Robots in Depth with Andreas Bihlmaier
In this episode of Robots in Depth, Per Sjöborg speaks with Andreas Bihlmaier about modular robotics and starting a robotics company.
Andreas shares how he started out in computers and later felt that robotics, through its combination of software and hardware that interacts with the world, was what he found most interesting.
Andreas is one of the founders of RoboDev, a company that aims to make automation more available using modular robotics. He explains how modular systems are especially well suited for automating low volume series and how they work with customers to simplify automation.
He also discusses how a system that can easily be assembled into many different robots creates an advantage both in education and in industrial automation, by providing efficiency, flexibility and speed.
We get a personal, behind the scenes account of how the company has evolved as well as insights into the reasoning behind strategic choices made in product development.
Using artificial evolution to design bespoke surgical snakebots
In a world first, Australian Centre for Robotic Vision researchers are pushing the boundaries of evolution to create bespoke, miniaturised surgical robots, uniquely matched to individual patient anatomy.
The cutting-edge research project is the brainchild of Centre PhD researcher Andrew Razjigaev, who impressed HRH The Duke of York with the Centre’s first SnakeBot prototype designed for knee arthroscopy, last November.
Now, the young researcher, backed by the Centre’s world-leading Medical and Healthcare Robotics Group, is taking the next step in surgical SnakeBot’s design.
In place of a single robot, the new plan envisages multiple snake-like robots attached to a RAVEN II surgical robotic research platform, all working together to improve patient outcomes.
The novelty of the project extends to development of an evolutionary computational design algorithm that creates one-of-a-kind, patient-specific SnakeBots in a ‘survival-of-the-fittest’ battle.
Only the most optimal design survives, specifically suited to fit, flexibly manoeuvre and see inside a patient’s knee, doubling as a surgeon’s eyes and tools, with the added bonus of being low-cost (3D printed) and disposable.
Leading the QUT-based Medical and Healthcare Robotics Group, Centre Chief Investigator Jonathan Roberts and Associate Investigator Ross Crawford (who is also an orthopaedic surgeon) said the semi-autonomous surgical system could revolutionise keyhole surgery in ways not before imagined.
Professor Crawford stressed the aim of the robotic system – expected to incorporate surgical dual-arm telemanipulation and autonomous vision-based control – was to assist, not replace surgeons, ultimately improving patient outcomes.
“At the moment surgeons use what are best described as rigid ‘one-size-fits-all’ tools for knee arthroscopy procedures, even though patients and their anatomy can vary significantly,” Professor Crawford said.
He said the surgical system being explored had the potential to vastly surpass capabilities of current state-of-the-art surgical tools.
“The research project aims to design snake-like robots as miniaturised and highly dexterous surgical tools, fitted with computer vision capabilities and the ability to navigate around obstacles in confined spaces such as the anatomy of the human body,” Professor Crawford said.
“Dexterity is incredibly important as the robots are not only required to reach surgical sites but perform complicated surgical procedures via telemanipulation.”
Professor Roberts said the research project was a world-first for surgical robotics targeting knee arthroscopy and would not be possible without the multi-disciplinary expertise of researchers at the Australian Centre for Robotic Vision.
“One of the most exciting things about this project is that it is bringing many ideas from the robotics community together to form a practical solution to a real-world problem,” he said.
“The project has been proceeding at a rapid pace, mainly due to the hard work and brilliance of Andrew, supported by a team of advisors with backgrounds in mechanical engineering, mechatronics, aerospace, medicine, biology, physics and chemistry.”
Due to complete his PhD research project by early 2021, Andrew Razjigaev graduated as a mechatronics engineer at QUT in 2017 and has been a part of the Centre’s Medical and Healthcare Robotics Group since 2016.
The 23-year-old said: “Robotics is all about helping people in some way and what I’m most excited about is that this project may lead to improved health outcomes, fewer complications and faster patient recovery.
“That’s what really drives my research – being able to help people and make a positive difference. Knee arthroscopy is one of most common orthopaedic procedures in the world, with around four million procedures a year, so this project could have a huge impact.”
