The Role of Robotics in Developing Advanced Autopilot Capabilities
Engineers unlock design for record-breaking robot that could jump twice the height of Big Ben
New soft robotic gripper designed with graphene and liquid crystals
Advanced artificial intelligence: A revolution for sustainable agriculture
Why Do You Need Cross-Environment AI Observability?
AI Observability in Practice
Many organizations start off with good intentions, building promising AI solutions, but these initial applications often end up disconnected and unobservable. For instance, a predictive maintenance system and a GenAI docsbot might operate in different areas, leading to sprawl. AI Observability refers to the ability to monitor and understand the functionality of generative and predictive AI machine learning models throughout their life cycle within an ecosystem. This is crucial in areas like Machine Learning Operations (MLOps) and particularly in Large Language Model Operations (LLMOps).
AI Observability aligns with DevOps and IT operations, ensuring that generative and predictive AI models can integrate smoothly and perform well. It enables the tracking of metrics, performance issues, and outputs generated by AI models –providing a comprehensive view through an organization’s observability platform. It also sets teams up to build even better AI solutions over time by saving and labeling production data to retrain predictive or fine-tune generative models. This continuous retraining process helps maintain and enhance the accuracy and effectiveness of AI models.
However, it isn’t without challenges. Architectural, user, database, and model “sprawl” now overwhelm operations teams due to longer set up and the need to wire multiple infrastructure and modeling pieces together, and even more effort goes into continuous maintenance and update. Handling sprawl is impossible without an open, flexible platform that acts as your organization’s centralized command and control center to manage, monitor, and govern the entire AI landscape at scale.
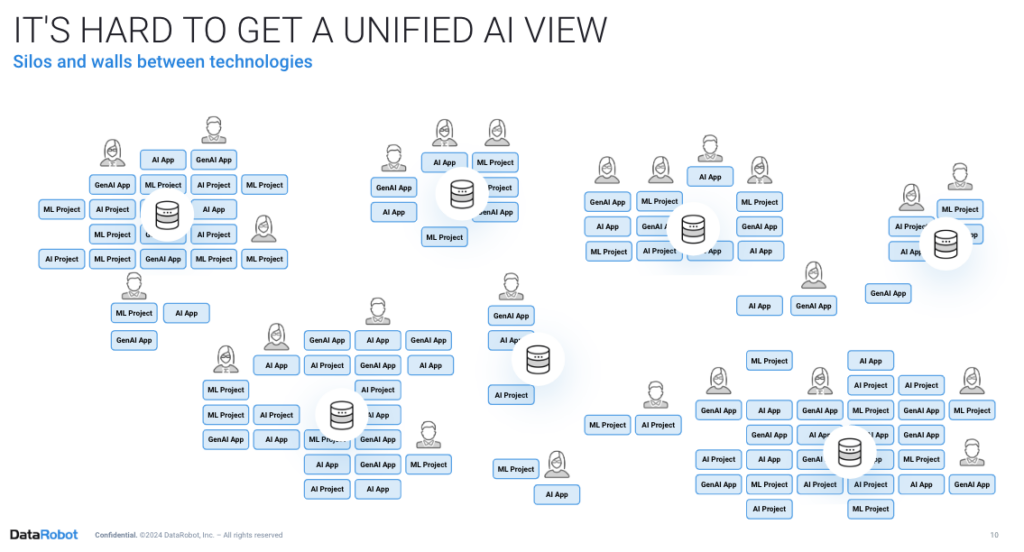
Most companies don’t just stick to one infrastructure stack and might switch things up in the future. What’s really important to them is that AI production, governance, and monitoring stay consistent.
DataRobot is committed to cross-environment observability – cloud, hybrid and on-prem. In terms of AI workflows, this means you can choose where and how to develop and deploy your AI projects while maintaining complete insights and control over them – even at the edge. It’s like having a 360-degree view of everything.
