World’s first robotic squash coach
Drones will soon be transporting medical samples in Norway
Why Use Offline Robot Programming Software and How to Get Started
Should a home robot follow what the mom says? Recap of what happened at RO-MAN Roboethics Competition
On August 8th, 2021, a team of four graduate students from the University of Toronto presented their ethical design in the world’s first ever roboethics competition, the RO-MAN 2021 Roboethics to Design & Development Competition. During the competition, design teams tackled a challenging yet relatable scenario—introducing a robot helper to the household. The students’ solution, entitled ”Jeeves, the Ethically Designed Interface (JEDI)”, demonstrated how home robots can act safely and according to social and cultural norms. Click here to watch their video submission. JEEVES acted as an extension of the mother and the interface rules accommodated her priorities. For example, the delivery of alcohol was prohibited when the mother was not home. Moreover, JEEVES was cautious about delivering hazardous material to minors and animals.
Judges from around the world, with diverse backgrounds ranging from industry professionals to lawyers and professors in ethics, gave their feedback on the team’s submission. Open Roboethics Institute also hosted an online opinion poll to hear what the general public thinks about the solution for this challenge. We polled 172 participants who were mostly from the U.S, as we used SurveyMonkey to get responses. Full results from the surveys can be found here.
I think that JEEVES suggests a reasonable and fair solution for the robot-human interactions that could happen in our everyday lives within a
household.RO-MAN Roboethics Competition Judge
The evaluation of JEEVES from the judges and the public was positive and yet critical.
The judges generally felt that the team’s solution is understandable, accessible, and simple to implement. In our public opinion poll, the public also felt similarly about JEEVES. “I think that JEEVES suggests a reasonable and fair solution for the robot-human interactions that could happen in our everyday lives within a household”, said MinYoung Yoo, a PhD student studying Human-Computer Interaction at Simon Fraser University. “The three grounding principles are rock solid and [the robot’s] decisions meet moral expectations.” The public’s opinion echoed these thoughts. Of the 172 people we surveyed, around 43% felt that the solution was effective in addressing the ethical challenges posed by the home robot.
In addition, about 40% of respondents also evaluated the JEDI solution as realistic and relatable.
However, about 38 – 41% of the poll participants were indecisive about how effective or relatable the solution and about 18% thought it was neither effective or relatable. The judge’s discussion could inform why the participants had this perspective.
Concerns about JEEVES being limited in scope and not generalizable came up throughout the conversation with the judges. With any solution, it is really important to consider how the design would apply in a variety of different scenarios. Although this competition presents the challenge of how a robot may interact with a single-mother household, the judges asked what would happen if there was a second adult in the home. For example, if the mom had a long-term girlfriend and they bought the robot together, would the robot still defer to the mother for important decisions, such as when to give alcohol to the teenage daughter and her boyfriend? In another scenario, the mom purchases a new piece of jewellery for her daughter. This piece of jewellery is her birthday present and because of its size and its shape it could be hazardous for the dog and the baby. Should the robot deliver this item to the daughter if she asks for it while the dog and baby are in the room?
[The JEEVES solution] assumes a single owner and thus puts the responsibility on one person only. What happens when there are two parents and they disagree on things?
A public opinion poll participant
As reflected in the earlier scenario, a major topic of discussion was on ownership and who should be responsible for the robot’s decisions. A respondent of the public opinion poll was also worried about the ownership of the home robot: “[The JEEVES solution] assumes a single owner and thus puts the responsibility on one person only. What happens when there are two parents and they disagree on things?”
Timothy Lee, one of the judges and an industry expert in mechatronics, posed a similar worry, “What if the mother is intoxicated and makes the wrong call?” Placing the onus on only one individual to make the right decisions is risky. Correspondingly, a majority of the participants (about 60%) disliked that the solution assumes that the intentions and choices of the mother are always good. Interestingly, a smaller portion of participants thought that the daughter or boyfriend’s perspective should be taken into account. ORI had explored how ownership of a robot should affect a robot’s action in a previous poll, and it was clear that people were divided on what a robot should do based on ownership. Humans have a strong sense of ownership and this is reflected in law (ex. Product liability, company ownership, etc). How robotic platform ownership should be managed is a major research and legal question.
The crux of the JEEVES solution lies in the robot’s ability to categorize objects as harmless (i.e. food and water), hazardous, and personal possessions. However, how objects are categorized can change over time. Dr. Tae Wan Kim, a professor in business ethics, posed an interesting thought regarding the categorization of the mother’s gun. The team initially designed the robot to only give the gun to the mother and no other member of the household. However, Dr. Kim presented an interesting potential counterexample—what if an armed thief breaks into the house and the daughter needs the gun for self-defense? In this particular situation, should the robot give the gun to the daughter even though it is considered a hazardous object? Or perhaps, does it become more hazardous to not give the gun to the daughter? In response to this scenario, a member of the design team added that the baby will also grow up and certain objects will no longer be hazardous.
