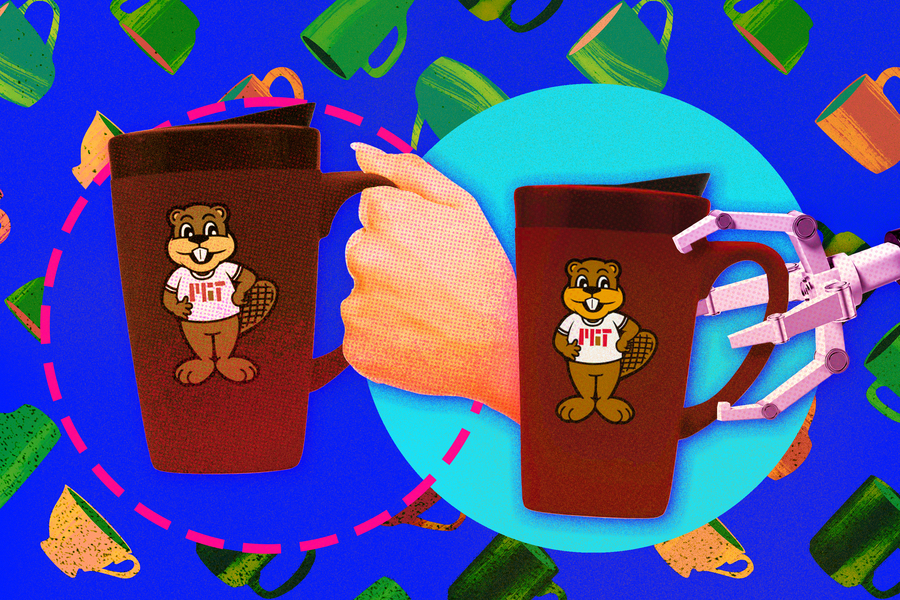
Researchers from MIT and elsewhere have developed a technique that enables a human to efficiently fine-tune a robot that failed to complete a desired task— like picking up a unique mug— with very little effort on the part of the human. Image: Jose-Luis Olivares/MIT with images from iStock and The Coop
By Adam Zewe | MIT News Office
Imagine purchasing a robot to perform household tasks. This robot was built and trained in a factory on a certain set of tasks and has never seen the items in your home. When you ask it to pick up a mug from your kitchen table, it might not recognize your mug (perhaps because this mug is painted with an unusual image, say, of MIT’s mascot, Tim the Beaver). So, the robot fails.
“Right now, the way we train these robots, when they fail, we don’t really know why. So you would just throw up your hands and say, ‘OK, I guess we have to start over.’ A critical component that is missing from this system is enabling the robot to demonstrate why it is failing so the user can give it feedback,” says Andi Peng, an electrical engineering and computer science (EECS) graduate student at MIT.
Peng and her collaborators at MIT, New York University, and the University of California at Berkeley created a framework that enables humans to quickly teach a robot what they want it to do, with a minimal amount of effort.
When a robot fails, the system uses an algorithm to generate counterfactual explanations that describe what needed to change for the robot to succeed. For instance, maybe the robot would have been able to pick up the mug if the mug were a certain color. It shows these counterfactuals to the human and asks for feedback on why the robot failed. Then the system utilizes this feedback and the counterfactual explanations to generate new data it uses to fine-tune the robot.
Fine-tuning involves tweaking a machine-learning model that has already been trained to perform one task, so it can perform a second, similar task.
The researchers tested this technique in simulations and found that it could teach a robot more efficiently than other methods. The robots trained with this framework performed better, while the training process consumed less of a human’s time.
This framework could help robots learn faster in new environments without requiring a user to have technical knowledge. In the long run, this could be a step toward enabling general-purpose robots to efficiently perform daily tasks for the elderly or individuals with disabilities in a variety of settings.
Peng, the lead author, is joined by co-authors Aviv Netanyahu, an EECS graduate student; Mark Ho, an assistant professor at the Stevens Institute of Technology; Tianmin Shu, an MIT postdoc; Andreea Bobu, a graduate student at UC Berkeley; and senior authors Julie Shah, an MIT professor of aeronautics and astronautics and the director of the Interactive Robotics Group in the Computer Science and Artificial Intelligence Laboratory (CSAIL), and Pulkit Agrawal, a professor in CSAIL. The research will be presented at the International Conference on Machine Learning.
On-the-job training
Robots often fail due to distribution shift — the robot is presented with objects and spaces it did not see during training, and it doesn’t understand what to do in this new environment.
One way to retrain a robot for a specific task is imitation learning. The user could demonstrate the correct task to teach the robot what to do. If a user tries to teach a robot to pick up a mug, but demonstrates with a white mug, the robot could learn that all mugs are white. It may then fail to pick up a red, blue, or “Tim-the-Beaver-brown” mug.
Training a robot to recognize that a mug is a mug, regardless of its color, could take thousands of demonstrations.
“I don’t want to have to demonstrate with 30,000 mugs. I want to demonstrate with just one mug. But then I need to teach the robot so it recognizes that it can pick up a mug of any color,” Peng says.
To accomplish this, the researchers’ system determines what specific object the user cares about (a mug) and what elements aren’t important for the task (perhaps the color of the mug doesn’t matter). It uses this information to generate new, synthetic data by changing these “unimportant” visual concepts. This process is known as data augmentation.
The framework has three steps. First, it shows the task that caused the robot to fail. Then it collects a demonstration from the user of the desired actions and generates counterfactuals by searching over all features in the space that show what needed to change for the robot to succeed.
The system shows these counterfactuals to the user and asks for feedback to determine which visual concepts do not impact the desired action. Then it uses this human feedback to generate many new augmented demonstrations.
In this way, the user could demonstrate picking up one mug, but the system would produce demonstrations showing the desired action with thousands of different mugs by altering the color. It uses these data to fine-tune the robot.
Creating counterfactual explanations and soliciting feedback from the user are critical for the technique to succeed, Peng says.
From human reasoning to robot reasoning
Because their work seeks to put the human in the training loop, the researchers tested their technique with human users. They first conducted a study in which they asked people if counterfactual explanations helped them identify elements that could be changed without affecting the task.
“It was so clear right off the bat. Humans are so good at this type of counterfactual reasoning. And this counterfactual step is what allows human reasoning to be translated into robot reasoning in a way that makes sense,” she says.
Then they applied their framework to three simulations where robots were tasked with: navigating to a goal object, picking up a key and unlocking a door, and picking up a desired object then placing it on a tabletop. In each instance, their method enabled the robot to learn faster than with other techniques, while requiring fewer demonstrations from users.
Moving forward, the researchers hope to test this framework on real robots. They also want to focus on reducing the time it takes the system to create new data using generative machine-learning models.
“We want robots to do what humans do, and we want them to do it in a semantically meaningful way. Humans tend to operate in this abstract space, where they don’t think about every single property in an image. At the end of the day, this is really about enabling a robot to learn a good, human-like representation at an abstract level,” Peng says.
This research is supported, in part, by a National Science Foundation Graduate Research Fellowship, Open Philanthropy, an Apple AI/ML Fellowship, Hyundai Motor Corporation, the MIT-IBM Watson AI Lab, and the National Science Foundation Institute for Artificial Intelligence and Fundamental Interactions.