Sensing Parkinson’s symptoms
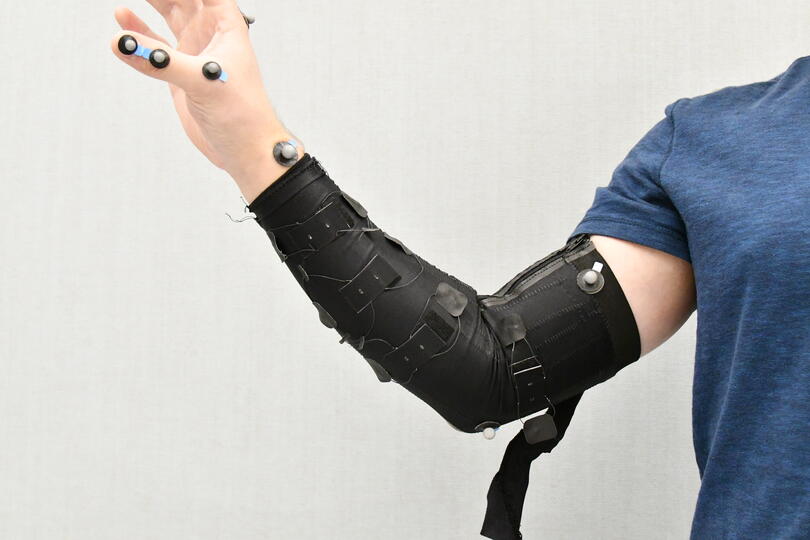
MyoExo integrates a series of sensors into a wearable device capable of detecting slight changes in muscle strain and bulging, enabling it to measure and track the symptoms of Parkinson’s disease. Credit: Oluwaseun Araromi
By Matthew Goisman/SEAS Communications
Nearly one million people in the United States live with Parkinson’s disease. The degenerative condition affects the neurons in the brain that produce the neurotransmitter dopamine, which can impact motor function in multiple ways, including muscle tremors, limb rigidity and difficulty walking.
There is currently no cure for Parkinson’s disease, and current treatments are limited by a lack of quantitative data about the progress of the disease.
MyoExo, a translation-focused research project based on technology developed at the Harvard John A. Paulson School of Engineering (SEAS) and the Wyss Institute for Biologically Inspired Engineering, aims to provide that data. The team is refining the technology and starting to develop a business plan as part of the Harvard Innovation Lab’s venture program. The MyoExo wearable device aims to not only provide a remote monitoring device for patients at-home setting but also be sensitive enough to aid early diagnostics of Parkinson’s disease.
“This is a disease that’s affecting a lot of people and it seems like the main therapeutics that tackle this have not changed significantly in the past several decades,” said Oluwaseun Araromi, Research Associate in Materials Science and Mechanical Engineering at SEAS and the Wyss Institute.
The MyoExo technology consists of a series of wearable sensors, each one capable of detecting slight changes in muscle strain and bulging. When integrated into a wearable device, the data can provide what Araromi described as “muscle-centric physiological signatures.”
“The enabling technology underlying this is a sensor that detects small changes in the shape of an object,” he said. “Parkinson’s disease, especially in its later stages, really expresses itself as a movement disorder, so sensors that can detect shape changes can also detect changes in the shape of muscle as people move.”
MyoExo emerged from research done in the Harvard Biodesign Lab of Conor Walsh, the Paul A. Maeder Professor of Engineering and Applied Sciences, and the Microrobotics Lab of Rob Wood, the Charles River Professor of Engineering and Applied Sciences at SEAS. Araromi, Walsh and Wood co-authored a paper on their research into resilient wearable sensors in November 2020, around the same time the team began to focus on medical applications of the technology.
“If we had these hypersensitive sensors in something that a person was wearing, we could detect how their muscles were bulging,” Walsh said. “That was more application-agnostic. We didn’t know exactly where that would be the most important, and I credit Seun and our Wyss collaborators for being the ones to think about identifying Parkinson’s applications.”
Araromi sees the MyoExo technology as having value for three major stakeholders: the pharmaceutical industry, clinicians and physicians, and patients. Pharmaceutical companies could use data from the wearable system to quantify their medications’ effect on Parkinson’s symptoms, while clinicians could determine if one treatment regimen is more effective than another for a specific patient. Patients could use the system to track their own treatment, whether that’s medication, physical therapy, or both.
