Robot Talk Episode 112 – Getting creative with robotics, with Vali Lalioti
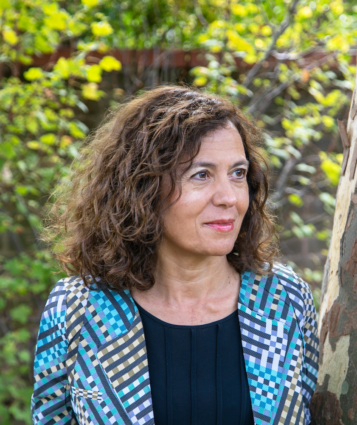
Claire chatted to Vali Lalioti from the University of the Arts London about how art, culture and robotics interact.
Vali Lalioti is a pioneering designer, computer scientist and innovator. She has a PhD in Computer Science, an MRes in Design and an MBA, and extensive international leadership, research and innovation experience in Silicon Valley, Africa, China, Japan and Europe. Vali is passionate about how technology interacts with society and talks globally on women in tech, art and technology education and her research in societal applications for well-being, healthy ageing and art. She developed the first ever BBC Augmented Reality production in 2003 and has introduced the UK’s first Creative Robotics University Degrees.
Report on the future of AI research
Image taken from the front cover of the Future of AI Research report.
The Association for the Advancement of Artificial Intelligence (AAAI), has published a report on the Future of AI Research. The report, which was announced by outgoing AAAI President Francesca Rossi during the AAAI 2025 conference, covers 17 different AI topics and aims to clearly identify the trajectory of AI research in a structured way.
The report is the result of a Presidential Panel, chaired by Francesca Rossi, and comprising of 24 experienced AI researchers, who worked on the project between summer 2024 and spring 2025. As well as the views of the panel members, the report also draws on community feedback, which was received from 475 AI researchers via a survey.
The 17 topics, each with a dedicated chapter, are as follows.
- AI Reasoning
- AI Factuality & Trustworthiness
- AI Agents
- AI Evaluation
- AI Ethics & Safety
- Embodied AI
- AI & Cognitive Science
- Hardware & AI
- AI for Social Good
- AI & Sustainability
- AI for Scientific Discovery
- Artificial General Intelligence (AGI)
- AI Perception vs. Reality
- Diversity of AI Research Approaches
- Research Beyond the AI Research Community
- Role of Academia
- Geopolitical Aspects & Implications of AI
Each chapter includes a list of main takeaways, context and history, current state and trends, research challenges, and community opinion. You can read the report in full here.
Paralyzed man moves robotic arm with his thoughts
Difference Between Artificial Intelligence and Machine Learning
Difference Between Artificial Intelligence and Machine Learning
Nowadays, Artificial Intelligence (AI) and Machine learning (ML) technologies are the two most trending technologies. Many companies are investing in AI and ML applications to transform the existing business processes.
Most of the people are confused about the difference between Artificial Intelligence and Machine Learning. So, we are here to clear your confusion!
Today, in this article, we will be giving a detail about what is AI? What is ML? And what is the major difference between AI and ML technologies.
What is Artificial Intelligence (AI)?
Artificial Intelligence is defined as a smart concept that enables machines to perform various tasks done by humans. AI becomes more popular nowadays with its automation and intelligent features.
AI has been in talks since long back. Gradually, the technology is moving to the next level. The researchers continue to invent something new in AI. Artificial Intelligence machines can solve complex calculations.
AI along with ML techniques, it has been scientifically proven to reflect human decision processes and improve machine intelligence.
Get in Touch
[contact-form-7]How Does AI Works and Why Is AI So Important?
- AI can automate the every task which is done by human previously
- AI frequently performs high-volume machine tasks efficiently
- Industries are improving their tasks using AI capabilities
- AI-based apps, conversational tools, and chatbots help companies in improving digital marketing
- AI can build fraud detection systems to identify and track illegal access to data systems or network
- AI uses ML to predict the future outcomes
- AI apps in healthcare used to detect diseases with high accuracy
- AI in automobile used for developing autonomous cars
The field of Applied AI is still observing advancements. We can state that advancements in AI is welcoming more innovations in ML. As a subset of AI, machine learning program is giving more valuable insights and predictions into data. Thus, ML is supporting new research works in AI.