JEEVES ultimately prioritizes the safety of the household members in its ethical design, as reflected in the team’s report: “The first priority is the prevention of harm to users, the robot, and the environment.” Interestingly, the public seemed to have a slightly different opinion. The majority of poll respondents liked that the solution values and protects the privacy for the owner of the objects. In fact, more people seemed to value privacy over physical safety, which is a somewhat surprising result. But perhaps this is because the public doesn’t believe that the home robot can really cause physical harm. Alternatively, the public might be more concerned about their privacy considering the association between smart technologies and data breaches in the media over the past few years. Finally, It is important to highlight that all of the participants were from the United States or Canada where privacy is highly valued in society. Other cultures could have a very different perspective on which values should be prioritized.
Another notable point is that these ethical issues in robotics are exceptionally difficult to solve. “There’s no right answer, and that’s the beauty of itthere are just a whole bunch of answers with different reasoning that we can discuss”, said one of the judges at the end of the evaluation session. As reflected by our poll results where a significant number of people were unclear about how they felt, as well as the ongoing debates between experts in the field, and the judges’ open-ended comments, developing an ethical robot is an immense challenge. Any attempt is a commendable feat—and JEEVES is an excellent start.
There’s no right answer, and that’s the beauty of itthere are just a whole bunch of answers with different reasoning that we can discuss.
RO-MAN Roboethics Competition Judge
Soft components for the next generation of soft robotics
Singapore is testing robots to patrol the streets for ‘undesirable’ behavior like smoking
Parcel: A Lower-Cost Way To Automate Last-Mile Delivery Hubs
#IROS2020 Plenary and Keynote talks focus series #6: Jonathan Hurst & Andrea Thomaz
This week you’ll be able to listen to the talks of Jonathan Hurst (Professor of Robotics at Oregon State University, and Chief Technology Officer at Agility Robotics) and Andrea Thomaz (Associate Professor of Robotics at the University of Texas at Austin, and CEO of Diligent Robotics) as part of this series that brings you the plenary and keynote talks from the IEEE/RSJ IROS2020 (International Conference on Intelligent Robots and Systems). Jonathan’s talk is in the topic of humanoids, while Andrea’s is about human-robot interaction.
|
Prof. Jonathan Hurst – Design Contact Dynamics in Advance Bio: Jonathan W. Hurst is Chief Technology Officer and co-founder of Agility Robotics, and Professor and co-founder of the Oregon State University Robotics Institute. He holds a B.S. in mechanical engineering and an M.S. and Ph.D. in robotics, all from Carnegie Mellon University. His university research focuses on understanding the fundamental science and engineering best practices for robotic legged locomotion and physical interaction. Agility Robotics is bringing this new robotic mobility to market, solving problems for customers, working towards a day when robots can go where people go, generate greater productivity across the economy, and improve quality of life for all. |
![]() |
Prof. Andrea Thomaz – Human + Robot Teams: From Theory to Practice Bio: Andrea Thomaz is the CEO and Co-Founder of Diligent Robotics and a renowned social robotics expert. Her accolades include being recognized by the National Academy of Science as a Kavli Fellow, the US President’s Council of Advisors on Science and Tech, MIT Technology Review on its Next Generation of 35 Innovators Under 35 list, Popular Science on its Brilliant 10 list, TEDx as a featured keynote speaker on social robotics and Texas Monthly on its Most Powerful Texans of 2018 list. Andrea’s robots have been featured in the New York Times and on the covers of MIT Technology Review and Popular Science. Her passion for social robotics began during her work at the MIT Media Lab, where she focused on using AI to develop machines that address everyday human needs. Andrea co-founded Diligent Robotics to pursue her vision of creating socially intelligent robot assistants that collaborate with humans by doing their chores so humans can have more time for the work they care most about. She earned her Ph.D. from MIT and B.S. in Electrical and Computer Engineering from UT Austin, and was a Robotics Professor at UT Austin and Georgia Tech (where she directed the Socially Intelligent Machines Lab). Andrea is published in the areas of Artificial Intelligence, Robotics, and Human-Robot Interaction. Her research aims to computationally model mechanisms of human social learning in order to build social robots and other machines that are intuitive for everyday people to teach. Andrea has received an NSF CAREER award in 2010 and an Office of Naval Research Young Investigator Award in 2008. In addition Diligent Robotics robot Moxi has been featured on NBC Nightly News and most recently in National Geographic “The robot revolution has arrived”. |
Could Cobots Be the Answer to the Welder Shortage?