“Some patients are very incentivized to track their progress,” Araromi said. “They want to know that if they were really good last week and did all of the exercises that they were prescribed, their wearable device would tell them their symptomatology has reduced by 5% compared to the week before. We envision that as something that would really encourage individuals to keep and adhere to their treatment regiments.”
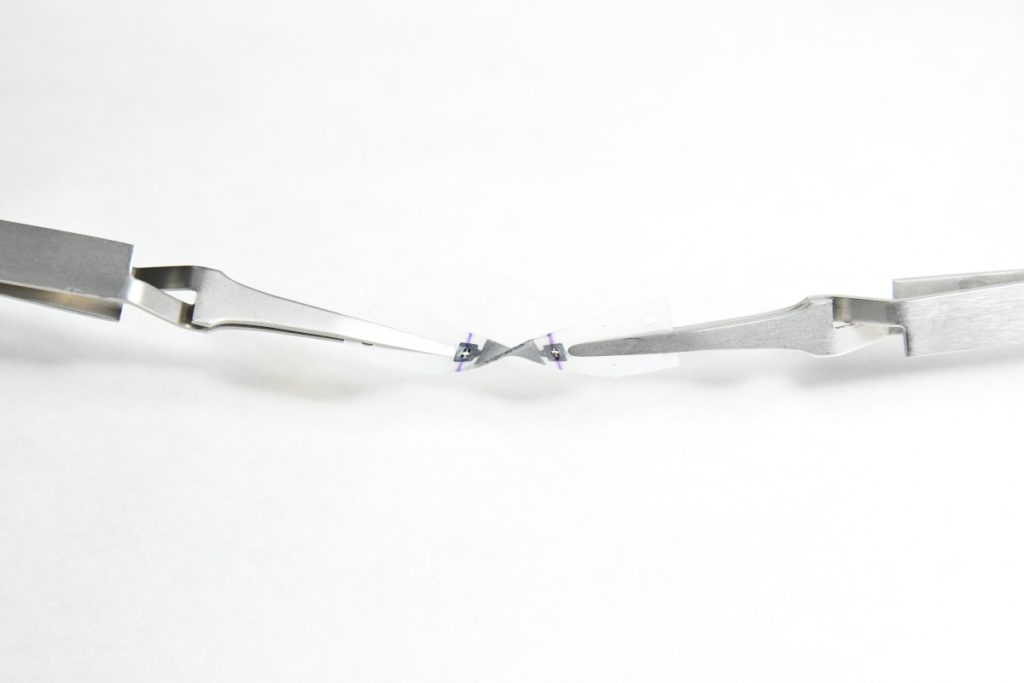
MyoExo’s sensor technology is based on research conducted in the Harvard Biodesign Lab of Conor Walsh and the Microrobotics Lab of Rob Wood at SEAS, and further developed through the Wyss Institute for Biologically Inspired Engineering and Harvard Innovation Labs venture program. Credit: Oluwaseun Araromi
Araromi joined SEAS and the Wyss Institute as a postdoctoral researcher in 2016, having earned a Ph.D in mechanical engineering from the University of Bristol in England and completed a postdoc at the Swiss Federal Institute of Technology Lausanne.
His interest in sensor technology made him a great fit for research spanning the Biodesign and Microrobotics labs, and his early work included helping develop an exosuit to aid with walking.
“I was initially impressed with Seun’s strong background in materials, transduction and physics,” Walsh said. “He really understood how you’d think about creating novel sensors with soft materials. Seun’s really the translation champion for the project in terms of driving forward the technology, but at the same time trying to think about the need in the market, and how we demonstrate that we can meet that.”
The technology is currently in the human testing phase to demonstrate proof of concept detection of clinically-relevant metrics with support from the Wyss Institute Validation Project program. Araromi wants to show that the wearable device can quantify the difference between the muscle movements of someone with Parkinson’s and someone without. From there, the goal is to demonstrate that the device can quantify whether a person has early- or late-stage symptoms of the disease, as well as their response to treatment.
“We are evaluating our technology and validating our technical approach, making sure that as it’s currently constructed, even in this crude form, we can get consistent data and results,” Araromi said. “We’re doing this in a small pilot phase, such that if there are issues, we can fix those issues, and then expand to a larger population where we would test our device on more individuals with Parkinson’s disease. That should really convince ourselves and hopefully the community that we are able to reach a few key technical milestones, and then garner more interest and potentially investment and partnership.”
