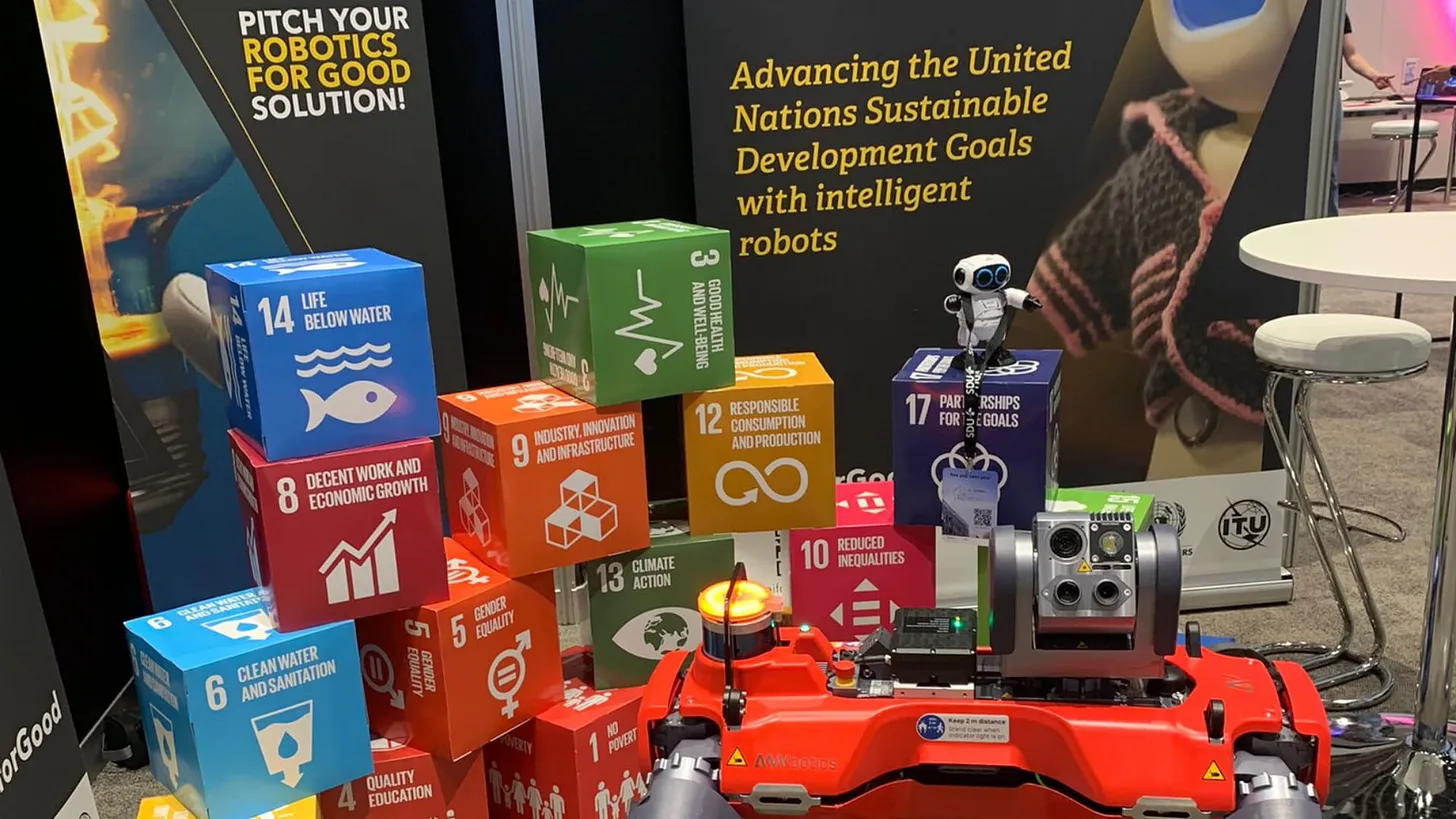
If you look at the UN Sustainable Development Goals, it’s clear that robots have a huge role to play in advancing the SDGs. However the field of Sustainable Robotics is more than just the application area. For every application that robotics can improve in sustainability, you have to also address the question – what are the additional costs or benefits all the way along the supply chain. What are the ‘externalities’, or additional costs/benefits, of using robots to solve the problem. Does the use of robotics bring a decrease or an increase to:
- power costs
- production costs
- labor costs
- supply chain costs
- supply chain mileage
- raw materials consumption
- and raw material choice
Solving our economic and environmental global challenges should not involve adding to the existing problems or creating new ones. So it’s important that we look beyond the first order ways in which robotics can solve global sustainable development goals and address every level at which robotics can have an impact.
