Honeywell Study: Advancements In Warehouse Automation Will Present New Job Classes, Career Opportunities
Deep learning in MRI beyond segmentation: Medical image reconstruction, registration, and synthesis
RoboTED: a case study in Ethical Risk Assessment
A few weeks ago I gave a short paper at the excellent International Conference on Robot Ethics and Standards (ICRES 2020), outlining a case study in Ethical Risk Assessment – see our paper here. Our chosen case study is a robot teddy bear, inspired by one of my favourite movie robots: Teddy, in A. I. Artificial Intelligence.
Although Ethical Risk Assessment (ERA) is not new – it is after all what research ethics committees do – the idea of extending traditional risk assessment, as practised by safety engineers, to cover ethical risks is new. ERA is I believe one of the most powerful tools available to the responsible roboticist, and happily we already have a published standard setting out a guideline on ERA for robotics in BS 8611, published in 2016.
Before looking at the ERA, we need to summarise the specification of our fictional robot teddy bear: RoboTed. First, RoboTed is based on the following technology:
- RoboTed is an Internet (WiFi) connected device,
- RoboTed has cloud-based speech recognition and conversational AI (chatbot) and local speech synthesis,
- RoboTed’s eyes are functional cameras allowing RoboTed to recognise faces,
- RoboTed has motorised arms and legs to provide it with limited baby-like movement and locomotion.
And second RoboTed is designed to:
- Recognise its owner, learning their face and name and turning its face toward the child.
- Respond to physical play such as hugs and tickles.
- Tell stories, while allowing a child to interrupt the story to ask questions or ask for sections to be repeated.
- Sing songs, while encouraging the child to sing along and learn the song.
- Act as a child minder, allowing parents to both remotely listen, watch and speak via RoboTed.
The tables below summarise the ERA of RoboTED for (1) psychological, (2) privacy & transparency and (3) environmental risks. Each table has 4 columns, for the hazard, risk, level of risk (high, medium or low) and actions to mitigate the risk. BS8611 defines an ethical risk as the “probability of ethical harm occurring from the frequency and severity of exposure to a hazard”; an ethical hazard as “a potential source of ethical harm”, and an ethical harm as “anything likely to compromise psychological and/or societal and environmental well-being”.
(1) Psychological Risks
(2) Security and Transparency Risks
(3) Environmental Risks
For a more detailed commentary on each of these tables see our full paper – which also, for completeness, covers physical (safety) risks. And here are the slides from my short ICRES 2020 presentation:
Through this fictional case study we argue we have demonstrated the value of ethical risk assessment. Our RoboTed ERA has shown that attention to ethical risks can
- suggest new functions, such as “RoboTed needs to sleep now”,
- draw attention to how designs can be modified to mitigate some risks,
- highlight the need for user engagement, and
- reject some product functionality as too risky.
But ERA is not guaranteed to expose all ethical risks. It is a subjective process which will only be successful if the risk assessment team are prepared to think both critically and creatively about the question: what could go wrong? As Shannon Vallor and her colleagues write in their excellent Ethics in Tech Practice toolkit design teams must develop the “habit of exercising the skill of moral imagination to see how an ethical failure of the project might easily happen, and to understand the preventable causes so that they can be mitigated or avoided”.
Raptor-inspired drone with morphing wing and tail
Multi-drone system autonomously surveys penguin colonies
Electrostatic Dissipative Flooring vs. Anti-Static Flooring: Which Flooring is Right for Your Facility?
How to Design an Exoskeleton
Researchers improve autonomous boat design
Researchers create robots that can transform their wheels into legs
Dog training methods help teach robots to learn new tricks
AI improves control of robot arms
How-To Guide on Mastering PACK EXPO Connects
Lobe.ai Review

Lobe.ai just released for open beta and the short story is that you should go try it out. I was lucky and got to test it in the closed beta so I figured i should review a short review.
Making AI more understandable and accessible for most people is something I spend a lot of time on and Lobe is without a doubt right down my alley. The tagline is “machine learning made simple” and that is exactly what they do.
Overall great tool and I see it as an actual advance in the AI technology by making AI and deep learning models even more accessible than the AutoML wave is already doing.
So what is Lobe.ai exactly?
Lobe.ai is an Automl tool. That means that you can make AI without coding. In Lobe’s case they work with image classification only. So in short you give Lobe a set of images with labels and Lobe will automatically find the most optimal model to classify the images.
Lobe is also acquired by Microsoft. I think that’s a pretty smart move by Microsoft. The big clouds can be difficult to get started with and especially Microsoft's current AutoML solutions is first of all only tabular data but also requires a good degree of technical skills to get started.
It’s free. I don’t really understand the business model yet, but so far the software is free. That is pretty cool but I’m still curious about how the plan on getting revenue to keep up the good work.
Features
Image classification
So far Lobe only has one main feature and that’s training and image classification network. And it does that pretty well. In all the tests I have done I have gotten decent results with only very little training data.
Speed
The speed is insane. The models are being trained in something that seems like a minut. That’s a really cool feature. You can also decide to train it for longer to get better accuracy.
Export
You can export the model to CoreML, TensorFlow, TensorFlow Lite and they also provide a local API.
Use Cases
I’m planning to use Lobe for both hobby and commercial projects. For commercial use I’m going to use it for the main three purposes:
Producing models
Since the quality is good and the export possibilities are ready for production I see no reason not to use this for production purposes when helping clients with AI projects. You might think it’s better to hand build models for commercial use, but my honest experience is that many simple problems should be solved with the simplest solution first. You might stay with a model build with Lobe for at least the first few iterations of a project and sometimes forever.
