Jellyfish-like robots could one day clean up the world’s oceans
Miniscule device could help preserve the battery life of tiny sensors
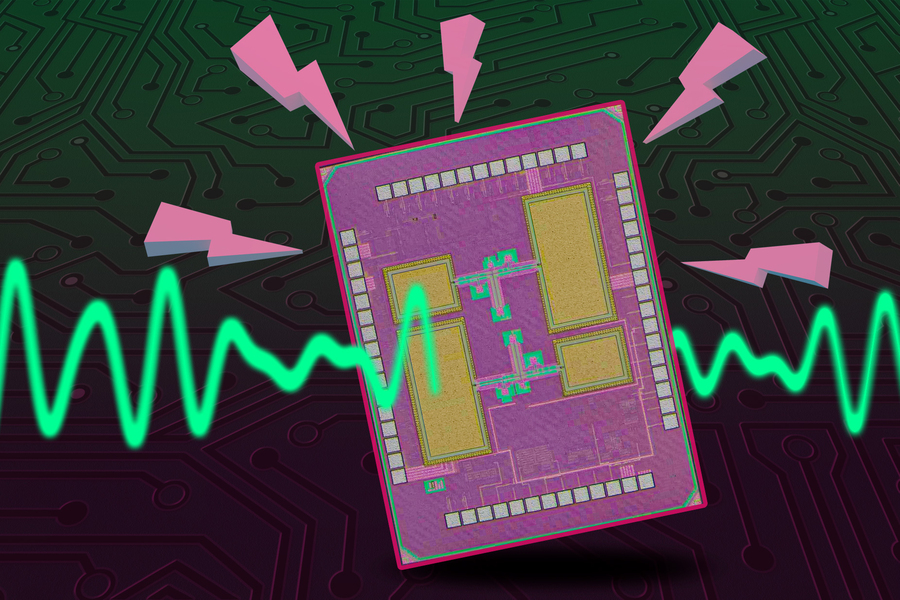
Researchers from MIT and elsewhere have built a wake-up receiver that communicates using terahertz waves, which enabled them to produce a chip more than 10 times smaller than similar devices. Their receiver, which also includes authentication to protect it from a certain type of attack, could help preserve the battery life of tiny sensors or robots. Image: Jose-Luis Olivares/MIT with figure courtesy of the researchers
By Adam Zewe | MIT News Office
Scientists are striving to develop ever-smaller internet-of-things devices, like sensors tinier than a fingertip that could make nearly any object trackable. These diminutive sensors have miniscule batteries which are often nearly impossible to replace, so engineers incorporate wake-up receivers that keep devices in low-power “sleep” mode when not in use, preserving battery life.
Researchers at MIT have developed a new wake-up receiver that is less than one-tenth the size of previous devices and consumes only a few microwatts of power. Their receiver also incorporates a low-power, built-in authentication system, which protects the device from a certain type of attack that could quickly drain its battery.
Many common types of wake-up receivers are built on the centimeter scale since their antennas must be proportional to the size of the radio waves they use to communicate. Instead, the MIT team built a receiver that utilizes terahertz waves, which are about one-tenth the length of radio waves. Their chip is barely more than 1 square millimeter in size.
They used their wake-up receiver to demonstrate effective, wireless communication with a signal source that was several meters away, showcasing a range that would enable their chip to be used in miniaturized sensors.
For instance, the wake-up receiver could be incorporated into microrobots that monitor environmental changes in areas that are either too small or hazardous for other robots to reach. Also, since the device uses terahertz waves, it could be utilized in emerging applications, such as field-deployable radio networks that work as swarms to collect localized data.
“By using terahertz frequencies, we can make an antenna that is only a few hundred micrometers on each side, which is a very small size. This means we can integrate these antennas to the chip, creating a fully integrated solution. Ultimately, this enabled us to build a very small wake-up receiver that could be attached to tiny sensors or radios,” says Eunseok Lee, an electrical engineering and computer science (EECS) graduate student and lead author of a paper on the wake-up receiver.