Here I propose 5 levels of sustainability to frame the discussion, much as the 5 levels of autonomy have helped define the stages of autonomous mobility.
Level 1: Robots for existing recycling
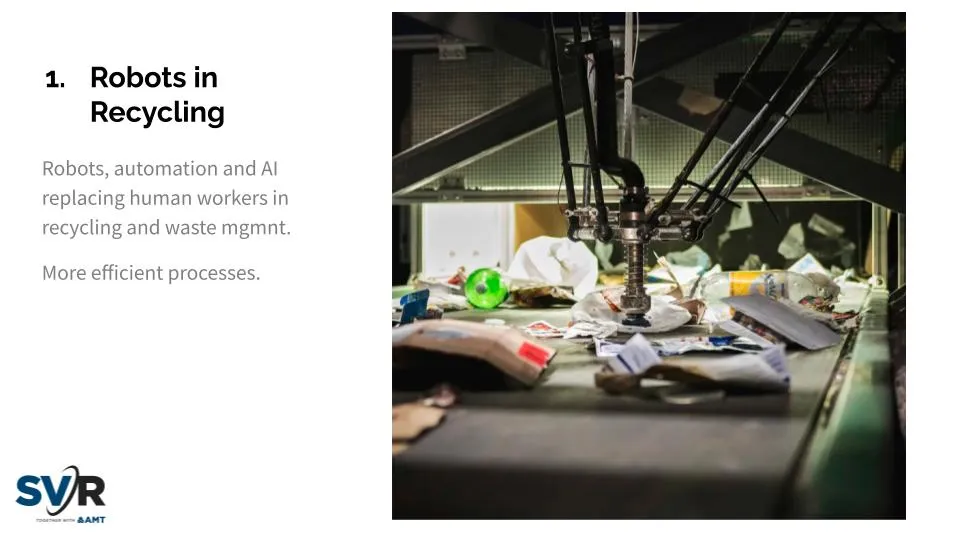
Level 1 of Sustainable Robotics is simply making existing processes in sustainability more efficient, affordable and deployable. Making recycling better. Companies that are great examples are: AMP Robotics, Recycleye, MachineEx, Pellenc ST, Greyparrot, Everlast Labs and Fanuc. Here’s an explainer video from Fanuc.
“Because of AI, because of the robotic arms, we have seen plants recover 10, 20, 30% more than what they have been doing previously,” said JD Ambati, CEO of EverestLabs. “They have been losing millions of dollars to the landfill, and because of AI, they were able to identify the value of the losses and deploy robotic arms to capture that.”
Some other examples of Level 1 use robots to better monitor aquaculture, or robots to clean or install solar farms and wind turbines. If the robotics technology improves existing recycling practices then it is at Level 1 of Sustainable Robotics.