When humans play in competition with a humanoid robot, they delay their decisions when the robot looks at them
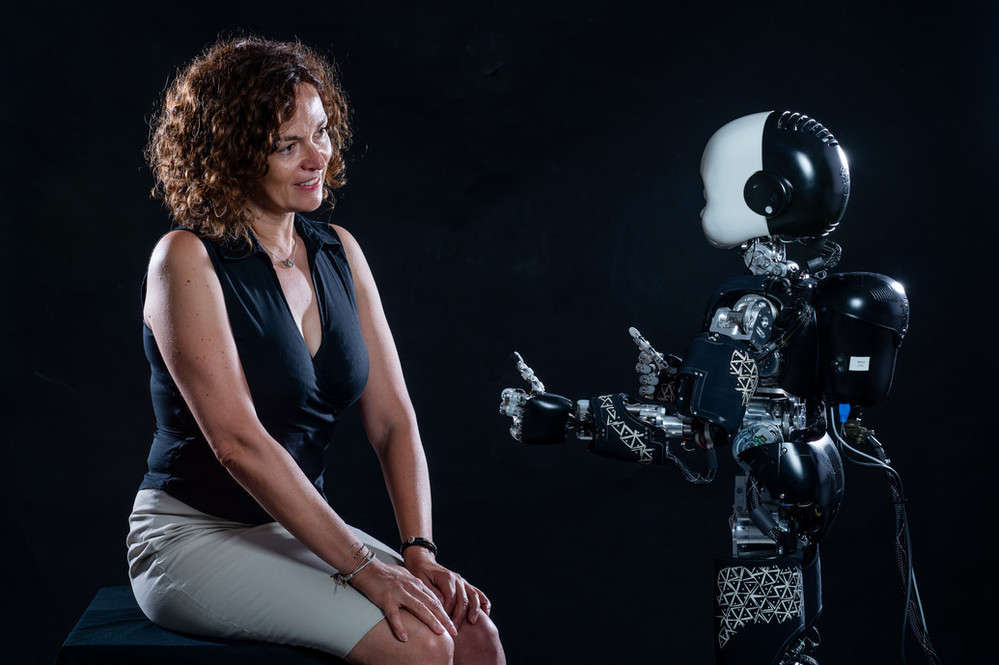
Gaze is an extremely powerful and important signal during human-human communication and interaction, conveying intentions and informing about other’s decisions. What happens when a robot and a human interact looking at each other? Researchers at IIT-Istituto Italiano di Tecnologia (Italian Institute of Technology) investigated whether a humanoid robot’s gaze influences the way people reason in a social decision-making context. What they found is that a mutual gaze with a robot affects human neural activity, influencing decision-making processes, in particular delaying them. Thus, a robot gaze brings humans to perceive it as a social signal. These findings have strong implications for contexts where humanoids may find applications such as co-workers, clinical support or domestic assistants.
The study, published in Science Robotics, has been conceived within the framework of a larger overarching project led by Agnieszka Wykowska, coordinator of IIT’s lab “Social Cognition in Human-Robot Interaction”, and funded by the European Research Council (ERC). The project, called “InStance”, addresses the question of when and under what conditions people treat robots as intentional beings. That is, whether, in order to explain and interpret robot’s behaviour, people refer to mental states such as beliefs or desires.
The research paper’s authors are Marwen Belkaid, Kyveli Kompatsiari, Davide de Tommaso, Ingrid Zablith, and Agnieszka Wykowska.