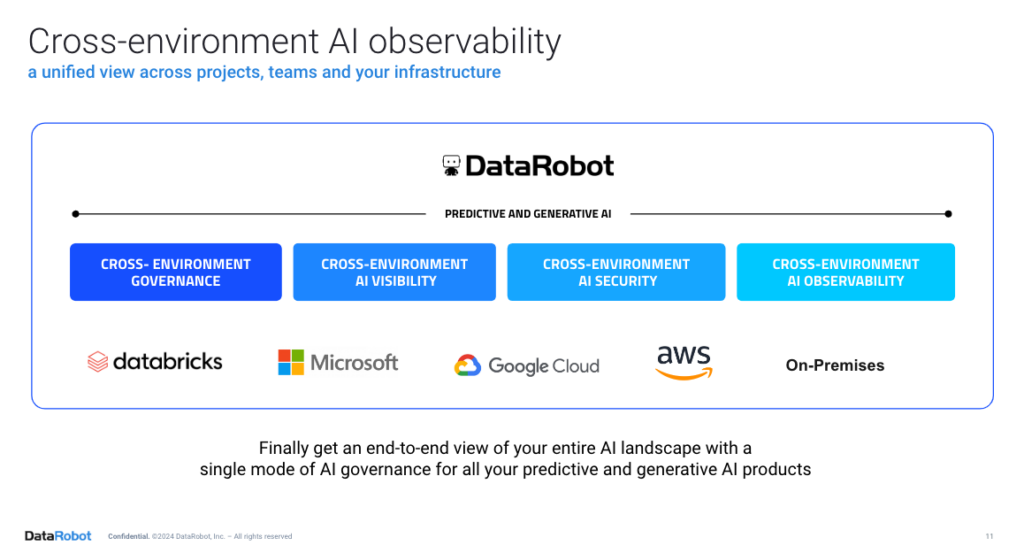
DataRobot offers 10 main out-of-the-box components to achieve a successful AI observability practice:
- Metrics Monitoring: Tracking performance metrics in real-time and troubleshooting issues.
- Model Management: Using tools to monitor and manage models throughout their lifecycle.
- Visualization: Providing dashboards for insights and analysis of model performance.
- Automation: Automating building, governance, deployment, monitoring, retraining stages in the AI lifecycle for smooth workflows.
- Data Quality and Explainability: Ensuring data quality and explaining model decisions.
- Advanced Algorithms: Employing out-of-the-box metrics and guards to enhance model capabilities.
- User Experience: Enhancing user experience with both GUI and API flows.
- AIOps and Integration: Integrating with AIOps and other solutions for unified management.
- APIs and Telemetry: Using APIs for seamless integration and collecting telemetry data.
- Practice and Workflows: Creating a supportive ecosystem around AI observability and taking action on what is being observed.
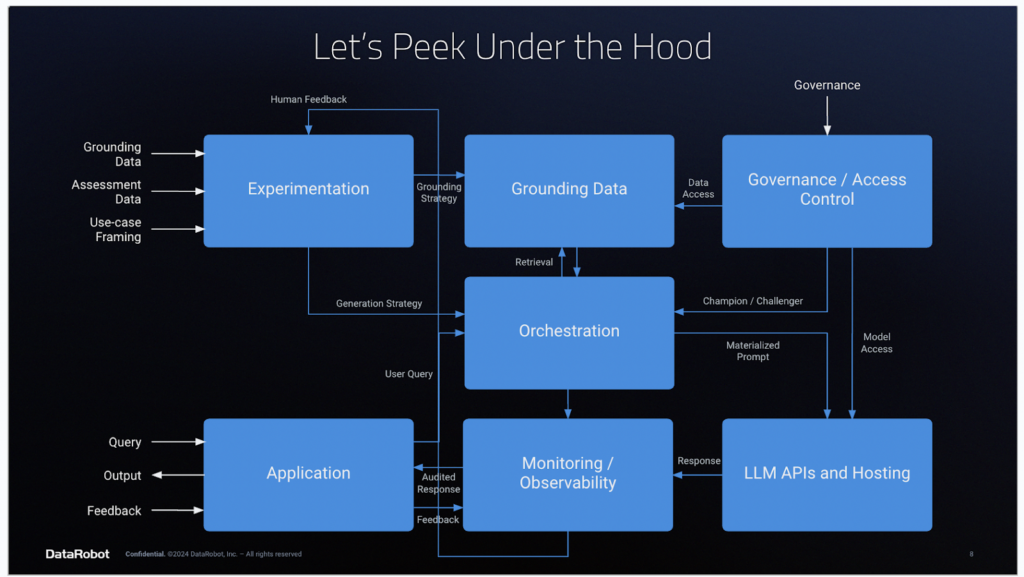
AI Observability In Action
Every industry implements GenAI Chatbots across various functions for distinct purposes. Examples include increasing efficiency, enhancing service quality, accelerating response times, and many more.
Let’s explore the deployment of a GenAI chatbot within an organization and discuss how to achieve AI observability using an AI platform like DataRobot.
