Vision Guided Robotics & Artificial Intelligence: An Explanation for the Non-Technical
#336: Mobile Outdoor Manipulation with RE2 Robotics, with Travis Schneider
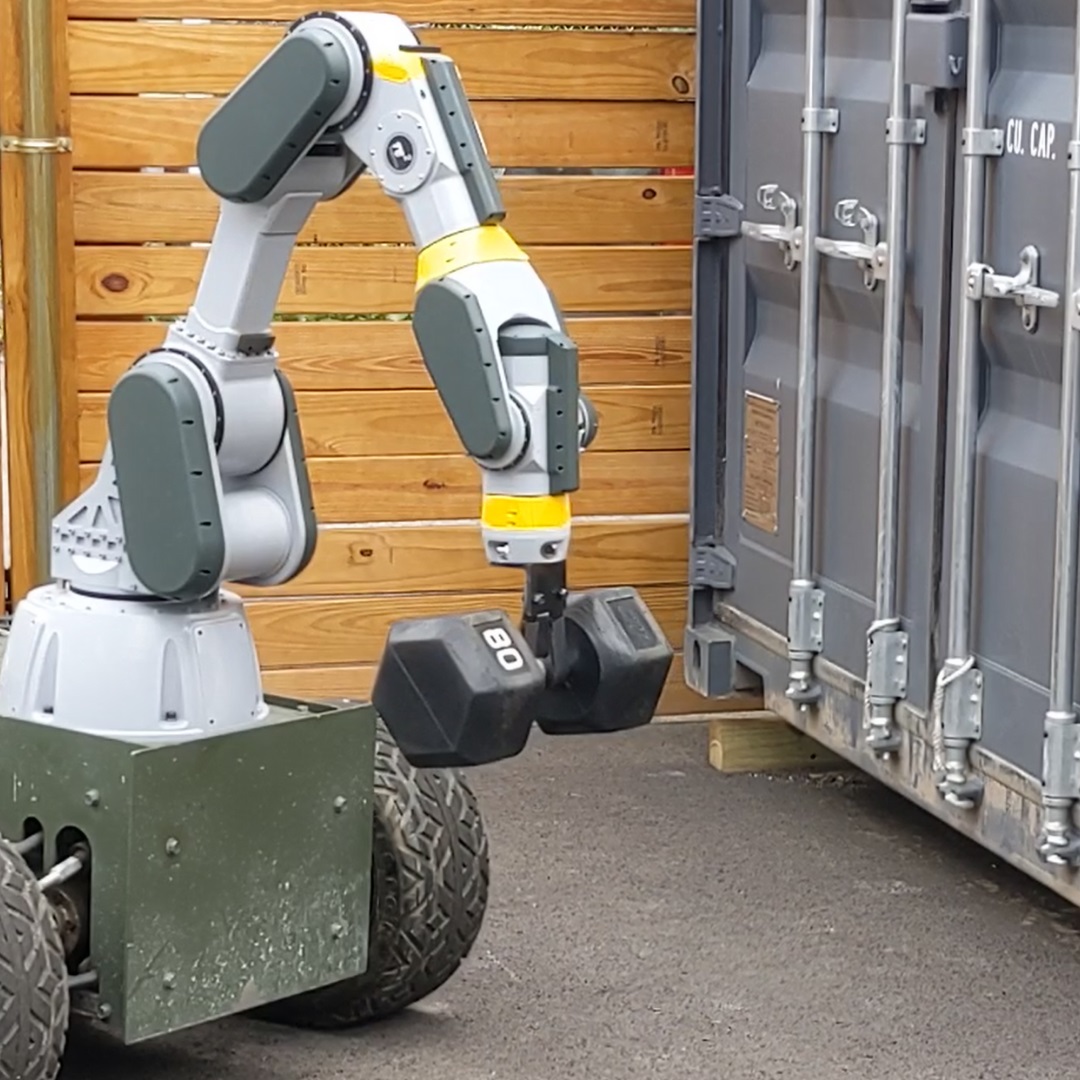
In this episode, Shihan Lu interviews Travis Schneider, Business Development Manager at RE2 Robotics, focusing on their work on mobile outdoor manipulation. Travis introduces several robotic products and services of RE2, including RE2 Sapien robotic arms and human-centered system integration, and how they can be used to help rather than replace workers. He also shares his thoughts about challenges in human-robot collaboration.