Teaching
As I’m teaching people about applied AI, Lobe is going to be one of my go to tools from now on. It makes AI development very tangible and accessible and you can play around with it to get a feeling about AI without having to code. When project and product managers get to try developing models themself I expect a lot more understanding of edge cases and unforeseen problems.
Selling
When trying to convince a potential customer that they should invest in AI development you easily run into decision makers that have no understanding about AI. By showing Lobe and doing live tests I hope to be able to make the discussion more leveled since there’s a chance we are now talking about the same thing.
Compared with other AutoML solutions
The bad:
Less insights
In short you don’t get any model analysis like you would with Kortical for example.
Less options
As mentioned Lobe only offers image classification. Compared to Google Automl, that does that and objectregocnition, text, tabular, video and so on, it is still limited in use cases.
The good:
It’s Easy
This is the whole core selling point for Lobe and it does it perfectly. Lobe is so easy to use that it could easily be used for teaching 3rd graders.
It’s Fast
The model building is so fast that you can barely get a glass of water while training.
The average:
Quality
When I compared a model I build in Google AutoML to one I build in Lobe, Google seemed to be a bit better but not by far. That being said the Google model took me 3 hours to train vs. minutes with Lobe.
Future possibilities
For me to see Lobe.ai can go in two different directions in the future. They can either go for making a bigger part of the pipeline and let you build small apps on top of the models or they can go for more types of models such as tabular models or text classification. Both directions could be pretty interesting and whatever direction they go for I’m looking forward to testing it out.
Conclusion
In conclusion Lobe.ai is a great step forward for accessible AI and already in it’s beta it’s very impressive and surely will be the first in a new niche of AI.
It doesn’t get easier than this and with the export functionality it’s actually a good candidate for many commercial products.
Make sure you test it out, even if it’s just for fun.
Lily the barn owl reveals how birds fly in gusty winds
Scientists from the University of Bristol and the Royal Veterinary College have discovered how birds are able to fly in gusty conditions – findings that could inform the development of bio-inspired small-scale aircraft.
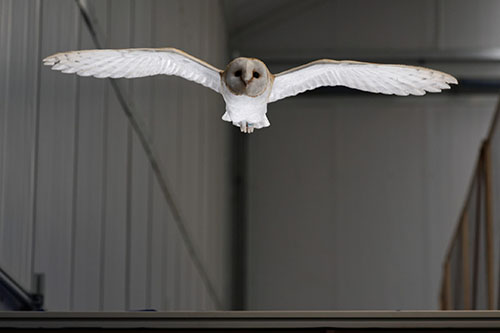
“Birds routinely fly in high winds close to buildings and terrain – often in gusts as fast as their flight speed. So the ability to cope with strong and sudden changes in wind is essential for their survival and to be able to do things like land safely and capture prey,” said Dr Shane Windsor from the Department of Aerospace Engineering at the University of Bristol.
“We know birds cope amazingly well in conditions which challenge engineered air vehicles of a similar size but, until now, we didn’t understand the mechanics behind it,” said Dr Windsor.
The study, published in Proceedings of the Royal Society B, reveals how bird wings act as a suspension system to cope with changing wind conditions. The team, which included Bristol PhD student Nicholas Durston and researchers Jialei Song and James Usherwood from Dongguan University of Technology in China and the RVC respectively, used an innovative combination of high-speed, video-based 3D surface reconstruction, computed tomography (CT) scans, and computational fluid dynamics (CFD) to understand how birds ‘reject’ gusts through wing morphing, i.e. by changing the shape and posture of their wings.
In the experiment, conducted in the Structure and Motion Laboratory at the Royal Veterinary College, the team filmed Lily, a barn owl, gliding through a range of fan-generated vertical gusts, the strongest of which was as fast as her flight speed. Lily is a trained falconry bird who is a veteran of many nature documentaries, so wasn’t fazed in the least by all the lights and cameras. “We began with very gentle gusts in case Lily had any difficulties, but soon found that – even at the highest gust speeds we could make – Lily was unperturbed; she flew straight through to get the food reward being held by her trainer, Lloyd Buck,” commented Professor Richard Bomphrey of the Royal Veterinary College.
“Lily flew through the bumpy gusts and consistently kept her head and torso amazingly stable over the trajectory, as if she was flying with a suspension system. When we analysed it, what surprised us was that the suspension-system effect wasn’t just due to aerodynamics, but benefited from the mass in her wings. For reference, each of our upper limbs is about 5% of our body weight; for a bird it’s about double, and they use that mass to effectively absorb the gust,” said joint lead-author Dr Jorn Cheney from the Royal Veterinary College.
“Perhaps most exciting is the discovery that the very fastest part of the suspension effect is built into the mechanics of the wings, so birds don’t actively need to do anything for it to work. The mechanics are very elegant. When you strike a ball at the sweetspot of a bat or racquet, your hand is not jarred because the force there cancels out. Anyone who plays a bat-and-ball sport knows how effortless this feels. A wing has a sweetspot, just like a bat. Our analysis suggests that the force of the gust acts near this sweetspot and this markedly reduces the disturbance to the body during the first fraction of a second. The process is automatic and buys just enough time for other clever stabilising processes to kick in,” added joint lead-author, Dr Jonathan Stevenson from the University of Bristol.
Dr Windsor said the next step for the research, which was funded by the European Research Council (ERC), Air Force Office of Scientific Research and the Wellcome Trust, is to develop bio-inspired suspension systems for small-scale aircraft.