The Robotics Engineering Job Market: Expected Growth and Changes
Sensors Expo & Conference Reveals “Best of Sensors” 2019 Award Winners
How the Combination of Robotics and AI Can Transform Industries
The Shift to Driverless Trucks Will Open Up Fulfilling Careers
Wearable robot ‘WalkON Suit’ off to Cybathlon 2020
For less than $200, engineering students built a realistic robotic fish
Sandia’s crawling robots, drones detect damage to save wind blades
Robots may care for you in old age—and your children will teach them
iRobot Introduces the Root Coding Robot Through Acquisition of Root Robotics
#289: On Design in Human-Robot Interaction, with Bilge Mutlu
In this episode, Audrow Nash interviews Bilge Mutlu, Associate Professor at the University of Wisconsin–Madison, about design-thinking in human-robot interaction. Professor Mutlu discusses design-thinking at a high-level, how design relates to science, and he speaks about the main areas of his work: the design space, the evaluation space, and how features are used within a context. He also gives advice on how to apply a design-oriented mindset.
Bilge Mutlu
Bilge Mutlu is an Associate Professor of Computer Science, Psychology, and Industrial Engineering at the University of Wisconsin–Madison. He directs the Wisconsin HCI Laboratory and organizes the WHCI+D Group. He received his PhD degree from Carnegie Mellon University‘s Human-Computer Interaction Institute.
Links
The world’s smallest autonomous racing drone
Racing team 2018-2019: Christophe De Wagter, Guido de Croon, Shuo Li, Phillipp Dürnay, Jiahao Lin, Simon Spronk
Autonomous drone racing
Drone racing is becoming a major e-sports. Enthusiasts – and now also professionals – transform drones into seriously fast racing platforms. Expert drone racers can reach speeds up to 190 km/h. They fly by looking at a first-person view (FPV) of their drone, which has a camera transmitting images mounted on the front.
In recent years, the advance in areas such as artificial intelligence, computer vision, and control has raised the question whether drones would not be able to fly faster than humans. The advantage for the drone could be that it can sense much more than the human pilot (like accelerations and rotation rates with its inertial sensors) and process all image data quicker on board of the drone. Moreover, its intelligence could be shaped purely for only one goal: racing as fast as possible.
In the quest for a fast-flying, autonomous racing drone, multiple autonomous racing drone competitions have been organized in the academic community. These “IROS” drone races (where IROS stands for one of the most well-known world-wide robotics conferences) have been held from 2016 on. Over these years, the speed of the drones has been gradually improving, with the faster drones in the competition now moving at ~2 m/s.
Smaller
Most of the autonomous racing drones are equipped with high-performance processors, with multiple, high-quality cameras and sometimes even with laser scanners. This allows these drones to use state-of-the-art solutions to visual perception, like building maps of the environment or tracking accurately how the drone is moving over time. However, it also makes the drones relatively heavy and expensive.
At the Micro Air Vehicle laboratory (MAVLab) of TU Delft, we have as aim to make light-weight and cheap autonomous racing drones. Such drones could be used by many drone racing enthusiasts to train with or fly against. If the drone becomes small enough, it could even be used for racing at home. Aiming for “small” means serious limitations to the sensors and processing that can be carried onboard. This is why in the IROS drone races we have always focused on monocular vision (a single camera) and on software algorithms for vision, state estimation, and control that are computationally highly efficient.
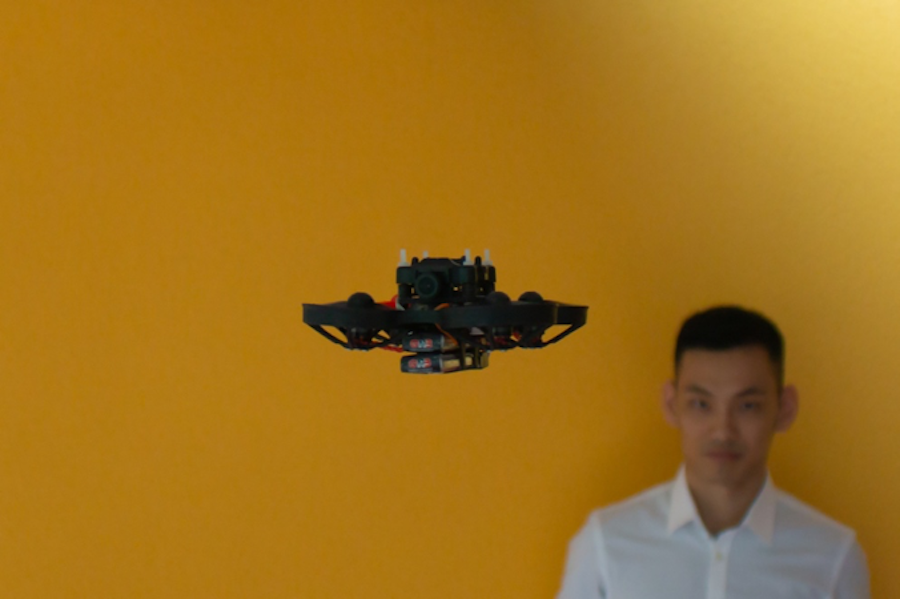
With its 72 grams and 10 cm diameter, the modified “Trashcan” drone is currently the smallest autonomous racing drone in the world. In the background, Shuo Li, PhD student at working on autonomous drone racing at the MAVLab.