Interactive Perception at Toyota Research Institute
Dr. Carolyn Matl, Research Scientist at Toyota Research Institute, explains why Interactive Perception and soft tactile sensors are critical for manipulating challenging objects such as liquids, grains, and dough. She also dives into “StRETcH” a Soft to Resistive Elastic Tactile Hand, a variable stiffness soft tactile end-effector, presented by her research group.
Carolyn Matl
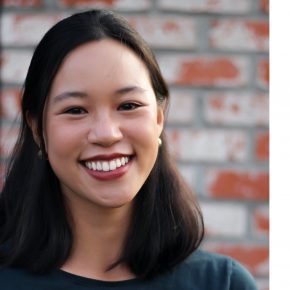
Carolyn Matl is a research scientist at the Toyota Research Institute, where she works on robotic perception and manipulation with the Mobile Manipulation Team. She received her B.S.E in Electrical Engineering from Princeton University in 2016, and her Ph.D. in Electrical Engineering and Computer Sciences at the University of California, Berkeley in 2021. At Berkeley, she was awarded the NSF Graduate Research Fellowship and was advised by Ruzena Bajcsy. Her dissertation work focused on developing and leveraging non-traditional sensors for robotic manipulation of complicated objects and substances like liquids and doughs.
Carolyn Matl’s Related Research Videos
Links
- StRETcH: a Soft to Resistive Elastic Tactile Hand
- Carolyn Matl’s personal website
- Download mp3 (48.7 MB)
- Subscribe to Robohub using iTunes, RSS, or Spotify
- Support us on Patreon
Engineers Meet Industry Demand By Growing Their Robotics & IAS Expertise
Molecular robots that work cooperatively in swarms
High Performance Inertial Sensors for Robotic Systems
New method allows robot vision to identify occluded objects
#ICRA2022 Science Communication Awards
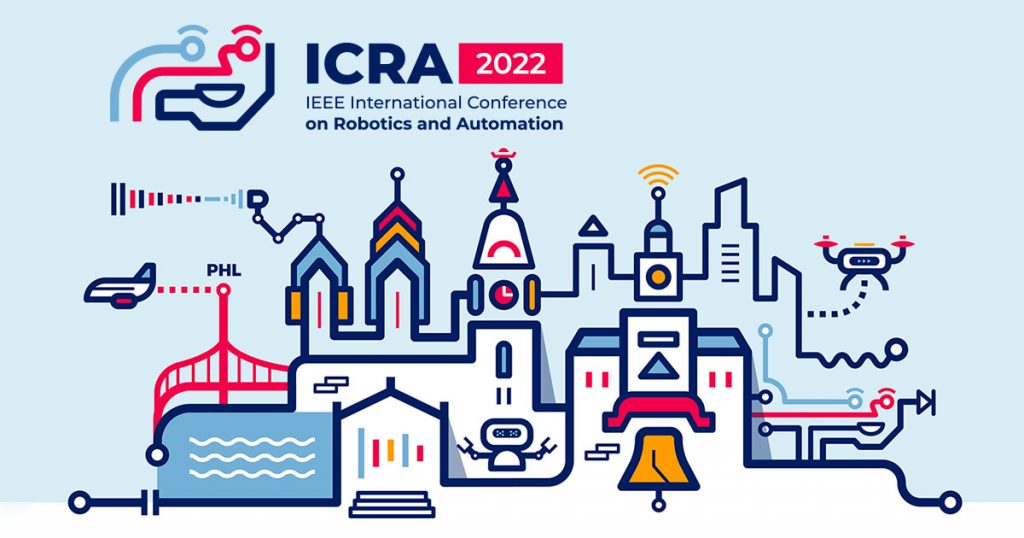
Number of awards: 2
Amount: travel and accommodation (up to 2k) + free registration
Application deadline: 22 April 2022
We are offering up to 2 Science Communication Awards to motivated roboticists keen on helping us cover ICRA. Your coverage could include videos, interviews, podcasts, blogs, social media, or art. Please apply by sending the following information by April 22 to sabine.hauert@bristol.ac.uk with the subject [ICRA SciCommAward]:
- Name
- Who you are (one paragraph)
- Previous experience in science communication (one paragraph with links to material)
- What you’d like to cover at ICRA and where you will publish material produced (one paragraph)
- Agreement to cross-post your content to the ICRA website or other third-party channels (yes/no).
