The Role Of Artificial Intelligence In Healthcare Sector
The Role of Artificial Intelligence in Healthcare Sector!
The role of Artificial Intelligence (AI) in healthcare offers advanced and precise healthcare services. AI and Machine Learning (ML) technologies offer thrilling opportunities for the healthcare sector. From molecular research and development functions to clinical decisions, everything is performed precisely with AI.
The current scenario of AI in healthcare backed up by ML is smarter enough to identify tumors. Besides, artificial intelligence in healthcare also helps in diagnosing severe diseases efficiently.
[contact-form-7]In this article, we compiled a list of the best applications of AI in the healthcare sector.
The Best applications of AI in the healthcare sector
Applications of AI in healthcare are incredible. The AI technology for the healthcare sector is driving abundant benefits for care service providers. Especially with the use of predictive analytics, AI is transforming the healthcare industry rapidly.
Here are the best benefits of AI in healthcare industry
-
AI For Fast Diagnosis
The benefits of AI in healthcare sector are enormous. , the role of artificial intelligence in healthcare for optimizing disease diagnosis has a high impact on the industry.
AI software solutions, using the potentialities of Machine Learning, and Deep Learning techniques, process vast patient data faster and quickly and accurately make treatment decisions.
Further, predictive analytical features of AI healthcare apps can seamlessly predict diseases faster and help physicians deliver the best care services on time to the patients. Thus, artificial intelligence in healthcare supports practitioners to make quick conclusions and provide the right treatment at the right time.
USM is one of the top AI development companies in the USA. We develop intelligent AI apps for healthcare organizations. Our team of AI experts, with vast proven knowledge of trending concepts of AI, builds fully advanced disease detection software solutions that save lots of time and increase patients care level.
Further, intelligent AI-powered healthcare applications will derive insights into the patient’s health data and offer personalized treatment recommendations to the patients.
AI machines can process vast historic patient data faster for ensuring quick and accurate treatment decisions. AI can predict disease faster than a physician. Thus, artificial intelligence in healthcare supports practitioners for quicker data access for providing the right treatment at the right time.
USM has an innovative AI mobility solution for healthcare service providers. Our care planning AI solution physicians can send treatment recommendations to patients. This solution might be useful for enhancing treatment levels. Get this life-saving AI solution right now!
Let us know our healthcare app requirements and get a free app quote!
-
AI for Drug Development
The drug development use case is the best answer for what is the main role of artificial intelligence in healthcare. Yes, AI in drug development is one of the best use cases of the technology. The use of AI in healthcare in pharmaceuticals plays a significant role in developing advanced drugs to treat complex diseases.
Further, driven by the capabilities of data prediction, AI algorithms in healthcare apps developed for drug discovery and development also predict the toxicity of molecules based on their chemical structure. It is one of the top benefits of using AI in drug development.
Artificial intelligence is widely deploying by pharmaceutical firms for developing new drugs to tackle novel diseases. Companies are using AI for drug research and development functions.
-
AI Automates Admin Tasks
It is one of the best applications of AI in healthcare. AI is the symbol for automation and it is evolving successfully with its intelligent automation capabilities. Automating front-desk operations is one of the major roles of using AI in the healthcare sector.
By automating health insurance services, back-office tasks, patient data management, discharge summary generation, and many more data accessing, storing, and processing activities, AI healthcare solutions are assisting healthcare service providers in terms of streamlined operational processes and enhanced productivity.
Insurance companies are highly investing in AI apps for streamlining internal claiming processes, validating automatically, and disbursing amounts to the insurer on time. Moreover, AI-powered automation applications have the potential to process hundreds of claims simultaneously and accurately validate every word for checking the credit eligibility of each client.
This application is widely used for automating health insurance services. The insurance companies in the healthcare industry are relying on AI for boosting their internal processes. Artificial intelligent systems help them in processing claims automatically with human interference.
AI-powered automation systems analyze hundreds of claims and accurately checks for the credit eligibility of each client.
-
AI Robots For Minimal Invasive Surgeries
Artificial Intelligence and its role in Healthcare has already reached the next level and robotic surgeries are the best examples for this scenario. The robotic surgery includes minimum cuts and slits. Besides, compared to physicians, robots perform surgery with less pain and little incision line. Hence, AI-powered robotic surgeries in healthcare are stress-free and time-saving.
The robotic surgery includes minimum cuts and slits. Besides, compared to physicians, robots perform surgery with less pain and little incision line. Accordingly, telemedicine is also gaining popularity nowadays. Remote surgery using robots and telemedicine with assistants are giving tremendous benefits to care service providers.
Thus, the future of surgery using AI in healthcare is stress-free. Thanks to such advanced AI technology for the healthcare industry.
-
AI for post-discharge services
Few advanced AI apps for healthcare allows doctors to help patients post-discharge. Patients can get detailed data of medication, follow-ups, and physician contact details.
USM has a similar AI app for healthcare service providers. Get your artificial intelligence solution now.
Recommended: AI In Healthcare: The Future of Healthcare Sector Will be Around “AI”
-
Monitoring Post-discharge Patients
Advanced AI apps for healthcare allow doctors to monitor post-discharge patients. Using AI-based electronic health records management system, healthcare service providers can access patient’s health data, monitor detailed medication, schedule follow-ups, and book online appointments with physicians in an emergency.