A 72-gram autonomous racing drone
Here, we report on how we made a tiny autonomous racing drone fly through a racing track with on average 2 m/s, which is competitive with other, larger state-of-the-art autonomous racing drones.
The drone, which is a modified Eachine “Trashcan”, is 10 cm in diameter and weighs 72 grams. This weight includes a 17-gram JeVois smart-camera, which consists of a single, rolling shutter CMOS camera, a 4-core ARM v7 1.34 GHz processor with 256 MB RAM, and a 2-core Mali GPU. Although limited compared to the processors used on other drones, we consider it as more than powerful enough: With the algorithms we explain below, the drone actually only uses a single CPU core. The JeVois camera communicates with a 4.2gram Crazybee F4 Pro Flight Controller running Paparazzi autopilot, via the MAVLink communication protocol. Both the JeVois code and Paparazzi code is open source and available to the community.
An important characteristic of our approach to drone racing is that we do not rely on accurate, but computationally expensive methods for visual Simultaneous Localization And Mapping (SLAM) or Visual Inertial Odometry (VIO). Instead, we focus on having the drone predict its motion as good as possible with an efficient prediction model and correct any drift of the model with vision-based gate detections.
Prediction
A typical prediction model would involve the integration of the accelerometer readings. However, on small drones the Inertial Measurement Unit (IMU) is subject to a lot of vibration, leading to noisier accelerometer readings. Integrating such noisy measurements quickly leads to an enormous drift in both the velocity and position estimates of the drone. Therefore, we have opted for a simpler solution, in which the IMU is only used to determine the attitude of the drone. This attitude can then be used to predict the forward acceleration, as illustrated in the figure below. If one assumes the drone to fly at a constant height, the force in the z-direction has to equal the gravity force. Given a specific pitch angle, this relation leads to a specific forward force due to the thrust. The prediction model then updates the velocity based on this predicted forward force and the expected drag force given the estimated velocity.
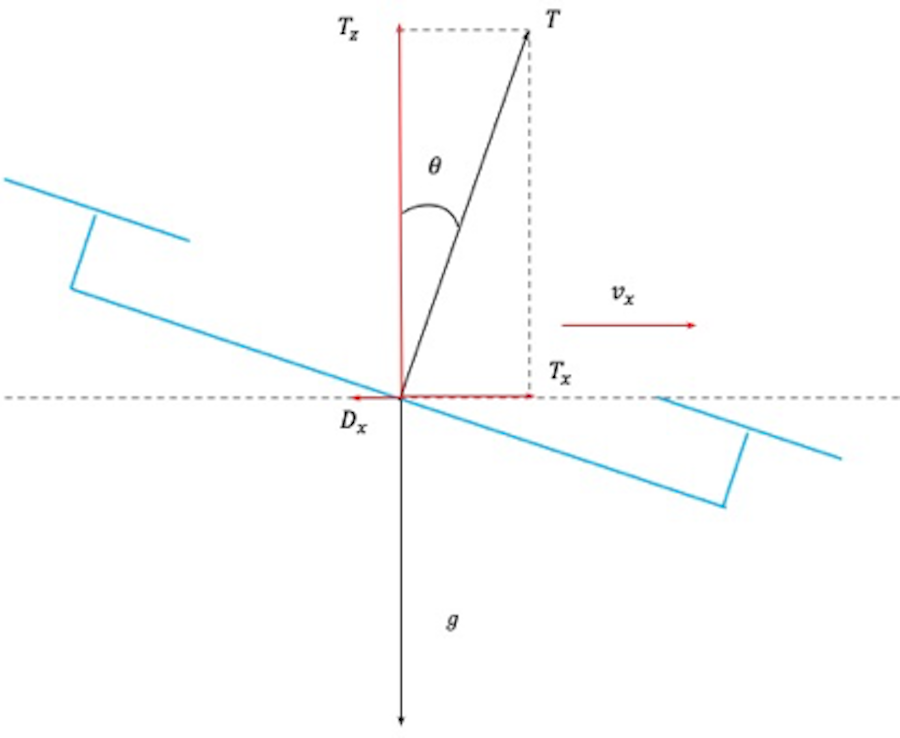
Vision-based corrections
The prediction model is corrected with the help of vision-based position measurements. First, a snake-gate algorithm is used to detect the colored gate in the image. This algorithm is extremely efficient, as it only processes a small portion of the image’s pixels. It samples random image locations and when it finds the right color, it starts following it around to determine the shape. After a detection, the known size of the gate is used to determine the drone’s relative position to the gate (see the figure below). This is a standard perspective-N-point problem. The output of this process is a relative position to a gate. Subsequently, we figure out which gate on the racing track is most likely in our view, and transform the relative position to the gate to a global position measurement. Since our vision process often outputs quite precise position estimates but sometimes also produces significant outliers, we do not use a Kalman filter but a Moving Horizon Estimator for the state estimation. This leads to much more robust position and velocity estimates in the presence of outliers.