Step 1: Collect relevant traces and metrics
DataRobot and its MLOps capabilities provide world-class scalability for model deployment. Models across the organization, regardless of where they were built, can be supervised and managed under one single platform. In addition to DataRobot models, open-source models deployed outside of DataRobot MLOps can also be managed and monitored by the DataRobot platform.
AI observability capabilities within the DataRobot AI platform help ensure that organizations know when something goes wrong, understand why it went wrong, and can intervene to optimize the performance of AI models continuously. By tracking service, drift, prediction data, training data, and custom metrics, enterprises can keep their models and predictions relevant in a fast-changing world.
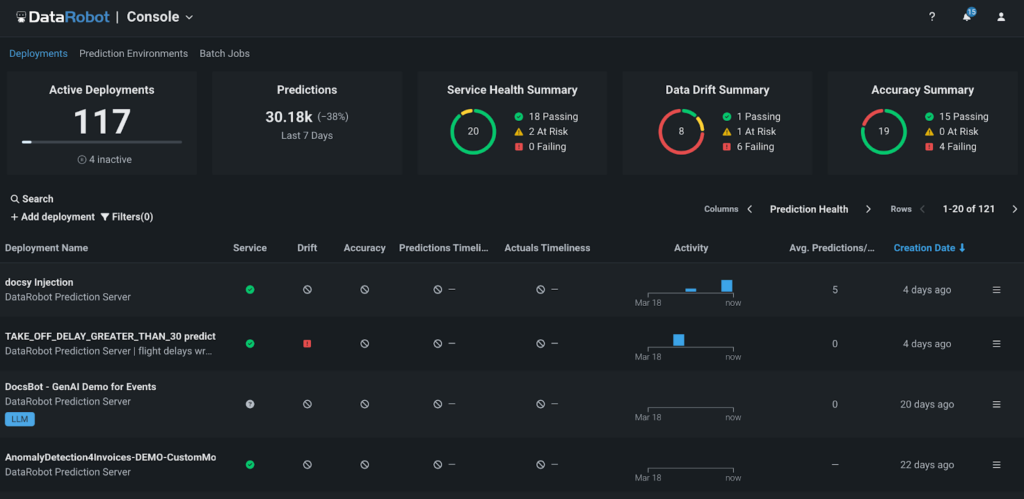
Step 2: Analyze data
With DataRobot, you can utilize pre-built dashboards to monitor traditional data science metrics or tailor your own custom metrics to address specific aspects of your business.
These custom metrics can be developed either from scratch or using a DataRobot template. Use those metrics for the models built or hosted in DataRobot or outside of it.
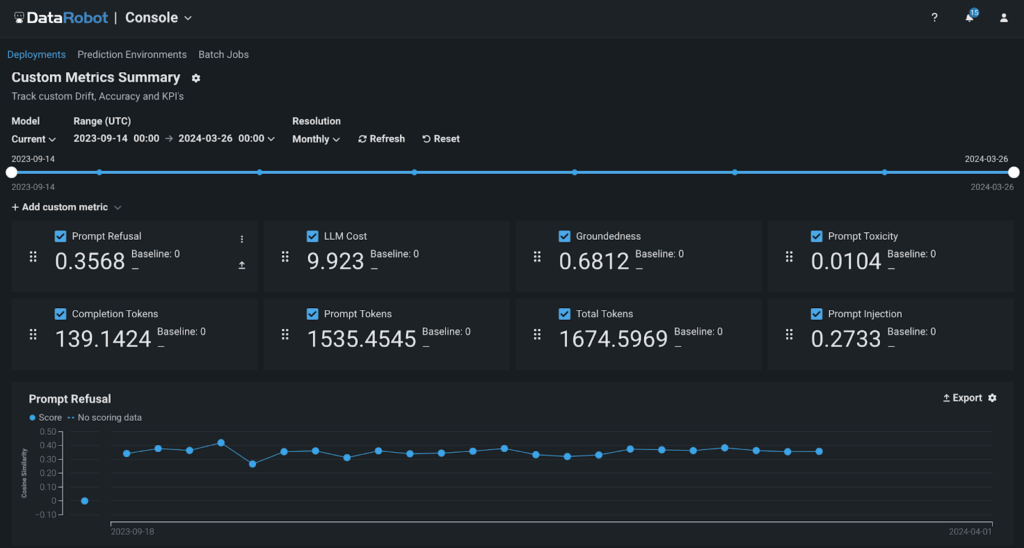
‘Prompt Refusal’ metrics represent the percentage of the chatbot responses the LLM couldn’t address. While this metric provides valuable insight, what the business truly needs are actionable steps to minimize it.
