The flying, swimming and tunneling robots inspired by nature
Autonomous excavator constructs a 6-meter-high dry-stone wall
A new optimization framework for robot motion planning
Terabase Energy Completes First Commercial Deployment of Terafab™ Solar Construction Automation System
A wearable robot that assists people with walking
3D Image Processing Solution With 2D Cameras, AI and Robotics Optimizes the Manufacturing Landscape
Toyota Research Institute Showcases Latest AI-Assisted Driving Technology
This 3D printer can watch itself fabricate objects
Interview with Dautzenberg Roman: #IROS2023 Best Paper Award on Mobile Manipulation sponsored by OMRON Sinic X Corp.
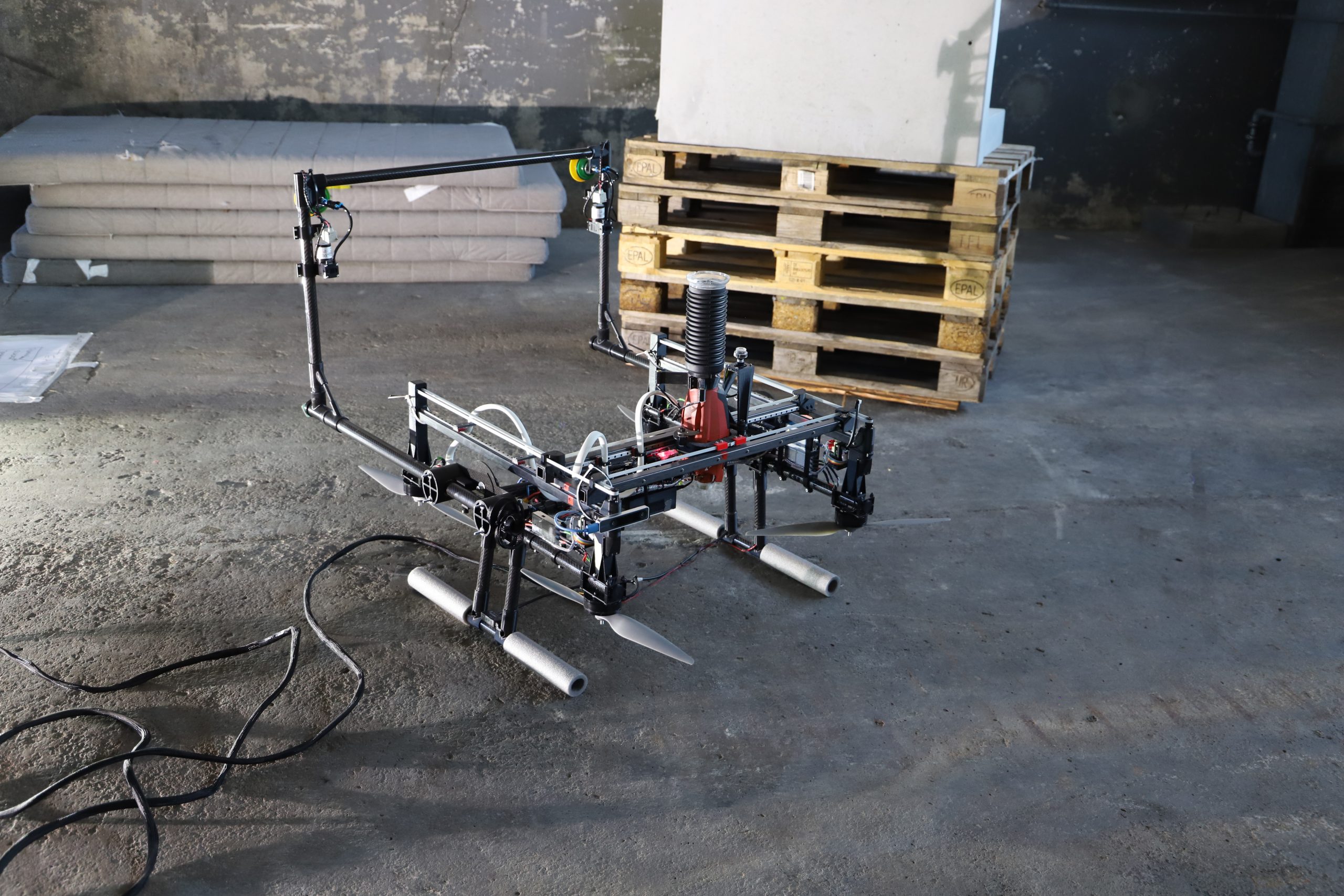
Congratulations to Dautzenberg Roman and his team of researchers, who won the IROS 2023 Best Paper Award on Mobile Manipulation sponsored by OMRON Sinic X Corp. for their paper “A perching and tilting aerial robot for precise and versatile power tool work on vertical walls“. Below, the authors tell us more about their work, the methodology, and what they are planning next.