-
AI Personal Nursing Assistants
It is one of the best applications of AI in healthcare or AI in medicine. As of research, over 50% of patients are comfortable and safe being with virtual nursing assistants. The AI and ML-powered virtual nursing assistants operate with voice and checks patients on time.
8. AI for Quick Navigation
Nobody knows when a person needs emergency treatment. User-friendly AI-powered mobile apps act as a bridge between hospitals and clients. The best example of AI in healthcare for this application is USM’s AI healthcare app.
This next-gen. AI app for healthcare benefits patients to find the nearest healthcare centers. Accordingly, it also gives data on room availability, fab facilities, and so on. If you are a healthcare service provider, get this AI app for healthcare for providing quick and personalized services to clients.
Other Potential Advantages Of Artificial Intelligence In Healthcare
8. Aware Of Regularity Frameworks
In the recent past, healthcare regulations in the United States are updating to keep up with the evolving digital healthcare market. The U.S. Food and Drug Administration (FDA) has been taking incremental phases to amendment the existing rules and regulations. Recently, it was introduced the “Digital Health Innovation Action Plan” to guide the agency’s role in advancing effective digital health technologies.
Besides, the FDA also focused on a digital health software Pre-Cert Pilot Program. It is enrolling software-as-a-medical-device (SaMD) developers in this project. This pilot project helps the FDA to determine the performance indicators that are needed for per-certifying the product. Using this, the FDA helps developers in identifying new ways of product approval procedures that are seamless.
Accordingly, another regularity framework, “Policy for Device Software Functions and Mobile Medical Applications” was introduced in 2019 for higher-risk software. This new policy comprises various guidance documents that label how the agency plans to regulate software that helps in clinical decision support (CDS). The CDS software identifies a patient’s medical conditions as it uses ML algorithms.
9. Achieving FDA Approval
To comply with the changing FDA approval processes, software developers must consider how to design and roll out their products under the FDA rules. Particularly, developers should focus on achieving FDA approval for the software which comes under the higher risk category.
Here, artificial intelligence-powered diagnostic tools and applications come in place. The developers should be more focus on FDA rules when they develop AI-powered healthcare tools and software. As AI is evolving across all sectors, the healthcare industry is also investing in AI to automate its medical diagnostic processes.
Software Update is a major concern that developers face today. Because, based on the market requirements, the developers will update the existing software products. They will add new features to advance the functionalities using emerging AI and ML technologies. But, when they change the technical description of the software, the FDA approval status for the old version of the software will be at risk.
Similarly, organizations must know the company’s product development plans and approach that they follow to get FDA approvals. This provides investors a clear variation of the company over its competitors in the same industry.
USM Business Systems design and deliver AI-powered compliance safety healthcare solutions and services. We develop healthcare mobility solutions using AI, ML, and other emerging technologies. Our innovative AI-powered healthcare solutions for healthcare highly focused on patient safety, secure payment methods, and real-time communication.
Let’s talk.
Are you ready to design an AI-based healthcare app? So, get your artificial intelligence solution quote for healthcare now!
Talk to our AI experts. They’ll let you know the how to develop a compliance safety healthcare applications. Let’s talk.
#3 Black-Box Nature of AI Is Hampering Its Growth In Clinical Applications
Yes, artificial intelligence is a black-box in its nature. This is one of the major drawbacks of the rapid adoption of AI-based applications in healthcare.
We’ll brief it out. Can all healthcare AI-powered applications track and assess the decision making procedure when a negative outcome happens? Will the set of trained data to the ML algorithms is visible to users? Will the reason for a negative outcome is identified by the technology itself?
The developers should focus on all the above points while designing an AI-powered software for healthcare. Because, if your software is posing more negative outcomes, then the application will be banned in the market and the efforts you put on design, development, and getting approvals will be wasted in minutes.
Accordingly, AI-systems need more data to perform the tasks that they assigned to do. But, if you feed the AI systems with wrong data, it will provide incorrect conclusions such as misdiagnosis and improper treatment recommendations. Error Detection algorithms help you out in this scenario. Thanks to technology developments.
Currently, many healthcare service providers are using artificial intelligent-based medical diagnostic devices to provide better services and optimize patient diagnosis process. For instance, FDA approved AI-powered imaging diagnostic software/tools are helping clinicians in diagnosing and treating various health conditions such as cardiovascular disorders, diabetic conditions, and cancer.
However, the adoption of these AI tools is sluggish in the market. There is a need to publish the AI’s benefits in healthcare. More awareness of artificial intelligence in healthcare to witness industry adoption and get the credibility of AI technology.
The Future Of AI In Healthcare
Implementation of AI in healthcare was at the pre-mature stage. Without significant investments, AI adoption in healthcare is slow and difficult. The market researchers are estimating that the clinical health AI applications will save $150 billion per annum for the United States economy by 2025.
Artificial intelligence in healthcare is used for multipurpose. AI can sense machines, learn, and perform both clinical and administrative tasks. Hence, health AI will augment every task done by humans intelligently and automatically. The graph below depicts the most promising healthcare applications of AI that are attracting more investments.
According to the market research reports, the market value of AI in healthcare is at nearly $12 billion in 2021 and is expected to reach approximately $ 187 billion by 2030. Implementation of AI in healthcare was at the pre-mature stage. Significant investments in AI has to be done for its complete rollout.
