Happy holidays everyone! Here are some more robot videos to get you into the holiday spirit.
Have a last minute holiday robot video of your own that you’d like to share? Send your submissions to sabine.hauert@robohub.org. Read More
Robots with sticky feet can climb up, down, and all around
By Lindsay Brownell
Jet engines can have up to 25,000 individual parts, making regular maintenance a tedious task that can take over a month per engine. Many components are located deep inside the engine and cannot be inspected without taking the machine apart, adding time and costs to maintenance. This problem is not only confined to jet engines, either; many complicated, expensive machines like construction equipment, generators, and scientific instruments require large investments of time and money to inspect and maintain.
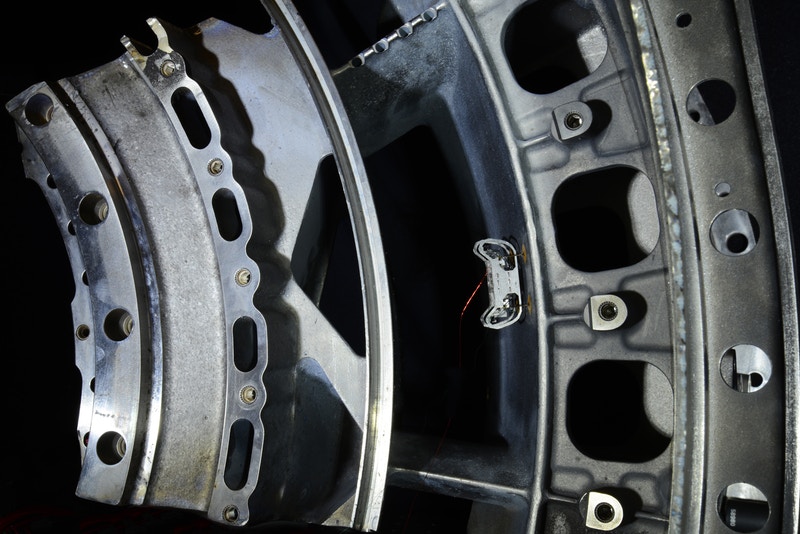
Researchers at Harvard University’s Wyss Institute for Biologically Inspired Engineering and John A. Paulson School of Engineering and Applied Sciences (SEAS) have created a micro-robot whose electroadhesive foot pads, origami ankle joints, and specially engineered walking gait allow it to climb on vertical and upside-down conductive surfaces, like the inside walls of a commercial jet engine. The work is reported in Science Robotics.
“Now that these robots can explore in three dimensions instead of just moving back and forth on a flat surface, there’s a whole new world that they can move around in and engage with,” said first author Sébastien de Rivaz, a former Research Fellow at the Wyss Institute and SEAS who now works at Apple. “They could one day enable non-invasive inspection of hard-to-reach areas of large machines, saving companies time and money and making those machines safer.”
The new robot, called HAMR-E (Harvard Ambulatory Micro-Robot with Electroadhesion), was developed in response to a challenge issued to the Harvard Microrobotics Lab by Rolls-Royce, which asked if it would be possible to design and build an army of micro-robots capable of climbing inside parts of its jet engines that are inaccessible to human workers. Existing climbing robots can tackle vertical surfaces, but experience problems when trying to climb upside-down, as they require a large amount of adhesive force to prevent them from falling.
The team based HAMR-E on one of its existing micro-robots, HAMR, whose four legs enable it to walk on flat surfaces and swim through water. While the basic design of HAMR-E is similar to HAMR, the scientists had to solve a series of challenges to get HAMR-E to successfully stick to and traverse the vertical, inverted, and curved surfaces that it would encounter in a jet engine.
First, they needed to create adhesive foot pads that would keep the robot attached to the surface even when upside-down, but also release to allow the robot to “walk” by lifting and placing its feet. The pads consist of a polyimide-insulated copper electrode, which enables the generation of electrostatic forces between the pads and the underlying conductive surface. The foot pads can be easily released and re-engaged by switching the electric field on and off, which operates at a voltage similar to that required to move the robot’s legs, thus requiring very little additional power. The electroadhesive foot pads can generate shear forces of 5.56 grams and normal forces of 6.20 grams – more than enough to keep the 1.48-gram robot from sliding down or falling off its climbing surface. In addition to providing high adhesive forces, the pads were designed to be able to flex, thus allowing the robot to climb on curved or uneven surfaces.