- Estimated travel budget
Awards will be announced on 25 April.
Smart, Compact Electromechanical Actuators Improve Productivity and Space Efficiency for Industrial AGVs
Combining turgor design and electro-osmosis to create strong and fast hydrogel actuators
ABB Robotics showcases future of retail using recovered marine plastic at London’s Selfridges
A flexible way to grab items with feeling
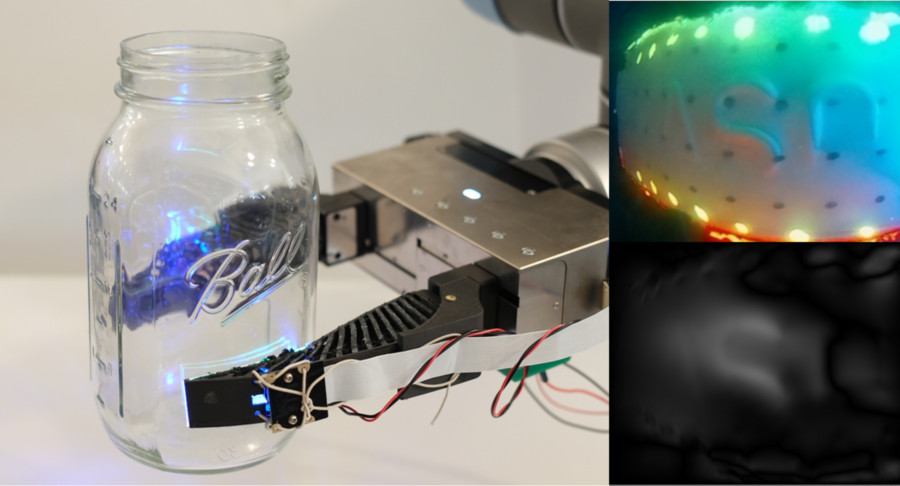
The GelSight Fin Ray gripper holds a glass Mason jar with its tactile sensing. Photo courtesy of MIT CSAIL.
By Rachel Gordon | MIT CSAIL
The notion of a large metallic robot that speaks in monotone and moves in lumbering, deliberate steps is somewhat hard to shake. But practitioners in the field of soft robotics have an entirely different image in mind — autonomous devices composed of compliant parts that are gentle to the touch, more closely resembling human fingers than R2-D2 or Robby the Robot.
That model is now being pursued by Professor Edward Adelson and his Perceptual Science Group at MIT’s Computer Science and Artificial Intelligence Laboratory (CSAIL). In a recent project, Adelson and Sandra Liu — a mechanical engineering PhD student at CSAIL — have developed a robotic gripper using novel “GelSight Fin Ray” fingers that, like the human hand, is supple enough to manipulate objects. What sets this work apart from other efforts in the field is that Liu and Adelson have endowed their gripper with touch sensors that can meet or exceed the sensitivity of human skin.
Their work was presented last week at the 2022 IEEE 5th International Conference on Soft Robotics.
The fin ray has become a popular item in soft robotics owing to a discovery made in 1997 by the German biologist Leif Kniese. He noticed that when he pushed against a fish’s tail with his finger, the ray would bend toward the applied force, almost embracing his finger, rather than tilting away. The design has become popular, but it lacks tactile sensitivity. “It’s versatile because it can passively adapt to different shapes and therefore grasp a variety of objects,” Liu explains. “But in order to go beyond what others in the field had already done, we set out to incorporate a rich tactile sensor into our gripper.”
The gripper consists of two flexible fin ray fingers that conform to the shape of the object they come in contact with. The fingers themselves are assembled from flexible plastic materials made on a 3D printer, which is pretty standard in the field. However, the fingers typically used in soft robotic grippers have supportive cross-struts running through the length of their interiors, whereas Liu and Adelson hollowed out the interior region so they could create room for their camera and other sensory components.
The camera is mounted to a semirigid backing on one end of the hollowed-out cavity, which is, itself, illuminated by LEDs. The camera faces a layer of “sensory” pads composed of silicone gel (known as “GelSight”) that is glued to a thin layer of acrylic material. The acrylic sheet, in turn, is attached to the plastic finger piece at the opposite end of the inner cavity. Upon touching an object, the finger will seamlessly fold around it, melding to the object’s contours. By determining exactly how the silicone and acrylic sheets are deformed during this interaction, the camera — along with accompanying computational algorithms — can assess the general shape of the object, its surface roughness, its orientation in space, and the force being applied by (and imparted to) each finger.
