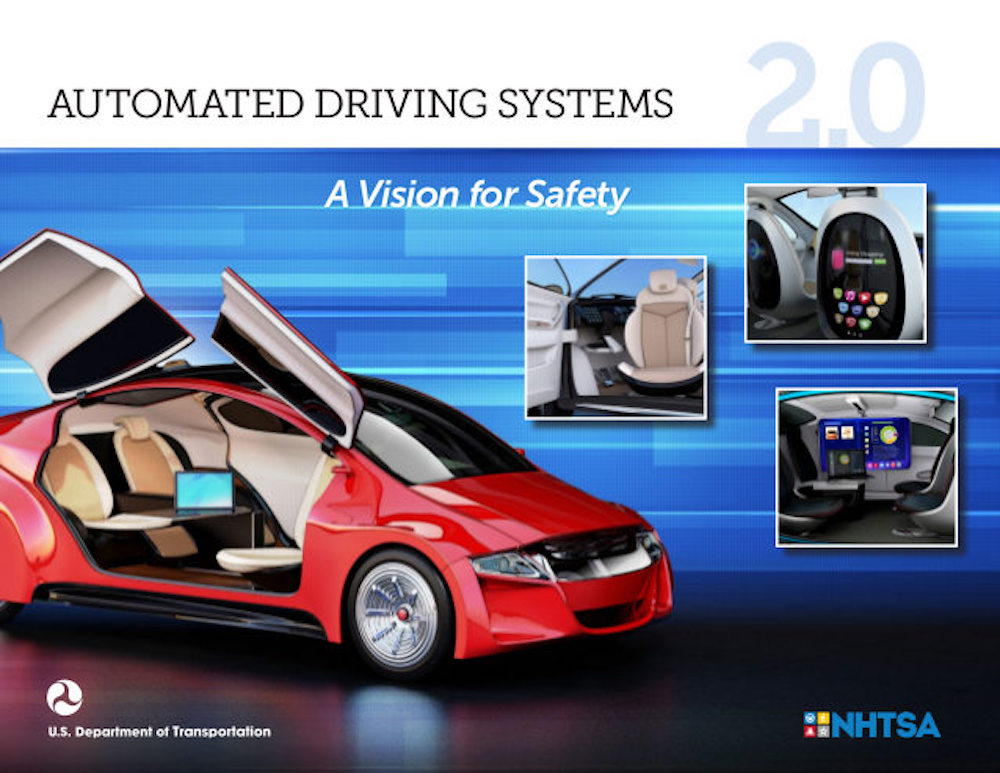
NHTSA released their latest draft robocar regulations just a week after the U.S. House passed a new regulatory regime and the senate started working on its own. The proposed regulations preempt state regulation of vehicle design, and allow companies to apply for high volume exemptions from the standards that exist for human-driven cars.
It’s clear that the new approach will be quite different from the Obama-era one, much more hands-off. There are not a lot of things to like about the Trump administration but this could be one of them. The prior regulations reached 116 pages with much detail, though they were mostly listed as “voluntary.” I wrote a long critique of the regulations in a 4 part series which can be found in my NHTSA tag. They seem to have paid attention to that commentary and the similar commentary of others.
At 26 pages, the new report is much more modest, and actually says very little. Indeed, I could sum it up as follows:
- Do the stuff you’re already doing
- Pay attention to where and when your car can drive and document that
- Document your processes internally and for the public
- Go to the existing standards bodies (SAE, ISO etc.) for guidance
- Create a standard data format for your incident logs
- Don’t forget all the work on crash avoidance, survival and post-crash safety in modern cars that we worked very hard on
- Plans for how states and the feds will work together on regulating this
Goals vs. Approaches
The document does a better job at understanding the difference between goals — public goods that it is the government’s role to promote — and approaches to those goals, which should be entirely the province of industry.
The new document is much more explicit that the 12 “safety design elements” are voluntary. I continue to believe that there is a risk they may not be truly voluntary, as there will be great pressure to conform with them, and possible increased liability for those who don’t, but the new document tries to avoid that, and its requests are much milder.
The document understands the important realization that developers in this space will be creating new paths to safety and establishing new and different concepts of best practices. Existing standards have value, but they can at best encode conventional wisdom. Robocars will not be created using conventional wisdom. The new document takes the approach of more likely recommending that the existing standards be considered, which is a reasonable plan.
A lightweight regulatory philosophy
My own analysis is guided by a lightweight regulatory approach which has been the norm until now. The government’s role is to determine important public goals and interests, and to use regulations and enforcement when, and only when, it becomes clear that industry can’t be trusted to meet these goals on its own.
In particular, the government should very rarely regulate how something should be done, and focus instead on what needs to happen as the end result, and why. In the past, all automotive safety technologies were developed by vendors and deployed, sometimes for decades, before they were regulated. When they were regulated, it was more along the lines of “All cars should now have anti-lock brakes.” Only with the more mature technologies have the regulations had to go into detail on how to build them.
