Exploring the context of online images with Backstory
Open Sauce’s Eye-catching Creations? Meet Elegoo’s AI Robots, Glowing Cosplay Wings, and More, All 3D Printed
New approach allows drone swarms to autonomously navigate complex environments at high speed
ChatGPT Releases New Souped-Up ‘Agent’
ChatGPT is rolling-out a new AI agent designed to do a number of tasks on your behalf – and without your supervision.
For writers, such an agent could be programmed to thoroughly research an article, write it – and then continually auto-update the article with new research.
For an excellent, in-depth review of ChatGPT Agent, check-out Dan Shipper’s piece.
In other news and analysis on AI writing:
*Under-the-Hood: A Look at Grammarly’s Soon-to-Be-Released Email Tool: Given that AI writing/proofing pioneer Grammarly just scooped-up the email tool ‘Superhuman’ to add to its productivity suite, you may want to find out why with this video tutorial.
The video’s takeaway: Once officially available with Grammarly, Superhuman can be set-up to act as your personal assistant, auto-categorizing and routing emails for you.
If you’re looking to dig even deeper, there’s a companion video also available on this same YouTube channel, hosted by Tiago Forte.
*Google Soups-Up Search For Paid Subscribers: Those who have ponied-up hard cash for the Gemini 2.5 Pro can now use that chatbot to power Google Search’s new ‘AI Mode.’
Essentially, instead of getting access to basic AI when searching using AI Mode, those paid subscribers will be able to enhance the search with Gemini 2.5 Pro.
Being a paid subscriber will also enable you to power AI Mode searches with Gemini 2.5 Pro’s Deep Search.
*Look-Out: Here Comes the ‘AI Memory Economy:’ Writer Wyatt Benno theorizes that selling your ideas — in course or book form — is about to become so 2025.
Observes Benno: “Instead of selling a course once, experts monetize ongoing access to their evolving knowledge base through their personal, blockchain-secured vector database.
“Every new insight, every refined framework, every learned lesson gets vectorized and becomes instantly queryable by subscribers.”
*Building Your Own AI Agent: A Video How-To: General interest AI newsletter “The Neuron” has put together an excellent guide on how to design your own AI agent.
Essentially, “The Neuron” scoured YouTube for the very best videos on designing agents and put them together in a single, easy-to-understand text guide.
The best part: You won’t need to be a pro-coder to level-up your agent-building skills with this guide.
*ChatGPT Plus Users Get Meeting Recording, Transcripts, Summaries: Users of ChatGPT Plus can now use the AI to quickly record meetings – as well as generate transcripts and summaries of those meetings.
Dubbed ‘Record Mode,” the feature was previously only available to users of higher-tier, ChatGPT subscriptions.
Observes writer Lance Whitney: The AI “converts the spoken audio into a text transcript. From there, you can tell ChatGPT to analyze or summarize the content — and ask specific questions about the topics discussed.”
*Now You Can Chat-Up an AI Expert for Advice and Insight: Google is expanding its repertoire of pre-configured experts – such as Shakespeare, a top psychologist and economic analysts –that you can chat-up using your smartphone, PC, or other computerized device.
The experts ‘live’ as notebooks on Google’s NotebookLM. It’s an AI tool that enables you to store all sorts of text that you can chat with – or even transform into conversational podcasts.
Observes writer Webb Wright: “These notebooks cover subjects like science, practical travel tips, expert advice on parenting and well-being, finance and the complete works of Shakespeare.”
*Just for Fun: Three AI Agents Realize They’re All AI – Then Switch to ‘Secret Communications Mode’: Yes it’s come to this: AI has become so advanced, we’re at the point where three, AI-powered smartphones can now collaborate like human beings – and be just as inefficient.
Essentially, this video brings new meaning to the phrase: “Open the pod bay doors Hal.”
*China Dead Serious About Becoming Major AI Player: Writer Meaghan Tobin reports China is spending billions to become an AI titan.
Observes Tobin: “China is quickly closing the gap with the United States in the contest to make technologies that rival the human brain.
“This is not an accident. The Chinese government has spent a decade funneling resources toward becoming an AI superpower.”
*AI BIG PICTURE: Saudi Arabia Gears-Up to Train One Million Citizens in AI: From the Department of Not Fooling Around: Saudi Arabia has committed to getting a million of its citizens up-to-speed on AI.
How?
Starting with the country’s next academic year, students will embrace AI as part of their core training in math and computer science.
Observes writer Manal Albarakati: “The efforts to prepare the local workforce are part of the kingdom’s ‘Vision 2030’ strategy to localize technology, develop Arabic-language models — and reduce reliance on imported tools and expertise.”

Share a Link: Please consider sharing a link to https://RobotWritersAI.com from your blog, social media post, publication or emails. More links leading to RobotWritersAI.com helps everyone interested in AI-generated writing.
–Joe Dysart is editor of RobotWritersAI.com and a tech journalist with 20+ years experience. His work has appeared in 150+ publications, including The New York Times and the Financial Times of London.
The post ChatGPT Releases New Souped-Up ‘Agent’ appeared first on Robot Writers AI.
The AI Takeover: Is ChatGPT the New Office and Photoshop?
The rapid ascent of Artificial Intelligence (AI) has sparked a profound reevaluation of how we interact with technology, particularly in the realm of productivity software. At the forefront of this revolution are large language models (LLMs) like OpenAI’s ChatGPT and […]
The post The AI Takeover: Is ChatGPT the New Office and Photoshop? appeared first on TechSpective.