Liu and Adelson tested out their gripper in an experiment during which just one of the two fingers was “sensorized.” Their device successfully handled such items as a mini-screwdriver, a plastic strawberry, an acrylic paint tube, a Ball Mason jar, and a wine glass. While the gripper was holding the fake strawberry, for instance, the internal sensor was able to detect the “seeds” on its surface. The fingers grabbed the paint tube without squeezing so hard as to breach the container and spill its contents.
The GelSight sensor could even make out the lettering on the Mason jar, and did so in a rather clever way. The overall shape of the jar was ascertained first by seeing how the acrylic sheet was bent when wrapped around it. That pattern was then subtracted, by a computer algorithm, from the deformation of the silicone pad, and what was left was the more subtle deformation due just to the letters.
Glass objects are challenging for vision-based robots because of the refraction of the light. Tactile sensors are immune to such optical ambiguity. When the gripper picked up the wine glass, it could feel the orientation of the stem and could make sure the glass was pointing straight up before it was slowly lowered. When the base touched the tabletop, the gel pad sensed the contact. Proper placement occurred in seven out of 10 trials and, thankfully, no glass was harmed during the filming of this experiment.
Wenzhen Yuan, an assistant professor in the Robotics Institute at Carnegie Mellon University who was not invovled with the research, says, “Sensing with soft robots has been a big challenge, because it is difficult to set up sensors — which are traditionally rigid — on soft bodies,” Yuan says. “This paper provides a neat solution to that problem. The authors used a very smart design to make their vision-based sensor work for the compliant gripper, in this way generating very good results when robots grasp objects or interact with the external environment. The technology has lots of potential to be widely used for robotic grippers in real-world environments.”
Liu and Adelson can foresee many possible applications for the GelSight Fin Ray, but they are first contemplating some improvements. By hollowing out the finger to clear space for their sensory system, they introduced a structural instability, a tendency to twist, that they believe can be counteracted through better design. They want to make GelSight sensors that are compatible with soft robots devised by other research teams. And they also plan to develop a three-fingered gripper that could be useful in such tasks as picking up pieces of fruit and evaluating their ripeness.
Tactile sensing, in their approach, is based on inexpensive components: a camera, some gel, and some LEDs. Liu hopes that with a technology like GelSight, “it may be possible to come up with sensors that are both practical and affordable.” That, at least, is one goal that she and others in the lab are striving toward.
The Toyota Research Institute and the U.S. Office of Naval Research provided funds to support this work.
Interview with Andrea Thomaz (co-founder of Diligent Robotics): socially intelligent automation solutions for hospitals
By Sonia Roberts, with additional editing by Dharini Dutia
Diligent Robotics, founded by Andrea Thomaz and Vivian Chu, develops socially intelligent automation solutions for hospitals. Moxi, their flagship robot, delivers items like medications and wound dressings between departments to save the clinical staff’s time. Diligent has just closed their Series B funding round with $30 million.
We sat down with Dr. Thomaz to talk about Moxi, how to manage people’s expectations about robots, and advice for young people and women in robotics. This interview has been lightly edited for clarity.
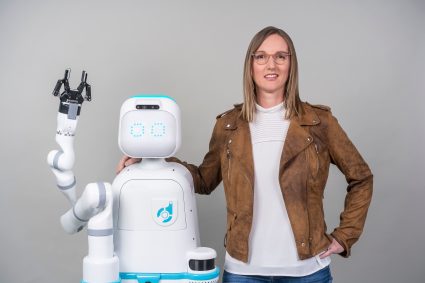
Andrea Thomaz with Diligent’s flagship robot Moxi.
What kinds of problems are you trying to solve with Moxi?
We are building Moxi to help hospitals with the massive workforce shortage that they’re seeing now more than ever. We actually started the company with the same intention several years ago before there was a worldwide pandemic, and it really has just gotten to be an even bigger problem for hospitals. I feel really strongly that robots have a place to play in teamwork environments, and hospitals are a great example of that. There’s no one person’s job in a hospital that you would actually want to give over to automation or robots, but there are tiny little bits of a lot of people’s jobs that are absolutely able to be automated and that we can give over to delivery robots in particular like Moxi. [The main problem we’re trying to solve with Moxi is] point to point delivery, where we’re fetching and gathering things and taking them from one area of the hospital to another.