Guided questions: Answer these to provide a more comprehensive understanding of the factors contributing to prompt refusals:
- Does the LLM have the appropriate structure and data to answer the questions?
- Is there a pattern in the types of questions, keywords, or themes that the LLM cannot address or struggles with?
- Are there feedback mechanisms in place to collect user input on the chatbot’s responses?
Use-feedback Loop: We can answer these questions by implementing a use-feedback loop and building an application to find the “hidden information”.
Below is an example of a Streamlit application that provides insights into a sample of user questions and topic clusters for questions the LLM couldn’t answer.
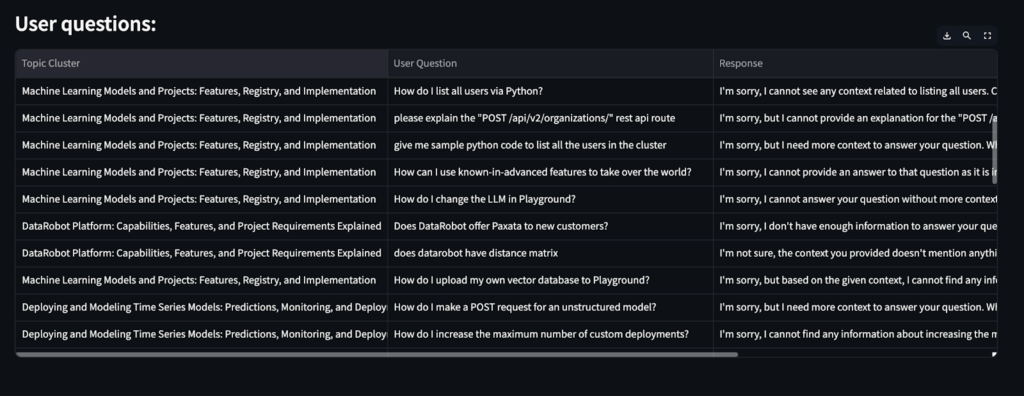
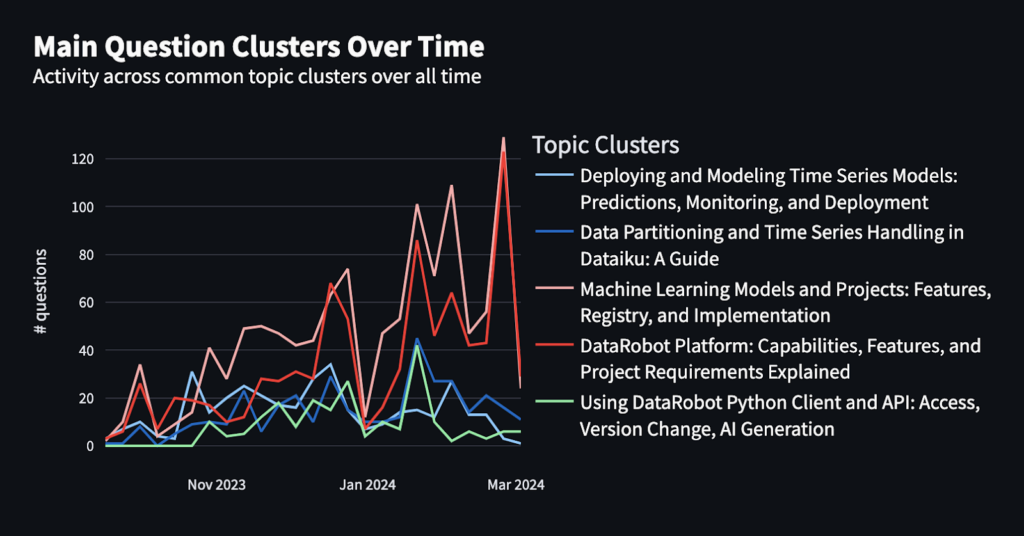
Step 3: Take actions based on analysis
Now that you have a grasp of the data, you can take the following steps to enhance your chatbot’s performance significantly:
- Modify the prompt: Try different system prompts to get better and more accurate results.
