The Top 5 Things Every Manufacturing CIO Should Know
Robots are everywhere – improving how they communicate with people could advance human-robot collaboration
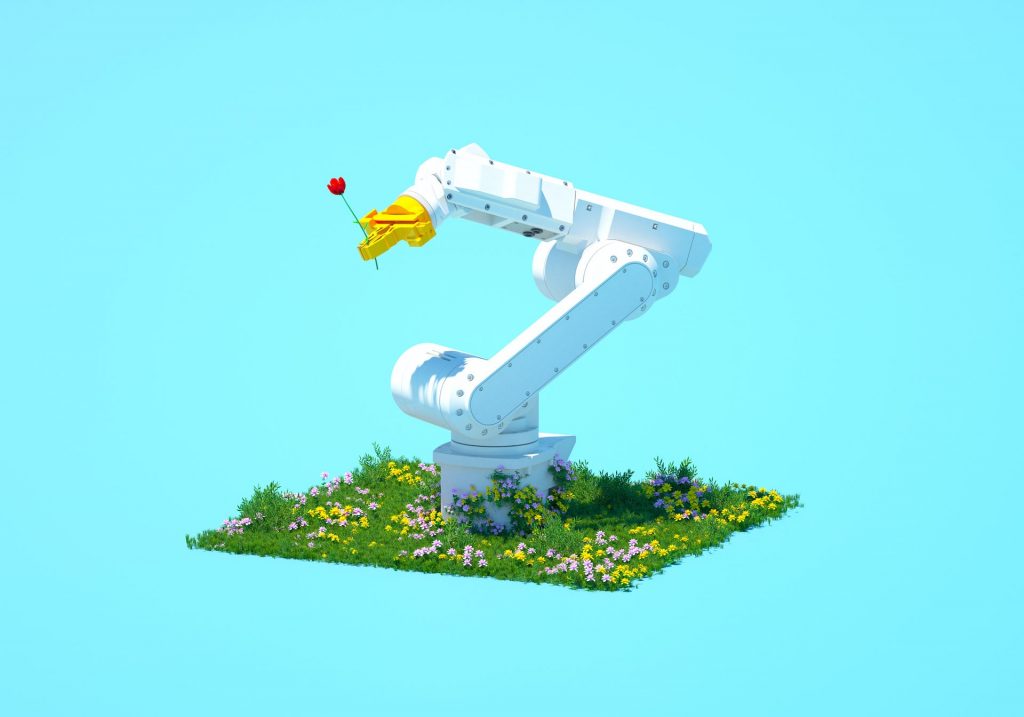
Emotionally intelligent’ robots could improve their interactions with people. Andriy Onufriyenko/Moment via Getty Images
By Ramana Vinjamuri (Assistant Professor of Computer Science and Electrical Engineering, University of Maryland, Baltimore County)
Robots are machines that can sense the environment and use that information to perform an action. You can find them nearly everywhere in industrialized societies today. There are household robots that vacuum floors and warehouse robots that pack and ship goods. Lab robots test hundreds of clinical samples a day. Education robots support teachers by acting as one-on-one tutors, assistants and discussion facilitators. And medical robotics composed of prosthetic limbs can enable someone to grasp and pick up objects with their thoughts.
Figuring out how humans and robots can collaborate to effectively carry out tasks together is a rapidly growing area of interest to the scientists and engineers that design robots as well as the people who will use them. For successful collaboration between humans and robots, communication is key.
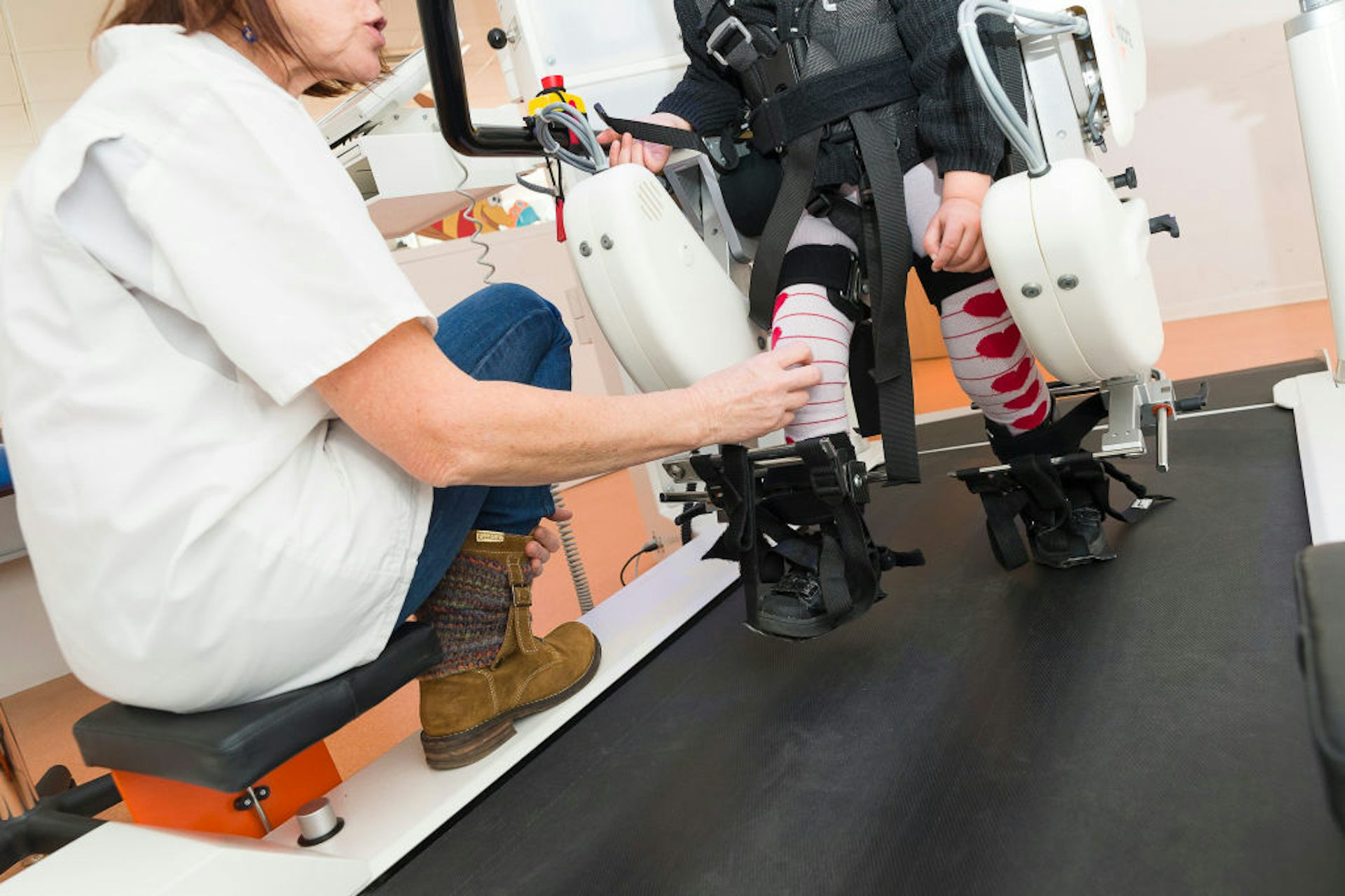
Robotics can help patients recover physical function in rehabilitation. BSIP/Universal Images Group via Getty Images
How people communicate with robots
Robots were originally designed to undertake repetitive and mundane tasks and operate exclusively in robot-only zones like factories. Robots have since advanced to work collaboratively with people with new ways to communicate with each other.
