Control system enables four-legged robots to jump across uneven terrain in real time
How to Select the Best Motor for a Jointed Arm Robot
Robotics Today latest talks – Raia Hadsell (DeepMind), Koushil Sreenath (UC Berkeley) and Antonio Bicchi (Istituto Italiano di Tecnologia)
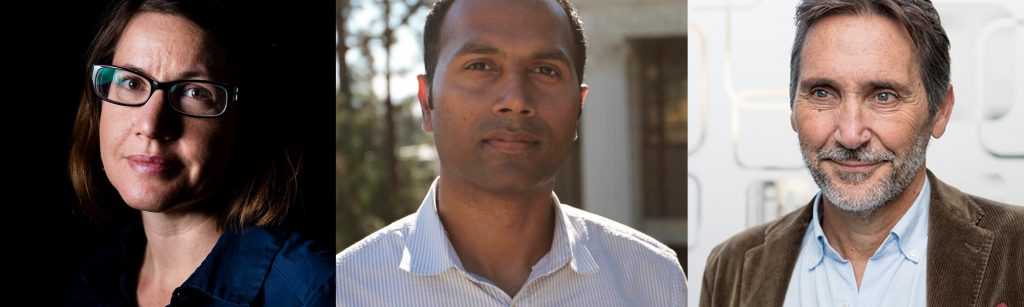
Robotics Today held three more online talks since we published the one from Amanda Prorok (Learning to Communicate in Multi-Agent Systems). In this post we bring you the last talks that Robotics Today (currently on hiatus) uploaded to their YouTube channel: Raia Hadsell from DeepMind talking about ‘Scalable Robot Learning in Rich Environments’, Koushil Sreenath from UC Berkeley talking about ‘Safety-Critical Control for Dynamic Robots’, and Antonio Bicchi from the Istituto Italiano di Tecnologia talking about ‘Planning and Learning Interaction with Variable Impedance’.
![]() |
Raia Hadsell (DeepMind) – Scalable Robot Learning in Rich Environments Abstract: As modern machine learning methods push towards breakthroughs in controlling physical systems, games and simple physical simulations are often used as the main benchmark domains. As the field matures, it is important to develop more sophisticated learning systems with the aim of solving more complex real-world tasks, but problems like catastrophic forgetting and data efficiency remain critical, particularly for robotic domains. This talk will cover some of the challenges that exist for learning from interactions in more complex, constrained, and real-world settings, and some promising new approaches that have emerged. Bio: Raia Hadsell is the Director of Robotics at DeepMind. Dr. Hadsell joined DeepMind in 2014 to pursue new solutions for artificial general intelligence. Her research focuses on the challenge of continual learning for AI agents and robots, and she has proposed neural approaches such as policy distillation, progressive nets, and elastic weight consolidation to solve the problem of catastrophic forgetting. Dr. Hadsell is on the executive boards of ICLR (International Conference on Learning Representations), WiML (Women in Machine Learning), and CoRL (Conference on Robot Learning). She is a fellow of the European Lab on Learning Systems (ELLIS), a founding organizer of NAISys (Neuroscience for AI Systems), and serves as a CIFAR advisor. |
![]() |
Koushil Sreenath (UC Berkeley) – Safety-Critical Control for Dynamic Robots: A Model-based and Data-driven Approach Abstract: Model-based controllers can be designed to provide guarantees on stability and safety for dynamical systems. In this talk, I will show how we can address the challenges of stability through control Lyapunov functions (CLFs), input and state constraints through CLF-based quadratic programs, and safety-critical constraints through control barrier functions (CBFs). However, the performance of model-based controllers is dependent on having a precise model of the system. Model uncertainty could