What is the topic of the research in your paper?
Our paper shows a an aerial robot (think “drone”) which can exert large forces in the horizontal direction, i.e. onto walls. This is a difficult task, as UAVs usually rely on thrust vectoring to apply horizontal forces and thus can only apply small forces before losing control authority. By perching onto walls, our system no longer needs the propulsion to remain at a desired site. Instead we use the propellers to achieve large reaction forces in any direction, also onto walls! Additionally, perching allows extreme precision, as the tool can be moved and re-adjusted, as well as being unaffected by external disturbances such as gusts of wind.
Could you tell us about the implications of your research and why it is an interesting area for study?
Precision, force exertion and mobility are the three (of many) criteria where robots – and those that develop them – make trade-offs. Our research shows that the system we designed can exert large forces precisely with only minimal compromises on mobility. This widens the horizon of conceivable tasks for aerial robots, as well as serving as the next link in automating the chain of tasks need to perform many procedures on construction sites, or on remote, complex or hazardous environments.
Could you explain your methodology?
The main aim of our paper is to characterize the behavior and performance of the system, and comparing the system to other aerial robots. To achieve this, we investigated the perching and tool positioning accuracy, as well as comparing the applicable reaction forces with other systems.
Further, the paper shows the power consumption and rotational velocities of the propellers for the various phases of a typical operation, as well as how certain mechanism of the aerial robot are configured. This allows for a deeper understanding of the characteristics of the aerial robot.
What were your main findings?
Most notably, we show the perching precision to be within +-10cm of a desired location over 30 consecutive attempts and tool positioning to have mm-level accuracy even in a “worst-case” scenario. Power consumption while perching on typical concrete is extremely low and the system is capable of performing various tasks (drilling, screwing) also in quasi-realistic, outdoor scenarios.
What further work are you planning in this area?
Going forward, enhancing the capabilities will be a priority. This relates both to the types of surface manipulations that can be performed, but also the surfaces onto which the system can perch.
About the author
![]() |
Dautzenberg Roman is currently a Masters student at ETH Zürich and Team Leader at AITHON. AITHON is a research project which is transforming into a start-up for aerial construction robotics. They are a core team of 8 engineers, working under the guidance of the Autonomous Systems Lab at ETH Zürich and located at the Innovation Park Switzerland in Dübendorf. |
An approach to plan the actions of robot teams in uncertain conditions
Robot Talk Episode 62 – Jorvon Moss
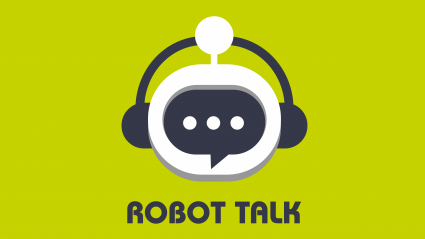
Claire chatted to Jorvon (Odd-Jayy) Moss from Digikey about making robots at home, and robot design and aesthetics.
Commonly known as Odd-Jayy, Jorvon Moss is an accomplished Maker best known for his Robotic Oddities. Jayy’s art background, BFA in Illustration, and self-taught electronics skills have combined to help launch his career and promote the wonderful world of STEAM (Science, Technology, Engineering, Art, Math). This achievement, and the many viral videos under his belt, gained him recognition from major forces in the industry; including Digi-Key Electronics, Tested Inc. with Adam Savage, various electronic and tech Faires, and as the first Black person in Make Magazine.