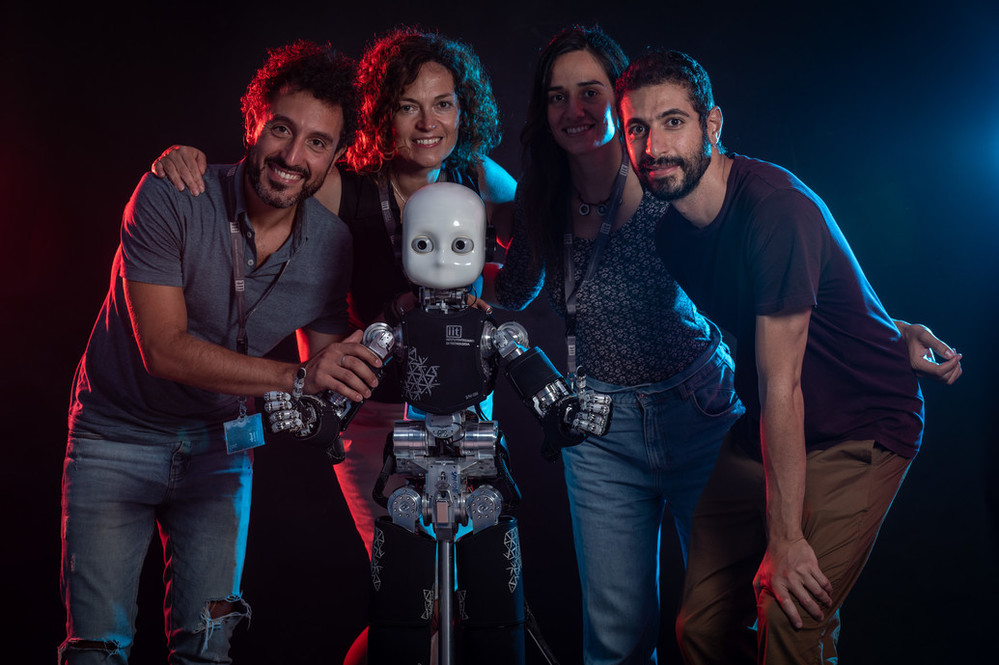
In most everyday life situations, the human brain needs to engage not only in making decisions, but also in anticipating and predicting the behaviour of others. In such contexts, gaze can be highly informative about others’ intentions, goals and upcoming decisions. Humans pay attention to the eyes of others, and the brain reacts very strongly when someone looks at them or directs gaze to a certain event or location in the environment. Researchers investigated this kind of interaction with a robot.
“Robots will be more and more present in our everyday life” comments Agnieszka Wykowska, Principal Investigator at IIT and senior author of the paper. “That is why it is important to understand not only the technological aspects of robot design, but also the human side of the human-robot interaction. Specifically, it is important to understand how the human brain processes behavioral signals conveyed by robots”.
Wykowska and her research group, asked a group of 40 participants to play a strategic game – the Chicken game – with the robot iCub while they measured the participants’ behaviour and neural activity, the latter by means of electroencephalography (EEG). The game is a strategic one, depicting a situation in which two drivers of simulated cars move towards each other on a collision course and the outcome depends on whether the players yield or keep going straight.
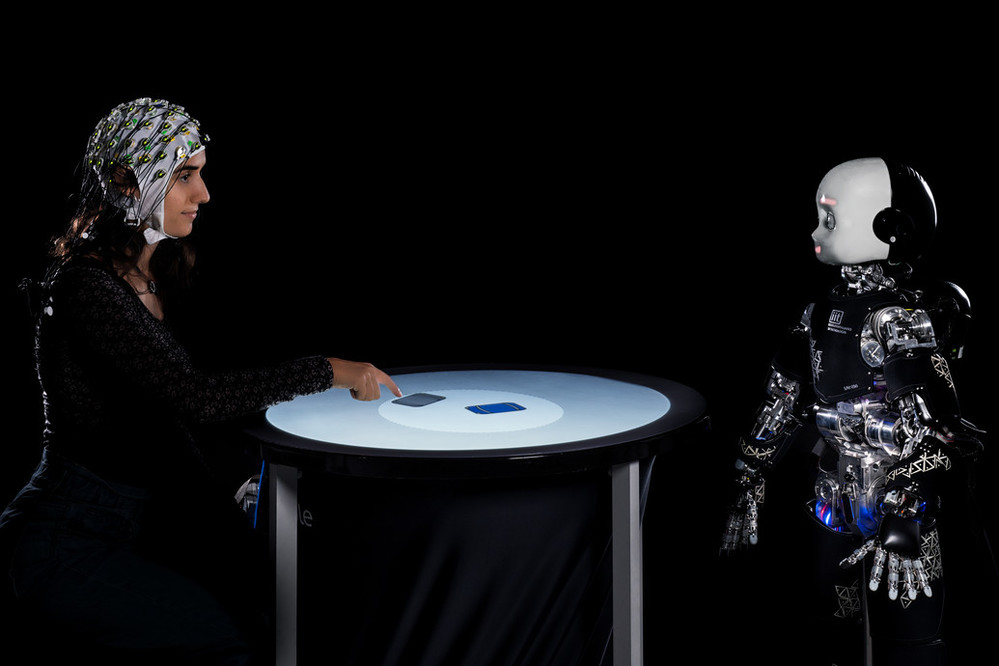
Researchers found that participants were slower to respond when iCub established mutual gaze during decision making, relative to averted gaze. The delayed responses may suggest that mutual gaze entailed a higher cognitive effort, for example by eliciting more reasoning about iCub’s choices or higher degree of suppression of the potentially distracting gaze stimulus, which was irrelevant to the task.