lead not only to poor performance but could also destabilize the system as well as violate safety constraints. I will present recent results on using model-based control along with data-driven methods to address stability and safety for systems with uncertain dynamics. In particular, I will show how reinforcement learning as well as Gaussian process regression can be used along with CLF and CBF-based control to address the adverse effects of model uncertainty. Bio: Koushil Sreenath is an Assistant Professor of Mechanical Engineering, at UC Berkeley. He received a Ph.D. degree in Electrical Engineering and Computer Science and a M.S. degree in Applied Mathematics from the University of Michigan at Ann Arbor, MI, in 2011. He was a Postdoctoral Scholar at the GRASP Lab at University of Pennsylvania from 2011 to 2013 and an Assistant Professor at Carnegie Mellon University from 2013 to 2017. His research interest lies at the intersection of highly dynamic robotics and applied nonlinear control. His work on dynamic legged locomotion was featured on The Discovery Channel, CNN, ESPN, FOX, and CBS. His work on dynamic aerial manipulation was featured on the IEEE Spectrum, New Scientist, and Huffington Post. His work on adaptive sampling with mobile sensor networks was published as a book. He received the NSF CAREER, Hellman Fellow, Best Paper Award at the Robotics: Science and Systems (RSS), and the Google Faculty Research Award in Robotics. |
![]() |
Antonio Bicchi (Istituto Italiano di Tecnologia) – Planning and Learning Interaction with Variable Impedance Abstract: In animals and in humans, the mechanical impedance of their limbs changes not only in dependence of the task, but also during different phases of the execution of a task. Part of this variability is intentionally controlled, by either co-activating muscles or by changing the arm posture, or both. In robots, impedance can be varied by varying controller gains, stiffness of hardware parts, and arm postures. The choice of impedance profiles to be applied can be planned off-line, or varied in real time based on feedback from the environmental interaction. Planning and control of variable impedance can use insight from human observations, from mathematical optimization methods, or from learning. In this talk I will review the basics of human and robot variable impedance, and discuss how this impact applications ranging from industrial and service robotics to prosthetics and rehabilitation. Bio: Antonio Bicchi is a scientist interested in robotics and intelligent machines. After graduating in Pisa and receiving a Ph.D. from the University of Bologna, he spent a few years at the MIT AI Lab of Cambridge before becoming Professor in Robotics at the University of Pisa. In 2009 he founded the Soft Robotics Laboratory at the Italian Institute of Technology in Genoa. Since 2013 he is Adjunct Professor at Arizona State University, Tempe, AZ. He has coordinated many international projects, including