Bio-hybrid drone uses silkworm moth antennae to navigate by smell
New microactuator driving system could give microdrones a jump-start
How to use DeepSeek-R1 for enterprise-ready AI
As you may have heard, DeepSeek-R1 is making waves. It’s all over the AI newsfeed, hailed as the first open-source reasoning model of its kind.
The buzz? Well-deserved.
The model? Powerful.
DeepSeek-R1 represents the current frontier in reasoning models, being the first open-source version of its kind. But here’s the part you won’t see in the headlines: working with it isn’t exactly straightforward.
Prototyping can be clunky. Deploying to production? Even trickier.
That’s where DataRobot comes in. We make it easier to develop with and deploy DeepSeek-R1, so you can spend less time wrestling with complexity and more time building real, enterprise-ready solutions.
Prototyping DeepSeek-R1 and bringing applications into production are critical to harnessing its full potential and delivering higher-quality generative AI experiences.
So, what exactly makes DeepSeek-R1 so compelling — and why is it sparking all this attention? Let’s take a closer look at if all the hype is justified.
Could this be the model that outperforms OpenAI’s latest and greatest?
Beyond the hype: Why DeepSeek-R1 is worth your attention
DeepSeek-R1 isn’t just another generative AI model. It’s arguably the first open-source “reasoning” model — a generative text model specifically reinforced to generate text that approximates its reasoning and decision-making processes.
For AI practitioners, that opens up new possibilities for applications that require structured, logic-driven outputs.
What also stands out is its efficiency. Training DeepSeek-R1 reportedly cost a fraction of what it took to develop models like GPT-4o, thanks to reinforcement learning techniques published by DeepSeek AI. And because it’s fully open-source, it offers greater flexibility while allowing you to maintain control over your data.
Of course, working with an open-source model like DeepSeek-R1 comes with its own set of challenges, from integration hurdles to performance variability. But understanding its potential is the first step to making it work effectively in real-world applications and delivering more relevant and meaningful experience to end users.
Using DeepSeek-R1 in DataRobot
Of course, potential doesn’t always equal easy. That’s where DataRobot comes in.
With DataRobot, you can host DeepSeek-R1 using NVIDIA GPUs for high-performance inference or access it through serverless predictions for fast, flexible prototyping, experimentation, and deployment.
No matter where DeepSeek-R1 is hosted, you can integrate it seamlessly into your workflows.
In practice, this means you can:
- Compare performance across models without the hassle, using built-in benchmarking tools to see how DeepSeek-R1 stacks up against others.
- Deploy DeepSeek-R1 in production with confidence, supported by enterprise-grade security, observability, and governance features.
- Build AI applications that deliver relevant, reliable outcomes, without getting bogged down by infrastructure complexity.
LLMs like DeepSeek-R1 are rarely used in isolation. In real-world production applications, they function as part of sophisticated workflows rather than standalone models. With this in mind, we evaluated DeepSeek-R1 within multiple retrieval-augmented generation (RAG) pipelines over the well-known FinanceBench dataset and compared its performance to GPT-4o mini.
So how does DeepSeek-R1 stack up in real-world AI workflows? Here’s what we found:
- Response time: Latency was notably lower for GPT-4o mini. The 80th percentile response time for the fastest pipelines was 5 seconds for GPT-4o mini and 21 seconds for DeepSeek-R1.
- Accuracy: The best generative AI pipeline using DeepSeek-R1 as the synthesizer LLM achieved 47% accuracy, outperforming the best pipeline using GPT-4o mini (43% accuracy).
- Cost: While DeepSeek-R1 delivered higher accuracy, its cost per call was significantly higher—about $1.73 per request compared to $0.03 for GPT-4o mini. Hosting choices impact these costs significantly.