Driven by its incredible benefits of Artificial intelligence investments in healthcare are anticipated to increase in next coming years. The automatic and intelligent capabilities of AI will augment healthcare processes and ensure high productivity.
Recommended: AI In Healthcare: The Future of Healthcare Sector Will be Around “AI”
On the global artificial intelligence healthcare market front, the investments are stunning and are increasing every year. Both the public and private sectors are heavily investing in health AI. Every healthcare company is in plans of implementing AI strategies to become competitive in the market.
From clinical diagnosis and treatment to robotic surgery and drug development, AI plays an essential role in healthcare. The healthcare AI will completely change the structure of the existing healthcare market in the next decade.
Yes, the complete roll-out of AI will take years, but AI technology, ML, and predictive analytics together bring an advanced healthcare solution that was never before available. According to researchers, AI is widely used for automating admin and operational tasks in the near term future.
Are you ready to automate your healthcare services?
Connect with USM, a leading AI App Development Company.
[contact-form-7]Prying open the AI black box
A method to enable safe mobile robot navigation in dynamic environments
Zebra Study: Only 16% of Manufacturers Have Real-Time Visibility into Manufacturing Production
Robot Talk Episode 90 – Robotically Augmented People
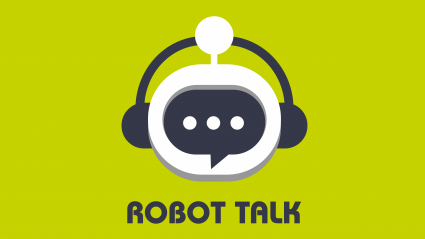
Robotics is helping to rehabilitate and increase human abilities in areas like mobility and stamina. Innovations in robotic devices, exoskeletons, and wearable tech aim to offer disabled people different perspectives and new experiences, as well as supporting humans more widely to access, inhabit and work safely in dangerous and extreme conditions. What does the future hold for these technologies and the people they will become a part of?
In this special live recording at the Victoria and Albert Museum as part of the Great Exhibition Road Festival, Claire chatted to Milia Helena Hasbani (Imperial College London), Benjamin Metcalfe (University of Bath) and Dani Clode (Cambridge University) about robotic prosthetics and human augmentation.
Milia Helena Hasbani is a researcher in assistive technology at Imperial College London. She is passionate about improving people’s lives through innovation in healthcare and technology in multi-disciplinary environments interfacing with engineers, clinicians, and patients. Her research focuses on the control of active prosthetic arms, combining user intention for wrist movements with a computer vision system for dynamically selecting the grasp type to be used. Benjamin Metcalfe is a biomedical engineer who specialises in neural interfaces and implanted devices. He is Head of the Department of Electronic & Electrical Engineering at the University of Bath and Deputy Director of the Bath Institute for the Augmented Human. He is also Vice-President (Academic) of the Institute of Physics and Engineering in Medicine. His interests explore the collision between technology and biology and the extent to which engineering can be used to augment and enhance human performance. Dani Clode is an augmentation and prosthetics designer. She is the Senior Technical Specialist at the Plasticity Lab at Cambridge University and a collaborator of the Alternative Limb Project. Dani’s work investigates the future architecture of our bodies, challenging the perception and boundaries of extending the human form. Her main project the ‘Third Thumb’ is currently being utilised in collaboration with neuroscientists at Cambridge University, investigating the brain’s ability to adapt to human augmentation.
Can AI learn like us?
Trained quickly, inspected swiftly – Handling and defect inspection of indexable inserts
The Role of Robotics in Developing Advanced Autopilot Capabilities
Engineers unlock design for record-breaking robot that could jump twice the height of Big Ben
New soft robotic gripper designed with graphene and liquid crystals
Advanced artificial intelligence: A revolution for sustainable agriculture
Why Do You Need Cross-Environment AI Observability?
AI Observability in Practice
Many organizations start off with good intentions, building promising AI solutions, but these initial applications often end up disconnected and unobservable. For instance, a predictive maintenance system and a GenAI docsbot might operate in different areas, leading to sprawl. AI Observability refers to the ability to monitor and understand the functionality of generative and predictive AI machine learning models throughout their life cycle within an ecosystem. This is crucial in areas like Machine Learning Operations (MLOps) and particularly in Large Language Model Operations (LLMOps).
AI Observability aligns with DevOps and IT operations, ensuring that generative and predictive AI models can integrate smoothly and perform well. It enables the tracking of metrics, performance issues, and outputs generated by AI models –providing a comprehensive view through an organization’s observability platform. It also sets teams up to build even better AI solutions over time by saving and labeling production data to retrain predictive or fine-tune generative models. This continuous retraining process helps maintain and enhance the accuracy and effectiveness of AI models.
However, it isn’t without challenges. Architectural, user, database, and model “sprawl” now overwhelm operations teams due to longer set up and the need to wire multiple infrastructure and modeling pieces together, and even more effort goes into continuous maintenance and update. Handling sprawl is impossible without an open, flexible platform that acts as your organization’s centralized command and control center to manage, monitor, and govern the entire AI landscape at scale.