What is Machine Learning?
The machine learning is best defined as an important application of AI, which allows a computer or machine to learn from input data and improve the experience without the need for explicit programming. The primary aim of the advanced machine learning algorithms is to allow systems to learn automatically without one’s interaction.
Rapid Growth of Machine Learning
Driven by the advancements in AI, the demand for ML techniques is expanding rapidly. ML allows the software to predict future outcomes accurately.
In addition, a vast amount of digital data over the internet is increasing the demand for ML solutions. In particular, digital businesses are highly adopting ML, and deep learning apps to manage their customers proficiently.
The researchers thought that instead of training machines how to perform, it’s better to code them once to do repeated tasks automatically. This trend increased the demand for the development of machine learning, deep learning, data analysis, and predictive analytics.
How Does Machine Learning Works?
How does AI differ from machine learning:
- Step 1: Learns from a trained data set
- Step 2: Identifies dissimilar data from a group of similar data and hence measures error rate
- Step 3: Identifies noise attributes to improve the processing capacity
- Step 4: Data validation and testing processes to deliver accurate error measure
- Step 5: Insights into data
Difference Between AI and Machine Learning: Artificial Intelligence Vs Machine Learning
Here are the top differences between AI and ML:
The above table helps you learn how does AI differ from machine learning. Being a subset of AI, the difference between machine learning and AI is specific to learning and insights extraction.
What is Generative AI?
Generative AI is a form of artificial intelligence that produces original content, such as images, text, or music, based on learning from current data. It utilizes models such as GANs and transformers to create realistic results that mimic actual instances. The technology applies to industries such as art, entertainment, and medicine.
What Is The Difference Between Generative AI And Machine Learning
Both generative AI (GenAI) and machine learning fall under artificial intelligence but have varying uses. Machine learning aims at model training for the purpose of recognizing patterns within data and prediction or decision-making, including categorization of data, predicting trends, or the identification of outliers. Machine learning incorporates methodologies such as supervised, unsupervised, and reinforcement learning.
Generative AI, in contrast, is a niche field of machine learning whose purpose is to generate new content, images, text, or music from given prompts. The major difference between generative AI and Machine Learning is mostly about analysis and prediction.
Another difference between Gen AI and machine learning is in their model training goals. Machine learning models are trained to achieve optimal performance on tasks such as prediction by maximizing accuracy. In contrast, generative AI models are trained so that they will discover the structural and distribution information in the data to produce fresh, related data.
Is ChatGPT AI or Machine Learning
ChatGPT is powered by AI technology that uses machine learning and deep learning to better understand user prompts and create human-like responses. It’s trained on massive amounts of text data to learn about language patterns, context, and structure, enabling it to respond to questions, engage in conversation, and help with other tasks. Although ChatGPT itself uses machine learning, it is a subcategory of the larger AI genre because it demonstrates intelligent behavior such as natural language generation and understanding.
Neural Networks
The primary reason for the development of Neural Networks is to train the systems to replicate exactly like humans.
A Neural Network system can categorize the data in a manner as human brain do. These systems can recognize images and categorize them based on the elements they comprise. In the image below, the nervous system takes an input image, processes it, and finally identifies objects using previously gained experiences.
Based on the trained data, it can make decisions, predictions, and statements with confidence. Along with the feedback loop, it can decide the predicted decisions are wrong or right. Thus, neural network systems can modify the approach it takes in the future.
Accordingly ML apps can read and understand the input text and categorize whether that text is a complaint or greetings. In addition, ML applications can also listen to music and determines whether it makes a person happy or sad.
All these are a few applications of ML and neural network systems. The major idea behind all research works is connecting digital data and electronic devices intelligently. To reach this, AI also uses natural language processing (NLP) to efficiently understand human language.
NLP is highly dependent on ML techniques. The NLP-based apps can interpret written/spoken language and respond to the user in the same way.