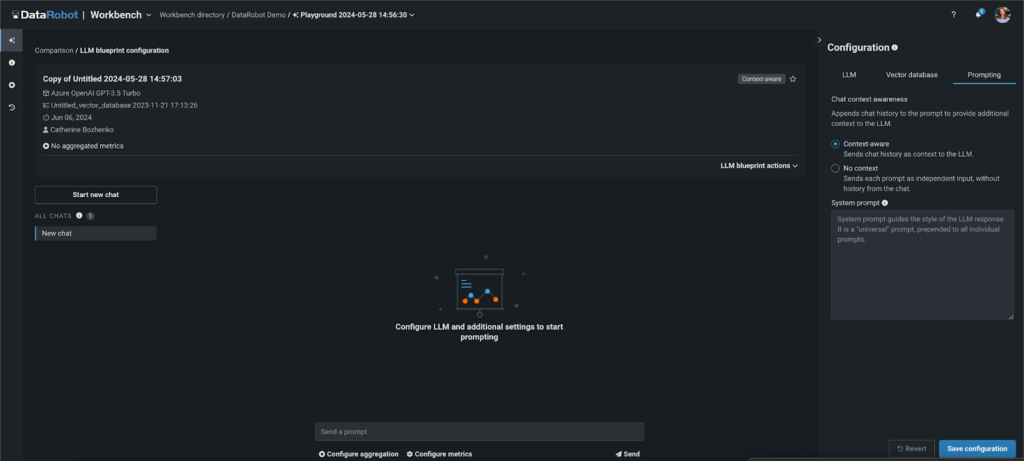
- Improve Your Vector database: Identify the questions the LLM didn’t have answers to, add this information to your knowledge base, and then retrain the LLM.
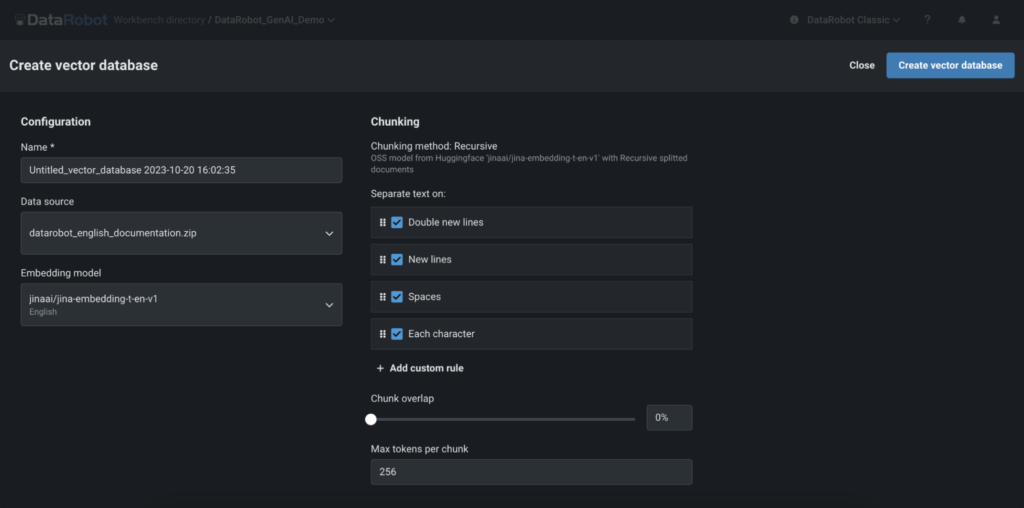
- Fine-tune or Replace Your LLM: Experiment with different configurations to fine-tune your existing LLM for optimal performance.
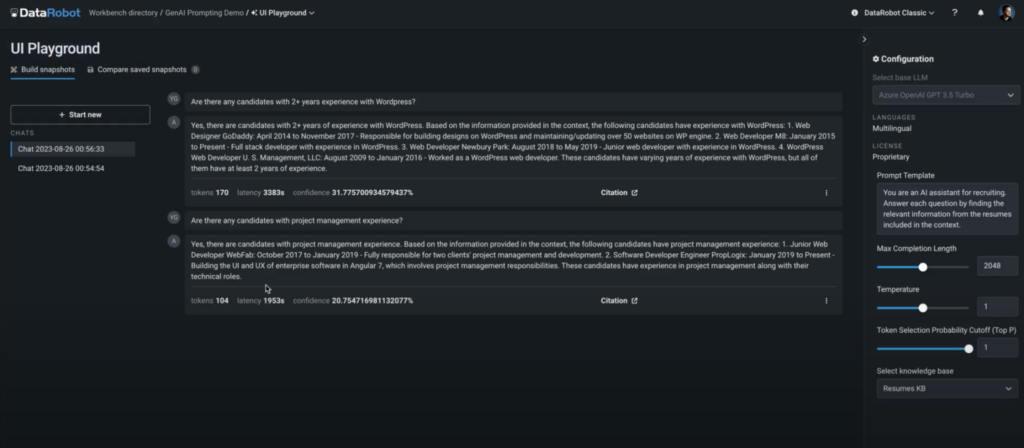
Alternatively, evaluate other LLM strategies and compare their performance to determine if a replacement is needed.