Hospitals have a lot of stuff that’s moving around every day. Every person in the hospital is going to have certain medications that need to be delivered to them, certain lab samples that need to be taken and delivered to the central lab, certain supplies that need to come up to them, food and nutrition every day. You have a lot of stuff that’s coming and going between patient units and all these different support departments.
Every one of these support departments has a process in place for getting the stuff moved around, but no matter what, there’s stuff that happens every single day that requires ad-hoc [deliveries] to happen between all of these departments and different nursing units. So sometimes that’s going to be a nurse that just needs to get something for their patient and they want that to happen as soon as possible. They’re trying to discharge their patient, they need a particular wound dressing kit, they’re going to run down and get it because they want to help their patient get out. Or if there’s something that needs to be hand carried because the regular rounding of medications has already happened, a lot of times you’ll have a pharmacy technician stop what they’re doing and go and run some infusion meds for a cancer patient, for example. It sort of falls between these departments. There’s different people that would be involved but a lot of times it does fall on the nursing units themselves. A nurse explained to us one time that nurses are the last line of defense in patient care.
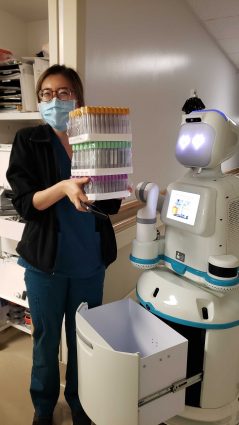
Moxi performing a delivery for a clinical staff member.
What is changing with this most recent round of funding?
Over the last 6-12 months, the demand has really skyrocketed such that we’re barely keeping up with the demand for people wanting to implement robots in their hospitals. That’s the reason why we’re raising this round of funding, expanding the team, and expanding our ability to capitalize on that demand. A couple of years ago, if we were working with a hospital it was because they had some special funds set aside for innovation or they had a CTO or a CIO that had a background in robotics, but it certainly wasn’t the first thing that every hospital CIO was thinking about. Now that has completely changed. We’re getting cold outreach on our website from CIOs of hospitals saying “I need to develop a robotic strategy for our hospital and I want to learn about your solution.” Through the pandemic, I think everyone has seen that the workforce shortage in hospitals is only getting worse in the near term. Everybody wants to plan for the future and do everything they can to take small tasks off of the plates of their clinical teams. It’s been really exciting to be part of that market change and see that shift to where everybody is really really open to automation. Before we had to say “No no no, this is not the future, I promise it’s not scifi, I promise these really work.” Now [the climate has] really shifted to people understanding “This is actually something that can impact my teams.”
[Two of our investors are hospitals, and] that’s been one of our most exciting parts of this round. It’s always great to have a successful funding round, but to have strategic partners like Cedars-Sinai and Shannon Healthcare coming in and saying “Yeah, we actually want to build this alongside you” — it’s pretty exciting to have customers like that.
What kinds of technical problems did you run into when you were either building Moxi or deploying it in a hospital environment? How did you solve those problems?
One that was almost surprising in how often it came up, and really impacted our ability [to run Moxi in the hospital environment] because we have a software-based robotic solution that is connecting at a regular basis to cloud services, [was that] we had no clue how terrible hospital WiFi was going to be. We actually spent quite a while building in backup systems to be able to use WiFi, backup to LTE if we have to, but be smart about that so we’re not spending a whole bunch of money on LTE data. That was a problem that seemed very specific to hospitals in particular.
Another one was security and compliance. We just didn’t know what some of the different requirements were for hospitals until we actually got into the environments and started interacting with customers and understanding what they wanted to use Moxi for. When we were first doing research trials in 2018 or 2019, we had a version of the robot that was a little bit different than the one we have today. It had lots of open containers so you could just put whatever you wanted to on the robot and send it over to another location. We quickly learned that that limited what the robot was allowed to carry, because so much of what [the customers] wanted was to understand who pulled something out of the robot. So now we have an RF badge reader on the robot that is connected to locking storage containers that are only going to open if you’re the kind of person that is allowed to open the robot. That was an interesting technical challenge that we didn’t know about until after we got out there.