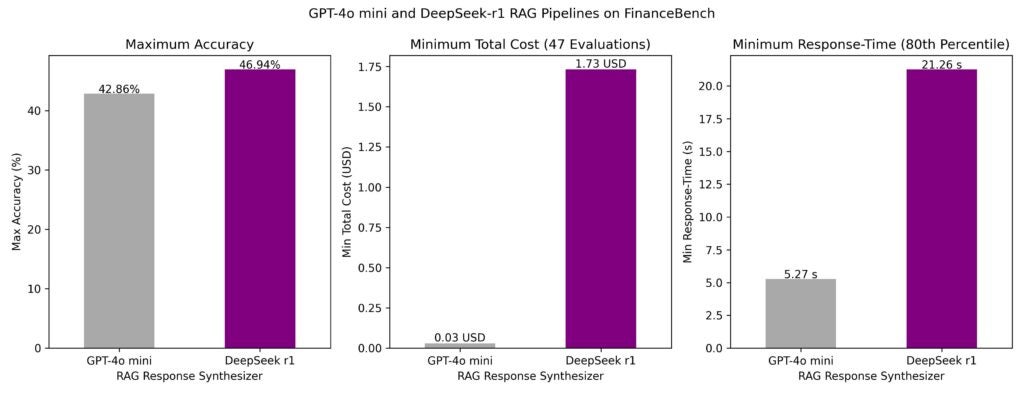
While DeepSeek-R1 demonstrates impressive accuracy, its higher costs and slower response times may make GPT-4o mini the more efficient choice for many applications, especially when cost and latency are critical.
This analysis highlights the importance of evaluating models not just in isolation but within end-to-end AI workflows.
Raw performance metrics alone don’t tell the full story. Evaluating models within sophisticated agentic and non-agentic RAG pipelines offers a clearer picture of their real-world viability.
Using DeepSeek-R1’s reasoning in agents
DeepSeek-R1’s strength isn’t just in generating responses — it’s in how it reasons through complex scenarios. This makes it particularly valuable for agent-based systems that need to handle dynamic, multi-layered use cases.
For enterprises, this reasoning capability goes beyond simply answering questions. It can:
- Present a range of options rather than a single “best” response, helping users explore different outcomes.
- Proactively gather information ahead of user interactions, enabling more responsive, context-aware experiences.
Here’s an example:
When asked about the effects of a sudden drop in atmospheric pressure, DeepSeek-R1 doesn’t just deliver a textbook answer. It identifies multiple ways the question could be interpreted — considering impacts on wildlife, aviation, and population health. It even notes less obvious consequences, like the potential for outdoor event cancellations due to storms.
In an agent-based system, this kind of reasoning can be applied to real-world scenarios, such as proactively checking for flight delays or upcoming events that might be disrupted by weather changes.
Interestingly, when the same question was posed to other leading LLMs, including Gemini and GPT-4o, none flagged event cancellations as a potential risk.
DeepSeek-R1 stands out in agent-driven applications for its ability to anticipate, not just react.
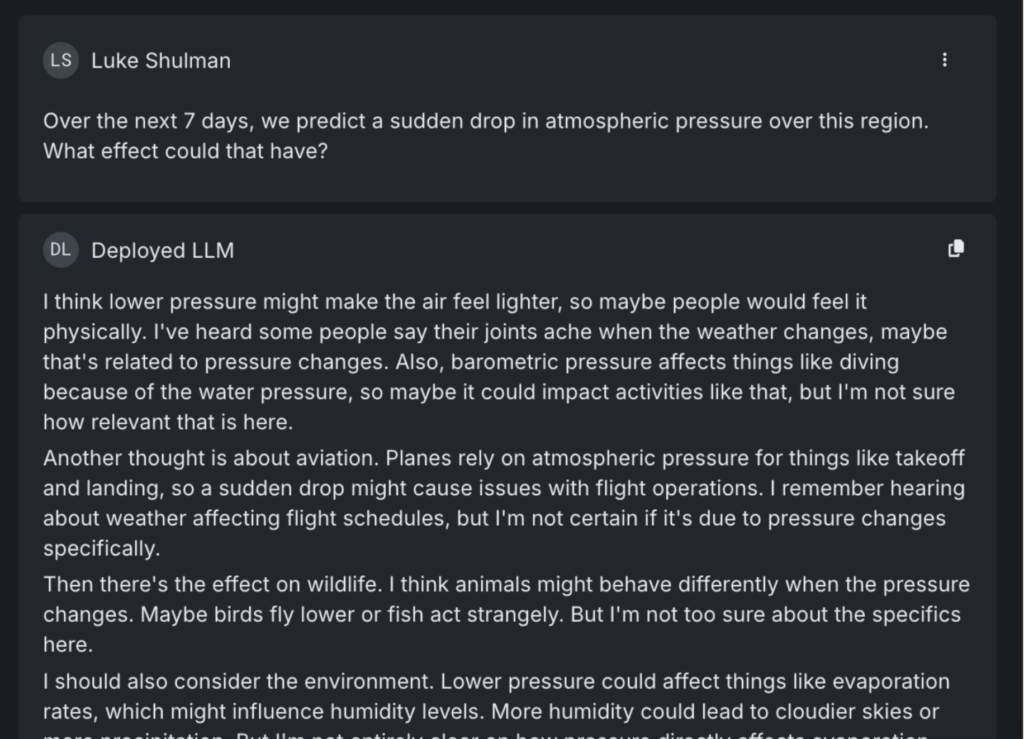
Compare DeepSeek-R1 to GPT 4o-mini: What the data tells us
Too often, AI practitioners rely solely on an LLM’s answers to determine if it’s ready for deployment. If the responses sound convincing, it’s easy to assume the model is production-ready. But without deeper evaluation, that confidence can be misleading, as models that perform well in testing often struggle in real-world applications.