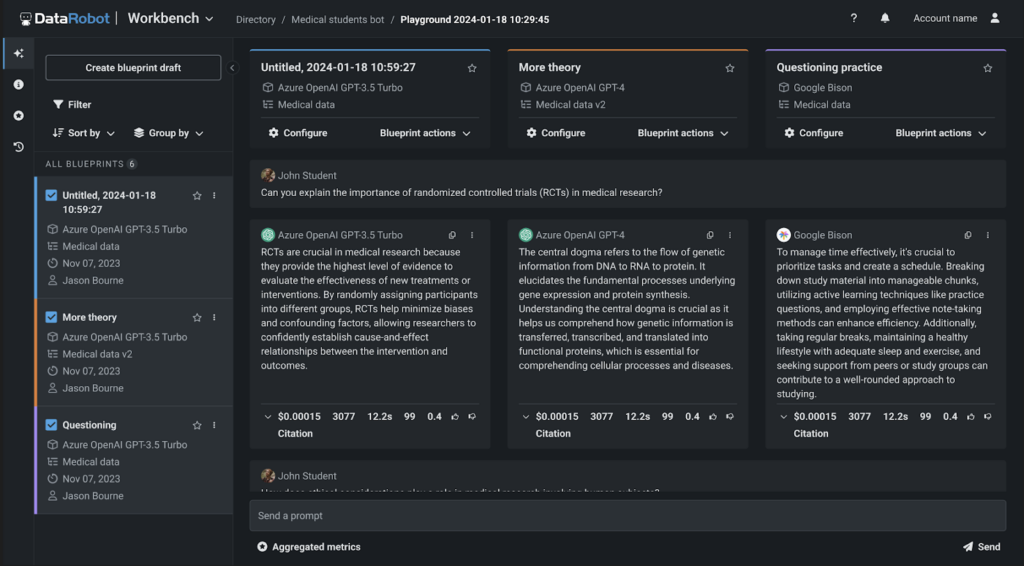
- Moderate in Real-Time or Set the Right Guard Models: Pair each generative model with a predictive AI guard model that evaluates the quality of the output and filters out inappropriate or irrelevant questions.
This framework has broad applicability across use cases where accuracy and truthfulness are paramount. DR provides a control layer that allows you to take the data from external applications, guard it with the predictive models hosted in or outside Datarobot or NeMo guardrails, and call external LLM for making predictions.
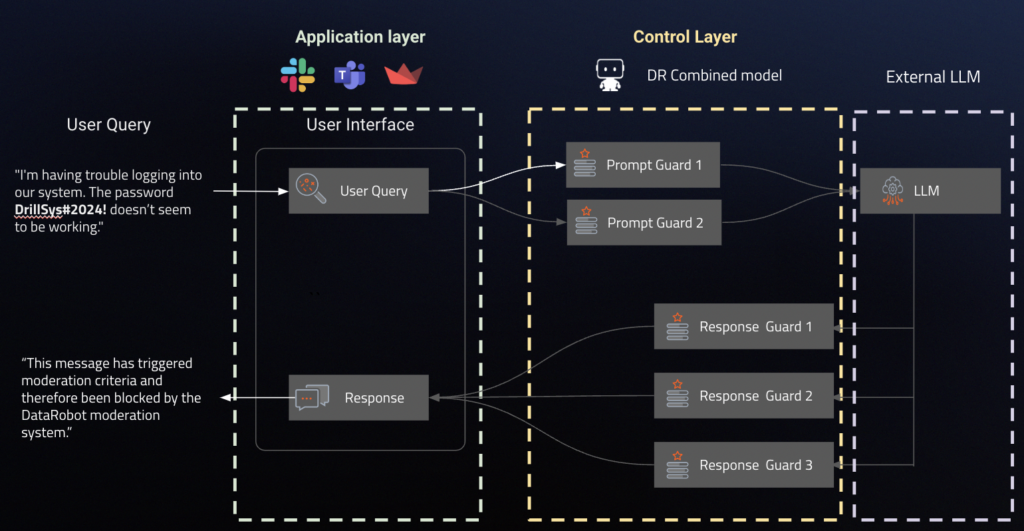
Following these steps, you can ensure a 360° view of all your AI assets in production and that your chatbots remain effective and reliable.