Travis Schneider
Travis Schneider, Business Development Manager at RE2 Robotics (RE2), works to foster commercial B2B partnerships with groups interested in leveraging RE2’s robotic technology for applications in a wide variety of markets, including aviation, construction, energy, and medical. With a background in Mechanical Engineering, Travis has an intimate knowledge of many of the fundamentals associated with robotic systems including electromechanical design, motion control, and associated software.
Links
- RE2 Robotics
- Download mp3 (12.7 MB)
- Subscribe to Robohub using iTunes, RSS, or Spotify
- Support us on Patreon
Access Control Key to Cybersecurity in B2B eCommerce
#IROS2020 Plenary and Keynote talks focus series #2: Frank Dellaert & Ashish Deshpande
Last Wednesday we started this series of posts showcasing the plenary and keynote talks from the IEEE/RSJ IROS2020 (International Conference on Intelligent Robots and Systems). This is a great opportunity to stay up to date with the latest robotics & AI research from top roboticists in the world. This week we’re bringing you Prof. Frank Dellaert (Georgia Institute of Technology; Google AI) and Prof. Ashish Deshpande (The University of Texas).
![]() |
Prof. Frank Dellaert – Perception in Aerial, Marine & Space Robotics: a Biased Outlook Bio: Frank Dellaert is a Professor in the School of Interactive Computing at the Georgia Institute of Technology and a Research Scientist at Google AI. While on leave from Georgia Tech in 2016-2018, he served as Technical Project Lead at Facebook’s Building 8 hardware division. Before that he was also Chief Scientist at Skydio, a startup founded by MIT grads to create intuitive interfaces for micro-aerial vehicles. His research is in the overlap between robotics and computer vision, and he is particularly interested in graphical model techniques to solve large-scale problems in mapping, 3D reconstruction, and increasingly model-predictive control. The GTSAM toolbox embodies many of the ideas his research group has worked on in the past few years and is available at https://gtsam.org. |
![]() |
Prof. Ashish Deshpande – Harmony Exoskeleton: A Journey from Robotics Lab to Stroke Bio: Ashish D. Deshpande is passionate about helping stroke patients recover from their disabilities and he believes robots could serve as important tools in the recovery process. He is a faculty member in Mechanical Engineering at The University of Texas at Austin, where he directs the ReNeu Robotics Lab. His work focuses on the study of human system and design of robotic systems toward the goals accelerating recovery after a neurological injury (e.g. stroke and spinal cord injury), improving the quality of lives of those living disabilities (e.g. amputation) and enhancing lives and productivity of workers, soldiers and astronauts. Specifically, his group has designed two novel exoskeletons for delivering engaging and subject-specific training for neuro-recovery of upper-body movements after stroke and spinal cord injury. Dr. Deshpande is a co-founder of Harmonic Bionics whose mission is to improve rehabilitation outcomes for the stroke patients. |
Increasing Warehouse Efficiency with Robotics
A robotic cat can teach us how real animals move
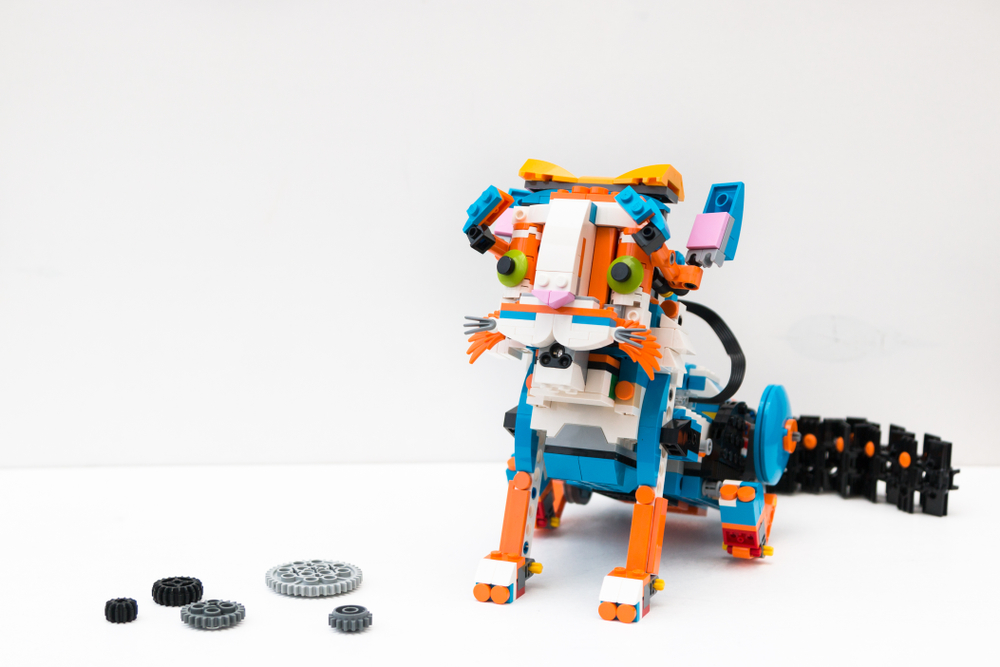
By Mischa Dijkstra, Frontiers science writer / Toyoaki Tanikawa, Department of Mechanical Engineering, Osaka University
In the young discipline of robotics-inspired biology, robots replace experimental animals, allowing researchers to learn about animals under a wider range of conditions than exist in nature or the laboratory.