Lee wrote the paper with his co-advisors and senior authors Anantha Chandrakasan, dean of the MIT School of Engineering and the Vannevar Bush Professor of Electrical Engineering and Computer Science, who leads the Energy-Efficient Circuits and Systems Group, and Ruonan Han, an associate professor in EECS, who leads the Terahertz Integrated Electronics Group in the Research Laboratory of Electronics; as well as others at MIT, the Indian Institute of Science, and Boston University. The research is being presented at the IEEE Custom Integrated Circuits Conference.
Scaling down the receiver
Terahertz waves, found on the electromagnetic spectrum between microwaves and infrared light, have very high frequencies and travel much faster than radio waves. Sometimes called “pencil beams,” terahertz waves travel in a more direct path than other signals, which makes them more secure, Lee explains.
However, the waves have such high frequencies that terahertz receivers often multiply the terahertz signal by another signal to alter the frequency, a process known as frequency mixing modulation. Terahertz mixing consumes a great deal of power.
Instead, Lee and his collaborators developed a zero-power-consumption detector that can detect terahertz waves without the need for frequency mixing. The detector uses a pair of tiny transistors as antennas, which consume very little power.
Even with both antennas on the chip, their wake-up receiver was only 1.54 square millimeters in size and consumed less than 3 microwatts of power. This dual-antenna setup maximizes performance and makes it easier to read signals.
Once received, their chip amplifies a terahertz signal and then converts analog data into a digital signal for processing. This digital signal carries a token, which is a string of bits (0s and 1s). If the token corresponds to the wake-up receiver’s token, it will activate the device.
Ramping up security
In most wake-up receivers, the same token is reused multiple times, so an eavesdropping attacker could figure out what it is. Then the hacker could send a signal that would activate the device over and over again, using what is called a denial-of-sleep attack.
“With a wake-up receiver, the lifetime of a device could be improved from one day to one month, for instance, but an attacker could use a denial-of-sleep attack to drain that entire battery life in even less than a day. That is why we put authentication into our wake-up receiver,” he explains.
They added an authentication block that utilizes an algorithm to randomize the device’s token each time, using a key that is shared with trusted senders. This key acts like a password — if a sender knows the password, they can send a signal with the right token. The researchers do this using a technique known as lightweight cryptography, which ensures the entire authentication process only consumes a few extra nanowatts of power.
They tested their device by sending terahertz signals to the wake-up receiver as they increased the distance between the chip and the terahertz source. In this way, they tested the sensitivity of their receiver — the minimum signal power needed for the device to successfully detect a signal. Signals that travel farther have less power.
“We achieved 5- to 10-meter longer distance demonstrations than others, using a device with a very small size and microwatt level power consumption,” Lee says.
But to be most effective, terahertz waves need to hit the detector dead-on. If the chip is at an angle, some of the signal will be lost. So, the researchers paired their device with a terahertz beam-steerable array, recently developed by the Han group, to precisely direct the terahertz waves. Using this technique, communication could be sent to multiple chips with minimal signal loss.
In the future, Lee and his collaborators want to tackle this problem of signal degradation. If they can find a way to maintain signal strength when receiver chips move or tilt slightly, they could increase the performance of these devices. They also want to demonstrate their wake-up receiver in very small sensors and fine-tune the technology for use in real-world devices.
“We have developed a rich technology portfolio for future millimeter-sized sensing, tagging, and authentication platforms, including terahertz backscattering, energy harvesting, and electrical beam steering and focusing. Now, this portfolio is more complete with Eunseok’s first-ever terahertz wake-up receiver, which is critical to save the extremely limited energy available on those mini platforms,” Han says.
Additional co-authors include Muhammad Ibrahim Wasiq Khan PhD ’22; Xibi Chen, an EECS graduate student; Ustav Banerjee PhD ’21, an assistant professor at the Indian Institute of Science; Nathan Monroe PhD ’22; and Rabia Tugce Yazicigil, an assistant professor of electrical and computer engineering at Boston University.
Enabling AMR Customer Success Through Inductive Charging Technology
How a horse whisperer can help engineers build better robots
Researchers develop ultra-tunable bistable structures for universal robotic applications
Grasping the future with a robotic arm-hand combo
Record-Breaking Crowds Celebrate Youth Robotics Teams & STEM Innovation at FIRST® Championship in Houston
How can we build human values into AI?