Summary
AI observability is essential for ensuring the effective and reliable performance of AI models across an organization’s ecosystem. By leveraging the DataRobot platform, businesses maintain comprehensive oversight and control of their AI workflows, ensuring consistency and scalability.
Implementing robust observability practices not only helps in identifying and preventing issues in real-time but also aids in continuous optimization and enhancement of AI models, ultimately creating useful and safe applications.
By utilizing the right tools and strategies, organizations can navigate the complexities of AI operations and harness the full potential of their AI infrastructure investments.
The post Why Do You Need Cross-Environment AI Observability? appeared first on DataRobot AI Platform.
Making “cheddar” with industrial automation – Achieving 83 per cent waste reduction in food manufacturing
Building Digital Trust in an AI-Powered World
In today’s digitally connected world, the business landscape is constantly evolving, and the rise in AI usage is further fueling this rapid enterprise transformation. But, at the same time, the technology is exacerbating an age-old challenge for businesses: establishing and […]
The post Building Digital Trust in an AI-Powered World appeared first on TechSpective.
Researchers teach AI to spot what you’re sketching
AI recognizes athletes’ emotions
Robo-revolution: From lab to market
New method for orchestrating successful collaboration among robots relies on patience
Generating audio for video
Top AI Tools for Research: Evaluating ChatGPT, Gemini, Claude, and Perplexity
In the fast-paced world of technology and information, staying ahead requires the right tools to streamline our efforts. This article marks the second installment in our series on AI productivity tools. Previously, we explored AI-driven solutions for scheduling and task management. Today, we shift our focus to a critical aspect of our professional and personal lives: research.
Research is a cornerstone of innovation, whether it’s for academic pursuits, business strategies, or personal projects. The landscape of research tools has been revolutionized by AI, particularly through the power of large language models (LLMs). These models enable a dynamic chatbot experience where users can ask initial questions and follow up with deeper inquiries based on the responses received.
In this article, we will delve into four leading AI tools that can be leveraged for research projects: ChatGPT, Gemini, Claude, and Perplexity. We will assess these tools based on key criteria such as the quality of their responses, their access to current information, their ability to reference original sources, their capacity to process and analyze uploaded files, and their subscription plans. We hope that this brief overview will help you choose the best tool for your various research projects.
If this in-depth educational content is useful for you, subscribe to our AI mailing list to be alerted when we release new material.
Top AI Research Tools
ChatGPT, Gemini, Claude, and Perplexity are the leading LLM-powered tools that can speed up your research for both business projects and personal tasks. Let’s briefly review their strengths and weaknesses across key factors.
ChatGPT
ChatGPT is a state-of-the-art LLM-powered tool developed by OpenAI, designed to assist with a wide range of tasks by understanding and generating human-like text.
Quality of Responses. ChatGPT, powered by the top-performing GPT-4o model, delivers well-structured and highly informative responses. Its advanced language processing capabilities ensure that the information provided is both relevant and comprehensive, making it a great tool for diverse research needs.
Current Data Access. ChatGPT is equipped with real-time web access, allowing it to pull the latest information available online. Additionally, CustomGPTs built on top of ChatGPT can tap into specific knowledge bases, offering enhanced responses tailored to particular fields of study. Notable examples include Consensus, Scholar GPT, SciSpace, Wolfram, and Scholar AI.
Source Referencing. While ChatGPT does provide links to its sources, these references are often grouped at the end of the response. This can make it challenging to trace specific statements back to their original sources, which may require additional effort to verify the information.
File Processing Capabilities. ChatGPT supports file uploads, enabling users to analyze and extract information from various documents. This feature is particularly useful for in-depth research, allowing for the incorporation of external data directly into the chat.
Subscription Plans. ChatGPT offers a Free plan that grants access to GPT-3.5 and limited features of GPT-4o, including basic data analysis, file uploads, and web browsing. For more advanced capabilities, the Plus plan is available at $20 per month. This plan provides full access to the state-of-the-art GPT-4o model, along with comprehensive data analysis, file uploads, and web browsing functionalities.
Gemini
Gemini is a cutting-edge AI tool designed by Google, leveraging powerful language models to assist with various research needs.
Quality of Responses. The application is powered by strong Gemini models. The responses are generally of high quality and effectively address the research questions posed. However, like all LLM-powered solutions, it can occasionally produce hallucinations or inaccuracies.
Current Data Access. Gemini has access to real-time information, ensuring that it provides up-to-date responses.