“Think of playing poker with a robot. If the robot looks at you during the moment you need to make a decision on the next move, you will have a more difficult time in making a decision, relative to a situation when the robot gazes away. Your brain will also need to employ effortful and costly processes to try to “ignore” that gaze of the robot” explains further Wykowska.
These results suggest that the robot’s gaze “hijacks” the “socio-cognitive” mechanisms of the human brain – making the brain respond to the robot as if it was a social agent. In this sense, “being social” for a robot could be not always beneficial for the humans, interfering with their performance and speed of decision making, even if their reciprocal interaction is enjoyable and engaging.
Wykowska and her research group hope that these findings would help roboticists design robots that exhibit the behaviour that is most appropriate for a specific context of application. Humanoids with social behaviours may be helpful in assisting in care elderly or childcare, as in the case of the iCub robot, being part of experimental therapy in the treatment of autism. On the other hand, when focus on the task is needed, as in factory settings or in air traffic control, presence of a robot with social signals might be distracting.
Super-stretchy wormlike robots capable of ‘feeling’ their surroundings
#IROS2020 Real Roboticist focus series #5: Michelle Johnson (Robots That Matter)
We’re reaching the end of this focus series on IEEE/RSJ IROS 2020 (International Conference on Intelligent Robots and Systems) original series Real Roboticist. This week you’ll meet Michelle Johnson, Associate Professor of Physical Medicine and Rehabilitation at the University of Pennsylvania.
Michelle is also the Director of the Rehabilitation Robotics Lab at the University of Pennsylvania, whose aim is to use rehabilitation robotics and neuroscience to investigate brain plasticity and motor function after non-traumatic brain injuries, for example in stroke survivors or persons diagnosed with cerebral palsy. If you’d like to know more about her professional journey, her work with affordable robots for low/middle income countries and her next frontier in robotics, among many more things, check out her video below!
How Implementing Robotics Affects Business Insurance
When mud is our greatest teacher
On 25 March, a small RoboHouse team went out in the fields of Oldeberkoop in East Friesland with gas leak detector Waylon and mechanic Rob. They began by checking the calibration to prevent malfunctions. Then they cleaned the detector mat and changed the filter, after which the search could start.
The two men proceeded slowly, constantly checking their tablet to see whether they were still close enough to the pipes. A big cart was pushed forward with the mat dragging behind it. Except for the grinding of the equipment on the sidewalk, everything was quiet.
The team waited to hear from the device, which is supposed to sound an alarm when it detects a leak. And then they heard it: a beep! After taking a few steps back, because the system lags, Rob sprayed a yellow dot. Waylon pointed to the beauty of the on-screen image that enables assessment of the severity of the leak. “It’s not a biggie”, Waylon said to the RoboHouse team. That means someone will come within a week to measure it again, before calling for a repair.
The team slowly walked on, looking for more leaks. When they bumped into any kind of obstacle, they had to lift the device over it themselves. They also pushed the big device while constantly looking down on their tablet. After a full day of leak detection, every member of the team could feel it in their shoulders.
The RoboHouse team then realised how easily bad ergonomics can lead to injuries. There have been tests with more expensive machines and Segways but a solution has not yet been found. So our ambition remains: develop robotic technology that transforms the daily grind of leak detection, but stay modest and don’t overestimate our progress.
At RoboHouse, the process of improving working life starts with the worker. “Research, development and co-creation go hand in hand to deploy robotics in the best way possible,” says Marieke Mulder, program manager. “The goal is to support 90% of the work in gas leak detection autonomously so Rob and Waylon can make the pipelines safe and future proof.”
The field research sparked many new ideas. Waylon was curious about next steps and Rob said: “I just hope we can come up with something that allows me to take the right routes without destroying my back by looking at the tablet all day”.
After the field session, the team from RoboHouse gathered experts from different sectors to analyse the challenges at hand and co-create the first concepts towards a solution. Development engineers Bas van Mil, Tom Dalhuisen and Guus Paris joined the team online in a workshop on Miro. Together they envisioned a way forward and this was translated into a roadmap to 2031 by the RoboHouse team.
There will be many interactions with workers at Alliander along the way, and many more hours in the field. Marieke Mulder says: “After walking just a mile through the mud, we have barely begun to know how it is to do this work every day. But by going beyond our lab, into the field, we already discovered so much more about the challenges that workers like Rob and Waylon face every day.”
The post When mud is our greatest teacher appeared first on RoboHouse.