Level 2: Robots enabling new recycling
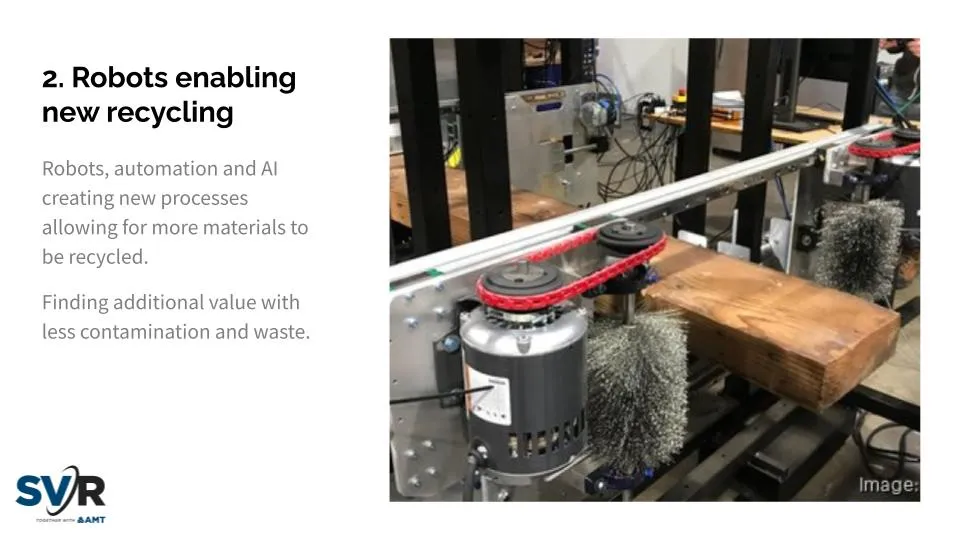
Level 2 of Sustainable Robotics is where robotics allows new materials to be recycled and in new industry application areas. A great example of this is Urban Machines, which salvages timber from construction sites and transforms it back into useable materials, something that was too difficult to do at any scale previously.
Construction using onsite materials and robotics 3D printing is another example, as seen in the NASA Habitat Challenge, sponsored by Caterpillar, Bechtel and Brick & Mortar Ventures.
Some other examples are the ocean or lake going garbage collecting robots like Waste Shark from Ran Marine, River Cleaning, or Searial Cleaners, a Quebec company whose robots were deployed in the Great Lakes Plastic Cleanup, helping to remove 74,000 plastic pieces from four lakes since 2020.
Searial Cleaners is angling for its BeBot and PixieDrone to be used as janitorial tools for beaches, marinas and golf courses, and the BeBot offers ample room for company branding. The equipment emerged from the mission of the Great Lakes Plastic Cleanup (GLPC) to harness new technologies against litter. The program also uses other devices including the Seabin, which sits in water and sucks in trash, and the Enviropod LittaTrap filter for stormwater drains.
If it’s a brand new way to practice recycling with robotic technology, then it’s at Level 2 of Sustainable Robotics.
Level 3: Robots electrifying everything
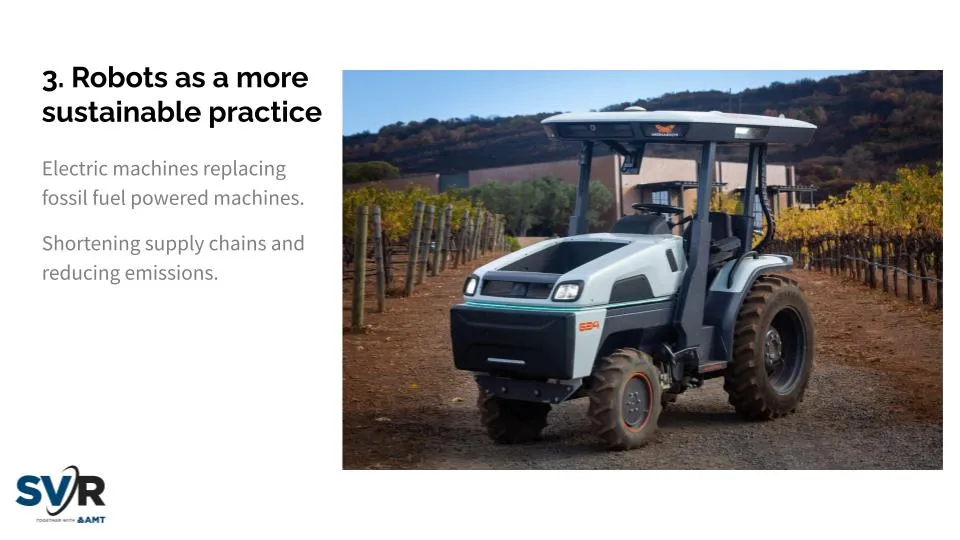
One of the biggest sustainability shifts enabled by robotics is the transition from fossil fuel powered transport, logistics and agricultural machinery into BEV, or Battery Electric Vehicle technology. On top of radically reducing emissions, the increasing use of smaller autonomous electric vehicles across first, last and middle mile can change the total number of trips taken, as well as reducing the need for large vehicles that are partially loaded taking longer trips.