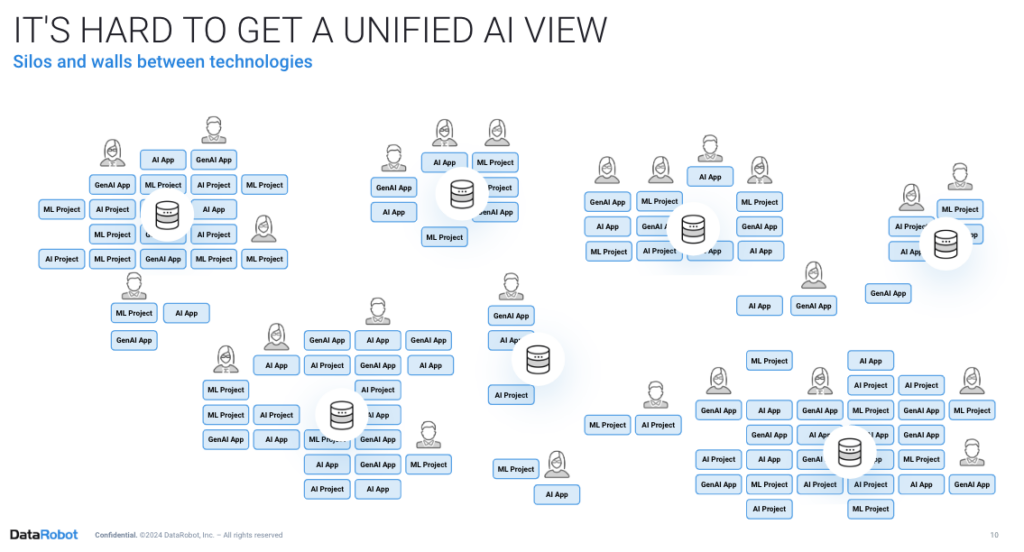
Most companies don’t just stick to one infrastructure stack and might switch things up in the future. What’s really important to them is that AI production, governance, and monitoring stay consistent.
DataRobot is committed to cross-environment observability – cloud, hybrid and on-prem. In terms of AI workflows, this means you can choose where and how to develop and deploy your AI projects while maintaining complete insights and control over them – even at the edge. It’s like having a 360-degree view of everything.
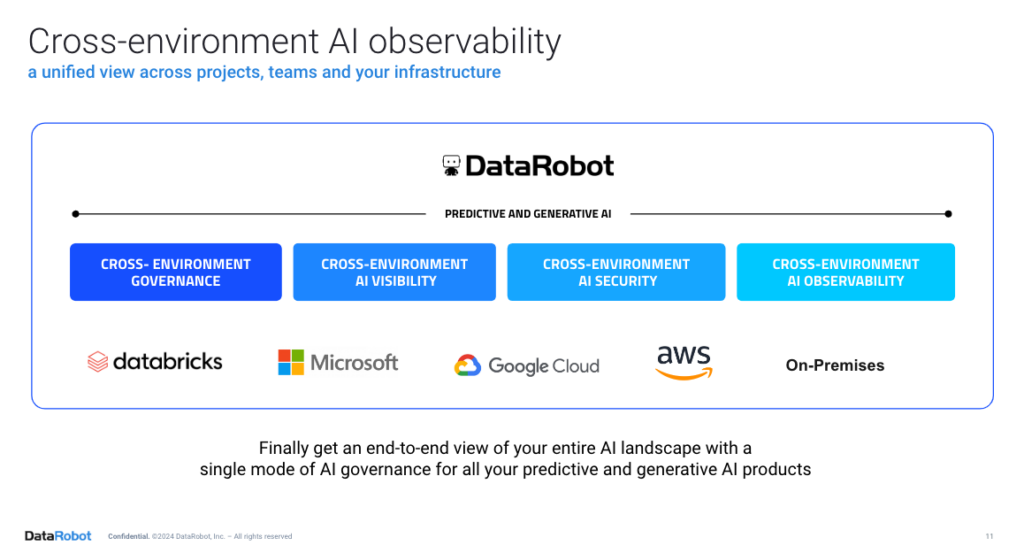
DataRobot offers 10 main out-of-the-box components to achieve a successful AI observability practice:
- Metrics Monitoring: Tracking performance metrics in real-time and troubleshooting issues.
- Model Management: Using tools to monitor and manage models throughout their lifecycle.
- Visualization: Providing dashboards for insights and analysis of model performance.
- Automation: Automating building, governance, deployment, monitoring, retraining stages in the AI lifecycle for smooth workflows.
- Data Quality and Explainability: Ensuring data quality and explaining model decisions.
- Advanced Algorithms: Employing out-of-the-box metrics and guards to enhance model capabilities.
- User Experience: Enhancing user experience with both GUI and API flows.
- AIOps and Integration: Integrating with AIOps and other solutions for unified management.
- APIs and Telemetry: Using APIs for seamless integration and collecting telemetry data.
- Practice and Workflows: Creating a supportive ecosystem around AI observability and taking action on what is being observed.