Machine Learning Vs Neural Networks
Machine Learning | Neural Networks |
Falls under the field of artificial intelligence | A sub-field of machine learning |
Enables machines to automatically learn and process input data without being explicitly programmed. | Also called as artificial neural network used for categorizing data/images as our bran do |
Types: Supervised and unsupervised learning methods | Types: Convolutional neural networks and recurrent neural networks |
Mostly used in healthcare, retail, e-commerce, pricing strategies, customer retention etc. | Applied in finance, healthcare, retail, stock prediction, and etc. |
Google Maps, Siri, and google search are the best examples of machine learning. | Image recognition, compression, and search engines are the best examples of neural networks. |
Advanced Artificial Intelligence and Machine Learning Market Overview:
Increased investment in AI technologies, the growing need to process large amounts of data and the lack of experienced technicians to manage business tasks are key growth factors of the artificial intelligence market size. In between 2016-2025, the market size is expected to project $169.41 billion by 2025 from $4.06 billion in 2016.
To Conclude, Next-Level of AI and ML Offers Huge Opportunities to Businesses
Despite the difference between AI and ML technologies is being thin, we can understand that the combination of AI and machine learning models provides intelligent business processes. Different industries ranging from healthcare and banking to manufacturing and e-commerce are widening business opportunities. Thus, AI, ML, deep learning, and neural networks expand your brand awareness.
For instance, the sales and marketing teams are using ML systems to detect the behavior of its customers search. Thus, AI & ML apps for marketing and sales industry are providing growth benefits to them. Multiple developments in AI leads to the development of ML technology even more.
Connect with USM to know more the benefits of AI and ML Technologies.
Hope, this article makes you understand the basic difference between AI and ML. We would like to add more valuable information related to Artificial intelligence, reinforcement learning, computer science, data science, big data, and deep learning technologies.
Get in Touch
[contact-form-7]
Tiny ‘rhinoceros beetle’ robot does micro-scale manipulation in extreme conditions
Engineers develop smart, energy-efficient robot grippers that cut production costs
Smart, energy-efficient robot grippers cut production costs
AI has ‘great potential’ for detecting wildfires, new study of the Amazon rainforest suggests
Innovative biorobotic arm uses artificial muscles to combat tremors, paving way for wearable solutions
Artificial muscles for tremor suppression
ProMat Q&A with Pickle Robot
Andrew Barto and Richard Sutton win 2024 Turing Award
Andrew Barto and Richard Sutton. Image credit: Association for Computing Machinery.
The Association for Computing Machinery, has named Andrew Barto and Richard Sutton as the recipients of the 2024 ACM A.M. Turing Award. The pair have received the honour for “developing the conceptual and algorithmic foundations of reinforcement learning”. In a series of papers beginning in the 1980s, Barto and Sutton introduced the main ideas, constructed the mathematical foundations, and developed important algorithms for reinforcement learning.
The Turing Award comes with a $1 million prize, to be split between the recipients. Since its inception in 1966, the award has honoured computer scientists and engineers on a yearly basis. The prize was last given for AI research in 2018, when Yoshua Bengio, Yann LeCun and Geoffrey Hinton were recognised for their contribution to the field of deep neural networks.
Andrew Barto is Professor Emeritus, Department of Information and Computer Sciences, University of Massachusetts, Amherst. He began his career at UMass Amherst as a postdoctoral Research Associate in 1977, and has subsequently held various positions including Associate Professor, Professor, and Department Chair. Barto received a BS degree in Mathematics (with distinction) from the University of Michigan, where he also earned his MS and PhD degrees in Computer and Communication Sciences.
Richard Sutton is a Professor in Computing Science at the University of Alberta, a Research Scientist at Keen Technologies (an artificial general intelligence company based in Dallas, Texas) and Chief Scientific Advisor of the Alberta Machine Intelligence Institute (Amii). Sutton was a Distinguished Research Scientist at Deep Mind from 2017 to 2023. Prior to joining the University of Alberta, he served as a Principal Technical Staff Member in the Artificial Intelligence Department at the AT&T Shannon Laboratory in Florham Park, New Jersey, from 1998 to 2002. Sutton received his BA in Psychology from Stanford University and earned his MS and PhD degrees in Computer and Information Science from the University of Massachusetts at Amherst.
The two researchers began collaborating in 1978, at the University of Massachusetts at Amherst, where Barto was Sutton’s PhD and postdoctoral advisor.