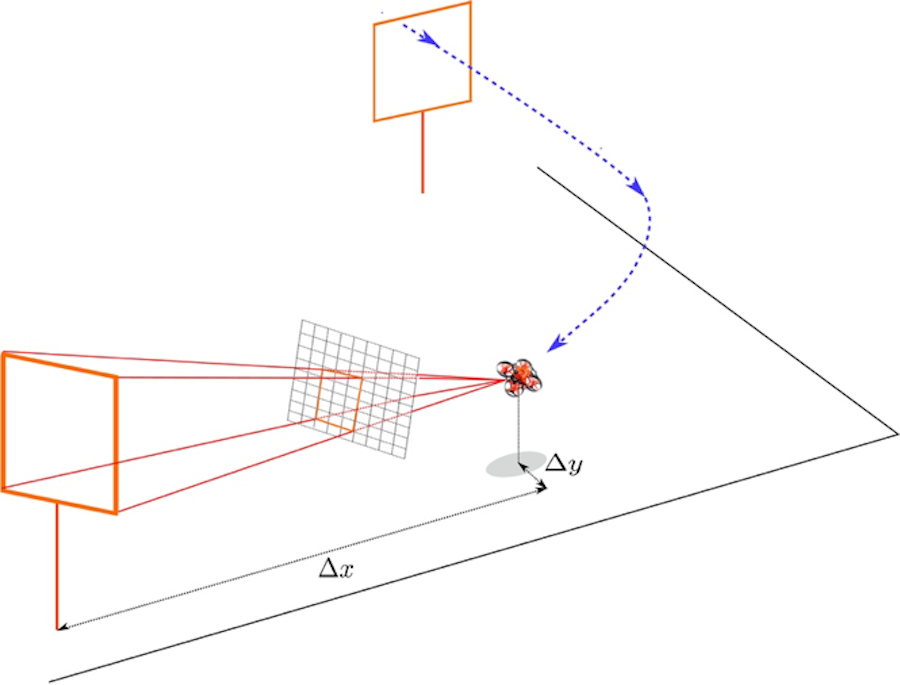
Racing performance and future steps
The drone used the newly developed algorithms to race along a 4-gate race track in TU Delft’s Cyberzoo. It can fly multiple laps at an average speed of 2 m/s, which is competitive with larger, state-of-the-art autonomous racing drones (see the video at the top). Thanks to the central role of gate detections in the drone’s algorithms, the drone can cope with moderate displacements of the gates.
Possible future directions of research are to make the drone smaller and fly faster. In principle, being small is an advantage, since the gates are relatively bigger. This allows the drone to choose its trajectory more freely than a big drone, which may allow for faster trajectories. In order to better exploit this characteristic, we would have to fit optimal control algorithms into the onboard processing. Moreover, we want to make the vision algorithms more robust – as the current color-based snake gate algorithm is quite dependent on lighting conditions. An obvious option here is to start using deep neural networks, which would have to fit within the dual-core Mali GPU on the JeVois.
Arxiv article: Visual Model-predictive Localization for Computationally Efficient Autonomous Racing of a 72-gram Drone, Shuo Li, Erik van der Horst, Philipp Duernay, Christophe De Wagter, Guido C.H.E. de Croon.
The little robot that could
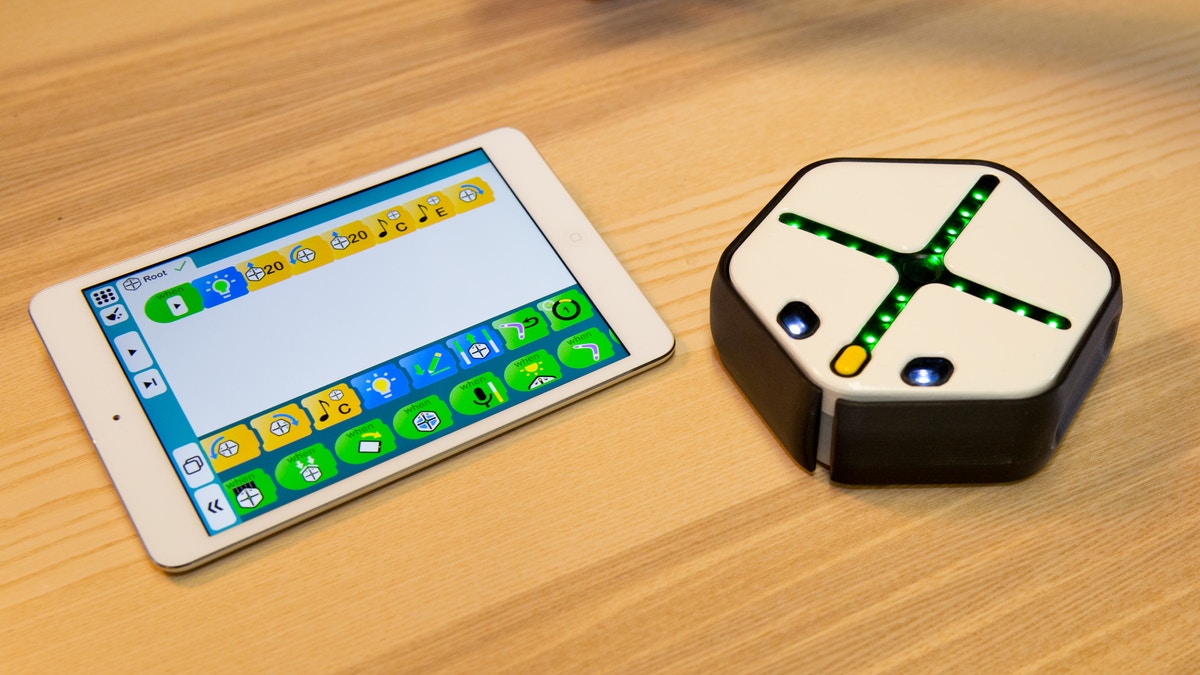
iRobot Corp. announced its acquisition of Root Robotics, Inc., whose educational Root coding robot got its start as a summer research project at the Wyss Institute for Biologically Inspired Engineering in 2011 and subsequently developed into a robust learning tool that is being used in over 500 schools to teach children between the ages of four and twelve how to code in an engaging, intuitive way. iRobot plans to incorporate the Root robot into its growing portfolio of educational robot products, and continue the work of scaling up production and expanding Root’s programming content that began when Root Robotics was founded by former Wyss Institute members in 2017.