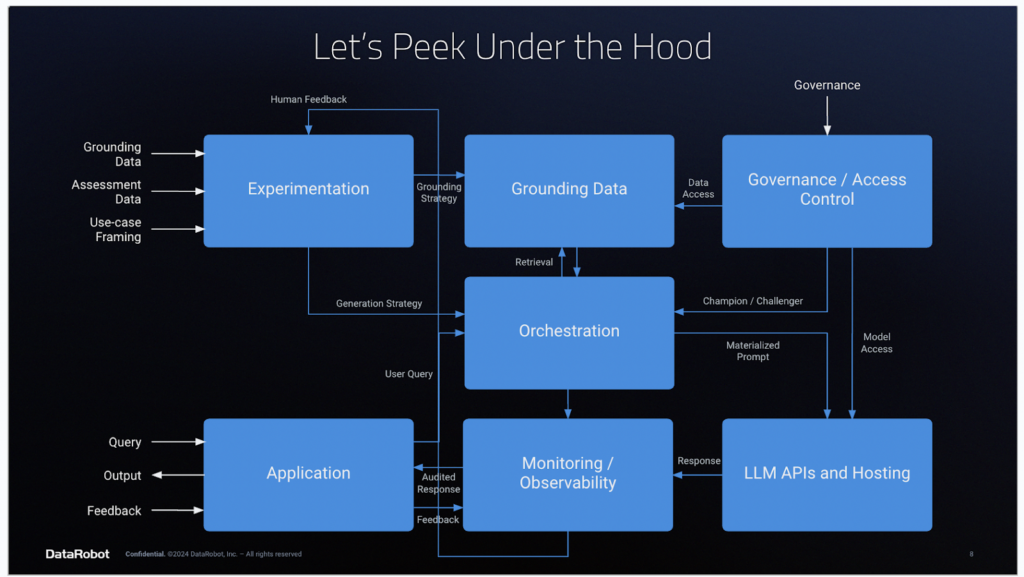
AI Observability In Action
Every industry implements GenAI Chatbots across various functions for distinct purposes. Examples include increasing efficiency, enhancing service quality, accelerating response times, and many more.
Let’s explore the deployment of a GenAI chatbot within an organization and discuss how to achieve AI observability using an AI platform like DataRobot.
Step 1: Collect relevant traces and metrics
DataRobot and its MLOps capabilities provide world-class scalability for model deployment. Models across the organization, regardless of where they were built, can be supervised and managed under one single platform. In addition to DataRobot models, open-source models deployed outside of DataRobot MLOps can also be managed and monitored by the DataRobot platform.
AI observability capabilities within the DataRobot AI platform help ensure that organizations know when something goes wrong, understand why it went wrong, and can intervene to optimize the performance of AI models continuously. By tracking service, drift, prediction data, training data, and custom metrics, enterprises can keep their models and predictions relevant in a fast-changing world.
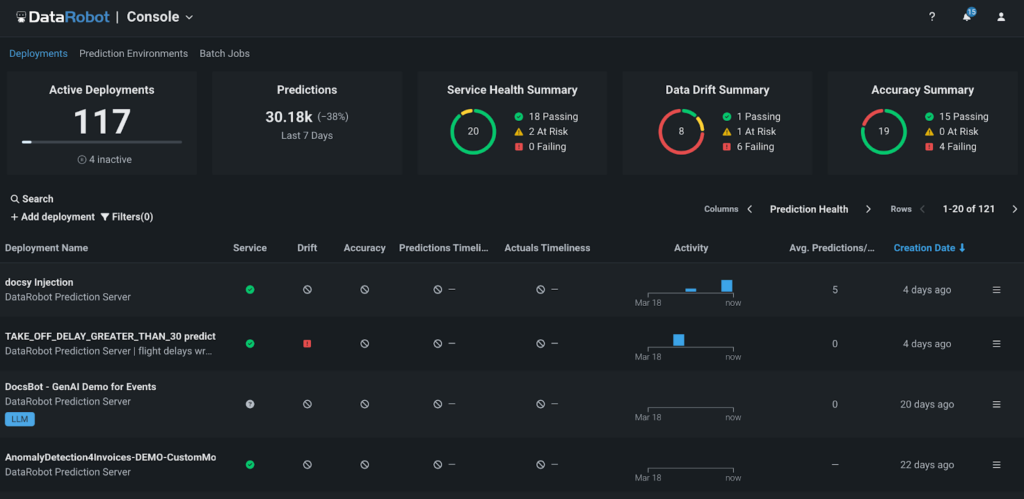
Step 2: Analyze data
With DataRobot, you can utilize pre-built dashboards to monitor traditional data science metrics or tailor your own custom metrics to address specific aspects of your business.
These custom metrics can be developed either from scratch or using a DataRobot template. Use those metrics for the models built or hosted in DataRobot or outside of it.