four grants from the European Research Council (ERC). He served the research community in several ways, including by launching the WorldHaptics conference and the IEEE Robotics and Automation Letters. He is currently the President of the Italian Institute of Robotics and Intelligent Machines. He has authored over 500 scientific papers cited more than 25,000 times. He supervised over 60 doctoral students and more than 20 postdocs, most of whom are now professors in universities and international research centers, or have launched their own spin-off companies. His students have received prestigious awards, including three first prizes and two nominations for the best theses in Europe on robotics and haptics. He is a Fellow of IEEE since 2005. In 2018 he received the prestigious IEEE Saridis Leadership Award. |
Transformers in computer vision: ViT architectures, tips, tricks and improvements
Raspberry Pi announces Build HAT—an add-on device that uses Pi hardware to control LEGO Technic motors
A system to transfer robotic dexterous manipulation skills from simulations to real robots
Artificial Intelligence, Robotics & Ethics
An online method to allocate tasks to robots on a team during natural disaster scenarios
Robot-Ready Conveying Optimizes Pick-and-Place
Sense Think Act Pocast: Erik Schluntz
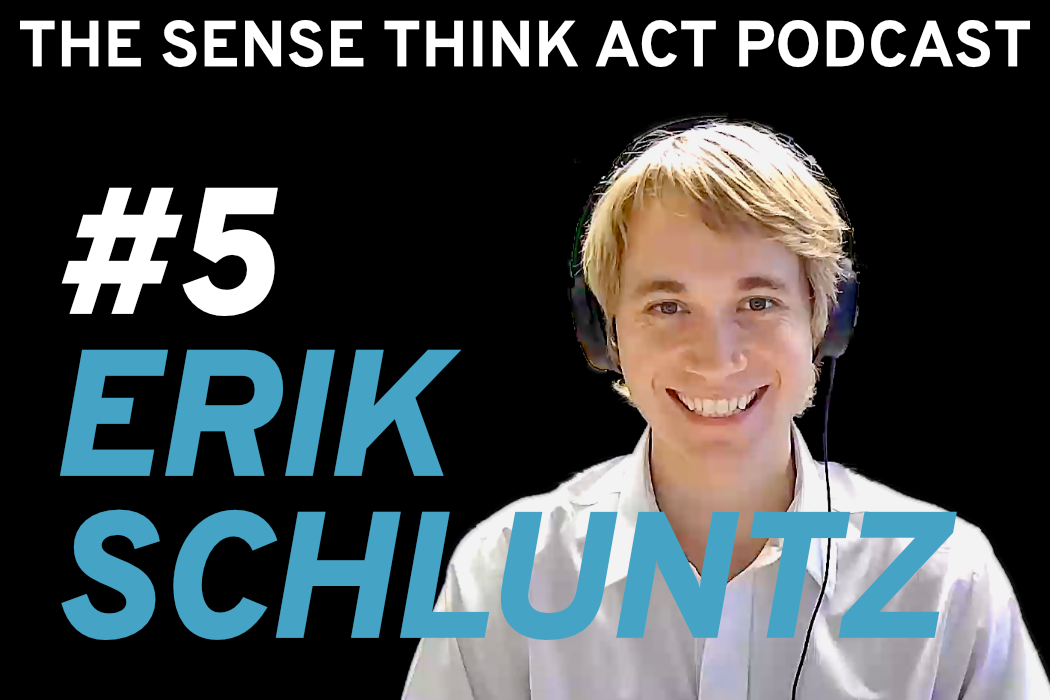
In this episode, Audrow Nash interviews Erik Schluntz, co-founder and CTO of Cobalt Robotics, which makes a security guard robot. Erik speaks about how their robot handles elevators, how they have humans-in-the-loop to help their robot make decisions, robot body language, and gives advice for entrepreneurs.
Episode Links
- Cobalt’s website
- Erik’s website
- Video introducing the Cobalt robot
- Video of the Cobalt robot using an elevator
Podcast info
Researchers successfully build four-legged swarm robots
Smart Robots ready to build a faster, cleaner, more profitable construction industry
New technology can make manual labor 60 percent easier
A robot that finds lost items
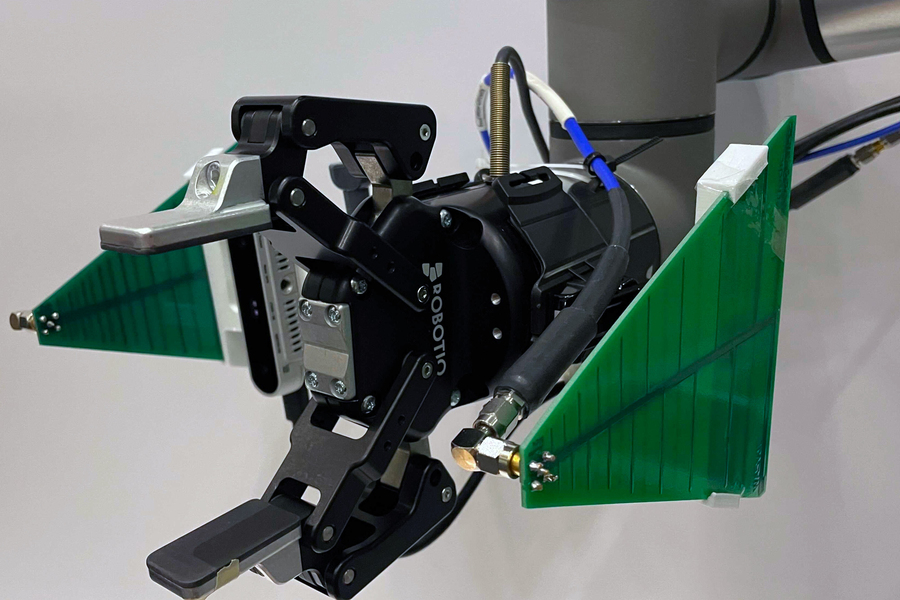
Researchers at MIT have developed a fully-integrated robotic arm that fuses visual data from a camera and radio frequency (RF) information from an antenna to find and retrieve objects, even when they are buried under a pile and fully out of view. Credits: Courtesy of the researchers
By Adam Zewe | MIT News Office
A busy commuter is ready to walk out the door, only to realize they’ve misplaced their keys and must search through piles of stuff to find them. Rapidly sifting through clutter, they wish they could figure out which pile was hiding the keys.
Researchers at MIT have created a robotic system that can do just that. The system, RFusion, is a robotic arm with a camera and radio frequency (RF) antenna attached to its gripper. It fuses signals from the antenna with visual input from the camera to locate and retrieve an item, even if the item is buried under a pile and completely out of view.