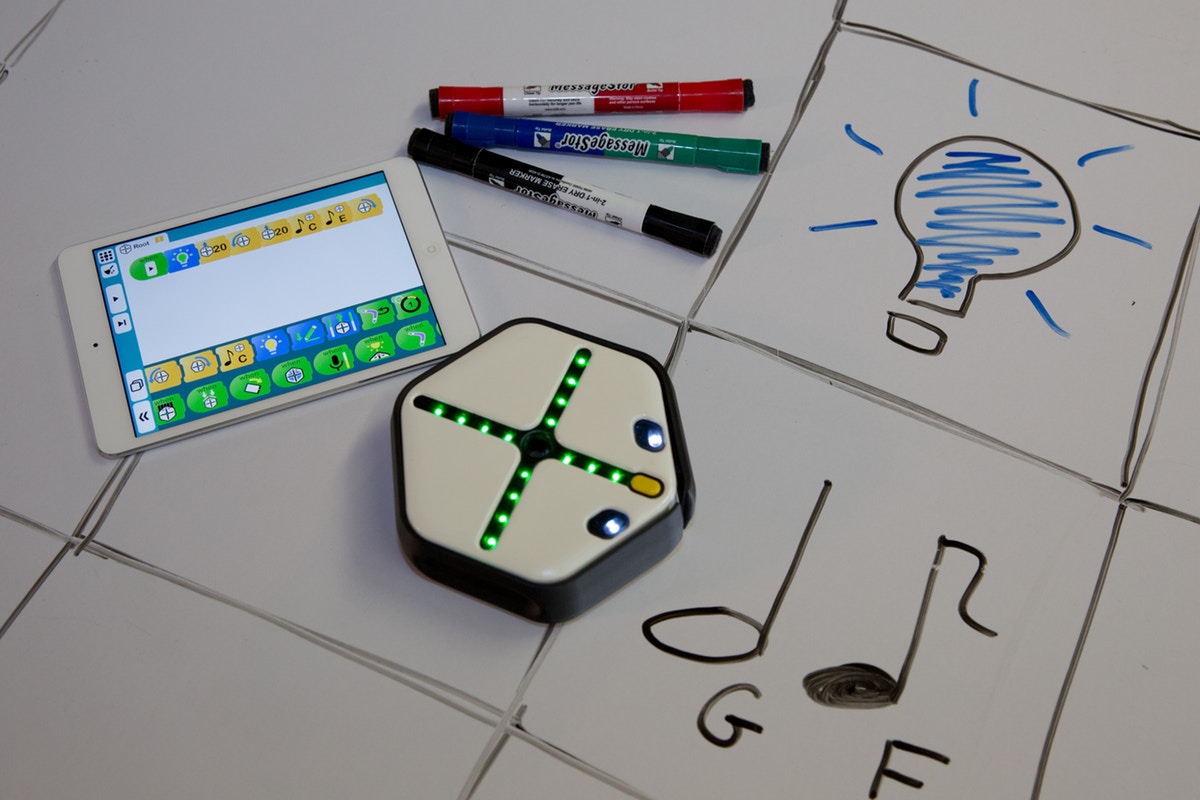
“We’re honored that we got to see a Wyss Institute technology go from its earliest stages to where we are today, with the opportunity to make a gigantic impact on the world,” said Zivthan Dubrovsky, former Bioinspired Robotics Platform Lead at the Wyss Institute and co-founder of Root Robotics who is now the General Manager of Educational Robots at iRobot. “We’re excited to see how this new chapter in Root’s story can further amplify our mission of making STEM education accessible to students of any age in any classroom around the world.”
Root began in the lab of Wyss Core Faculty Member and Bioinspired Robotics Platform co-lead Radhika Nagpal, Ph.D., who was investigating the idea of robots that could climb metal structures using magnetic wheels. “Most whiteboards in classrooms are backed with metal, so I thought it would be wonderful if a robot could automatically erase the whiteboard as I was teaching – ironically, we referred to it as a ‘Roomba® for whiteboards,’ because many aspects were directly inspired by iRobot’s Roomba at the time,” said Nagpal, who is also the Fred Kavli Professor of Computer Science at Harvard’s John A. Paulson School of Engineering and Applied Sciences (SEAS). “Once we had a working prototype, the educational potential of this robot was immediately obvious. If it could be programmed to detect ink, navigate to it, and erase it, then it could be used to teach students about coding algorithms of increasing complexity.”