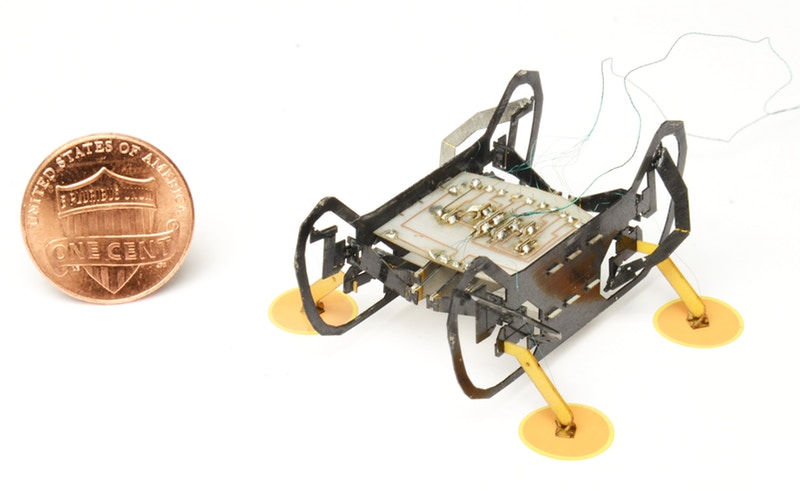
The scientists also created new ankle joints for HAMR-E that can rotate in three dimensions to compensate for rotations of its legs as it walks, allowing it to maintain its orientation on its climbing surface. The joints were manufactured out of layered fiberglass and polyimide, and folded into an origami-like structure that allows the ankles of all the legs to rotate freely, and to passively align with the terrain as HAMR-E climbs.
Finally, the researchers created a special walking pattern for HAMR-E, as it needs to have three foot pads touching a vertical or inverted surface at all times to prevent it from falling or sliding off. One foot releases from the surface, swings forward, and reattaches while the remaining three feet stay attached to the surface. At the same time, a small amount of torque is applied by the foot diagonally across from the lifted foot to keep the robot from moving away from the climbing surface during the leg-swinging phase. This process is repeated for the three other legs to create a full walking cycle, and is synchronized with the pattern of electric field switching on each foot.
When HAMR-E was tested on vertical and inverted surfaces, it was able to achieve more than one hundred steps in a row without detaching. It walked at speeds comparable to other small climbing robots on inverted surfaces and slightly slower than other climbing robots on vertical surfaces, but was significantly faster than other robots on horizontal surfaces, making it a good candidate for exploring environments that have a variety of surfaces in different arrangements in space. It is also able to perform 180-degree turns on horizontal surfaces.
HAMR-E also successfully maneuvered around a curved, inverted section of a jet engine while staying attached, and its passive ankle joints and adhesive foot pads were able to accommodate the rough and uneven features of the engine surface simply by increasing the electroadhesion voltage.
This iteration of HAMR-E is the first and most convincing step towards showing that this approach to a centimeter-scale climbing robot is possible, and that such robots could in the future be used to explore any sort of infrastructure, including buildings, pipes, engines, generators, and more
ROBERT WOOD
The team is continuing to refine HAMR-E, and plans to incorporate sensors into its legs that can detect and compensate for detached foot pads, which will help prevent it from falling off of vertical or inverted surfaces. HAMR-E’s payload capacity is also greater than its own weight, opening the possibility of carrying a power supply and other electronics and sensors to inspect various environments. The team is also exploring options for using HAMR-E on non-conductive surfaces.
“This iteration of HAMR-E is the first and most convincing step towards showing that this approach to a centimeter-scale climbing robot is possible, and that such robots could in the future be used to explore any sort of infrastructure, including buildings, pipes, engines, generators, and more,” said corresponding author Robert Wood, Ph.D., who is a Founding Core Faculty member of the Wyss Institute as well as the Charles River Professor of Engineering and Applied Sciences at SEAS.