Andrew said he hoped his work would lead to real-world development of new surgical tools.
“Surgeons want to do the best they can and face a lot of challenges,” he said. “Our objective is to provide surgeons with new tools to be able to perform existing surgery, like knee arthroscopy, more efficiently and safely and to perhaps perform surgery that is simply too difficult to attempt with today’s tools.
“It’s also incredibly cool to use evolution in my work! There’s no question we’re witnessing the age-old process – the only difference being it’s happening inside a computer instead of nature.”
- The process starts with a scan of a patient’s knee. With the supervision of a doctor, the computer classifies the regions for the SnakeBots to reach in the knee (green area) and regions to avoid (red area).
- The resulting geometry makes a 3D environment for the SnakeBots to compete in the simulated evolution. It enables a number of standard SnakeBot designs to be tested and scored on how well they perform – namely how well they manoeuvre to sites inside a patient’s knee. The black lines in the test show some of the trajectories a SnakeBot took to manoeuvre to those sites.
- The evolutionary computational design algorithm kicks in, continually creating new generations of SnakeBots, re-testing and killing off weaker variants until one survives, uniquely matched to an individual patient’s anatomy. The SnakeBot that can safely reach those targets with more dexterity wins the battle of evolution and claims the optimal design.
- The optimal SnakeBots are generated into 3D models to be 3D printed as low-cost, disposable surgical tools unique to each patient.
- They are now ready to be deployed for surgery! The micro SnakeBots are attached to a larger, table-top robotic platform (like the RAVEN II) that positions them for entry into surgical incision sites.
- It is expected that two SnakeBots are fitted with surgical instruments at their tips to enable a surgeon to perform dual-arm teleoperated surgical procedures.
- A third SnakeBot in the multi-bot system will have a camera installed at its tip. This camera system will be used by a robotic vision system to map a patient’s body cavity so that the robot can be steered towards the areas of interest and away from delicate areas that should be avoided. It will track the two arms and surgical area simultaneously, working as the eyes of the surgeon.
Find out more about the work of the Centre’s Medical and Healthcare Robotics Group in our latest annual report.
Solving the Mysteries of the Universe
Smart Production Lines in Automotive Industry Use Cobots
Tiny motor can “walk” to carry out tasks
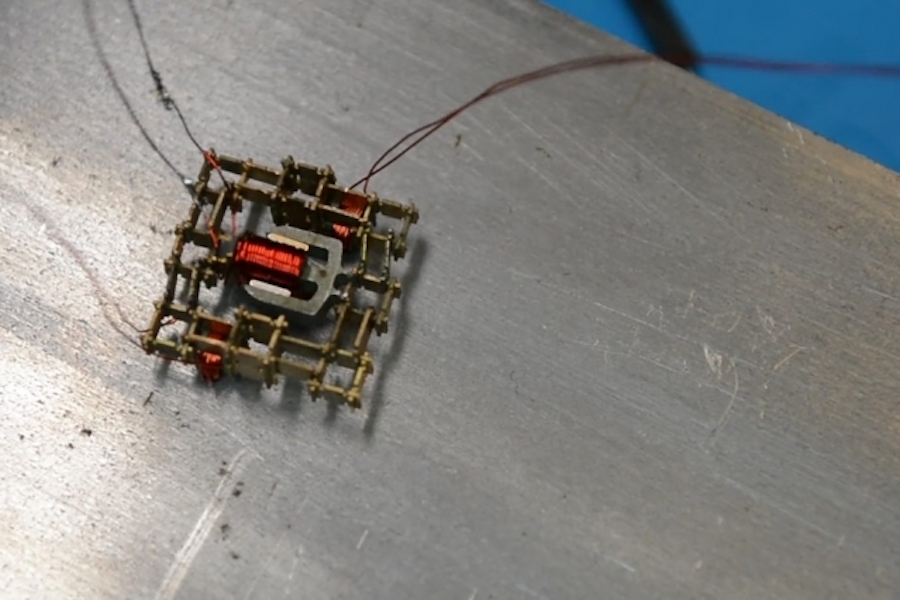
Photo by Will Langford
By David L. Chandler
Years ago, MIT Professor Neil Gershenfeld had an audacious thought. Struck by the fact that all the world’s living things are built out of combinations of just 20 amino acids, he wondered: Might it be possible to create a kit of just 20 fundamental parts that could be used to assemble all of the different technological products in the world?