Monarch Tractor’s MK-V is the world’s first electric tractor, and is ‘driver optional’, meaning it can be driven or operate autonomously, providing greater flexibility for farmers. Of course the increased use of computer vision and AI across all agrobots increase sustainability, by enabling precision or regenerative agriculture with less need for chemical solutions. Technically, these improvements to agricultural practice are Level 2 of Sustainable Robotics.
However, the use of smaller sized fully autonomous agricultural robots, such as Meropy, Burro.ai, SwarmFarm, Muddy Machines and Small Robot Company also reduces the size and soil compaction associated with agricultural machinery, and make it possible to tend smaller strip farms by machine. This is Level 3 of Sustainable Robotics.
Level 4: Robots
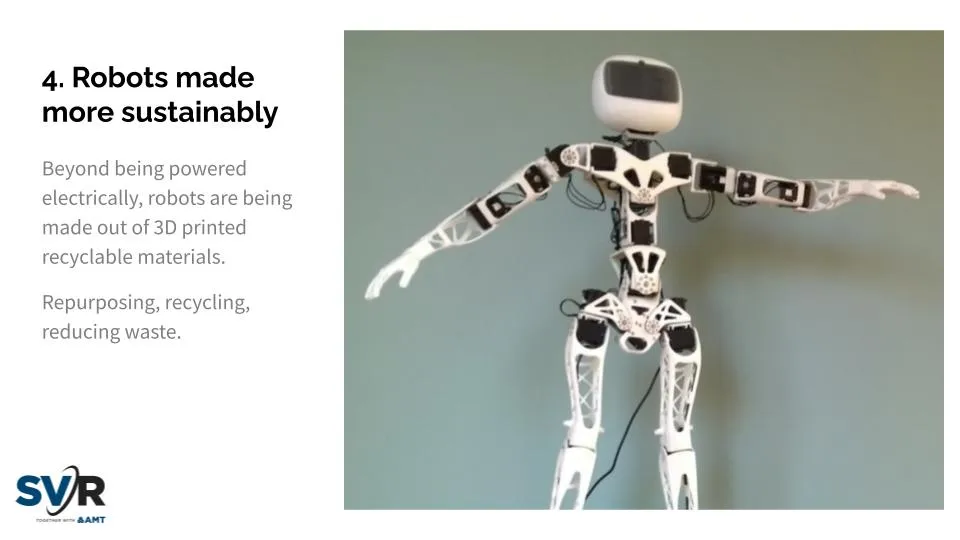
The higher the sustainability level, the deeper it is into the actual design and construction of the robot system. Switching to electric from fossil fuels is a small step. Switching to locally sourced or produced materials is another. Switching to recyclable materials is another step towards fully sustainable robotics.
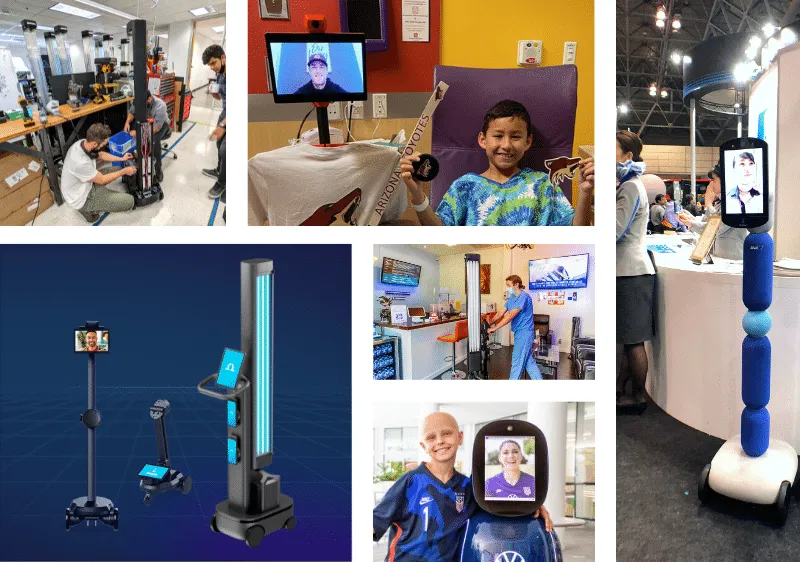
OhmniLabs utilize 3D printing in their robot construction, allowing them to export robots to 47 countries, while also manufacturing locally in Silicon Valley.