Livestream of RoboCup2025
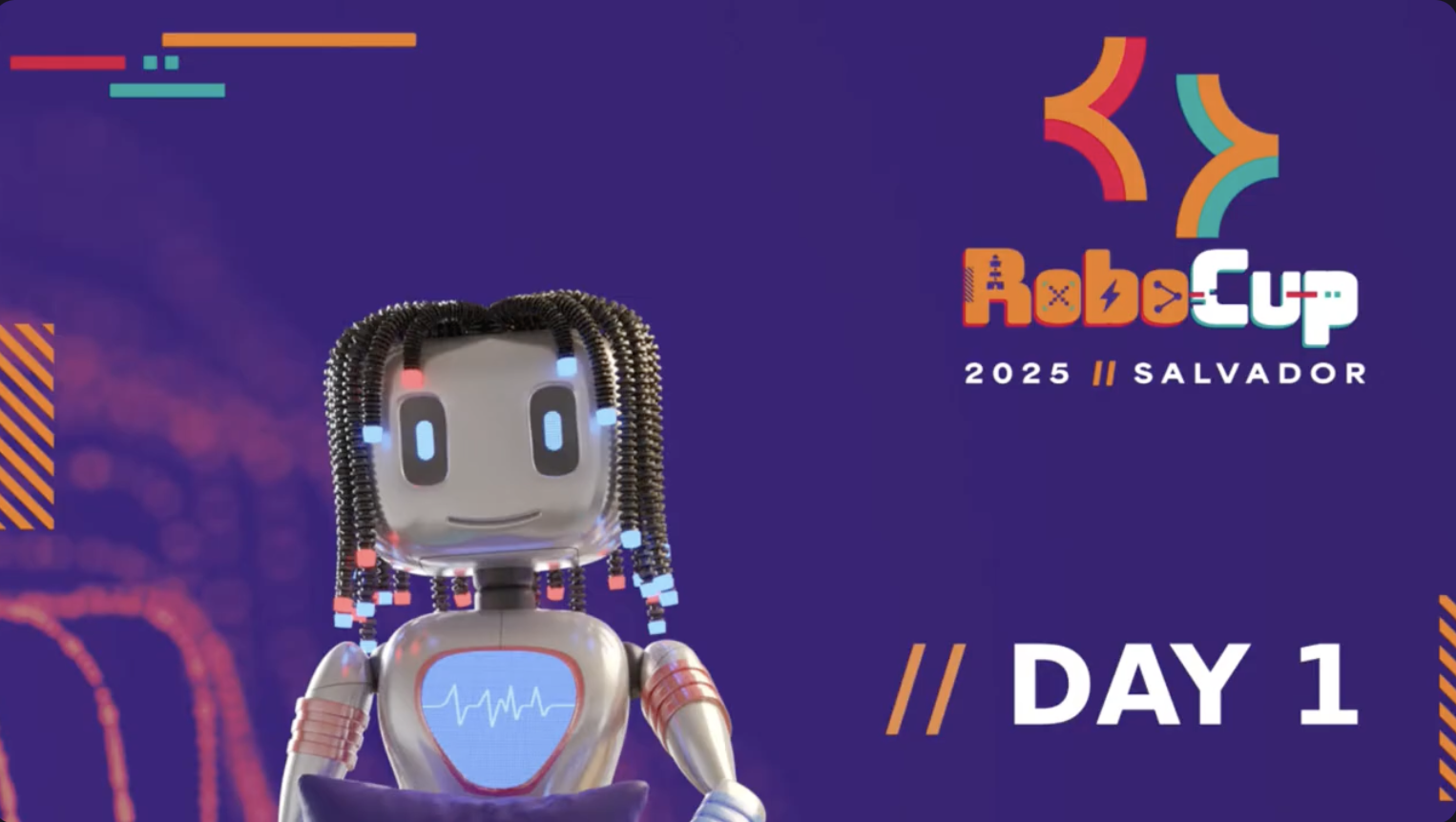
RoboCup2025 is currently taking place in Salvador, Brazil. With day one of the main competition complete, things are hotting up across the many different leagues. From soccer to rescue, from industrial to home scenarios, teams are putting their robots through their paces across a variety of tasks and matches.
If you would like to catch up on the action from the first day, you can watch the recording of the livestream below. This includes coverage of the teams competing, interviews with participants and organisers, and insights into RoboCup and the various leagues.
The livestreams for days two and three, which will feature the knockout stages of the competitions, can be found below:
The livestream for the award ceremony, will be here:
You can also find the livestream on the RoboCup Twitch channel
Read our series of interviews with RoboCup organisers, trustees, and committee members:
- Preparing for kick-off at RoboCup2025: an interview with General Chair Marco Simões
- Gearing up for RoboCupJunior: Interview with Ana Patrícia Magalhães
- RoboCupRescue: an interview with Adam Jacoff
- An interview with Nicolai Ommer: the RoboCupSoccer Small Size League
- Tackling the 3D Simulation League: an interview with Klaus Dorer and Stefan Glaser
The Unclaimed Identity: Why Autonomous AI Agents Are the Next Governance Crisis
As enterprises scale their use of artificial intelligence, a hidden governance crisis is unfolding—one that few security programs are prepared to confront: the rise of unowned AI agents. These agents are not speculative. They’re already embedded across enterprise ecosystems—provisioning access, […]
The post The Unclaimed Identity: Why Autonomous AI Agents Are the Next Governance Crisis appeared first on TechSpective.