How can we build human values into AI?
Grad student helps design ‘artificial muscles’ you can toss in the compost bin
Drones navigate unseen environments with liquid neural networks
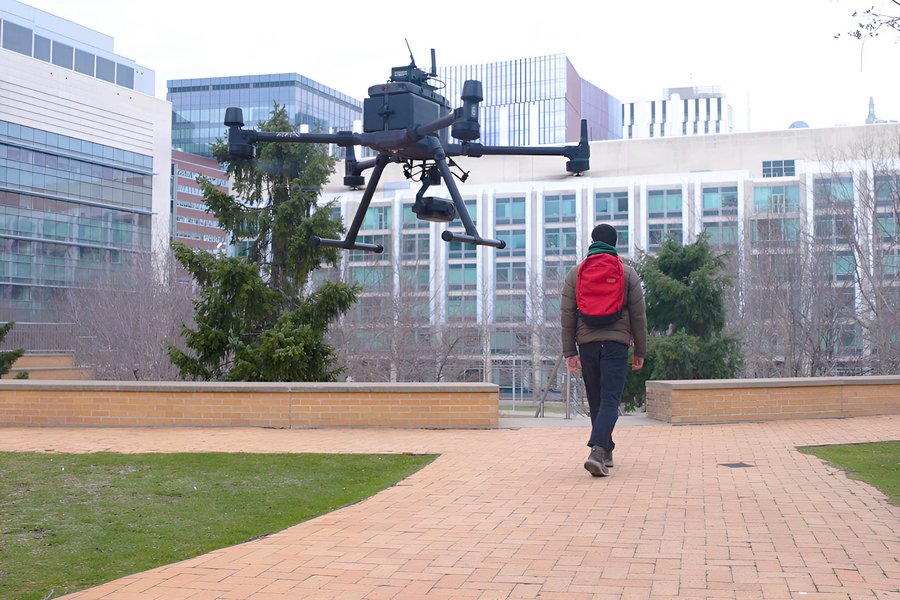
Makram Chahine, a PhD student in electrical engineering and computer science and an MIT CSAIL affiliate, leads a drone used to test liquid neural networks. Photo: Mike Grimmett/MIT CSAIL
By Rachel Gordon | MIT CSAIL
In the vast, expansive skies where birds once ruled supreme, a new crop of aviators is taking flight. These pioneers of the air are not living creatures, but rather a product of deliberate innovation: drones. But these aren’t your typical flying bots, humming around like mechanical bees. Rather, they’re avian-inspired marvels that soar through the sky, guided by liquid neural networks to navigate ever-changing and unseen environments with precision and ease.
Inspired by the adaptable nature of organic brains, researchers from MIT’s Computer Science and Artificial Intelligence Laboratory (CSAIL) have introduced a method for robust flight navigation agents to master vision-based fly-to-target tasks in intricate, unfamiliar environments. The liquid neural networks, which can continuously adapt to new data inputs, showed prowess in making reliable decisions in unknown domains like forests, urban landscapes, and environments with added noise, rotation, and occlusion. These adaptable models, which outperformed many state-of-the-art counterparts in navigation tasks, could enable potential real-world drone applications like search and rescue, delivery, and wildlife monitoring.
The researchers’ recent study, published in Science Robotics, details how this new breed of agents can adapt to significant distribution shifts, a long-standing challenge in the field. The team’s new class of machine-learning algorithms, however, captures the causal structure of tasks from high-dimensional, unstructured data, such as pixel inputs from a drone-mounted camera. These networks can then extract crucial aspects of a task (i.e., understand the task at hand) and ignore irrelevant features, allowing acquired navigation skills to transfer targets seamlessly to new environments.
Drones navigate unseen environments with liquid neural networks.