That prototype was largely built by Raphael Cherney, first as a Research Engineer in Nagpal’s group at Harvard in 2011, and then beginning in 2013 when he was hired to work on developing Root full-time along with Dubrovsky and other members of the Wyss Institute. “When Raphael and Radhika pitched me the idea of Root, I fell in love with it immediately,” said Dubrovsky. “My three daughters were all very young at the time and I wanted them to have exposure to STEM concepts like coding and engineering, but I was frustrated by the lack of educational systems that were designed for children their age. The idea of being able to create that for them was really what motivated me to throw all my weight behind the project.”
Under Cherney and Dubrovsky’s leadership, Root’s repertoire expanded to include drawing shapes on the whiteboard as it wheeled around, navigating through obstacles drawn on the whiteboard, playing music, and more. The team also developed Root’s coding interface, which has three levels of increasing complexity that are designed to help students from preschool to high school easily grasp the concepts of programming and use them to create their own projects. “The tangible nature of a robot really brings the code to life, because the robot is ‘real’ in a way that code isn’t – you can watch it physically carrying out the instructions that you’ve programmed into it,” said Cherney, who co-founded Root Robotics and is now a Principal Systems Engineer at iRobot. “It helps turn coding into a social activity, especially for kids, as they learn to work in teams and see coding as a fun and natural thing to do.”
Over the next three years the team iterated on Root’s prototype and began testing it in classrooms in and around Boston, getting feedback from students and teachers to get the robot closer to its production-ready form. “Robots are very hard to build, and the support we had from the Wyss Institute let us do it right, instead of just fast,” said Cherney. “We were able to develop Root from a prototype to a product that worked in schools and was doing what we envisioned, and the whole process was much smoother than it would have been if we had just been a team working in a garage.”
By 2016, they felt ready for commercialization. They ran a Kickstarter® campaign as a market test to see if they had a viable consumer business, and raised nearly $400,000 from almost 2,000 backers, far exceeding their target of $250,000. Buoyed by this vote of confidence from potential customers, Dubrovsky and Cherney left the Wyss Institute in the summer of 2017 to co-found Root Robotics with Nagpal serving as Scientific Advisor and $2.5 million in seed funds, and a license from Harvard’s Office of Technology Development. While most of their time at the Wyss Institute was spent getting the robot right, the company focused on getting the content of Root’s programming app up to par, setting up a classroom in their office and inviting students to come try out the robot, then updating their content with insights learned from those experiences.