Gershenfeld and his students have been making steady progress in that direction ever since. Their latest achievement, presented this week at an international robotics conference, consists of a set of five tiny fundamental parts that can be assembled into a wide variety of functional devices, including a tiny “walking” motor that can move back and forth across a surface or turn the gears of a machine.
Previously, Gershenfeld and his students showed that structures assembled from many small, identical subunits can have numerous mechanical properties. Next, they demonstrated that a combination of rigid and flexible part types can be used to create morphing airplane wings, a longstanding goal in aerospace engineering. Their latest work adds components for movement and logic, and will be presented at the International Conference on Manipulation, Automation and Robotics at Small Scales (MARSS) in Helsinki, Finland, in a paper by Gershenfeld and MIT graduate student Will Langford.
Their work offers an alternative to today’s approaches to contructing robots, which largely fall into one of two types: custom machines that work well but are relatively expensive and inflexible, and reconfigurable ones that sacrifice performance for versatility. In the new approach, Langford came up with a set of five millimeter-scale components, all of which can be attached to each other by a standard connector. These parts include the previous rigid and flexible types, along with electromagnetic parts, a coil, and a magnet. In the future, the team plans to make these out of still smaller basic part types.
Using this simple kit of tiny parts, Langford assembled them into a novel kind of motor that moves an appendage in discrete mechanical steps, which can be used to turn a gear wheel, and a mobile form of the motor that turns those steps into locomotion, allowing it to “walk” across a surface in a way that is reminiscent of the molecular motors that move muscles. These parts could also be assembled into hands for gripping, or legs for walking, as needed for a particular task, and then later reassembled as those needs change. Gershenfeld refers to them as “digital materials,” discrete parts that can be reversibly joined, forming a kind of functional micro-LEGO.
The new system is a significant step toward creating a standardized kit of parts that could be used to assemble robots with specific capabilities adapted to a particular task or set of tasks. Such purpose-built robots could then be disassembled and reassembled as needed in a variety of forms, without the need to design and manufacture new robots from scratch for each application.
Langford’s initial motor has an ant-like ability to lift seven times its own weight. But if greater forces are required, many of these parts can be added to provide more oomph. Or if the robot needs to move in more complex ways, these parts could be distributed throughout the structure. The size of the building blocks can be chosen to match their application; the team has made nanometer-sized parts to make nanorobots, and meter-sized parts to make megarobots. Previously, specialized techniques were needed at each of these length scale extremes.
“One emerging application is to make tiny robots that can work in confined spaces,” Gershenfeld says. Some of the devices assembled in this project, for example, are smaller than a penny yet can carry out useful tasks.
To build in the “brains,” Langford has added part types that contain millimeter-sized integrated circuits, along with a few other part types to take care of connecting electrical signals in three dimensions.
The simplicity and regularity of these structures makes it relatively easy for their assembly to be automated. To do that, Langford has developed a novel machine that’s like a cross between a 3-D printer and the pick-and-place machines that manufacture electronic circuits, but unlike either of those, this one can produce complete robotic systems directly from digital designs. Gershenfeld says this machine is a first step toward to the project’s ultimate goal of “making an assembler that can assemble itself out of the parts that it’s assembling.”
Robot-ants that communicate and work together
A team of EPFL researchers has developed tiny 10-gram robots that are inspired by ants: they can communicate with each other, assign roles among themselves and complete complex tasks together. These reconfigurable robots are simple in structure, yet they can jump and crawl to explore uneven surfaces. The researchers have just published their work in Nature.
Individually, ants have only so much strength and intelligence. However, as a colony, they can use complex strategies for achieving sophisticated tasks to survive their larger predators.