Cooperative control is one way to transmit information and messages between a robot and a person. It involves combining human abilities and decision making with robot speed, accuracy and strength to accomplish a task.
For example, robots in the agriculture industry can help farmers monitor and harvest crops. A human can control a semi-autonomous vineyard sprayer through a user interface, as opposed to manually spraying their crops or broadly spraying the entire field and risking pesticide overuse.
Robots can also support patients in physical therapy. Patients who had a stroke or spinal cord injury can use robots to practice hand grasping and assisted walking during rehabilitation.
Another form of communication, emotional intelligence perception, involves developing robots that adapt their behaviors based on social interactions with humans. In this approach, the robot detects a person’s emotions when collaborating on a task, assesses their satisfaction, then modifies and improves its execution based on this feedback.
For example, if the robot detects that a physical therapy patient is dissatisfied with a specific rehabilitation activity, it could direct the patient to an alternate activity. Facial expression and body gesture recognition ability are important design considerations for this approach. Recent advances in machine learning can help robots decipher emotional body language and better interact with and perceive humans.
Robots in rehab
Questions like how to make robotic limbs feel more natural and capable of more complex functions like typing and playing musical instruments have yet to be answered.
I am an electrical engineer who studies how the brain controls and communicates with other parts of the body, and my lab investigates in particular how the brain and hand coordinate signals between each other. Our goal is to design technologies like prosthetic and wearable robotic exoskeleton devices that could help improve function for individuals with stroke, spinal cord and traumatic brain injuries.
One approach is through brain-computer interfaces, which use brain signals to communicate between robots and humans. By accessing an individual’s brain signals and providing targeted feedback, this technology can potentially improve recovery time in stroke rehabilitation. Brain-computer interfaces may also help restore some communication abilities and physical manipulation of the environment for patients with motor neuron disorders.
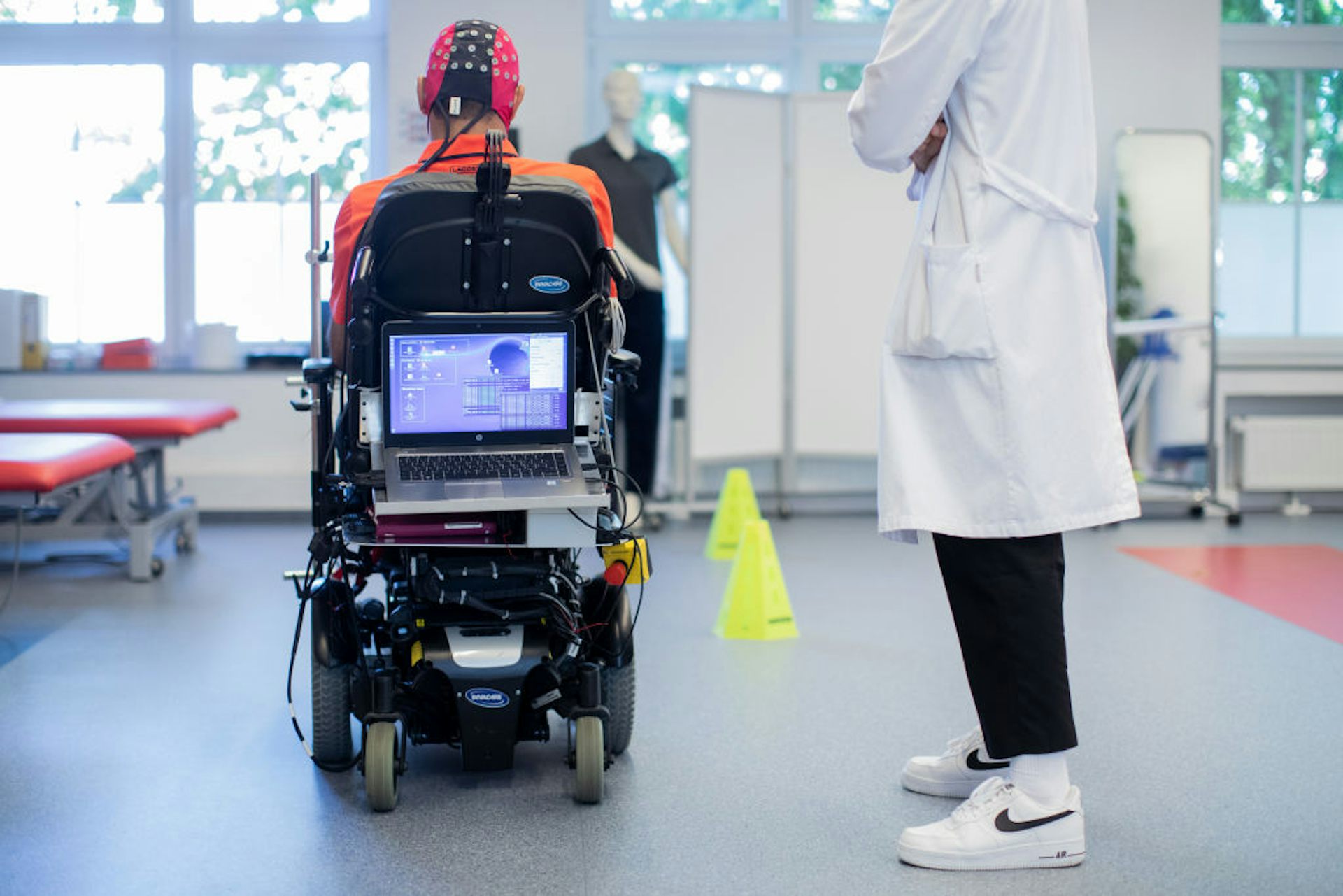
Brain-computer interfaces could allow people to control robotic arms by thought alone. Ramana Kumar Vinjamuri, CC BY-ND
The future of human-robot interaction
Effective integration of robots into human life requires balancing responsibility between people and robots, and designating clear roles for both in different environments.