“While academic scientists are very good at coming up with fundamental questions to explore in the lab, sometimes collaborations with industrial scientists who understand real-world problems are required to develop innovative technologies that can be translated into useful products. We are excited to help catalyze these collaborations here at the Wyss Institute, and to see the breakthrough advances that emerge,” said Wyss Founding Director Donald Ingber, M.D., Ph.D., who is also the Judah Folkman Professor of Vascular Biology at Harvard Medical School and the Vascular Biology Program at Boston Children’s Hospital, and Professor of Bioengineering at SEAS.
This study is co-authored by Benjamin Goldberg, Ph.D., Neel Doshi, Kaushik Jayaram, Ph.D., and Jack Zhou from the Wyss Institute and Harvard SEAS.
Support for this research was contributed by the Wyss Institute for Biologically Inspired Engineering at Harvard University, Rolls-Royce, and the US Army Research Office.
NVIDIA Jetson AGX Xavier Module for Next-Gen Autonomous Machines
The Growth of Non-Industrial Robotics Is Driving a Diversification of New Robotics Applications, According to Tractica
Why AI robot toys could be good for kids
Growing bio-inspired shapes with a 300-robot swarm
Work by I. Slavkov, D. Carrillo-Zapata, N. Carranza, X. Diego, F. Jansson, J. Kaandorp, S. Hauert, J. Sharpe
Our work published today in Science Robotics describes how we grow fully self-organised shapes using a swarm of 300 coin-sized robots. The work was led by James Sharpe at EMBL and the Centre for Genomic Regulation (CRG) in Barcelona – together with my team at the Bristol Robotics Laboratory and University of Bristol.
Here’s a video summarising the results, or you can read the paper here:
Self-organised shapes
Nature is capable of producing impressive functional shapes throughout embryonic development. Broadly, there are two ways to form these shapes.
1) Top-down control. Cells have access to information about their position through some coordinate system, for example generated through their molecular gradients. Cells use this information to decide their fate, which ultimately creates the shapes. There are beautiful examples of this strategy being used for robot swarms, check here for work by Rubenstein et al. (video).
2) Local self-organisation. Cells generate reaction-diffusion systems, such as those described by Alan Turing, resulting in simple periodic patterns. Cells can use these patterns to decide their fate and the resulting shape.
We use the second strategy, here’s how it works.
Patterning
We start from a swarm of 300 closely packed robots in a disc – each running the same code. Each robot stores two morphogens u and v, which you can think of as virtual chemical signals. Morphogen u activates itself and the other morphogen v, whereas v inhibits itself and the other morphogen u – this is a ‘reaction’ network. ‘Diffusion’ of u and v happens through communication from robot to robot. Symmetry breaking caused by the ‘reaction-diffusion’ system results in spots emerging on the swarm (or stripes if we change the parameters!). Areas with high-levels of morphogens are shown in green – that’s what we call a “Turing spot”.
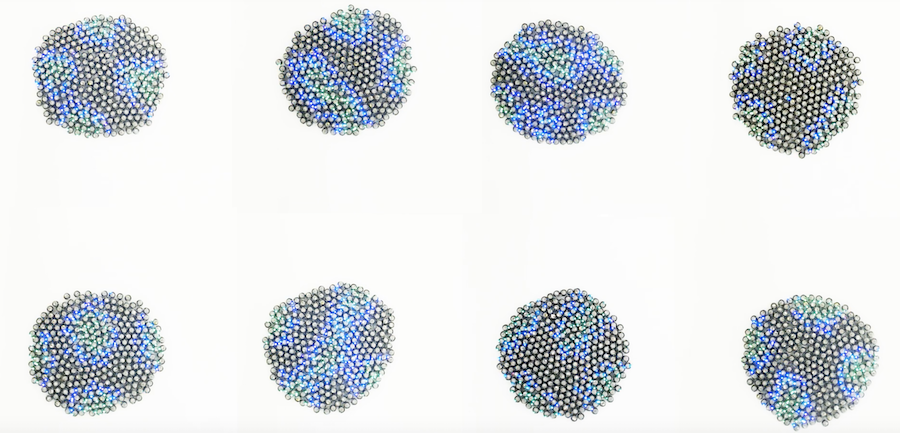
Tissue movement
In biology, cells may die or multiply depending on their patterning. As we can’t do either of those things with robots, we simply move robots from areas where they are no longer needed to areas of growth. The general idea is that robots that are on the edge of the swarm, and are not in a Turing spot, move along the edge of the swarm until they are near the spot. This causes protrusions to grow at the location of the Turing spots.