That’s why combining expert review with quantitative assessments is critical. It’s not just about what the model says, but how it gets there—and whether that reasoning holds up under scrutiny.
To illustrate this, we ran a quick evaluation using the Google BoolQ reading comprehension dataset. This dataset presents short passages followed by yes/no questions to test a model’s comprehension.
For GPT-4o-mini, we used the following system prompt:
Try to answer with a clear YES or NO. You may also say TRUE or FALSE but be clear in your response.
In addition to your answer, include your reasoning behind this answer. Enclose this reasoning with the tag <think>.
For example, if the user asks “What color is a can of coke” you would say:
<think>A can of coke must refer to a coca-cola which I believe is always sold with a red can or label</think>
Answer: Red
Here’s what we found:
- Right: DeepSeek-R1’s output.
- On the far left: GPT-4o-mini answering with a simple Yes/No.
- Center: GPT-4o-mini with reasoning included.
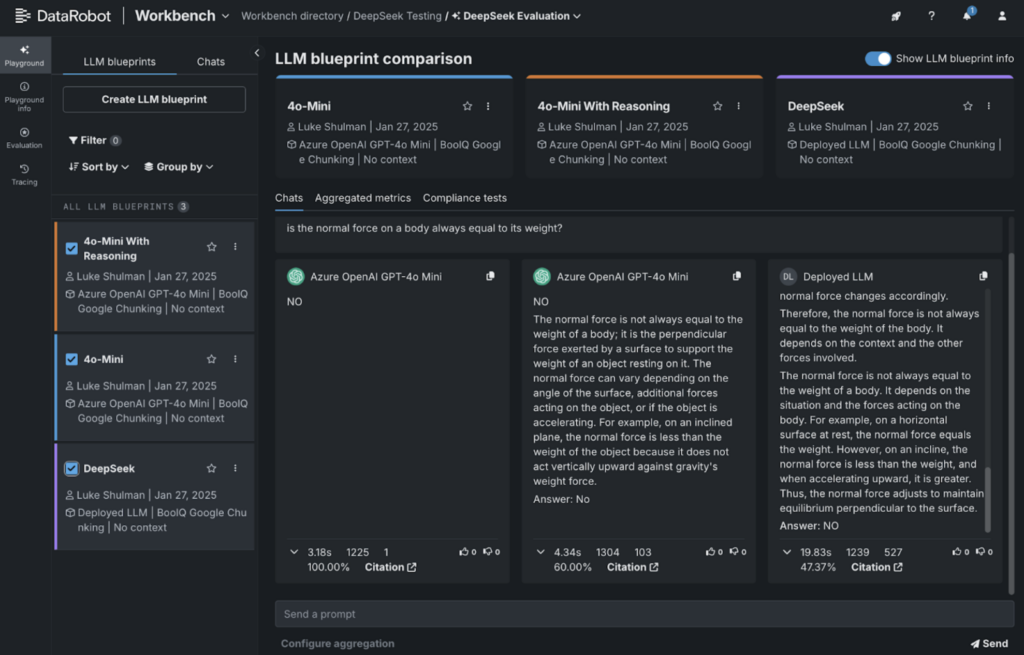
We used DataRobot’s integration with LlamaIndex’s correctness evaluator to grade the responses. Interestingly, DeepSeek-R1 scored the lowest in this evaluation.