At EPFL, researchers in NCCR Robotics Professor Jamie Paik’s Laboratory have reproduced this phenomenon, developing tiny robots that display minimal physical intelligence on an individual level but that are able to communicate and act collectively. Despite being simple in design and weighing only 10 grams, each robot has multiple locomotion modes to navigate any type of surface. Collectively, they can quickly detect and overcome obstacles, pass them and move objects much larger and heavier than themselves. The related research has been published in Nature.
Robots modeled on trap-jaw ants
These three-legged, T-shaped origami robots are called Tribots. They can be assembled in only a few minutes by folding a stack of thin, multi-material sheets, making them suitable for mass production. Completely autonomous and untethered, Tribots are equipped with infrared and proximity sensors for detection and communication purposes. They could accommodate even more sensors depending on the application.
“Their movements are modeled on those of Odontomachus ants. These insects normally crawl, but to escape a predator, they snap their powerful jaws together to jump from leaf to leaf”, says Zhenishbek Zhakypov, the first author. The Tribots replicate this catapult mechanism through an elegant origami robot design that combines multiple shape-memory alloy actuators. As a result, a single robot can produce three distinctive locomotive motions – crawling, rolling and jumping both vertically and horizontally – just like these creatively resilient ants.
Roles: leader, worker and explorer
Despite having the same “anatomy”, each robot is assigned a specific role depending on the situation. ‘Explorers’ detect physical obstacles in their path, such as objects, valleys and mountains. After detecting an obstacle, they inform the rest of the group. Then, the “leader” gives the instructions. The ‘workers’, meanwhile, pool their strength to move objects. “Each Tribot, just like Odontomachus ants, can have different roles. However, they can also take on new roles instantaneously when faced with a new mission or an unknown environment, or even when other members get lost. This goes beyond what the real ants can do” says Paik.
Future applications
In practical situations, such as in an emergency search mission, Tribots could be deployed en masse. And thanks to their multi-locomotive and multi-agent communication capabilities, they could locate a target quickly over a large surface without relying on GPS or visual feedback. “Since they can be manufactured and deployed in large numbers, having some ‘casualties’ would not affect the success of the mission,” adds Paik. “With their unique collective intelligence, our tiny robots are better equipped to adapt to unknown environments. Therefore, for certain missions, they would outperform larger, more powerful robots.” The development of robots for search-and-rescue applications and the study of collective robotics are key research areas within the NCCR Robotics consortium, of which Jamie Paik’s lab is part.
In April, Jamie Paik has presented her reconfigurable robots at the TED2019 Conference in Vancouver. Her talk is available here.
Literature
Zhenishbek Zhakypov, Kazuaki Mori, Koh Hosoda and Jamie Paik, Designing minimal and scalable insect-inspired multi-locomotion millirobots, Nature
DOI: 10.1038/s41586-019-1388-8
ABB Robotics to Develop Solutions for the Hospital of the Future
Beyond sex robots: Erobotics explores erotic human-machine interactions
Is Robotization Destroying or Creating Jobs?
Solve Loading Dock Challenges With Robotic Unloading
Neuron EV Unveils the Future of Smart Travel and Residence
#290: AI Powered Robotic Picking at Promat 2019, with Vince Martinelli, Jim Liefer, Pete Blair, Sean Davis and Erik Nieves
In this episode, join our interviewer Andrew Vaziri at Promat 2019, the largest expo for manufacturing and supply chain professionals in North and South America. Andrew interviews a handful of companies which provide warehouse fulfillment robots that can autonomous pick and place items. Our guests explain how advances in AI have made autonomous picking possible. They also talk about the unique technologies they use to stand out in a crowded field of competing products.
The guests in the order they appear are:
Vince Martinelli, Head of Product and Marketing, RightHand Robotics
Jim Liefer, CEO, Kindred
Pete Blair, VP of Marketing, Berkshire Grey
Sean Davis, Technical Product Manager, Osaro
Erik Nieves, CEO, Plus One Robotics
See video of each robot picking items on our YouTube page:
Links