Following these simple rules, we are able to grow shapes in a repeatable manner, although all the shapes are slightly different. If you watch the video, you’ll see that these shapes look quite organic. We did over 20 experiments with large robot swarms, each one taking about 3 hours.
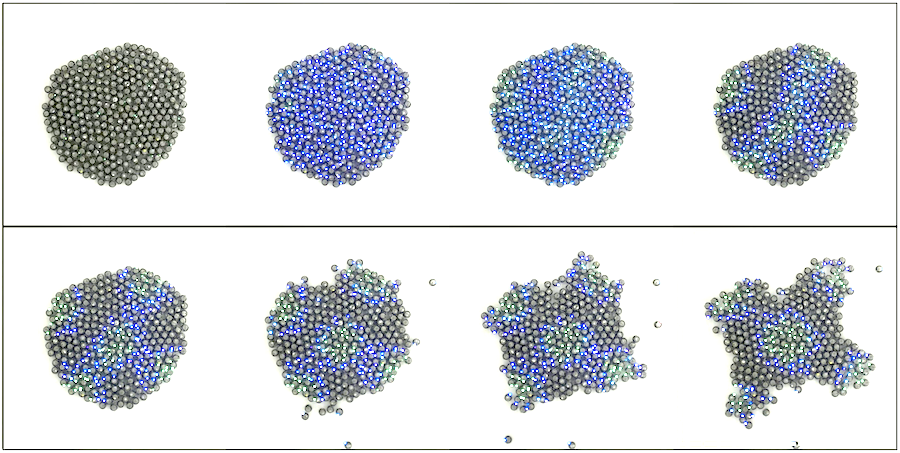
Because the rules are so simple, and only rely on local information, we get adaptability and robustness for free.
Adaptability
First, as the shape grows, the Turing spots move, showing that the patterning adapts to the shape of the swarm, and that the shape further adapts to the patterning. Second, we can easily change the starting configuration of the swarm (smaller number of robots, or a ‘rectangular’ starting conditions) and the shape still forms.
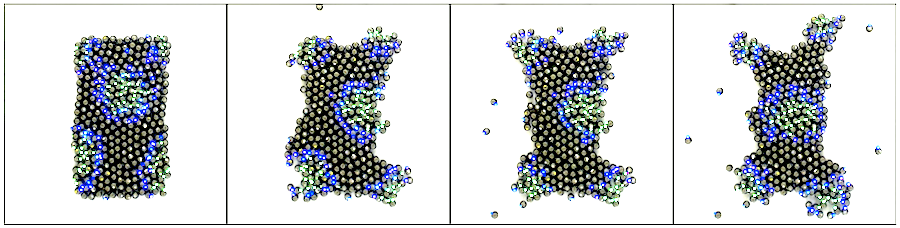
Robustness
Chopping off a protrusion, causes the robots to regrow it, or to reallocate robots to other protrusions in the swarm. Splitting the swarm causes it to self-heal.
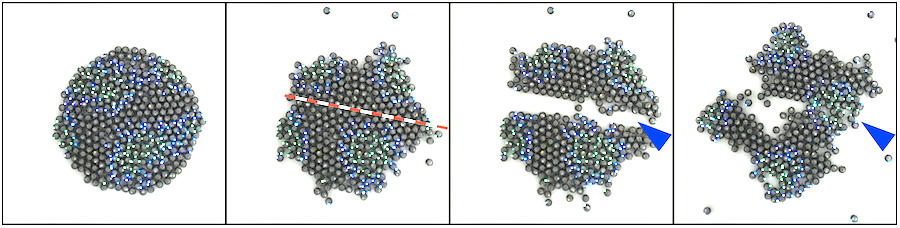
Potential for real world applications
While inspiration was taken from nature to grow the swarm shapes, the goal is ultimately to make large robot swarms for real-world applications. Imagine hundreds or thousands of tiny biodegradable robots growing shapes to explore a disaster environment after an earthquake or fire, or sculpting themselves into a dynamic 3D structure such as a temporary bridge that could automatically adjust its size and shape to fit any building or terrain. There is still a long way to go however, before we see such swarms outside the laboratory.