Once they achieved their vision for three different levels of programming targeting students of different ages, they shipped Root robots to their Kickstarter backers and made it available for purchase on their website in September 2018. Since then, over a million coding projects have been run on the Root app. “What’s been most rewarding for me personally is seeing my kids take Root to their classrooms and show their teachers and their peers what they’ve been able to make a robot do. Getting to see them problem-solve and iterate and then achieve something they’re proud of is priceless,” said Dubrovsky. “I’ve been pleasantly surprised by seeing people come up with new things to do with the robot that we never thought of,” added Cherney. “The way it seems to immediately unlock creativity is beautiful and inspiring.”
The Root robot has tremendous value as a tool for teaching students not only coding, but also concepts of AI, engineering and autonomous robots, all of which are very important for our future.
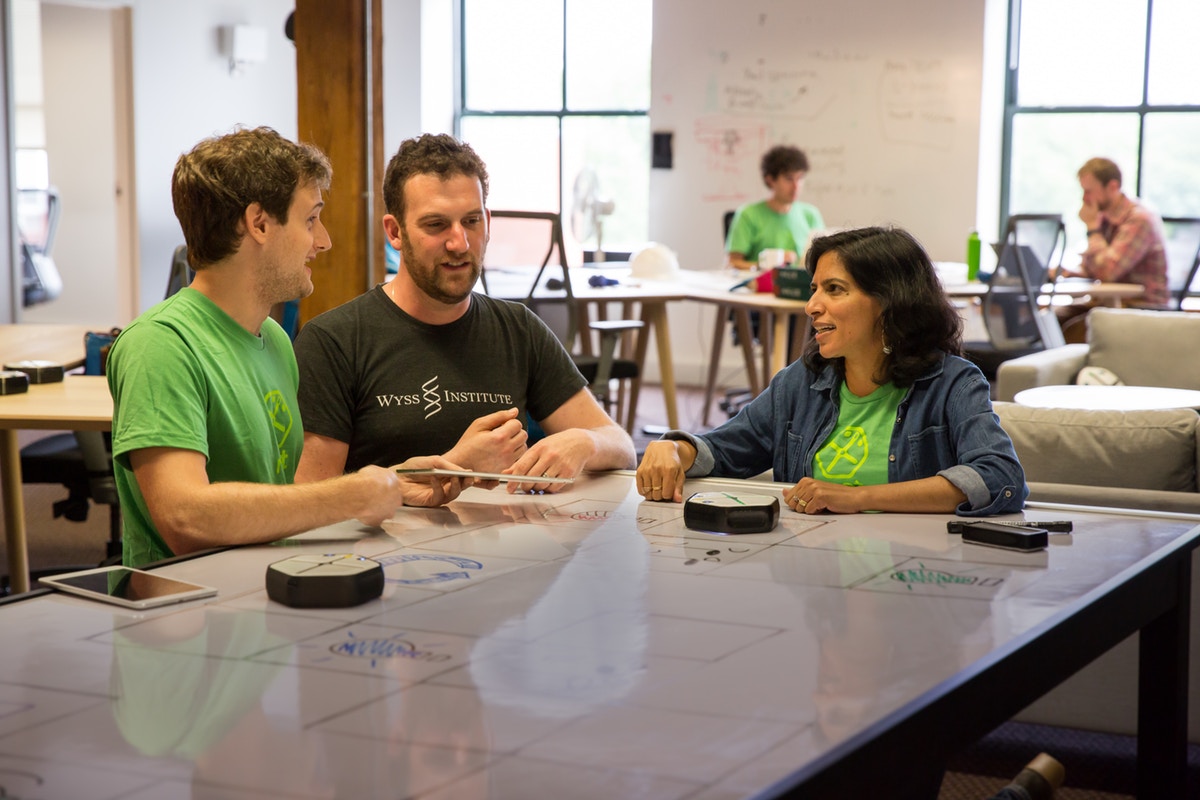
“One of the things that really attracted us to Root was that it was designed as an education product from the ground up, which fits perfectly with our own deep passion for using robots as a way of turbo charging STEM education,” said Colin Angle, chairman and CEO of iRobot. “The Root robot has tremendous value as a tool for teaching students not only coding, but also concepts of AI, engineering and autonomous robots, all of which are very important for our future.”
Nagpal is still sometimes floored by the fact that what started as an idea for a simple whiteboard-erasing robot ended up developing into such a robust teaching tool. “Without the Wyss Institute, I would not have even thought to try and commercialize this idea,” she said. “It supported an amazing team of engineers in creating and testing Root over several years, which allowed us to be able to raise the funds to launch the company with a product that was so well-developed that it now has the potential to really scale up and make a big difference in the world.”
“Root Robotics is one of the great success stories to come out of the Wyss Institute, partially because of how quickly the team recognized its potential impact and focused on de-risking it both technically and commercially,” said Wyss Founding Director Donald Ingber, M.D., Ph.D. “It was fantastic to see Root take root at the Institute, and we are immensely proud of them and their ability to develop a technology that can truly bring about positive change in our world by targeting children who are the creators and visionaries of tomorrow.” Ingber is also the Judah Folkman Professor of Vascular Biology at Harvard Medical School and the Vascular Biology Program at Boston Children’s Hospital, as well as Professor of Bioengineering at SEAS.
Spotting objects amid clutter
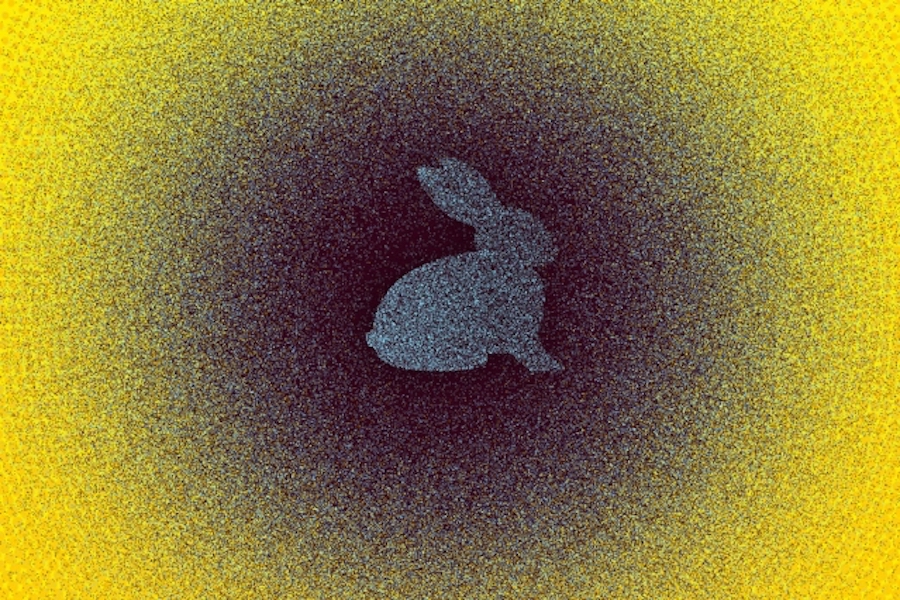
Image: Christine Daniloff, MIT
A new MIT-developed technique enables robots to quickly identify objects hidden in a three-dimensional cloud of data, reminiscent of how some people can make sense of a densely patterned “Magic Eye” image if they observe it in just the right way.
Robots typically “see” their environment through sensors that collect and translate a visual scene into a matrix of dots. Think of the world of, well, “The Matrix,” except that the 1s and 0s seen by the fictional character Neo are replaced by dots — lots of dots — whose patterns and densities outline the objects in a particular scene.