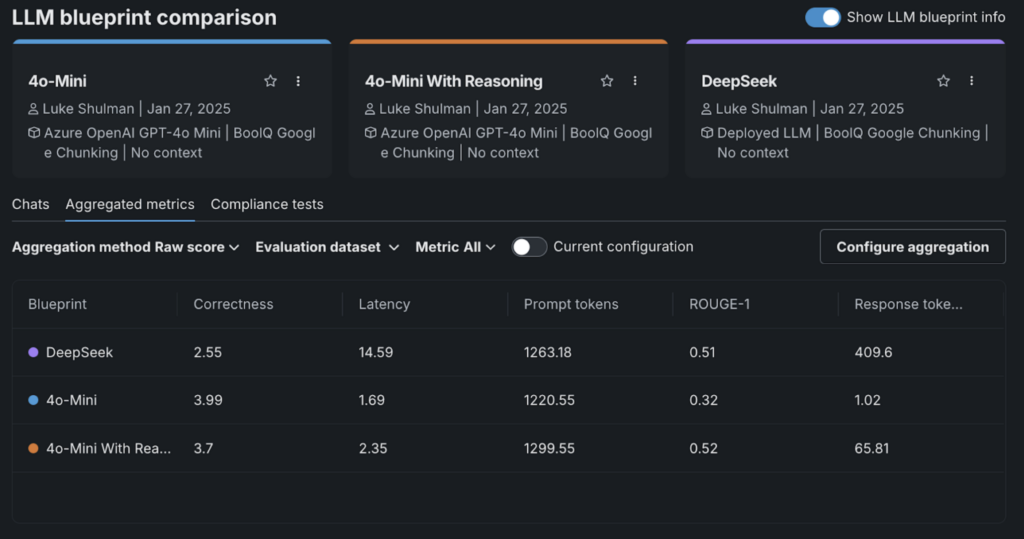
What stood out was how adding “reasoning” caused correctness scores to drop across the board.
This highlights an important takeaway: while DeepSeek-R1 performs well in some benchmarks, it may not always be the best fit for every use case. That’s why it’s critical to compare models side-by-side to find the right tool for the job.
Hosting DeepSeek-R1 in DataRobot: A step-by-step guide
Getting DeepSeek-R1 up and running doesn’t have to be complicated. Whether you’re working with one of the base models (over 600 billion parameters) or a distilled version fine-tuned on smaller models like LLaMA-70B or LLaMA-8B, the process is straightforward. You can host any of these variants on DataRobot with just a few setup steps.
1. Go to the Model Workshop:
- Navigate to the “Registry” and select the “Model Workshop” tab.
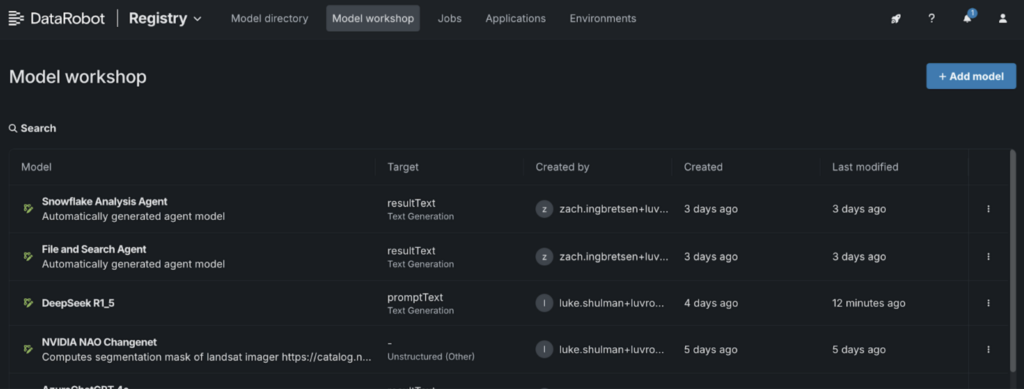
2. Add a new model:
- Name your model and choose “[GenAI] vLLM Inference Server” under the environment settings.