Team
James Sharpe (EMBL Barcelona) led the Swarm-Organ project, which was initiated at the Centre for Genomic Regulation (CRG) when Sharpe was a group leader there. Sabine Hauert (Bristol Robotics Laboratory and University of Bristol) was the key senior collaborator. Other collaborators were Fredrik Jansson (currently employed at Centrum Wiskunde & Informatica – CWI) and Jaap Kaandorp (University of Amsterdam – UvA).
Paper
You can read more in the paper Slavkov, I., Zapata D. C. et al., Science Robotics (2018).
Funding
The research leading to these results has received funding from the European Union Seventh Framework Programme (FP7) under grant agreement n° 601062, and the EPSRC Centre for Doctoral Training in Future Autonomous and Robotic Systems (FARSCOPE) at the Bristol Robotics Laboratory.
When high tech goes underground
From robotic companions to third thumbs, machines can change the human brain
Robots are being programmed to adapt in real time
It Takes a Swarm: These Robots Talk to Each Other, Make Decisions as a Group
MiR200 Improves Safety, Quality, and Competitiveness at Metro Plastics
Autonomous Mobility Grows Its Sea Legs — Toyota AI Investment in Sea Machines
Call for robot holiday videos 2018
That’s right! You better not run, you better not hide, you better watch out for brand new robot holiday videos on Robohub! Drop your submissions down our chimney at sabine.hauert@robohub.org and share the spirit of the season.
For inspiration, here are our first submissions .
Robot makes world-first baby coral delivery to Great Barrier Reef
In a world first, an undersea robot has dispersed microscopic baby corals (coral larvae) to help scientists working to repopulate parts of the Great Barrier Reef during this year’s mass coral spawning event.
Six weeks after winning the Great Barrier Reef Foundation’s $300,000 Out of the Blue Box Reef Innovation Challenge, Southern Cross University’s Professor Peter Harrison and QUT’s Professor Matthew Dunbabin trialled the ground-breaking initiative on Vlasoff Reef, near Cairns in north Queensland.
Professor Dunbabin engineered QUT’s reef protector RangerBot into LarvalBot specifically for the coral restoration project led by Professor Harrison.
The project builds on Professor Harrison’s successful larval reseeding technique piloted on the southern Great Barrier Reef in 2016 and 2017 in collaboration with the Great Barrier Reef Foundation, the Great Barrier Reef Marine Park Authority (GBRMPA) and Queensland Parks & Wildlife Service (QPWS), following successful small scale trials in the Philippines funded by the Australian Centre for International Agricultural Research.
Watch interview below
“This year represents a big step up for our larval restoration research and the first time we’ve been able to capture coral spawn on a bigger scale using large floating spawn catchers then rearing them into tiny coral larvae in our specially constructed larval pools and settling them on damaged reef areas,” Professor Harrison said.
“Winning the GBRF’s Reef Innovation Challenge meant that we could increase the scale of the work planned for this year using mega-sized spawn catchers and fast track an initial trial of LarvalBot as a novel method of dispersing the coral larvae out on to the Reef.
“With further research and refinement, this technique has enormous potential to operate across large areas of reef and multiple sites in a way that hasn’t previously been possible.
“We’ll be closely monitoring the progress of settled baby corals over coming months and working to refine both the technology and the technique to scale up further in 2019.”
This research and the larval production process was also directly supported by the recent successful SBIR 2018 Coral larval restoration research project on Vlasoff Reef led by Professor Harrison with Katie Chartrand (James Cook University) and Associate Professor David Suggett (University of Technology Sydney), in collaboration with Aroona Boat Charters, the GBRMPA and QPWS.
With a current capacity to carry around 100,000 coral larvae per mission and plans to scale up to millions of larvae, the robot gently releases the larvae onto damaged reef areas allowing it to settle and over time develop into coral polyps or baby corals.
Professor Dunbabin said LarvalBot could be compared to ‘an underwater crop duster’ operating very safely to ensure existing coral wasn’t disturbed.
“During this year’s trial, the robot was tethered so it could be monitored precisely but future missions will see it operate alone and on a much larger scale,” Professor Dunbabin said.
“Using an iPad to program the mission, a signal is sent to deliver the larvae and it is gently pushed out by LarvalBot. It’s like spreading fertiliser on your lawn.