As robots are increasingly working hand in hand with people, the ethical questions and challenges they pose cannot be ignored. Concerns surrounding privacy, bias and discrimination, security risks and robot morality need to be seriously investigated in order to create a more comfortable, safer and trustworthy world with robots for everyone. Scientists and engineers studying the “dark side” of human-robot interaction are developing guidelines to identify and prevent negative outcomes.
Human-robot interaction has the potential to affect every aspect of daily life. It is the collective responsibility of both the designers and the users to create a human-robot ecosystem that is safe and satisfactory for all.

Ramana Vinjamuri receives funding from National Science Foundation.
This article is republished from The Conversation under a Creative Commons license. Read the original article.
Robot assistants in the operating room promise safer surgery
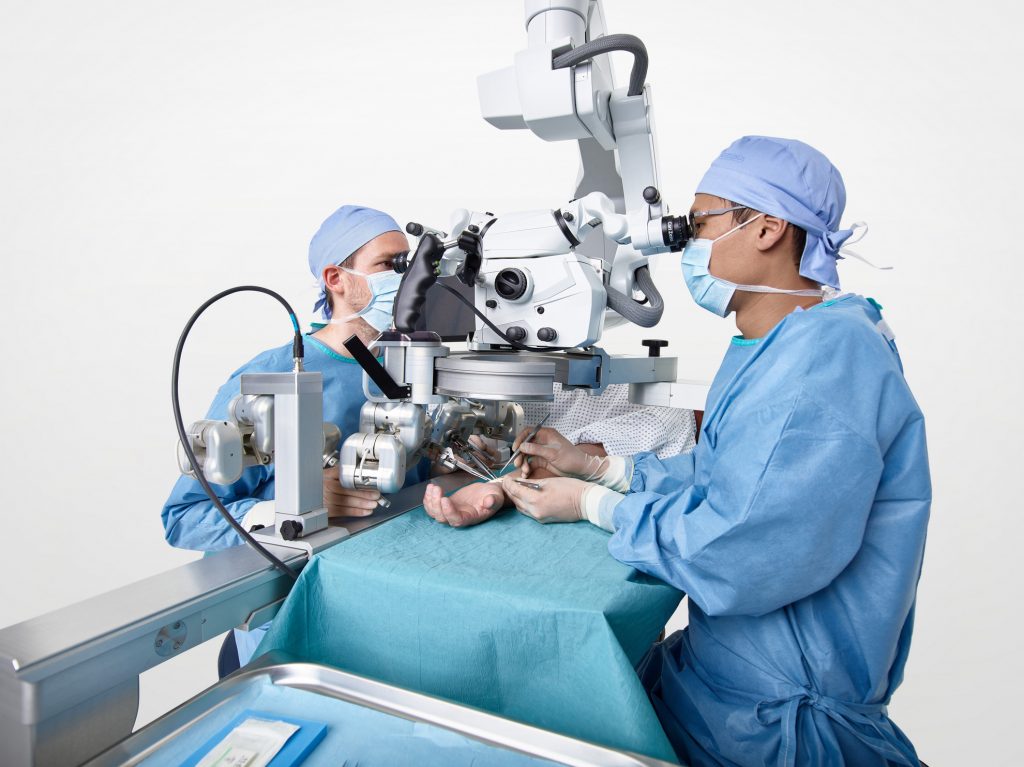
Advanced robotics can help surgeons carry out procedures where there is little margin for error. © Microsure BV, 2022
In a surgery in India, a robot scans a patient’s knee to figure out how best to carry out a joint replacement. Meanwhile, in an operating room in the Netherlands, another robot is performing highly challenging microsurgery under the control of a doctor using joysticks.
Such scenarios look set to become more common. At present, some manual operations are so difficult they can be performed by only a small number of surgeons worldwide, while others are invasive and depend on a surgeon’s specific skill.
Advanced robotics are providing tools that have the potential to enable more surgeons to carry out such operations and do so with a higher rate of success.
‘We’re entering the next revolution in medicine,’ said Sophie Cahen, chief executive officer and co-founder of Ganymed Robotics in Paris.