Meanwhile, Cornell researchers Wendy Ju and Ilan Mandel have introduced the phrase ‘Garbatrage’ to describe the opportunity to prototype or build robots using components recycled from other consumer electronics, like these hoverboards.
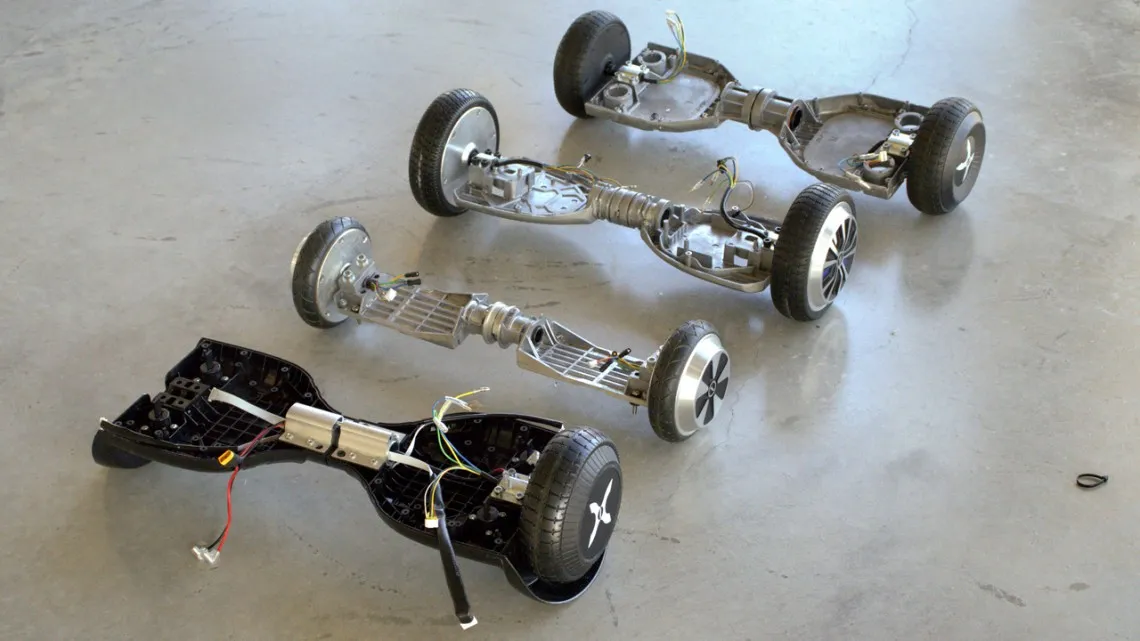
“The time is ripe for a practice like garbatrage, both for sustainability reasons and considering the global supply shortages and international trade issues of the last few years,” the researchers said.
This is a great example of Level 4 of Sustainable Robotics.
Level 5: Self-powering/repairing Robots
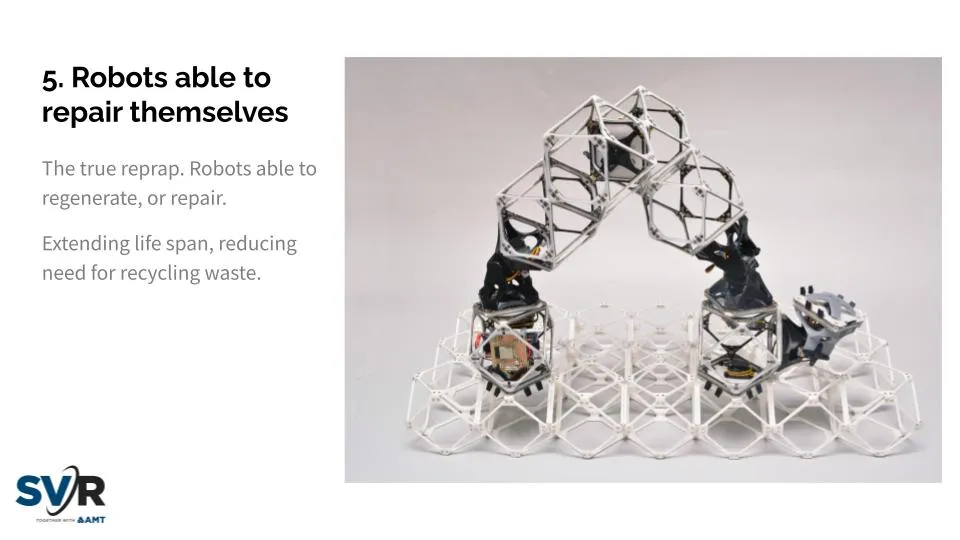
Self powering or self repairing or self recycling robots are the Level 5 of Sustainable Robotics. In research, there are solutions like MilliMobile: A battery-free autonomous robot capable of operating on harvested solar and RF power. MilliMobile, developed at the Paul G. Allen School of Computer Science & Engineering, is the size of a penny and can steer itself, sense its environment, and communicate wirelessly using energy harvested from light and radio waves.