“We are thrilled by the immense potential of our learning-based control approach for robots, as it lays the groundwork for solving problems that arise when training in one environment and deploying in a completely distinct environment without additional training,” says Daniela Rus, CSAIL director and the Andrew (1956) and Erna Viterbi Professor of Electrical Engineering and Computer Science at MIT. “Our experiments demonstrate that we can effectively teach a drone to locate an object in a forest during summer, and then deploy the model in winter, with vastly different surroundings, or even in urban settings, with varied tasks such as seeking and following. This adaptability is made possible by the causal underpinnings of our solutions. These flexible algorithms could one day aid in decision-making based on data streams that change over time, such as medical diagnosis and autonomous driving applications.”
A daunting challenge was at the forefront: Do machine-learning systems understand the task they are given from data when flying drones to an unlabeled object? And, would they be able to transfer their learned skill and task to new environments with drastic changes in scenery, such as flying from a forest to an urban landscape? What’s more, unlike the remarkable abilities of our biological brains, deep learning systems struggle with capturing causality, frequently over-fitting their training data and failing to adapt to new environments or changing conditions. This is especially troubling for resource-limited embedded systems, like aerial drones, that need to traverse varied environments and respond to obstacles instantaneously.
The liquid networks, in contrast, offer promising preliminary indications of their capacity to address this crucial weakness in deep learning systems. The team’s system was first trained on data collected by a human pilot, to see how they transferred learned navigation skills to new environments under drastic changes in scenery and conditions. Unlike traditional neural networks that only learn during the training phase, the liquid neural net’s parameters can change over time, making them not only interpretable, but more resilient to unexpected or noisy data.
In a series of quadrotor closed-loop control experiments, the drones underwent range tests, stress tests, target rotation and occlusion, hiking with adversaries, triangular loops between objects, and dynamic target tracking. They tracked moving targets, and executed multi-step loops between objects in never-before-seen environments, surpassing performance of other cutting-edge counterparts.
The team believes that the ability to learn from limited expert data and understand a given task while generalizing to new environments could make autonomous drone deployment more efficient, cost-effective, and reliable. Liquid neural networks, they noted, could enable autonomous air mobility drones to be used for environmental monitoring, package delivery, autonomous vehicles, and robotic assistants.
“The experimental setup presented in our work tests the reasoning capabilities of various deep learning systems in controlled and straightforward scenarios,” says MIT CSAIL Research Affiliate Ramin Hasani. “There is still so much room left for future research and development on more complex reasoning challenges for AI systems in autonomous navigation applications, which has to be tested before we can safely deploy them in our society.”
“Robust learning and performance in out-of-distribution tasks and scenarios are some of the key problems that machine learning and autonomous robotic systems have to conquer to make further inroads in society-critical applications,” says Alessio Lomuscio, professor of AI safety in the Department of Computing at Imperial College London. “In this context, the performance of liquid neural networks, a novel brain-inspired paradigm developed by the authors at MIT, reported in this study is remarkable. If these results are confirmed in other experiments, the paradigm here developed will contribute to making AI and robotic systems more reliable, robust, and efficient.”
Clearly, the sky is no longer the limit, but rather a vast playground for the boundless possibilities of these airborne marvels.
Hasani and PhD student Makram Chahine; Patrick Kao ’22, MEng ’22; and PhD student Aaron Ray SM ’21 wrote the paper with Ryan Shubert ’20, MEng ’22; MIT postdocs Mathias Lechner and Alexander Amini; and Daniela Rus.
This research was supported, in part, by Schmidt Futures, the U.S. Air Force Research Laboratory, the U.S. Air Force Artificial Intelligence Accelerator, and the Boeing Co.
Tackling the lack of specialists with innovative robotics solutions
Robot Talk Episode 45 – Francesco Giorgio-Serchi
Claire chatted to Francesco Giorgio-Serchi from the University of Edinburgh all about underwater robots, weather-proofing, and soft robotics.
Francesco Giorgio-Serchi is a Lecturer and Chancellor’s Fellow in Robotics and Autonomous Systems at the University of Edinburgh. His work encompasses the design and control of underwater vehicles for operation in extreme weather conditions. Previously he was a Research Fellow at the University of Southampton, within the Fluid-Structure-Interaction group, where he worked on the design of soft-bodied, bioinspired, aquatic vehicles. Dr. Giorgio-Serchi holds an MSc from the University of Pisa, Italy, in Marine Technologies and a PhD in Fluid Dynamics from the University of Leeds.