New knees
Cahen leads the EU-funded Ganymed project, which is developing a compact robot to make joint-replacement operations more precise, less invasive and – by extension – safer.
The initial focus is on a type of surgery called total knee arthroplasty (TKA), though Ganymed is looking to expand to other joints including the shoulder, ankle and hip.
Ageing populations and lifestyle changes are accelerating demand for such surgery, according to Cahen. Interest in Ganymed’s robot has been expressed in many quarters, including distributors in emerging economies such as India.
‘Demand is super-high because arthroplasty is driven by the age and weight of patients, which is increasing all over the world,’ Cahen said.
Arm with eyes
Ganymed’s robot will aim to perform two main functions: contactless localisation of bones and collaboration with surgeons to support joint-replacement procedures.
It comprises an arm mounted with ‘eyes’, which use advanced computer-vision-driven intelligence to examine the exact position and orientation of a patient’s anatomical structure. This avoids the need to insert invasive rods and optical trackers into the body.
“We’re entering the next revolution in medicine.”
– Sophie Cahen, Ganymed
Surgeons can then perform operations using tools such as sagittal saws – used for orthopaedic procedures – in collaboration with the robotic arm.
The ‘eyes’ aid precision by providing so-called haptic feedback, which prevents the movement of instruments beyond predefined virtual boundaries. The robot also collects data that it can process in real time and use to hone procedures further.
Ganymed has already carried out a clinical study on 100 patients of the bone-localisation technology and Cahen said it achieved the desired precision.
‘We were extremely pleased with the results – they exceeded our expectations,’ she said.
Now the firm is performing studies on the TKA procedure, with hopes that the robot will be fully available commercially by the end of 2025 and become a mainstream tool used globally.
‘We want to make it affordable and accessible, so as to democratise access to quality care and surgery,’ said Cahen.
Microscopic matters
Robots are being explored not only for orthopaedics but also for highly complex surgery at the microscopic level.
The EU-funded MEETMUSA project has been further developing what it describes as the world’s first surgical robot for microsurgery certified under the EU’s ‘CE’ regulatory regime.
Called MUSA, the small, lightweight robot is attached to a platform equipped with arms able to hold and manipulate microsurgical instruments with a high degree of precision. The platform is suspended above the patient during an operation and is controlled by the surgeon through specially adapted joysticks.
In a 2020 study, surgeons reported using MUSA to treat breast-cancer-related lymphedema – a chronic condition that commonly occurs as a side effect of cancer treatment and is characterised by a swelling of body tissues as a result of a build-up of fluids.
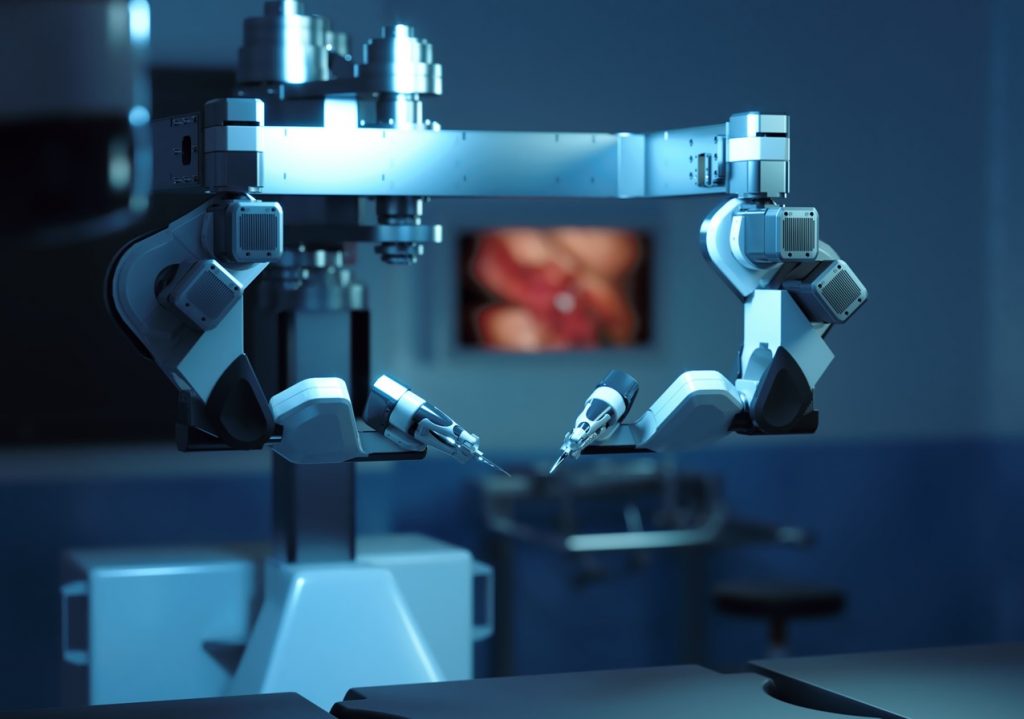
MUSA’s robotic arms. Microsure BV, 2022
To carry out the surgery, the robot successfully sutured – or connected – tiny lymph vessels measuring 0.3 to 0.8 millimetre in diameter to nearby veins in the affected area.
‘Lymphatic vessels are below 1 mm in diameter, so it requires a lot of skill to do this,’ said Tom Konert, who leads MEETMUSA and is a clinical field specialist at robot-assisted medical technology company Microsure in Eindhoven, the Netherlands. ‘But with robots, you can more easily do it. So far, with regard to the clinical outcomes, we see really nice results.’
Steady hands
When such delicate operations are conducted manually, they are affected by slight shaking in the hands, even with highly skilled surgeons, according to Konert. With the robot, this problem can be avoided.
MUSA can also significantly scale down the surgeon’s general hand movements rather than simply repeating them one-to-one, allowing for even greater accuracy than with conventional surgery.
‘When a signal is created with the joystick, we have an algorithm that will filter out the tremor,’ said Konert. ‘It downscales the movement as well. This can be by a factor-10 or 20 difference and gives the surgeon a lot of precision.’