The RFusion prototype the researchers developed relies on RFID tags, which are cheap, battery-less tags that can be stuck to an item and reflect signals sent by an antenna. Because RF signals can travel through most surfaces (like the mound of dirty laundry that may be obscuring the keys), RFusion is able to locate a tagged item within a pile.
Using machine learning, the robotic arm automatically zeroes-in on the object’s exact location, moves the items on top of it, grasps the object, and verifies that it picked up the right thing. The camera, antenna, robotic arm, and AI are fully integrated, so RFusion can work in any environment without requiring a special set up.
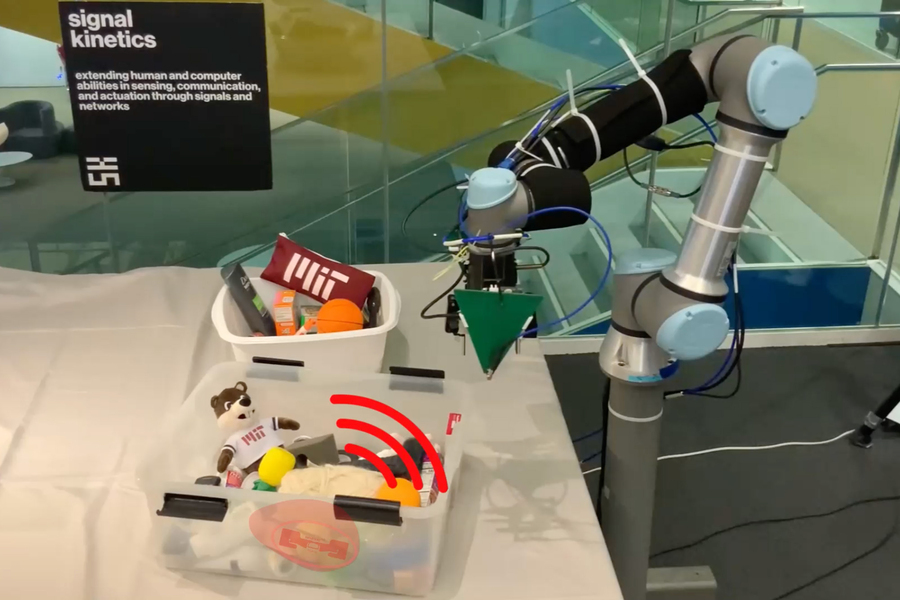
In this video still, the robotic arm is looking for keys hidden underneath items. Credits: Courtesy of the researchers
While finding lost keys is helpful, RFusion could have many broader applications in the future, like sorting through piles to fulfill orders in a warehouse, identifying and installing components in an auto manufacturing plant, or helping an elderly individual perform daily tasks in the home, though the current prototype isn’t quite fast enough yet for these uses.
“This idea of being able to find items in a chaotic world is an open problem that we’ve been working on for a few years. Having robots that are able to search for things under a pile is a growing need in industry today. Right now, you can think of this as a Roomba on steroids, but in the near term, this could have a lot of applications in manufacturing and warehouse environments,” said senior author Fadel Adib, associate professor in the Department of Electrical Engineering and Computer Science and director of the Signal Kinetics group in the MIT Media Lab.
Co-authors include research assistant Tara Boroushaki, the lead author; electrical engineering and computer science graduate student Isaac Perper; research associate Mergen Nachin; and Alberto Rodriguez, the Class of 1957 Associate Professor in the Department of Mechanical Engineering. The research will be presented at the Association for Computing Machinery Conference on Embedded Networked Senor Systems next month.
Sending signals
RFusion begins searching for an object using its antenna, which bounces signals off the RFID tag (like sunlight being reflected off a mirror) to identify a spherical area in which the tag is located. It combines that sphere with the camera input, which narrows down the object’s location. For instance, the item can’t be located on an area of a table that is empty.
But once the robot has a general idea of where the item is, it would need to swing its arm widely around the room taking additional measurements to come up with the exact location, which is slow and inefficient.
The researchers used reinforcement learning to train a neural network that can optimize the robot’s trajectory to the object. In reinforcement learning, the algorithm is trained through trial and error with a reward system.
“This is also how our brain learns. We get rewarded from our teachers, from our parents, from a computer game, etc. The same thing happens in reinforcement learning. We let the agent make mistakes or do something right and then we punish or reward the network. This is how the network learns something that is really hard for it to model,” Boroushaki explains.