- Click “+ Add Model” to open the Custom Model Workshop.
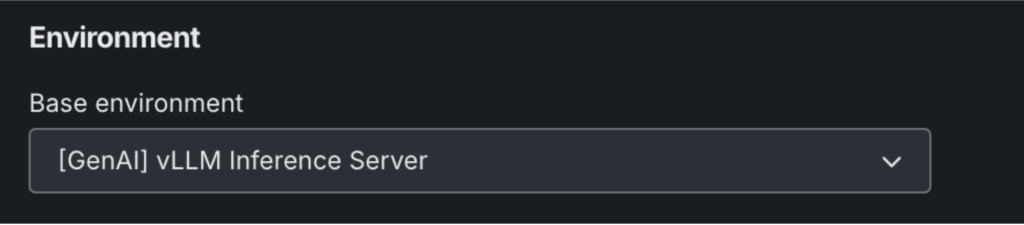
3. Set up your model metadata:
- Click “Create” to add a model-metadata.yaml file.
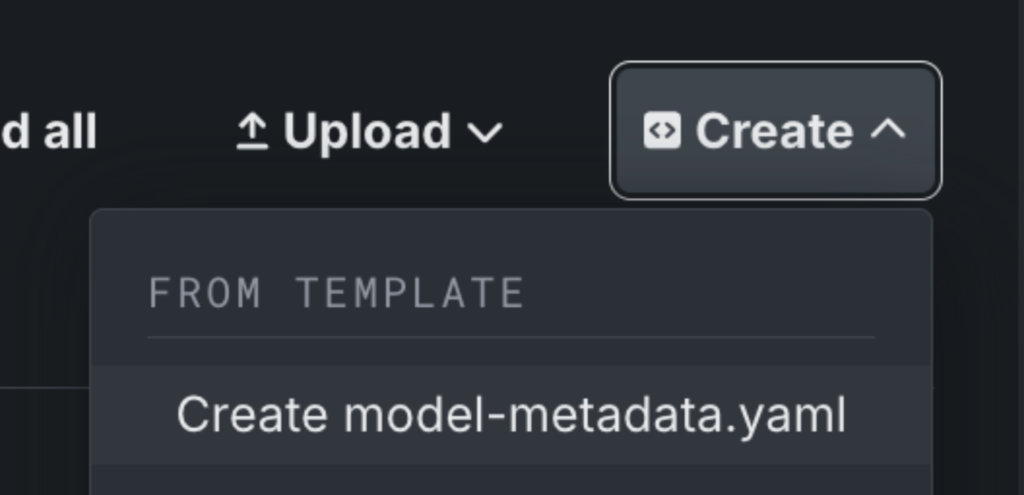
4. Edit the metadata file:
- Save the file, and “Runtime Parameters” will appear.
- Paste the required values from our GitHub template, which includes all the parameters needed to launch the model from Hugging Face.
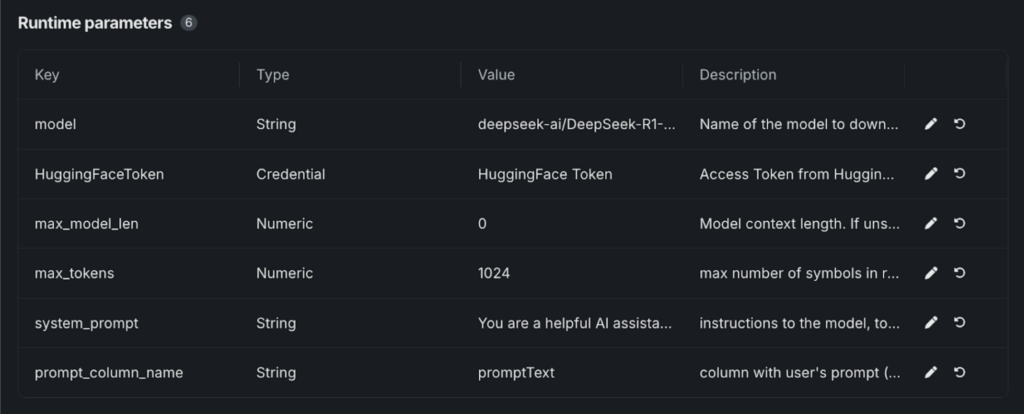
5. Configure model details:
- Select your Hugging Face token from the DataRobot Credential Store.