In addition to treating lymphedema, the current version of MUSA – the second, after a previous prototype – has been used for other procedures including nerve repair and soft-tissue reconstruction of the lower leg.
Next generation
Microsure is now developing a third version of the robot, MUSA-3, which Konert expects to become the first one available on a widespread commercial basis.
“When a signal is created with the joystick, we have an algorithm that will filter out the tremor.”
– Tom Konert, MEETMUSA
This new version will have various upgrades, such as better sensors to enhance precision and improved manoeuvrability of the robot’s arms. It will also be mounted on a cart with wheels rather than a fixed table to enable easy transport within and between operating theatres.
Furthermore, the robots will be used with exoscopes – a novel high-definition digital camera system. This will allow the surgeon to view a three-dimensional screen through goggles in order to perform ‘heads-up microsurgery’ rather than the less-comfortable process of looking through a microscope.
Konert is confident that MUSA-3 will be widely used across Europe and the US before a 2029 target date.
‘We are currently finalising product development and preparing for clinical trials of MUSA-3,’ he said. ‘These studies will start in 2024, with approvals and start of commercialisation scheduled for 2025 to 2026.’
MEETMUSA is also looking into the potential of artificial intelligence (AI) to further enhance robots. However, Konert believes that the aim of AI solutions may be to guide surgeons towards their goals and support them in excelling rather than achieving completely autonomous surgery.
‘I think the surgeon will always be there in the feedback loop, but these tools will definitely help the surgeon perform at the highest level in the future,’ he said.
Research in this article was funded via the EU’s European Innovation Council (EIC).
This article was originally published in Horizon, the EU Research and Innovation magazine.
Using drones and lasers, researchers pinpoint greenhouse gas leaks
Sheet-Jamming Technology Revolutionizes Soft Robotics Grippers
Robot Talk Episode 44 – Kat Thiel
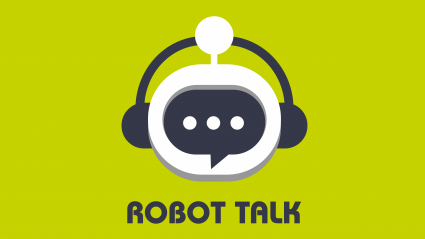
Claire chatted to Kat Thiel from Manchester Metropolitan University all about collaborative robots, micro-factories, and fashion manufacturing.
Kat Thiel is a Senior Research Associate at Manchester Metropolitan University’s Manchester Fashion Institute with a research focus on Fashion Practice Research and Industry 4.0., investigating agile cobotic tooling solutions for localised fashion manufacturing. Previously a researcher at the Royal College of Art, she worked on the Future Fashion Factory report ‘Benchmarking the Feasibility of the Micro-Factory Model for the UK Fashion Industry’ and co-produced produced the highly influential report ‘Reshoring UK Garment Manufacturing with Automation’ with Innovate UK KTN.
Kibele-PIMS Shows How Imaging Ensures Food is Reliably Sorted and Packaged
Exploring movement optimization for a cyborg cockroach with machine learning
Toward tactile and proximity sensing in large soft robots
Building a precise assistive-feeding robot that can handle any meal
Robots are everywhere—improving how they communicate with people could advance human-robot collaboration
Interactive fleet learning
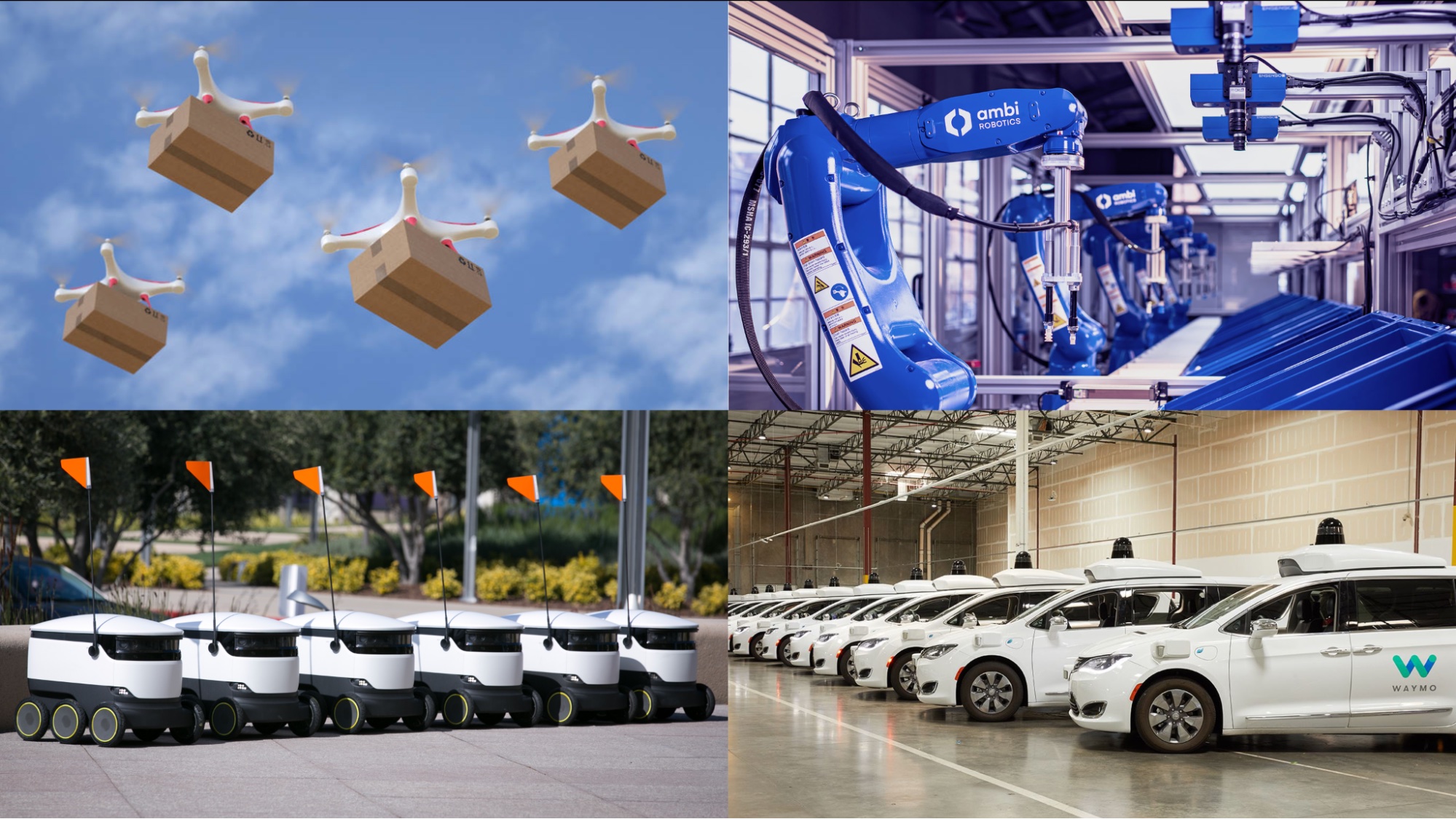
Commercial and industrial deployments of robot fleets: package delivery (top left), food delivery (bottom left), e-commerce order fulfillment at Ambi Robotics (top right), autonomous taxis at Waymo (bottom right).