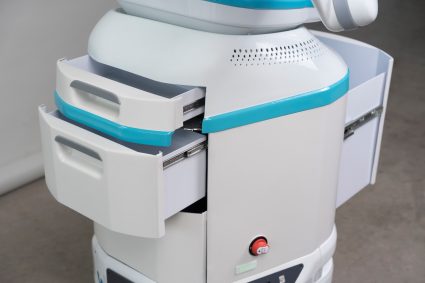
Moxi’s locking storage containers.
How did you work with nurses and the other healthcare professionals you were working with to figure out what would be the most helpful robot for them?
My background, and my co-founder Vivian Chu’s background, is in human-robot interaction so we knew that we didn’t know enough about nursing or the hospital environment. We spent the first 9 months of the company in 2018 building out our research prototype. It looked a lot like what Moxi looks like today. Under the hood it was completely different than what we have today in terms of the reliability and robustness of the hardware and software, but it was enough to get that platform out and have it deployed with nursing units. We embedded ourselves with four different nursing units across Texas over a year-long period. We would spend about 6-8 weeks with a nursing department, and we were just there — engineers, product people, and everybody in the company was cycling in and out a week or two at a time.
We would ask those nurses: “What would you actually want a robot like this to do?” Part of this that was really important was they didn’t have good ideas about what they would want the robot to do until they saw the robot. It was a very participatory design, where they had to see and get a sense for the robot before they would have good ideas of what they would want the robot to do. Then we would take those ideas [to the company] and come back and say “Yes we can do that,” or “No we can’t do that.” We came out of that whole process with a really great idea. We like to say that’s where we found our product market fit — that’s where we really understood that what was going to be most valuable for the robot to do was connecting the nursing units to these other departments. We can help a nurse with supply management and getting things from place to place within their department, or we can help them with things that are coming from really far away. [The second one] was actually impacting their time way way more.
Because the capabilities of robotic systems are usually misinterpreted, it can be really hard to manage the relationship with stakeholders and customers and set appropriate expectations. How did you manage that relationship?
We do a lot of demonstrations, but still with almost every single implementation you get questions about some robot in Hollywood, [and you have to say] “No, that’s the movies” and explain exactly what Moxi does.
From a design perspective, we also limit the English phrases that come out of Moxi’s mouth just because we don’t want to communicate a really high level of intelligence. There are lots of canned phrases and interactions on the iPad instead of via voice, and a lot of times the robot will just make meeps and beeps and flash lights and things like that.
Before starting the company, I had a lab, and one of the big research topics that we had for a number of years was embodied dialogue — how robots could have a real conversation with people. I had a very good appreciation for how hard that problem is, and also for just how much people want it. People come up to a robot, and they want it to be able to talk to them. How you can [set expectations] with the design and behavior of the robot has been a focus of mine since before we started the company. We purposefully don’t make the robot look very human-like because we don’t want there to be android human-level expectations, but [the robot does have a face and eyes so it can] communicate “I’m looking at that thing” and “I’m about to manipulate that thing,” which we think is important. It’s really about striking that balance.
What would you say is one lesson that you’ve learned from your work at Diligent so far and how are you looking to apply this lesson moving forward?
The difference between research and practice. On the one hand, the motivation and reason for starting a company is that you want to see the kinds of things that you’ve done in the research lab really make it out into the world and start to impact real people and their work. That’s been one of the most fascinating, impactful, and inspiring things about starting Diligent: Being able to go and see nurses when Moxi is doing work for them. They are so thankful! If you just hang back and watch Moxi come and do a delivery, almost always people are super excited to see the robot. They get their delivery and they’re like, “Oh, thank you Moxi!” That feels like we’re really making a difference in a way that you just don’t get with just research contributions that don’t make it all the way out into the world.
That being said though, there is a long tail of things that you have to solve from an engineering perspective beyond [developing a feature]. My VP of engineering Starr Corbin has this great way of putting it: The research team will get a certain thing on the product to be feature complete, where we’ve demonstrated that this feature works and it’s a good solution, but then there’s this whole phase that has to happen after that to get the feature to be production ready. I would say my biggest lesson is probably everything that it takes, and the entire team of people it takes, to get something from being feature complete to production ready. I have a deep appreciation for that. How fast we can move things out into the world is really dictated by some of that.