Source Referencing. Gemini does not provide direct links to sources within its responses. However, it includes a unique feature called the “Double-check the response” button. When used, this feature verifies the model’s statements through Google Search: confirmed statements are highlighted in green, unconfirmed or likely incorrect statements in brown, and statements with insufficient information are left unhighlighted. Additionally, links to the relevant Google Search results are provided for further verification.
File Processing Capabilities. Gemini supports file uploads, allowing users to analyze and extract information from various documents.
Subscription Plans. The basic version of Gemini is accessible for free and can handle complex requests using one of the latest models from the Gemini family, though not the most powerful. For more advanced features, users can subscribe to Gemini Advanced for $20 per month. This premium version leverages Google’s most powerful AI model, offering superior reasoning and problem-solving capabilities.
Claude
Claude is a sophisticated AI tool developed by Anthropic, designed to provide high-quality research assistance with a strong emphasis on safety and reliability. Known for its advanced language models and thoughtful design, Claude aims to deliver accurate and trustworthy responses while managing user expectations effectively.
Quality of Responses. The LLM models powering Claude are among the best in the industry, resulting in high-quality responses. Claude stands out for its focus on safety, reducing the likelihood of providing potentially harmful information. It also frequently states its limitations within its responses, such as its knowledge cutoff date and the scope of information it can access. This transparency helps manage user expectations and directs them to more accurate and up-to-date sources when necessary.
Current Data Access. Claude is designed to be a self-contained tool and does not access the web for real-time responses. Its answers are based on publicly available information up to its knowledge cutoff date, which is currently August 2023.
Source Referencing. Claude does not provide direct links to original sources in its responses. This can make it challenging for users to verify specific statements or trace information back to its origin.
File Processing Capabilities. Claude supports the upload of documents and images, allowing for more in-depth and relevant research.
Subscription plans. Claude offers a Free plan that provides access to the tool, with responses powered by the Claude 3 Sonnet model. For enhanced features, the Claude Pro plan is available at $20 per month. This plan provides access to Claude 3 Opus, the most advanced model, along with priority access during high-traffic periods.
Perplexity
Perplexity is a powerful AI research tool that utilizes advanced language models to deliver high-quality responses. It is designed to provide detailed and accurate information, with a particular emphasis on thorough source referencing and multimodal search capabilities.
Quality of Responses. Perplexity is powered by strong LLMs, including state-of-the-art models like GPT-4o, Claude-3, LLaMA 3, and others. This ensures that the quality of responses is generally very high. The tool is focused on providing accurate and detailed answers, supported by strong source referencing. However, it sometimes provides information that is not fully relevant, as it tends to include extensive details found online, which may not always directly answer the research question posed.
Current Data Access. Perplexity has real-time access to the web, ensuring that its responses are always up to date. This capability allows users to receive information on current events and the latest developments as they happen.
Source Referencing. One of Perplexity’s major strengths is its source referencing. Each response includes citations, making it easy to trace every statement back to its original source. Additionally, Perplexity’s search is multimodal, incorporating images, videos, graphs, charts, and visual cues found online, enhancing the comprehensiveness of the information provided.
File Processing Capabilities. The ability to upload and analyze files is available but limited in the free version of the tool, and unlimited with the Pro plan.
Subscription plans. Perplexity offers a Standard plan for free, which allows for unlimited quick searches and five Pro (more in-depth) searches per day. For more extensive use, the Pro plan costs $20 per month and allows up to 600 Pro searches per day. This plan provides enhanced capabilities for users with more demanding research needs.
Conclusion: Choosing the Right AI Research Tool for Your Needs
Each of the tools we reviewed – ChatGPT, Gemini, Claude, and Perplexity – offers unique strengths tailored to different research requirements.
ChatGPT excels in delivering well-structured and informative responses with robust file processing capabilities. Gemini stands out with its unique verification feature, though it lacks direct source referencing. Claude prioritizes safety and transparency, making it a reliable choice for users concerned about the accuracy and potential risks of AI-generated information. Perplexity offers unparalleled source referencing and multimodal search capabilities, ensuring detailed and visually enriched responses, though its relevancy can sometimes be hit-or-miss.
When choosing an AI research tool, consider the specific needs of your projects. By understanding the strengths and limitations of each tool, you can make an informed decision that enhances your research capabilities and supports your goals effectively.
Enjoy this article? Sign up for more AI research updates.
We’ll let you know when we release more summary articles like this one.
The post Top AI Tools for Research: Evaluating ChatGPT, Gemini, Claude, and Perplexity appeared first on TOPBOTS.