Worthwhile public goals include safety, of course, and the promotion of innovation. We want to encourage both competition and cooperation in the right places. We want to protect consumer rights and privacy. (The prior regulations proposed a mandatory sharing of incident data which is watered down greatly in these new regulations.)
I call this lightweight because others have called for a great deal more regulation. I don’t, however, view it is highly laissez-faire. Driving is already highly regulated, and the idea that regulators would need to write rules to prevent companies from doing things they have shown no evidence of doing seems odd to me. Particularly in a fast-changing field where regulators (and even developers) admit they have limited knowledge of what the technology’s final form will actually be.
Stating the obvious
While I laud the reduction of detail in these regulations, it’s worth pointing out that many of the remaining sections are stripped to the point of mostly outlining “motherhood” requirements — requirements which are obvious and that every developer has known for some time. You don’t have to say that the vehicle should follow the vehicle code and not hit other cars. Anybody who needs to be told that is not a robocar developer. The set of obvious goals belongs better in a non-governmental advice document (which this does in fact declare itself in part to be, though of course governmental) than in something considered regulatory.
Overstating the obvious and discouraging the “black box.”
Sometimes a statement can be both obvious but also possibly wrong in the light of new technology. The document has many requirements that vendors document their thinking and processes which may be very difficult to do with systems built with machine learning. Machine learning sometimes produces a “black box” that works, but there is minimal knowledge as to how it works. It may be that such systems will outperform other systems, leaving us with the dilemma of choosing between a superior system we can’t document and understand, and an inferior one we can.
There is a new research area known as “explainable AI” which hopes to bridge this gap and make it possible to document and understand why machine learning systems operate as they do. This is promising research but it may never be complete. In spite of this, EU regulations currently are already attempting to forbid unexplainable AI. This may cut off very productive avenues of development — we don’t know enough to be sure about this as yet.
Some minor notes
The name
The new report pushes a new term — Automated Driving Systems. It seems every iteration comes up with a new name. The field is really starting to need a name people agree on, since nobody seems to much like driverless cars, self-driving cars, autonomous vehicles, automated vehicles, robocars or any of the others. This one is just as unwieldy, and its acronym is an English word and thus hard to search for.
The levels
The SAE levels continue to be used. I have been critical of the levels before, recently in this satire. It is wrong to try to understand robocars primarily through the role of humans in their operation, and wrong to suggest there is a progression of levels based on that.
The 12 safety elements
As noted, most of the sections simply advise obvious policies which everybody is already doing, and advise that teams document what they are doing.
1. System Safety
This section is modest, and describes fairly common existing practices for high reliability software systems. (Almost to the point that there is no real need for the government to point them out.)
2. Operational Design Domain
The idea of defining the situations where the car can do certain things is a much better approach than imagining levels of human involvement. I would even suggest it replace the levels, and the human seen simply as one of the tools to be used to operate outside of certain domains. Still, I see minimal need for NHTSA to say this — everybody already knows that roads and their conditions are different and complex and need different classes of technology.
3. Object and Event Detection and Response, 4. Fallback, 5. Validation, 6. HMI
Again, this is fairly redundant. Vendors don’t need to be told that vehicles must obey the vehicle code and stay in their lane and not hit things. That’s already the law. They know that only with a fallback strategy can they approach the reliability needed.
7. Computer Security
While everything here is already on the minds of developers, I don’t fault the reminder here because traditional automakers have a history of having done security badly. The call for a central clearing house on attacks is good, though it should not necessarily be Auto-ISAC.
8. Occupant Protection
A great deal of the current FMVSS (Federal Motor Vehicle Safety Standards) are about this, and because many vehicles may use exemptions from FMVSS to get going, a reminder about this is in order.
10. Data Recording
The most interesting proposal in the prior document was a requirement for public sharing of incident and crash data so that all teams could learn from every problem any team encounters. This would speed up development and improve safety, but vendors don’t like the fact it removes a key competitive edge — their corpus of driving experience.
The new document calls for a standard data format, and makes general motherhood calls for storing data in a crash, something everybody already does.
The call for a standard is actually difficult. Every vehicle has a different sensor suite and its own tools to examine the sensor data. Trying to standardize that on a truly useful level is a serious task. I had expected this task to fall to outside testing companies, who would learn (possibly reverse engineering) the data formats of each car and try to put them in a standard format that was actually useful. I fear a standard agreed upon by major players (who don’t want to share their data) will be minimal and less useful.
State Roles
A large section of the document is about the bureaucratic distribution of roles between states and federal bodies. I will provide analysis of this later.
Conclusion
This document reflects a major change, almost a reversal, and largely a positive one. Going forward from here, I would encourage that the debate on regulation focus on
- What public goods does the government have an interest in protecting?
- Which ones are vendors showing they can’t be trusted to support voluntarily, both by present actions and past history?
- How can innovation be encouraged and facilitated, and good communication be made to the public about what’s going on
One of the key public goods missing from this document is privacy protection. This is one of the areas where vendors don’t have a great past history.
Another one is civil rights protection — for example what powers police will want over cars — where the government has a bad history.