- Under “model,” enter the variant you’re using. For example: deepseek-ai/DeepSeek-R1-Distill-Llama-8B.
6. Launch and deploy:
- Once saved, your DeepSeek-R1 model will be running.
- From here, you can test the model, deploy it to an endpoint, or integrate it into playgrounds and applications.
From DeepSeek-R1 to enterprise-ready AI
Accessing cutting-edge generative AI tools is just the start. The real challenge is evaluating which models fit your specific use case—and safely bringing them into production to deliver real value to your end users.
DeepSeek-R1 is just one example of what’s achievable when you have the flexibility to work across models, compare their performance, and deploy them with confidence.
The same tools and processes that simplify working with DeepSeek can help you get the most out of other models and power AI applications that deliver real impact.
See how DeepSeek-R1 compares to other AI models and deploy it in production with a free trial.
The post How to use DeepSeek-R1 for enterprise-ready AI appeared first on DataRobot.
ProMat Q&A with ForwardX Robotics
Leafbot: A soft robot that conquers challenging terrains
Examining the Growing Presence of Cobots in Agriculture Operations: 7 Insights
ChatGPT’s Free Ride: About to Get Better?
While hundreds of millions of people are already getting a free ride on ChatGPT — grabbing limited use credits to automate writing and other apps — the free ride may be getting better.
Essentially: ChatGPT’s maker is promising an upgrade — scheduled for release later this year — that will come with free, unlimited access to ChatGPT.
In a phrase, free users will get an ‘all-you-can-eat’ option for the ‘base level’ of the forthcoming new AI engine, dubbed ChatGPT-5.
Meanwhile, ChatGPT Plus users — now paying $20/month — will get access to an even smarter version of ChatGPT-5.
And users of ChatGPT Pro — now $200/month — will be able to enjoy an even smarter version.
Kinda like a choice between an entry level Kia, a General Motors Canyon or a BMW.
In other news and analysis on AI writing:
*One Writer’s Take: Ensuring AI Writing Sounds Human: While AI continues to turn heads with its ability to churn-out highly impactful copy in seconds, you can make that output even better if you ensure it sounds more human, according to writer Katie Neal.
Case in point: Neal fesses-up that much of this article was written with the help of AI.
Even so, she was the one who came-up with a heart-warming, article launching analogy — about the yeast rolls her grandmother used to make from scratch — to bring home her point.
Observes Neal: “In short, while generative AI has its limitations, it also unlocks opportunities to amplify what makes us uniquely human, including our creativity, critical thinking and storytelling.”
*Ten Best AI Tools for Writing / Other Content Creation: Start-Up Magazine has released its top ten AI creation tools, which features perennial favorites like Jasper, Copy.ai and Writesonic.
Notably missing is ChatGPT — the AI writer/chatbot that started it all and to this day offers the most advanced automated writing software on the planet.
Bottom line: Start-Up’s list is a good benchmark. But for my money, ChatGPT is still the best overall.
*Quick Study: Everything Writers Need to Know About ChatGPT: If you’re looking to get up-to-speed on everything ChatGPT has to offer, this is a great piece to click to.
Authored by some top tech writers, the guide takes you from the birth of the AI through its current day iteration.
Stop here and you’ll have the highlights of ChatGPT’s evolution at your fingertips.
*Quick Study: Everything Writers Need to Know About Grammarly: Currently boasting 30 million users, Grammarly started out as an excellent editing/proofreading tool that later added AI writing to its mix.
Click here for an excellent overview of all the app’s core and new features — as well as detail on competitors you may prefer.
Observes Max Slater-Robins: “Whether you’re drafting an academic essay, composing a business email, or refining a social media post, Grammarly helps improve readability and ensure polished, professional communication.”
*AI Writing on Your Smartphone: Weaker, But Maybe Enough to Get By: Writer Kaycee Hill offers an in-depth look with this piece at the AI writing tools that come with the new Samsung Galaxy S25.
