A soft touch for robotic hardware
SILVER2 aquatic robot walks around on the seabed
Mobile Robotics and Market Adaptations after COVID-19
Mobile Robotics and Market Adaptations after COVID-19
Planetary exploration rover avoids sand traps with ‘rear rotator pedaling’
Flexible Conveyor Manufacturer Glide-Line Overcomes Space, Size, & Product Handling Constraints for Aerospace Industry Integrator
Flexible Conveyor Manufacturer Glide-Line Overcomes Space, Size, & Product Handling Constraints for Aerospace Industry Integrator
From SLAM to Spatial AI
You can watch this seminar here at 1PM EST (10AM PST) on May 15th.
Andrew Davison (Imperial College London)
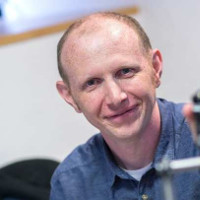
Abstract: To enable the next generation of smart robots and devices which can truly interact with their environments, Simultaneous Localisation and Mapping (SLAM) will progressively develop into a general real-time geometric and semantic `Spatial AI’ perception capability. I will give many examples from our work on gradually increasing visual SLAM capability over the years. However, much research must still be done to achieve true Spatial AI performance. A key issue is how estimation and machine learning components can be used and trained together as we continue to search for the best long-term scene representations to enable intelligent interaction. Further, to enable the performance and efficiency required by real products, computer vision algorithms must be developed together with the sensors and processors which form full systems, and I will cover research on vision algorithms for non-standard visual sensors and graph-based computing architectures.
Biography: Andrew Davison is Professor of Robot Vision and Director of the Dyson Robotics Laboratory at Imperial College London. His long-term research focus is on SLAM (Simultaneous Localisation and Mapping) and its evolution towards general `Spatial AI’: computer vision algorithms which enable robots and other artificial devices to map, localise within and ultimately understand and interact with the 3D spaces around them. With his research group and collaborators he has consistently developed and demonstrated breakthrough systems, including MonoSLAM, KinectFusion, SLAM++ and CodeSLAM, and recent prizes include Best Paper at ECCV 2016 and Best Paper Honourable Mention at CVPR 2018. He has also had strong involvement in taking this technology into real applications, in particular through his work with Dyson on the design of the visual mapping system inside the Dyson 360 Eye robot vacuum cleaner and as co-founder of applied SLAM start-up SLAMcore. He was elected Fellow of the Royal Academy of Engineering in 2017.
Robotics Today Seminars
“Robotics Today – A series of technical talks” is a virtual robotics seminar series. The goal of the series is to bring the robotics community together during these challenging times. The seminars are scheduled on Fridays at 1PM EDT (10AM PDT) and are open to the public. The format of the seminar consists of a technical talk live captioned and streamed via Web and Twitter (@RoboticsSeminar), followed by an interactive discussion between the speaker and a panel of faculty, postdocs, and students that will moderate audience questions.
Stay up to date with upcoming seminars with the Robotics Today Google Calendar (or download the .ics file) and view past seminars on the Robotics Today Youtube Channel. And follow us on Twitter!
Upcoming Seminars
Seminars will be broadcast at 1PM EST (10AM PST) here.
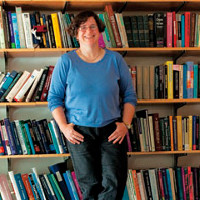
22 May 2020: Leslie Kaelbling (MIT)
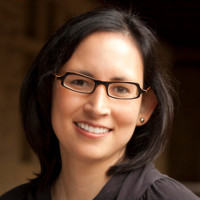
29 May 2020: Allison Okamura (Stanford)
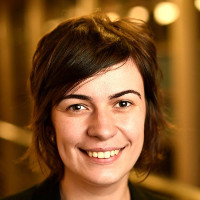
12 June 2020: Anca Dragan (UC Berkeley)
Past Seminars
We’ll post links to the recorded seminars soon!
Organizers
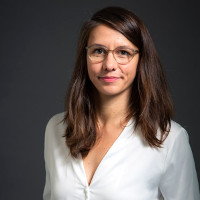
Stanford
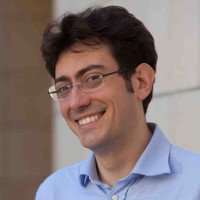
Stanford
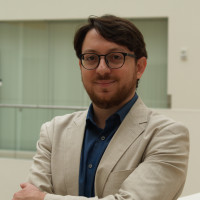
MIT
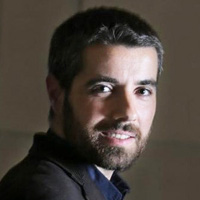
MIT
Contact
Velodyne Lidar Sensors Power 3D Data Capture in New NavVis VLX Mapping System
Business Perspectives and the Impacts of COVID-19 – Q&A with BitFlow Inc.
Soft robotic exosuit makes stroke survivors walk faster and farther
Researchers develop real-time physics engine for soft robotics
‘Data clouds fusion’ helps robots work as a team in hazardous situations
#309: Learning to Grasp, with Jeannette Bohg
In this episode, Lilly Clark interviews Jeannette Bohg, Assistant Professor at Stanford, about her work in interactive perception and robot learning for grasping and manipulation tasks. Bohg discusses how robots and humans are different, the challenge of high dimensional data, and unsolved problems including continuous learning and decentralized manipulation.
Jeannette Bohg is an Assistant Professor of Computer Science at Stanford University. She was a group leader at MPI until September 2017 and remains affiliated as a guest researcher. Her research focuses on perception for autonomous robotic manipulation and grasping. She is specifically interested in developing methods that are goal-directed, real-time and multi-modal such that they can provide meaningful feedback for execution and learning.
Before joining the Autonomous Motion lab in January 2012, Jeannette Bohg was a PhD student at the Computer Vision and Active Perception lab (CVAP) at KTH in Stockholm. Her thesis on Multi-modal scene understanding for Robotic Grasping was performed under the supervision of Prof. Danica Kragic. She studied at Chalmers in Gothenburg and at the Technical University in Dresden where she received her Masters in Art and Technology and her Diploma in Computer Science, respectively.
Links