In the case of RFusion, the optimization algorithm was rewarded when it limited the number of moves it had to make to localize the item and the distance it had to travel to pick it up.
Once the system identifies the exact right spot, the neural network uses combined RF and visual information to predict how the robotic arm should grasp the object, including the angle of the hand and the width of the gripper, and whether it must remove other items first. It also scans the item’s tag one last time to make sure it picked up the right object.
Cutting through clutter
The researchers tested RFusion in several different environments. They buried a keychain in a box full of clutter and hid a remote control under a pile of items on a couch.
But if they fed all the camera data and RF measurements to the reinforcement learning algorithm, it would have overwhelmed the system. So, drawing on the method a GPS uses to consolidate data from satellites, they summarized the RF measurements and limited the visual data to the area right in front of the robot.
Their approach worked well — RFusion had a 96 percent success rate when retrieving objects that were fully hidden under a pile.
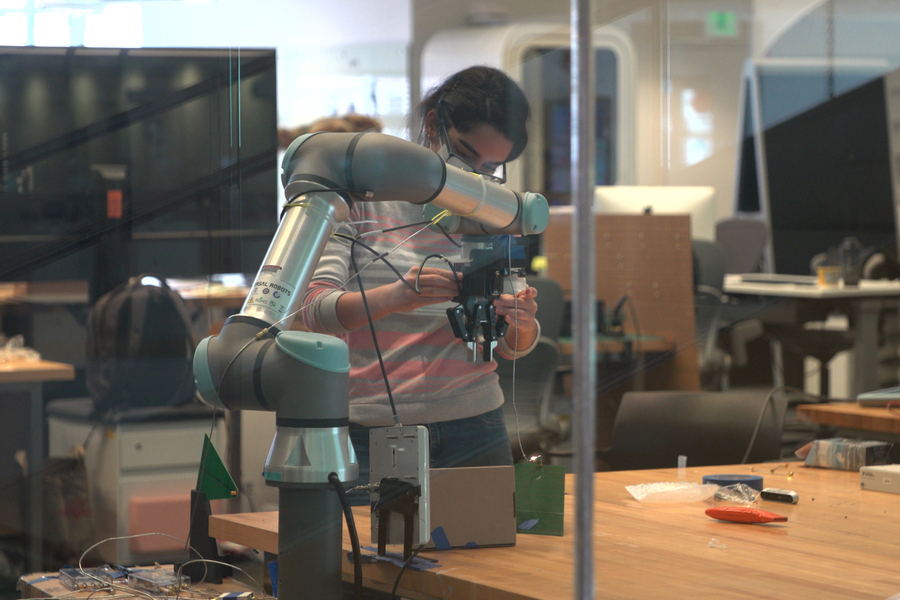
“We let the agent make mistakes or do something right and then we punish or reward the network. This is how the network learns something that is really hard for it to model,” co-author Tara Boroushaki, pictured here, explains. Credits: Courtesy of the researchers
“Sometimes, if you only rely on RF measurements, there is going to be an outlier, and if you rely only on vision, there is sometimes going to be a mistake from the camera. But if you combine them, they are going to correct each other. That is what made the system so robust,” Boroushaki says.
In the future, the researchers hope to increase the speed of the system so it can move smoothly, rather than stopping periodically to take measurements. This would enable RFusion to be deployed in a fast-paced manufacturing or warehouse setting.
Beyond its potential industrial uses, a system like this could even be incorporated into future smart homes to assist people with any number of household tasks, Boroushaki says.
“Every year, billions of RFID tags are used to identify objects in today’s complex supply chains, including clothing and lots of other consumer goods. The RFusion approach points the way to autonomous robots that can dig through a pile of mixed items and sort them out using the data stored in the RFID tags, much more efficiently than having to inspect each item individually, especially when the items look similar to a computer vision system,” says Matthew S. Reynolds, CoMotion Presidential Innovation Fellow and associate professor of electrical and computer engineering at the University of Washington, who was not involved in the research. “The RFusion approach is a great step forward for robotics operating in complex supply chains where identifying and ‘picking’ the right item quickly and accurately is the key to getting orders fulfilled on time and keeping demanding customers happy.”
The research is sponsored by the National Science Foundation, a Sloan Research Fellowship, NTT DATA, Toppan, Toppan Forms, and the Abdul Latif Jameel Water and Food Systems Lab.