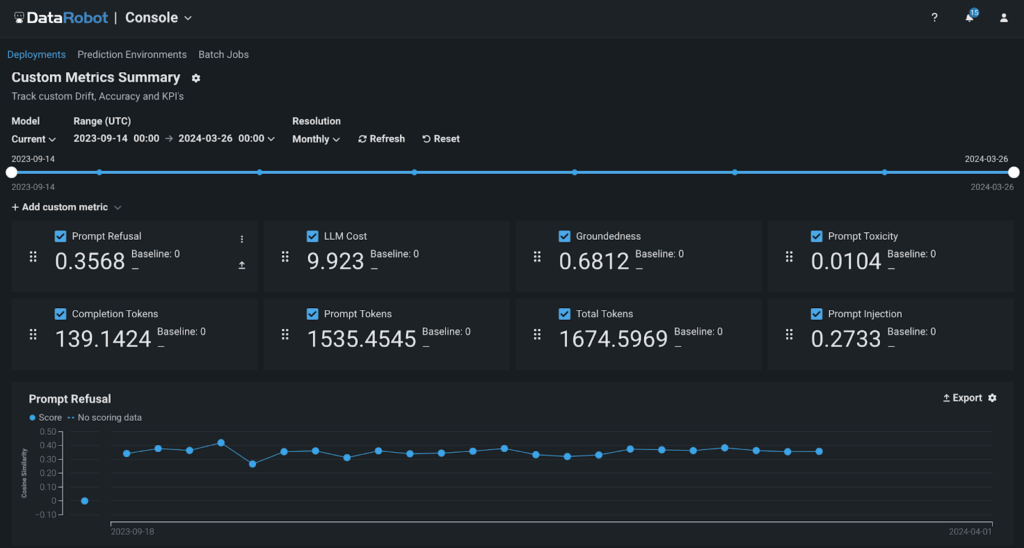
‘Prompt Refusal’ metrics represent the percentage of the chatbot responses the LLM couldn’t address. While this metric provides valuable insight, what the business truly needs are actionable steps to minimize it.
Guided questions: Answer these to provide a more comprehensive understanding of the factors contributing to prompt refusals:
- Does the LLM have the appropriate structure and data to answer the questions?
- Is there a pattern in the types of questions, keywords, or themes that the LLM cannot address or struggles with?
- Are there feedback mechanisms in place to collect user input on the chatbot’s responses?
Use-feedback Loop: We can answer these questions by implementing a use-feedback loop and building an application to find the “hidden information”.
Below is an example of a Streamlit application that provides insights into a sample of user questions and topic clusters for questions the LLM couldn’t answer.
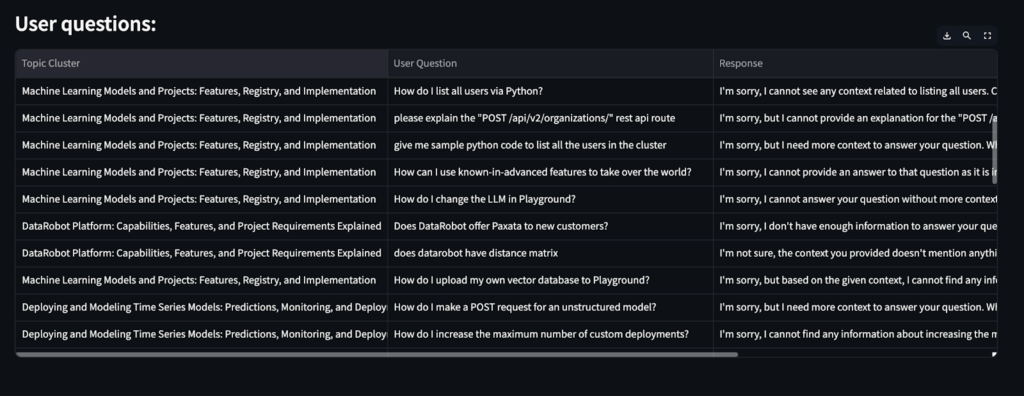
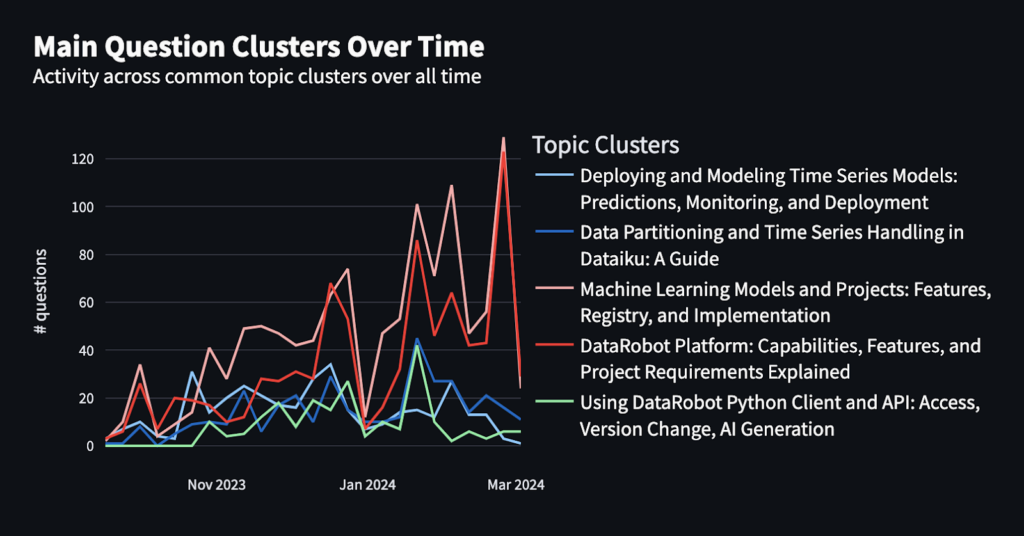
Step 3: Take actions based on analysis
Now that you have a grasp of the data, you can take the following steps to enhance your chatbot’s performance significantly:
- Modify the prompt: Try different system prompts to get better and more accurate results.