In the last few years we have seen an exciting development in robotics and artificial intelligence: large fleets of robots have left the lab and entered the real world. Waymo, for example, has over 700 self-driving cars operating in Phoenix and San Francisco and is currently expanding to Los Angeles. Other industrial deployments of robot fleets include applications like e-commerce order fulfillment at Amazon and Ambi Robotics as well as food delivery at Nuro and Kiwibot.
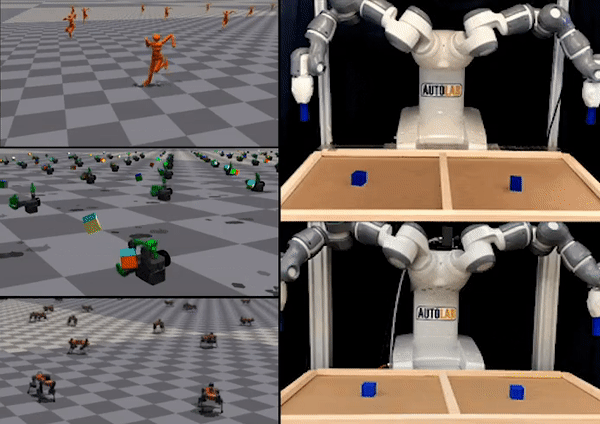
Figure 1: “Interactive Fleet Learning” (IFL) refers to robot fleets in industry and academia that fall back on human teleoperators when necessary and continually learn from them over time.
These robots use recent advances in deep learning to operate autonomously in unstructured environments. By pooling data from all robots in the fleet, the entire fleet can efficiently learn from the experience of each individual robot. Furthermore, due to advances in cloud robotics, the fleet can offload data, memory, and computation (e.g., training of large models) to the cloud via the Internet. This approach is known as “Fleet Learning,” a term popularized by Elon Musk in 2016 press releases about Tesla Autopilot and used in press communications by Toyota Research Institute, Wayve AI, and others. A robot fleet is a modern analogue of a fleet of ships, where the word fleet has an etymology tracing back to flēot (‘ship’) and flēotan (‘float’) in Old English.
Data-driven approaches like fleet learning, however, face the problem of the “long tail”: the robots inevitably encounter new scenarios and edge cases that are not represented in the dataset. Naturally, we can’t expect the future to be the same as the past! How, then, can these robotics companies ensure sufficient reliability for their services?
One answer is to fall back on remote humans over the Internet, who can interactively take control and “tele-operate” the system when the robot policy is unreliable during task execution. Teleoperation has a rich history in robotics: the world’s first robots were teleoperated during WWII to handle radioactive materials, and the Telegarden pioneered robot control over the Internet in 1994. With continual learning, the human teleoperation data from these interventions can iteratively improve the robot policy and reduce the robots’ reliance on their human supervisors over time. Rather than a discrete jump to full robot autonomy, this strategy offers a continuous alternative that approaches full autonomy over time while simultaneously enabling reliability in robot systems today.
The use of human teleoperation as a fallback mechanism is increasingly popular in modern robotics companies: Waymo calls it “fleet response,” Zoox calls it “TeleGuidance,” and Amazon calls it “continual learning.” Last year, a software platform for remote driving called Phantom Auto was recognized by Time Magazine as one of their Top 10 Inventions of 2022. And just last month, John Deere acquired SparkAI, a startup that develops software for resolving edge cases with humans in the loop.