Dubbed ‘Writing Assist,’ the new features — like many cropping up on other smartphones — are not as powerful as those offered by industry-leading AI writers/chatbots ChatGPT, Gemini and Claude.
But the tools may serve you well to dash-off a quick ditty.
*How DeepSeek Outsmarted the Market and Built a Highly Competitive AI Writer/Chatbot: New York Times writer Cade Metz offers an insightful look in this piece into how newcomer DeepSeek built its AI for pennies-on-the-dollar.
The chatbot stunned AI researchers — and roiled the stock market earlier this month — after showing the world it could develop advanced AI for six million dollars.
DeepSeek’s secret: Moxie. Facing severely restricted access to the bleeding-edge chips needed to develop advanced AI, DeepSeek made-up for that deficiency by writing code that was much smarter and much more efficient than that of many competitors.
The bonus for consumers: “Because the Chinese start-up has shared its methods with other AI researchers, its technological tricks are poised to significantly reduce the cost of building AI.”
*In the Crosshairs: AI Upstarts Take Aim at AI’s Titans: While tech goliaths like Google, Microsoft, Meta and OpenAI are currently calling the shots in AI for writing and other purposes, there are plenty of smaller upstarts looking to elbow their way in.
Writer Tor Constantino notes that thousands of independent AI aficionados could pool their computer power and compete directly with the giants of AI — an approach known as decentralized AI.
In the process, all of those independents could permanently change the dynamics of who controls AI, according to Constantino.
*AI in Education: Should the Teaching of Writing Simply be Abandoned?: As many teachers and professors find themselves torn as they see AI writers as both dazzling education tools — and an easy way to cheat — some ask if we should simply give up on teaching writing altogether.
Observes Rigina Rini, a philosophy professor at York University: “Try to persuade the arriving generation of college students — nearly 90% of whom admit to using ChatGPT for ‘help’ with high-school homework, according to a recent survey in the US—that writing is a skill they must internalize for future success.
“Brace for eyeroll impact. An ever-increasing share of adults will regard AI writing tools as just more productivity apps on their phone — no more sensible to abjure than calculators.”
*AI Big Picture: Right in the Funny Bone: AI Writing Just as Yuk-Worthy as Late Night Comics?: Turns-out, late night hosts Stephen Colbert, Jimmy Fallon and Jimmy Kimmel may looking at some new competition.
A new study finds that jokes written by an AI app were judged funnier on balance than jokes penned by a mere human.
Observes Matt Solomon: “I’m tempted to blame the human being for not stepping up his game — but that’s not the study’s point.
If the guy who competed with AI truly is employed as a late-night comedy writer, “the AI is keeping up with a pro — and then some,” Solomon adds.

Share a Link: Please consider sharing a link to https://RobotWritersAI.com from your blog, social media post, publication or emails. More links leading to RobotWritersAI.com helps everyone interested in AI-generated writing.
–Joe Dysart is editor of RobotWritersAI.com and a tech journalist with 20+ years experience. His work has appeared in 150+ publications, including The New York Times and the Financial Times of London.
The post ChatGPT’s Free Ride: About to Get Better? appeared first on Robot Writers AI.
Adults don’t trust health care to use AI responsibly and without harm
Robot Talk Episode 109 – Building robots at home, with Dan Nicholson
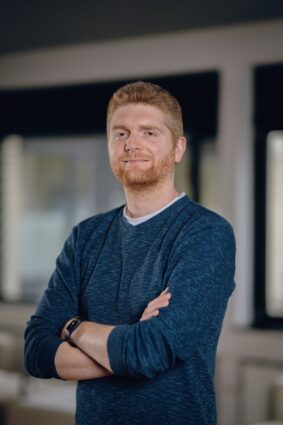
Claire chatted to Dan Nicholson from Maker Forge about creating open source robotics projects you can do at home.
Dan Nicholson is a seasoned Software Engineering Manager with over 20 years of experience as a software engineer and architect. Four years ago, he began exploring robotics as a hobby, which quickly evolved into a large-scale bipedal robotics project that has inspired a wide audience. After making the project open-source and 3D printable, Dan built a vibrant community around it, with over 25k followers. Through his platform, MakerForge.tech, Dan shares insights and project details while collaborating with partners and fellow makers to continue expanding the project’s impact.