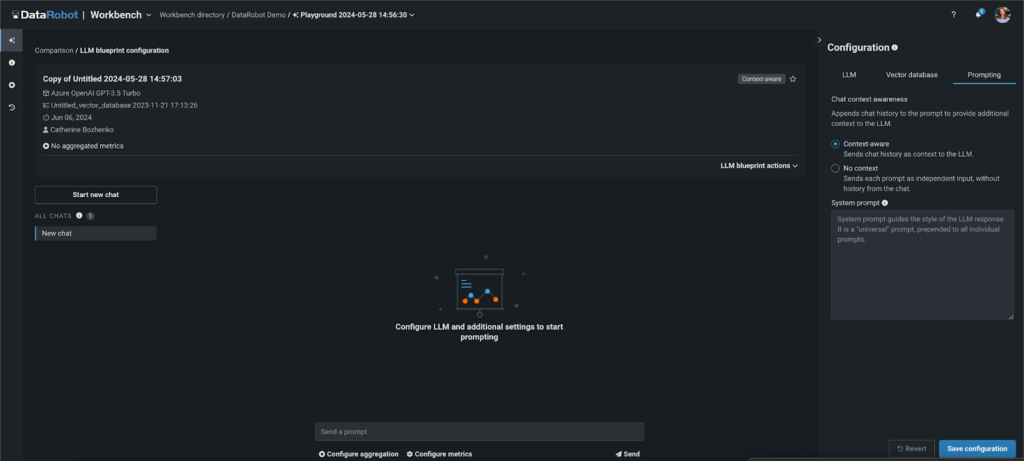
- Improve Your Vector database: Identify the questions the LLM didn’t have answers to, add this information to your knowledge base, and then retrain the LLM.
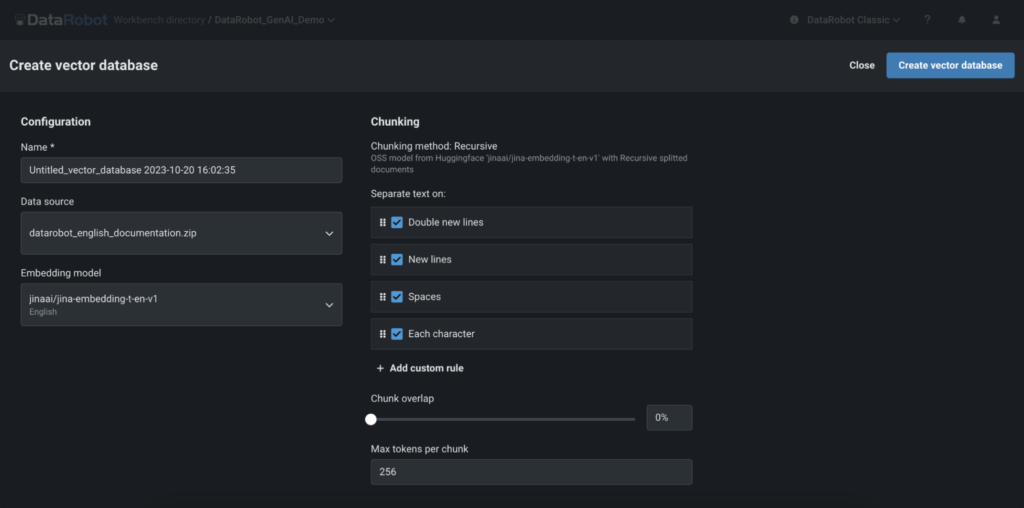
- Fine-tune or Replace Your LLM: Experiment with different configurations to fine-tune your existing LLM for optimal performance.
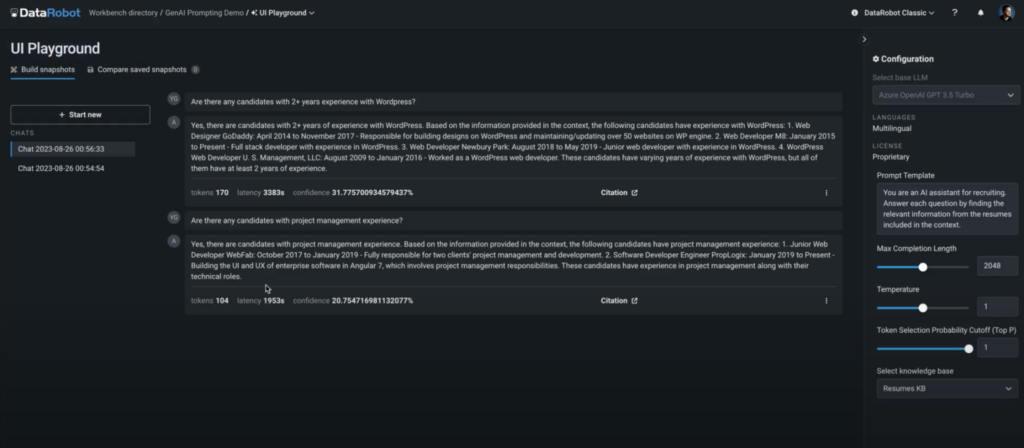
Alternatively, evaluate other LLM strategies and compare their performance to determine if a replacement is needed.