It’s not just research though. In the last two years, a number of solar powered agricultural robots have entered the market. Solinftec has a solar powered spray robot, as has EcoRobotix and AIGEN, which is also powered by wind.
Modular robotics will reduce our material wastage and energy needs by making robotics multipurpose, rather than requiring multiple specialist robots. Meanwhile self powering and self repairing technologies will allow robots to enter many previously unreachable areas, including off planet, while removing our reliance on the grid. As robots incorporate self repairing materials, the product lifecycle is increased. This is Level 5 of Sustainable Robotics.
And in the future?
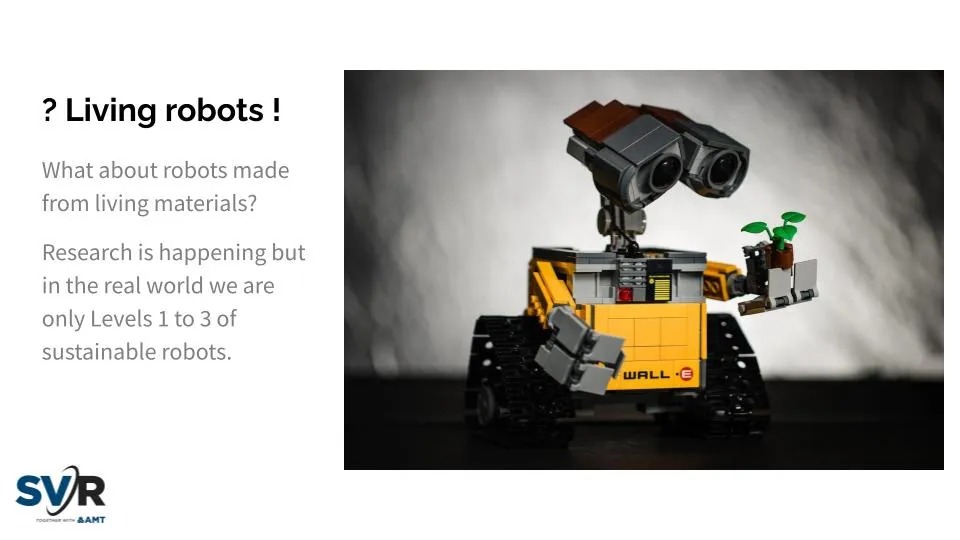
While we’re waiting for the future, here are a couple of resources for turning your entire company into a sustainable robotics company. Sustainable Manufacturing 101 from ITA, the International Trade Administration and the Sustainable Manufacturing Toolkit from the OECD.
References
- https://www.cnbc.com/2023/08/08/everestlabs-using-robotic-arms-and-ai-to-make-recycling-more-efficient.html
- https://www.greenbiz.com/article/great-lakes-are-awash-plastic-can-robots-and-drones-help
- https://www.economist.com/science-and-technology/2020/02/06/using-artificial-intelligence-agricultural-robots-are-on-the-rise
- https://www.wired.co.uk/article/farming-robots-small-robot-company-tractors
- https://news.cornell.edu/stories/2023/09/garbatrage-spins-e-waste-prototyping-gold