Conventional techniques that try to pick out objects from such clouds of dots, or point clouds, can do so with either speed or accuracy, but not both.
With their new technique, the researchers say a robot can accurately pick out an object, such as a small animal, that is otherwise obscured within a dense cloud of dots, within seconds of receiving the visual data. The team says the technique can be used to improve a host of situations in which machine perception must be both speedy and accurate, including driverless cars and robotic assistants in the factory and the home.
“The surprising thing about this work is, if I ask you to find a bunny in this cloud of thousands of points, there’s no way you could do that,” says Luca Carlone, assistant professor of aeronautics and astronautics and a member of MIT’s Laboratory for Information and Decision Systems (LIDS). “But our algorithm is able to see the object through all this clutter. So we’re getting to a level of superhuman performance in localizing objects.”
Carlone and graduate student Heng Yang will present details of the technique later this month at the Robotics: Science and Systems conference in Germany.
“Failing without knowing”
Robots currently attempt to identify objects in a point cloud by comparing a template object — a 3-D dot representation of an object, such as a rabbit — with a point cloud representation of the real world that may contain that object. The template image includes “features,” or collections of dots that indicate characteristic curvatures or angles of that object, such the bunny’s ear or tail. Existing algorithms first extract similar features from the real-life point cloud, then attempt to match those features and the template’s features, and ultimately rotate and align the features to the template to determine if the point cloud contains the object in question.
But the point cloud data that streams into a robot’s sensor invariably includes errors, in the form of dots that are in the wrong position or incorrectly spaced, which can significantly confuse the process of feature extraction and matching. As a consequence, robots can make a huge number of wrong associations, or what researchers call “outliers” between point clouds, and ultimately misidentify objects or miss them entirely.
Carlone says state-of-the-art algorithms are able to sift the bad associations from the good once features have been matched, but they do so in “exponential time,” meaning that even a cluster of processing-heavy computers, sifting through dense point cloud data with existing algorithms, would not be able to solve the problem in a reasonable time. Such techniques, while accurate, are impractical for analyzing larger, real-life datasets containing dense point clouds.
Other algorithms that can quickly identify features and associations do so hastily, creating a huge number of outliers or misdetections in the process, without being aware of these errors.
“That’s terrible if this is running on a self-driving car, or any safety-critical application,” Carlone says. “Failing without knowing you’re failing is the worst thing an algorithm can do.”
A relaxed view
Yang and Carlone instead devised a technique that prunes away outliers in “polynomial time,” meaning that it can do so quickly, even for increasingly dense clouds of dots. The technique can thus quickly and accurately identify objects hidden in cluttered scenes.
The MIT-developed technique quickly and smoothly matches objects to those hidden in dense point clouds (left), versus existing techniques (right) that produce incorrect, disjointed matches. Gif: Courtesy of the researchers
The researchers first used conventional techniques to extract features of a template object from a point cloud. They then developed a three-step process to match the size, position, and orientation of the object in a point cloud with the template object, while simultaneously identifying good from bad feature associations.
The team developed an “adaptive voting scheme” algorithm to prune outliers and match an object’s size and position. For size, the algorithm makes associations between template and point cloud features, then compares the relative distance between features in a template and corresponding features in the point cloud. If, say, the distance between two features in the point cloud is five times that of the corresponding points in the template, the algorithm assigns a “vote” to the hypothesis that the object is five times larger than the template object.
The algorithm does this for every feature association. Then, the algorithm selects those associations that fall under the size hypothesis with the most votes, and identifies those as the correct associations, while pruning away the others. In this way, the technique simultaneously reveals the correct associations and the relative size of the object represented by those associations. The same process is used to determine the object’s position.
The researchers developed a separate algorithm for rotation, which finds the orientation of the template object in three-dimensional space.
To do this is an incredibly tricky computational task. Imagine holding a mug and trying to tilt it just so, to match a blurry image of something that might be that same mug. There are any number of angles you could tilt that mug, and each of those angles has a certain likelihood of matching the blurry image.
Existing techniques handle this problem by considering each possible tilt or rotation of the object as a “cost” — the lower the cost, the more likely that that rotation creates an accurate match between features. Each rotation and associated cost is represented in a topographic map of sorts, made up of multiple hills and valleys, with lower elevations associated with lower cost.
But Carlone says this can easily confuse an algorithm, especially if there are multiple valleys and no discernible lowest point representing the true, exact match between a particular rotation of an object and the object in a point cloud. Instead, the team developed a “convex relaxation” algorithm that simplifies the topographic map, with one single valley representing the optimal rotation. In this way, the algorithm is able to quickly identify the rotation that defines the orientation of the object in the point cloud.
With their approach, the team was able to quickly and accurately identify three different objects — a bunny, a dragon, and a Buddha — hidden in point clouds of increasing density. They were also able to identify objects in real-life scenes, including a living room, in which the algorithm quickly was able to spot a cereal box and a baseball hat.
Carlone says that because the approach is able to work in “polynomial time,” it can be easily scaled up to analyze even denser point clouds, resembling the complexity of sensor data for driverless cars, for example.
“Navigation, collaborative manufacturing, domestic robots, search and rescue, and self-driving cars is where we hope to make an impact,” Carlone says.