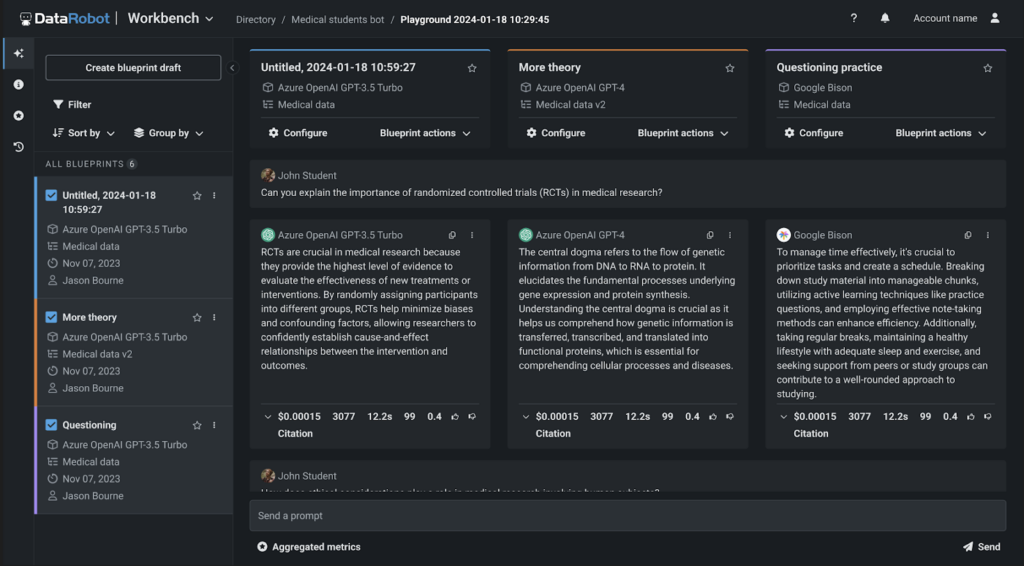
- Moderate in Real-Time or Set the Right Guard Models: Pair each generative model with a predictive AI guard model that evaluates the quality of the output and filters out inappropriate or irrelevant questions.
This framework has broad applicability across use cases where accuracy and truthfulness are paramount. DR provides a control layer that allows you to take the data from external applications, guard it with the predictive models hosted in or outside Datarobot or NeMo guardrails, and call external LLM for making predictions.
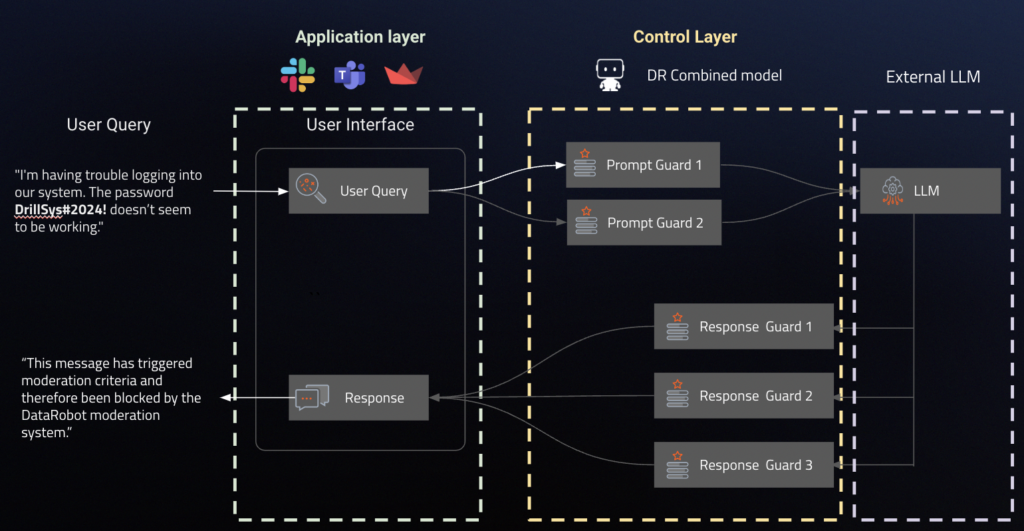
Following these steps, you can ensure a 360° view of all your AI assets in production and that your chatbots remain effective and reliable.
Summary
AI observability is essential for ensuring the effective and reliable performance of AI models across an organization’s ecosystem. By leveraging the DataRobot platform, businesses maintain comprehensive oversight and control of their AI workflows, ensuring consistency and scalability.
Implementing robust observability practices not only helps in identifying and preventing issues in real-time but also aids in continuous optimization and enhancement of AI models, ultimately creating useful and safe applications.
By utilizing the right tools and strategies, organizations can navigate the complexities of AI operations and harness the full potential of their AI infrastructure investments.
The post Why Do You Need Cross-Environment AI Observability? appeared first on DataRobot AI Platform.
Making “cheddar” with industrial automation – Achieving 83 per cent waste reduction in food manufacturing
Building Digital Trust in an AI-Powered World
In today’s digitally connected world, the business landscape is constantly evolving, and the rise in AI usage is further fueling this rapid enterprise transformation. But, at the same time, the technology is exacerbating an age-old challenge for businesses: establishing and […]
The post Building Digital Trust in an AI-Powered World appeared first on TechSpective.