What is the secret behind the steady but oh-so-elegant way in which cats move? That’s the subject of a study in Frontiers in Neurorobotics by scientists from Osaka University, who built a novel, 47cm-long and 7.6kg-heavy robotic cat. Based on previous research on the gait of real domestic cats, the authors deduced that key to the cats’ sleek movement must lie in a previously unknown reflex circuit, which they call the “reciprocal excitatory circuit between hip and knee extensors”.
According to their hypothesis, this reflex circuit has two essential features. The first is the mechanical unloading of a cat’s ankle extensor muscles, which prompts the transition from the stance to the swing phase. The second is muscle movement within the hip joint, which initiates the next transition, from swing to stance.
Trial-and-error with their robotic cat showed that their hypothesis was correct: even without a central pattern generator, a key feature of most moving robots, the novel reflex circuit gave a steady gait to the ‘robocat’, mimicking the movement of real cats.
The study’s first author, Toyoaki Tanikawa, is a young roboticist from Japan. After graduating from the advanced course in industrial and systems engineering at the National Institute of Technology-Kagawa College in early 2019, Tanikawa entered the MSc program at Osaka University’s Department of Mechanical Engineering, where he has done research on walking robots for the past two years.
Here he explains what first brought him to the field of robotics, how he and his supervisors assistant professor Masuda Youichi and Prof Ishikawa Masato developed the new ‘robocat’, and how mutual inspiration and exchange of knowledge between robotics and biology can benefit both fields.
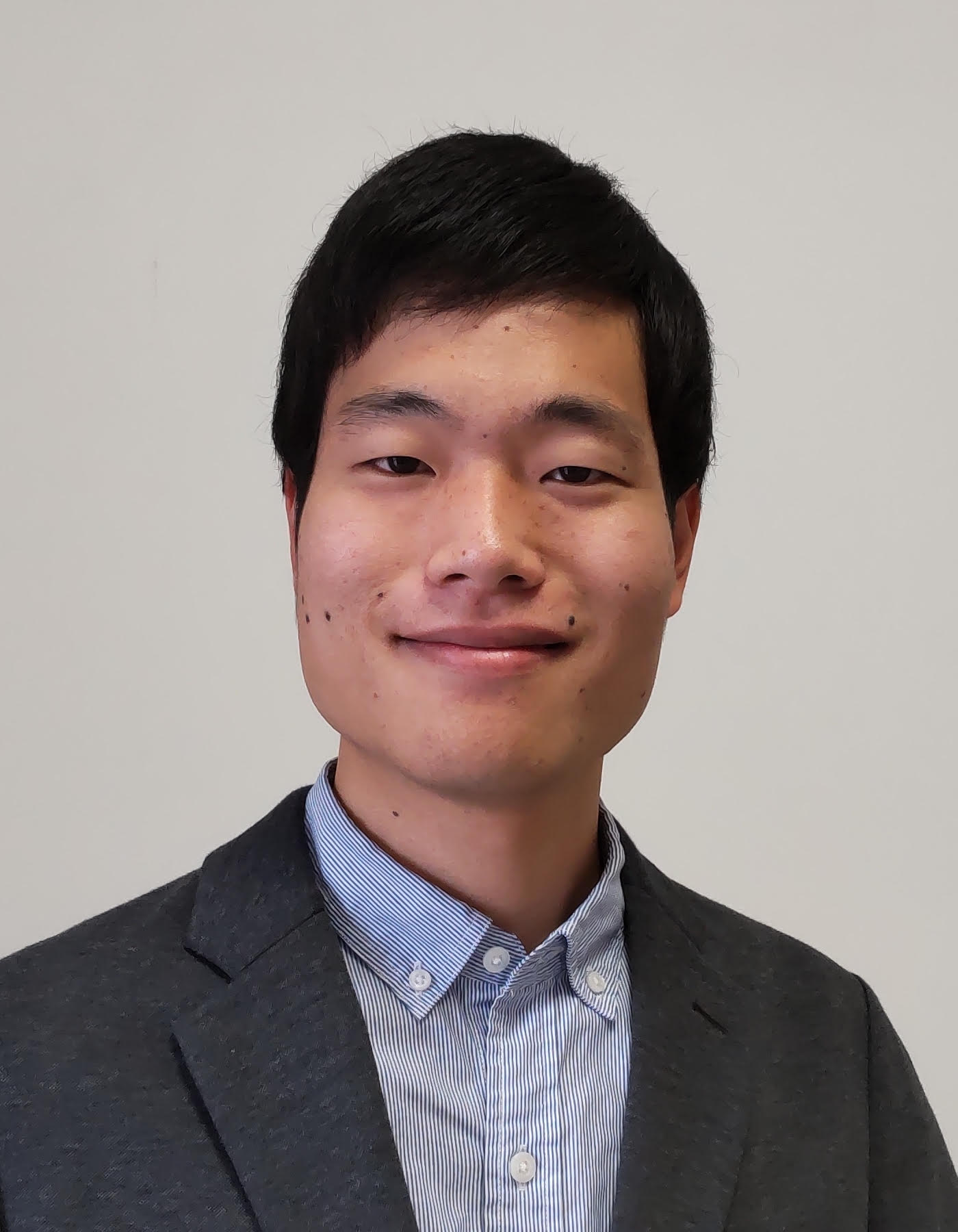
Because open access and sharing research is part of Frontiers’ mission, we want to give researchers the voice to express themselves and their research with more creativity and freedom than they otherwise would have in publishing an academic paper.
If you’ve recently published your paper with Frontiers and believe you have a great story to tell, then send an email to press@frontiersin.org with ‘Frontier Scientists’ and your name in the subject line.