“The robot is very smart, and as it glides along we target where the larvae need to be distributed so new colonies can form and new coral communities can develop.
“We have plans to do this again in Australia and elsewhere and I’m looking forward to working with Professor Harrison and Southern Cross University, the Great Barrier Reef Foundation and other collaborators to help tackle an important problem.”
This project builds on the work by Professor Dunbabin who developed RangerBot to help control the coral-killing crown-of-thorns starfish which is responsible for 40 per cent of the reef’s decline in coral cover.
Great Barrier Reef Foundation Managing Director Anna Marsden said, “It’s exciting to see this project progress from concept to implementation in a matter of weeks, not years. The recent IPCC report highlights that we have a very short window in which to act for the long term future of the Reef, underscoring the importance of seeking every opportunity to give our reefs a fighting chance.
“This project is testament to the power of collaboration between science, business and philanthropy. With the support of the Tiffany & Co. Foundation, whose longstanding support for coral reef conservation globally spans almost two decades, our international call for innovations to help the Reef has uncovered a solution that holds enormous promise for restoring coral reefs at scales never before possible.”
Following the success of this initial trial in 2018, the researchers plan to fully implement their challenge-winning proposal in 2019, building even larger mega spawn-catchers and solar powered floating larval incubation pools designed to rear hundreds of millions of genetically diverse, heat-tolerant coral larvae to be settled on damaged reefs through a combination of larval clouds and LarvalBots.
A new drone can change its shape to fly through a narrow gap
A research team from the University of Zurich and EPFL has developed a new drone that can retract its propeller arms in flight and make itself small to fit through narrow gaps and holes. This is particularly useful when searching for victims of natural disasters.
Inspecting a damaged building after an earthquake or during a fire is exactly the kind of job that human rescuers would like drones to do for them. A flying robot could look for people trapped inside and guide the rescue team towards them. But the drone would often have to enter the building through a crack in a wall, a partially open window, or through bars – something the typi-cal size of a drone does not allow.
To solve this problem, researchers from Scaramuzza lab at the University of Zurich and Floreano lab at EPFL created a new kind of drone. Both groups are part of the National Centre of Competence in Research (NCCR) Robotics funded by the Swiss National Science Foundation. Inspired by birds that fold their wings in mid-air to cross narrow passages, the new drone can squeeze itself to pass through gaps and then go back to its previous shape, all the while continuing to fly. And it can even hold and transport objects along the way.
Mobile arms can fold around the main frame
“Our solution is quite simple from a mechanical point of view, but it is very versatile and very au-tonomous, with onboard perception and control systems,” explains Davide Falanga, researcher at the University of Zurich and the paper’s first author. In comparison to other drones, this morphing drone can maneuver in tight spaces and guarantee a stable flight at all times.
The Zurich and Lausanne teams worked in collaboration and designed a quadrotor with four pro-pellers that rotate independently, mounted on mobile arms that can fold around the main frame thanks to servo-motors. The ace in the hole is a control system that adapts in real time to any new position of the arms, adjusting the thrust of the propellers as the center of gravity shifts.
“The morphing drone can adopt different configurations according to what is needed in the field,” adds Stefano Mintchev, co-author and researcher at EPFL. The standard configuration is X-shaped, with the four arms stretched out and the propellers at the widest possible distance from each other. When faced with a narrow passage, the drone can switch to a “H” shape, with all arms lined up along one axis or to a “O” shape, with all arms folded as close as possible to the body. A “T” shape can be used to bring the onboard camera mounted on the central frame as close as possible to objects that the drone needs to inspect.
First step to fully autonomous rescue searches
In the future, the researchers hope to further improve the drone structure so that it can fold in all three dimensions. Most importantly, they want to develop algorithms that will make the drone truly autonomous, allowing it to look for passages in a real disaster scenario and automatically choose the best way to pass through them. “The final goal is to give the drone a high-level instruction such as ‘enter that building, inspect every room and come back’ and let it figure out by itself how to do it,” says Falanga.
Literature
Davide Falanga, Kevin Kleber, Stefano Mintchev, Dario Floreano, Davide Scaramuzza. The Foldable Drone: A Morphing Quadrotor that can Squeeze and Fly. IEEE Robotics and Auto-mation Letter, 10 December 2018. DOI:10.1109/LRA.2018.2885575