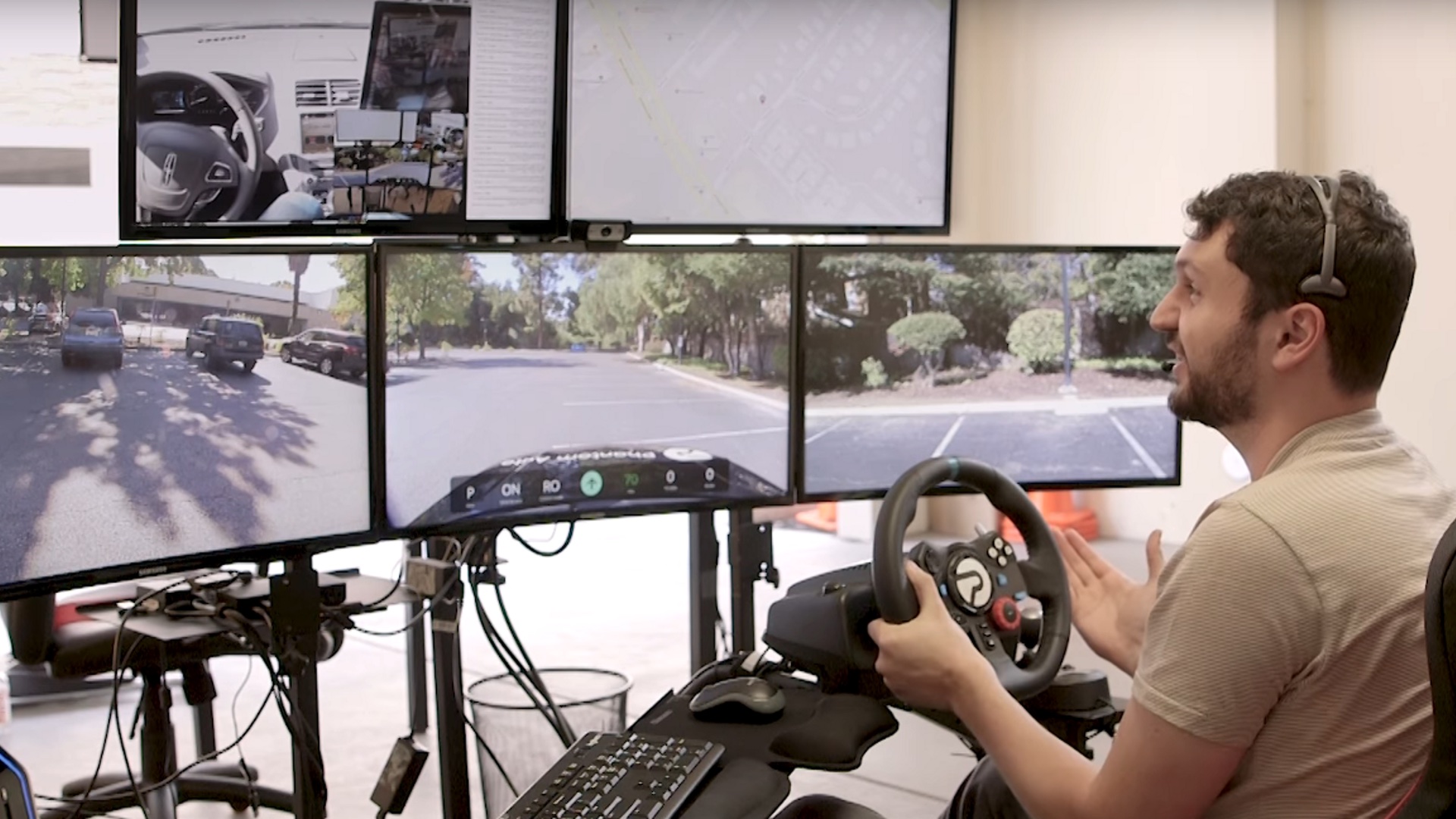
A remote human teleoperator at Phantom Auto, a software platform for enabling remote driving over the Internet.
Despite this growing trend in industry, however, there has been comparatively little focus on this topic in academia. As a result, robotics companies have had to rely on ad hoc solutions for determining when their robots should cede control. The closest analogue in academia is interactive imitation learning (IIL), a paradigm in which a robot intermittently cedes control to a human supervisor and learns from these interventions over time. There have been a number of IIL algorithms in recent years for the single-robot, single-human setting including DAgger and variants such as HG-DAgger, SafeDAgger, EnsembleDAgger, and ThriftyDAgger; nevertheless, when and how to switch between robot and human control is still an open problem. This is even less understood when the notion is generalized to robot fleets, with multiple robots and multiple human supervisors.
IFL Formalism and Algorithms
To this end, in a recent paper at the Conference on Robot Learning we introduced the paradigm of Interactive Fleet Learning (IFL), the first formalism in the literature for interactive learning with multiple robots and multiple humans. As we’ve seen that this phenomenon already occurs in industry, we can now use the phrase “interactive fleet learning” as unified terminology for robot fleet learning that falls back on human control, rather than keep track of the names of every individual corporate solution (“fleet response”, “TeleGuidance”, etc.). IFL scales up robot learning with four key components:
- On-demand supervision. Since humans cannot effectively monitor the execution of multiple robots at once and are prone to fatigue, the allocation of robots to humans in IFL is automated by some allocation policy
. Supervision is requested “on-demand” by the robots rather than placing the burden of continuous monitoring on the humans.
- Fleet supervision. On-demand supervision enables effective allocation of limited human attention to large robot fleets. IFL allows the number of robots to significantly exceed the number of humans (e.g., by a factor of 10:1 or more).
- Continual learning. Each robot in the fleet can learn from its own mistakes as well as the mistakes of the other robots, allowing the amount of required human supervision to taper off over time.
- The Internet. Thanks to mature and ever-improving Internet technology, the human supervisors do not need to be physically present. Modern computer networks enable real-time remote teleoperation at vast distances.
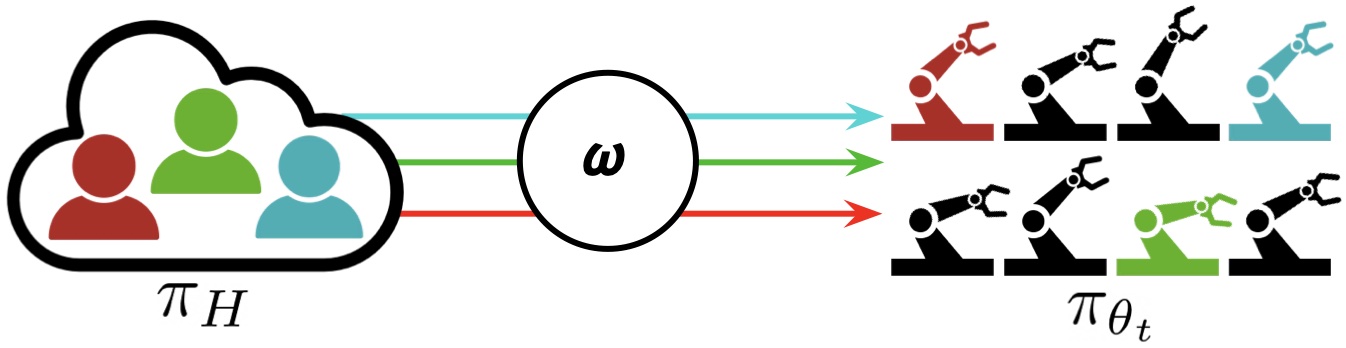
In the Interactive Fleet Learning (IFL) paradigm, M humans are allocated to the robots that need the most help in a fleet of N robots (where N can be much larger than M). The robots share policy and learn from human interventions over time.