What inspired you to become a researcher? Do you have any specific memories that set off a spark?
What made me decide to do the research was the robot competition, called RoboCon, in my school days. When I was a student at Kagawa Tech, I was a member of a team participating in that competition. I developed the circuitry and software for some competition robots, and experienced that the performance of a robot can vary greatly depending on its control.
Since then, I have been interested in what kind of control can maximize the performance of a robot. For this reason, in my master’s course, I conducted research on control inspired by animals that can move adaptively in various environments.
Can you tell us about the research you’re currently working on?
We focused on understanding the mechanisms of animal locomotion by developing quadruped robots that can reproduce the neuromuscular dynamics of animals.
Reproducing control structures of animals in robots has two aims. First, to contribute to the well-known field of biology-inspired robotics, where the structure of a robot is made similar to that of an animal. We hope that our results will thus result in fundamental new technology for realizing robots that move efficiently and flexibly like animals.
Second, we aim to contribute to biology in return. At present, experiments using real animals are strictly controlled and restricted, making it difficult to study the inner workings of living, moving animals. In the young research field of robotics-inspired biology, researchers are trying to understand animals by using robots instead of experimental animals. In the future, as more and more robots replace experimental animals, researchers may be able to investigate animal mechanisms under a wider variety of experimental conditions.
In the future, as more and more robots replace experimental animals, researchers may be able to investigate animal mechanisms under a wider variety of experimental conditions.
Toyoaki Tanikawa
In your opinion, why is your research important?
First, in our new study we contribute to the development of novel scientific methods to reproduce and understand quadrupeds using robots, as described above. Another important result is that we have provided a new hypothesis about the locomotion mechanism of cats.