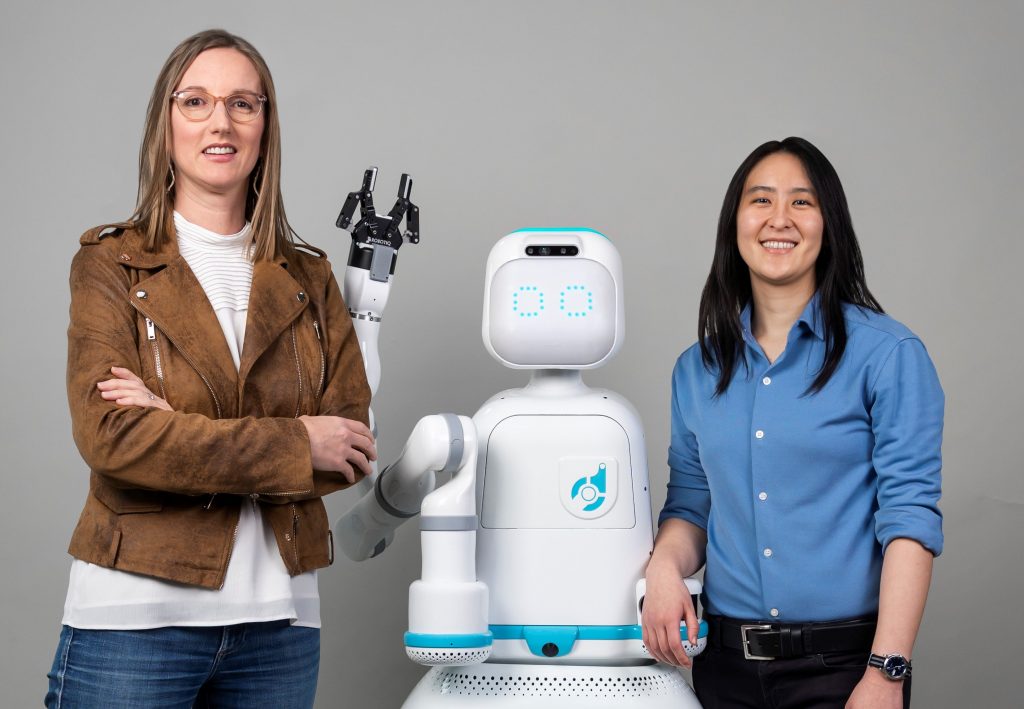
Andrea Thomaz (left) and Vivian Chu with Moxi.
What advice would you give young women in robotics?
If I put my professor hat on, I always had advice that I liked to give women in robotics, in academia, and just kind of pursuing things in general. Imposter syndrome is real, and everybody feels it. All you can do to combat it is not underestimate yourself. Speak up and know that you deserve a seat at the table. It’s all about hard work, but also making sure that your voice is heard. Some of the mentorship that I gave to a lot of my women grad students when I was a professor was around speaking engagements, speaking styles, and communication. It can be really uncomfortable when you’re the only anything in the room to stand up and feel like you deserve to be the one speaking, and so the more that you practice doing that, the more comfortable it can feel, the more confident you’ll feel in yourself and your voice. I think finding that confident voice is a really important skill that you have to develop early on in your career.
What’s one piece of advice you’ve received that you always turn to when things are tough?
There are two mentors that I’ve had who are women in AI and robotics. [In my] first year as a faculty member [the first mentor] came and gave a research seminar talk. I for some reason got to take her out to lunch by myself, so we had this amazing one-on-one. We talked a little bit about her talk, probably half of the lunch we talked about technical things, and then she just kind of turned the conversation [around] and said “Andrea, don’t forget to have a family.” Like, don’t forget to focus on that part of your life — it’s the most important thing. She got on a soapbox and said “You have to have a work life balance it’s so important. Don’t forget to focus on building a family for yourself, whatever that looks like.” That really stuck with me, especially as [when you’re] early in your career you’re worried about nothing but success. It was really powerful to have somebody strong and influential like that telling you “No, no, this is important and you need to focus on this.”
The other person that’s always been an inspiration and mentor for me that I’ll highlight [was the professor teaching a class I TA’d for at MIT]. I had found a bug in one of her homework problems, and she was like, “Oh, fascinating.” She was so excited that I had found a question that she didn’t know the answer to. She [just said], “Oh my gosh I don’t know, let’s go find out!” I remember her being this great professor at MIT, and she was excited to find something that she didn’t know and go and learn about it together as opposed to being embarrassed that she didn’t know something. I learned a lot from that interaction: That it’s fun to not know something because then you get to go and find the answer, and no matter who you are, you’re never expected to know everything.