We assume that the robots share a common control policy and that the humans share a common control policy
. We also assume that the robots operate in independent environments with identical state and action spaces (but not identical states). Unlike a robot swarm of typically low-cost robots that coordinate to achieve a common objective in a shared environment, a robot fleet simultaneously executes a shared policy in distinct parallel environments (e.g., different bins on an assembly line).
The goal in IFL is to find an optimal supervisor allocation policy , a mapping from
(the state of all robots at time t) and the shared policy
to a binary matrix that indicates which human will be assigned to which robot at time t. The IFL objective is a novel metric we call the “return on human effort” (ROHE):
where the numerator is the total reward across robots and timesteps and the denominator is the total amount of human actions across robots and timesteps. Intuitively, the ROHE measures the performance of the fleet normalized by the total human supervision required. See the paper for more of the mathematical details.
Using this formalism, we can now instantiate and compare IFL algorithms (i.e., allocation policies) in a principled way. We propose a family of IFL algorithms called Fleet-DAgger, where the policy learning algorithm is interactive imitation learning and each Fleet-DAgger algorithm is parameterized by a unique priority function that each robot in the fleet uses to assign itself a priority score. Similar to scheduling theory, higher priority robots are more likely to receive human attention. Fleet-DAgger is general enough to model a wide range of IFL algorithms, including IFL adaptations of existing single-robot, single-human IIL algorithms such as EnsembleDAgger and ThriftyDAgger. Note, however, that the IFL formalism isn’t limited to Fleet-DAgger: policy learning could be performed with a reinforcement learning algorithm like PPO, for instance.
IFL Benchmark and Experiments
To determine how to best allocate limited human attention to large robot fleets, we need to be able to empirically evaluate and compare different IFL algorithms. To this end, we introduce the IFL Benchmark, an open-source Python toolkit available on Github to facilitate the development and standardized evaluation of new IFL algorithms. We extend NVIDIA Isaac Gym, a highly optimized software library for end-to-end GPU-accelerated robot learning released in 2021, without which the simulation of hundreds or thousands of learning robots would be computationally intractable. Using the IFL Benchmark, we run large-scale simulation experiments with N = 100 robots, M = 10 algorithmic humans, 5 IFL algorithms, and 3 high-dimensional continuous control environments (Figure 1, left).
We also evaluate IFL algorithms in a real-world image-based block pushing task with N = 4 robot arms and M = 2 remote human teleoperators (Figure 1, right). The 4 arms belong to 2 bimanual ABB YuMi robots operating simultaneously in 2 separate labs about 1 kilometer apart, and remote humans in a third physical location perform teleoperation through a keyboard interface when requested. Each robot pushes a cube toward a unique goal position randomly sampled in the workspace; the goals are programmatically generated in the robots’ overhead image observations and automatically resampled when the previous goals are reached. Physical experiment results suggest trends that are approximately consistent with those observed in the benchmark environments.
Takeaways and Future Directions
To address the gap between the theory and practice of robot fleet learning as well as facilitate future research, we introduce new formalisms, algorithms, and benchmarks for Interactive Fleet Learning. Since IFL does not dictate a specific form or architecture for the shared robot control policy, it can be flexibly synthesized with other promising research directions. For instance, diffusion policies, recently demonstrated to gracefully handle multimodal data, can be used in IFL to allow heterogeneous human supervisor policies. Alternatively, multi-task language-conditioned Transformers like RT-1 and PerAct can be effective “data sponges” that enable the robots in the fleet to perform heterogeneous tasks despite sharing a single policy. The systems aspect of IFL is another compelling research direction: recent developments in cloud and fog robotics enable robot fleets to offload all supervisor allocation, model training, and crowdsourced teleoperation to centralized servers in the cloud with minimal network latency.
While Moravec’s Paradox has so far prevented robotics and embodied AI from fully enjoying the recent spectacular success that Large Language Models (LLMs) like GPT-4 have demonstrated, the “bitter lesson” of LLMs is that supervised learning at unprecedented scale is what ultimately leads to the emergent properties we observe. Since we don’t yet have a supply of robot control data nearly as plentiful as all the text and image data on the Internet, the IFL paradigm offers one path forward for scaling up supervised robot learning and deploying robot fleets reliably in today’s world.
This post is based on the paper “Fleet-DAgger: Interactive Robot Fleet Learning with Scalable Human Supervision” by Ryan Hoque, Lawrence Chen, Satvik Sharma, Karthik Dharmarajan, Brijen Thananjeyan, Pieter Abbeel, and Ken Goldberg, presented at the Conference on Robot Learning (CoRL) 2022. For more details, see the paper on arXiv, CoRL presentation video on YouTube, open-source codebase on Github, high-level summary on Twitter, and project website.
If you would like to cite this article, please use the following bibtex:
@article{ifl_blog,
title={Interactive Fleet Learning},
author={Hoque, Ryan},
url={https://bair.berkeley.edu/blog/2023/04/06/ifl/},
journal={Berkeley Artificial Intelligence Research Blog},
year={2023}
}