By exploring reflex circuits that could result in a walking quadruped robot, we arrived at a simple novel reflex circuit, which we have named ‘reciprocal excitatory circuit between hip and knee extensors’. By simply reproducing the reciprocal circuit in each leg, the robot generated steady walking motions. We also show that the robot’s gait becomes unstable when the reciprocal circuit is cut off, and that the reciprocal excitatory circuit produced a phenomenon known to occur in cats, called ‘prolongation of the stance phase’. This suggests that the reciprocal excitatory circuit is an important component of the cat’s neural circuit.
Are there any common misconceptions about this area of research? How would you address them?
Robotics is often thought of as a technology that is only for building better robots. However, if used in a different way, robotics can be a very useful scientific tool for reproducing and understanding dynamical systems with complex interactions, such as animals. Of course, not all phenomena discovered in the robot will be observed in animals, but the cycle of discovering new phenomena can be accelerated by providing new hypotheses to biology based on findings from the robot.
What are some of the areas of research you’d like to see tackled in the years ahead?
I graduated from the master’s course this spring and got a job at a company. If I have a chance to do my research in the future, I would like to work on the motion control of robots, which is a further development of our results.
How has open science benefited the reach and impact of your research?
Papers submitted to open access journals have fewer copyright issues. Therefore, I can use various means such as images and videos to advertise the results of my research to the public. Also, since I am leaving the university this spring, being able to read academic papers for free will be a great advantage.
Read Tanikawa et al’s study in Frontiers in Neurorobotics: A Reciprocal Excitatory Reflex Between Extensors Reproduces the Prolongation of Stance Phase in Walking Cats: Analysis on a Robotic Platform.
This article was initially published on the Frontiers blog.
A tactile sensing mechanism for soft robotic fingers
Robotics Changed Industry 4.0; Now, How Will Industry 4.0 Change Robotics?
#IROS2020 Real Roboticist focus series #1: Davide Scaramuzza (Drones & Magic)
Are you curious about the people behind the robots? The series ‘Real Roboticist’, produced by the 2020 IEEE/RSJ International Conference on Intelligent Robots and Systems (IROS), shows the people at the forefront of robotics research from a more personal perspective. How did they become roboticists? What made them proud and what challenges did they face? What advice would they give to their younger self? What does a typical day look like? And where do they see the future of robotics? In case you missed it during the On-Demand conference, no worries! IEEE has recently made their original series public, and every Sunday we’ll bring you an interview with a real roboticist for you to get inspired.
This week is the turn of Davide Scaramuzza, Professor and Director of the Robotics and Perception Group at the University of Zürich. In his talk, Davide explains his journey from Electronics Engineering to leading a top robotics vision research group developing a promising technology: event cameras. He’ll also speak about the challenges he faced along the way, and even how he combines the robotics research with another of his passions, magic. Curious about where the magic happens? Davide also takes you around his research lab during the interview. Let the magic happen!
Kitchen robot in Riga cooks up new future for fast food
Decoding how salamanders walk
Tips for choosing a 3D vision system – The future of vision systems in manufacturing
Student designs compact lifesaving drone for beach rescue teams
r/Robotics showcase
The first Reddit Robotics Showcase will take place online this weekend (31st July – 1 August). Multitude of projects underway in the r/robotics reddit community will be showcased. There will be nearly 30 presentations from members of the r/robotics community, ranging from hobbyists, professionals, academics, industrial, students, etc. across four different categories: simulation, mobile robots, manipulation, and legged robots. The showcase will be livestreamed via the Reddit Robotics Showcase YouTube channel, and you will be able to pose questions to the participants.
Keynote Speakers
Saturday, 31st of July
Simulation: Brian Gerkey – Open Robotics – 10:00 EDT (15:00 BST, 23:00 JST)
Mobile Robots: Mark Emerton – UKRI – 14:00 EDT (19:00 BST, 03:00 JST)
Sunday, 1st of August
Manipulation: Rich Walker – Shadow Robot Company – 10:00 EDT (15:00 BST, 23:00 JST)
Legged Robots: Shamel Fahmi – Italian Institute of Technology – 14:00 EDT (19:00 BST, 03:00 JST)
For more information and the full programme, please visit the website.