This research was supported in part by the Army Research Laboratory, the Office of Naval Research, and the Google Daydream Research Program.
Tackling sustainability and urbanization with AI-enabled furniture
At the turn of the twentieth century, the swelling populations of newly arrived immigrants in New York City’s Lower East Side reached a boiling point, forcing the City to pass the 1901 Tenement House Act. Recalling this legislation, New York City’s Mayor’s Office recently responded to its own modern housing crisis by enabling developers for the first time to build affordable micro-studio apartments of 400 square feet. One of the primary drivers of allocating tens of thousands of new micro-units is the adoption of innovative design and construction technologies that enable modular and flexible housing options. As Mayor de Blasio affirmed, “Housing New York 2.0 commits us to creating 25,000 affordable homes a year and 300,000 homes by 2026. Making New York a fairer city for today and for future generations depends on it.”
Urban space density is not just a New York City problem, but a world health concern. According to the United Nations, more than half of the Earth’s population currently resides in cities and this is projected to climb to close to three-quarters by 2050. In response to this alarming trend the UN drafted the 2030 Agenda for Sustainable Development. Stressing the importance of such an effort, UN Deputy Secretary Amina J. Mohammed declared, “It is clear that it is in cities where the battle for sustainability will be won or lost. Cities are the organizing mechanisms of the twenty-first century. They are where young people in all parts of the world flock to develop new skills, attain new jobs, and find opportunities in which to innovate and create their futuresThe 2030 Agenda for Sustainable Development is the most ambitious agenda ever set forth for humanity.”
Absent from the UN study is utilizing mechatronics to address the challenges of urbanization. For example, robots have been deployed on construction sites in China to rapidly print building materials. There are also a handful of companies utilizing machines to cost effectively produce modular homes with the goal of replacing mud-huts and sheet metal shanties. However, the progress of automating low-to-middle income housing has been slow going until this week. Ikea, the world’s largest furniture retailer which specializes in low cost decorating solutions, announced on Tuesday the launch of Rognan – a morphing robotic furniture system for the micro-home. Collaborating with the Swedish design powerhouse is hardware startup, Ori Living. The MIT spin-out first introduced its chameleon-changing furniture platform two years ago with an expandable wardrobe that quickly shifted from bookcase/home office to walk-in closet at the touch of a button. Today such systems can be bought through the company’s website for a price upwards of $5,000. It is expected that the partnership with IKEA will bring enormous economies of scale with the mass production of its products.
The first markets targeted by IKEA next year for Rognan are the cramped neighborhoods of Hong Kong and Japan, where the average citizen lives in 160 square feet. Seana Strawn, IKEA’s product developer for new innovations, explains “Instead of making the furniture smaller, we transform the furniture to the function that you need at that time When you sleep, you do not need your sofa. When you use your wardrobe, you do not need your bed etc.”
Ori founder, Hasier Larrea, elaborates on his use of machine learning to size the space to the occupants requirements. “Every floor is different, so you need a product that’s smart enough to know this, and make a map of the floor,” describes Larrea. By using sensors to create an image of the space, the robot seamlessly transforms from closet-to-bed-to-desk-to-media center. To better understand the marketability of such a system, I polled a close friend who analyzes such innovations for a large Wall Street bank. This potential customer remarked that he will wait to purchase his own Rognan until it can anticipate his living habits, automatically sense when it is time for work, play or bed.
Ori’s philosophy is enabling people to “live large in a small footprint.” Larrea professes that the only way to combat urbanization is thinking differently. As the founder exclaims, “We cannot keep designing spaces the same way we’ve been designing spaces 20 years ago, or keep using all the same furniture we were using in homes that were twice the size, or three times the size. We need to start thinking about furniture that adapts to us, and not the other way around.” Larrea’s credo can be heard in the words of former Tesla technologist Sankarshan Murthy who aims to revolutionize interior design with robotic ceiling dropping storage furniture. Murthy’s startup, Bubblebee Spaces, made news last April with the announcement of a $4.3 million funding round led by Loup Ventures. Similar to a Broadway set change, Bubblebee lowers and hoists up wooden cases on an as needed basis, complete with an iPhone or iPad controller. “Instead of square feet you start looking at real estate in volume. You are already paying for all this air and ceiling space you are not using. We unlock that for you,” brags Murthy. Ori is also working on a modern Murphy Bed that lowers from ceiling, as the company’s press release stated last November its newest product, “a bed that seamlessly lowers from the ceiling, or lifts to the ceiling to reveal a stylish sofa” all at the beckon of one’s Alexa device.
In 1912, William Murphy received his patent for the “Disappearing Bed. Today’s robotic furniture, now validated by the likes of Ikea, could be the continuation of his vision. Several years ago, MIT student Daniel Leithinger first unveiled a shape shifting table. As Leithinger reminisces, “We were inspired by those pinscreen toys where you press your hand on one end, and it shows on the other side.” While it was never intended to be commercialized, the inventor was blown away by the emails he received. “One person said we should apply it to musical interfaces and another person said it would be great to use to help blind children understand art and other things. These are things we didn’t even think about,” shares Leithinger. As Ori and Bubblebee are working diligently to replace old couch springs for new gears and actuators, the benefits of such technology are sure to go beyond just better storage as we enter the new age of the AI Home.