The Hero of Notre-Dame
Showing robots how to do your chores
Image: Christine Daniloff, MIT
By Rob Matheson
Training interactive robots may one day be an easy job for everyone, even those without programming expertise. Roboticists are developing automated robots that can learn new tasks solely by observing humans. At home, you might someday show a domestic robot how to do routine chores. In the workplace, you could train robots like new employees, showing them how to perform many duties.
Making progress on that vision, MIT researchers have designed a system that lets these types of robots learn complicated tasks that would otherwise stymie them with too many confusing rules. One such task is setting a dinner table under certain conditions.
At its core, the researchers’ “Planning with Uncertain Specifications” (PUnS) system gives robots the humanlike planning ability to simultaneously weigh many ambiguous — and potentially contradictory — requirements to reach an end goal. In doing so, the system always chooses the most likely action to take, based on a “belief” about some probable specifications for the task it is supposed to perform.
In their work, the researchers compiled a dataset with information about how eight objects — a mug, glass, spoon, fork, knife, dinner plate, small plate, and bowl — could be placed on a table in various configurations. A robotic arm first observed randomly selected human demonstrations of setting the table with the objects. Then, the researchers tasked the arm with automatically setting a table in a specific configuration, in real-world experiments and in simulation, based on what it had seen.
To succeed, the robot had to weigh many possible placement orderings, even when items were purposely removed, stacked, or hidden. Normally, all of that would confuse robots too much. But the researchers’ robot made no mistakes over several real-world experiments, and only a handful of mistakes over tens of thousands of simulated test runs.
“The vision is to put programming in the hands of domain experts, who can program robots through intuitive ways, rather than describing orders to an engineer to add to their code,” says first author Ankit Shah, a graduate student in the Department of Aeronautics and Astronautics (AeroAstro) and the Interactive Robotics Group, who emphasizes that their work is just one step in fulfilling that vision. “That way, robots won’t have to perform preprogrammed tasks anymore. Factory workers can teach a robot to do multiple complex assembly tasks. Domestic robots can learn how to stack cabinets, load the dishwasher, or set the table from people at home.”
Joining Shah on the paper are AeroAstro and Interactive Robotics Group graduate student Shen Li and Interactive Robotics Group leader Julie Shah, an associate professor in AeroAstro and the Computer Science and Artificial Intelligence Laboratory.
Bots hedging bets
Robots are fine planners in tasks with clear “specifications,” which help describe the task the robot needs to fulfill, considering its actions, environment, and end goal. Learning to set a table by observing demonstrations, is full of uncertain specifications. Items must be placed in certain spots, depending on the menu and where guests are seated, and in certain orders, depending on an item’s immediate availability or social conventions. Present approaches to planning are not capable of dealing with such uncertain specifications.
A popular approach to planning is “reinforcement learning,” a trial-and-error machine-learning technique that rewards and penalizes them for actions as they work to complete a task. But for tasks with uncertain specifications, it’s difficult to define clear rewards and penalties. In short, robots never fully learn right from wrong.
The researchers’ system, called PUnS (for Planning with Uncertain Specifications), enables a robot to hold a “belief” over a range of possible specifications. The belief itself can then be used to dish out rewards and penalties. “The robot is essentially hedging its bets in terms of what’s intended in a task, and takes actions that satisfy its belief, instead of us giving it a clear specification,” Ankit Shah says.
The system is built on “linear temporal logic” (LTL), an expressive language that enables robotic reasoning about current and future outcomes. The researchers defined templates in LTL that model various time-based conditions, such as what must happen now, must eventually happen, and must happen until something else occurs. The robot’s observations of 30 human demonstrations for setting the table yielded a probability distribution over 25 different LTL formulas. Each formula encoded a slightly different preference — or specification — for setting the table. That probability distribution becomes its belief.
“Each formula encodes something different, but when the robot considers various combinations of all the templates, and tries to satisfy everything together, it ends up doing the right thing eventually,” Ankit Shah says.
Following criteria
The researchers also developed several criteria that guide the robot toward satisfying the entire belief over those candidate formulas. One, for instance, satisfies the most likely formula, which discards everything else apart from the template with the highest probability. Others satisfy the largest number of unique formulas, without considering their overall probability, or they satisfy several formulas that represent highest total probability. Another simply minimizes error, so the system ignores formulas with high probability of failure.
Designers can choose any one of the four criteria to preset before training and testing. Each has its own tradeoff between flexibility and risk aversion. The choice of criteria depends entirely on the task. In safety critical situations, for instance, a designer may choose to limit possibility of failure. But where consequences of failure are not as severe, designers can choose to give robots greater flexibility to try different approaches.
With the criteria in place, the researchers developed an algorithm to convert the robot’s belief — the probability distribution pointing to the desired formula — into an equivalent reinforcement learning problem. This model will ping the robot with a reward or penalty for an action it takes, based on the specification it’s decided to follow.
In simulations asking the robot to set the table in different configurations, it only made six mistakes out of 20,000 tries. In real-world demonstrations, it showed behavior similar to how a human would perform the task. If an item wasn’t initially visible, for instance, the robot would finish setting the rest of the table without the item. Then, when the fork was revealed, it would set the fork in the proper place. “That’s where flexibility is very important,” Ankit Shah says. “Otherwise it would get stuck when it expects to place a fork and not finish the rest of table setup.”
Next, the researchers hope to modify the system to help robots change their behavior based on verbal instructions, corrections, or a user’s assessment of the robot’s performance. “Say a person demonstrates to a robot how to set a table at only one spot. The person may say, ‘do the same thing for all other spots,’ or, ‘place the knife before the fork here instead,’” Ankit Shah says. “We want to develop methods for the system to naturally adapt to handle those verbal commands, without needing additional demonstrations.”
The Tentacle Bot
By Leah Burrows
Of all the cool things about octopuses (and there are a lot), their arms may rank among the coolest.
Two-thirds of an octopus’s neurons are in its arms, meaning each arm literally has a mind of its own. Octopus arms can untie knots, open childproof bottles, and wrap around prey of any shape or size. The hundreds of suckers that cover their arms can form strong seals even on rough surfaces underwater.
Imagine if a robot could do all that.
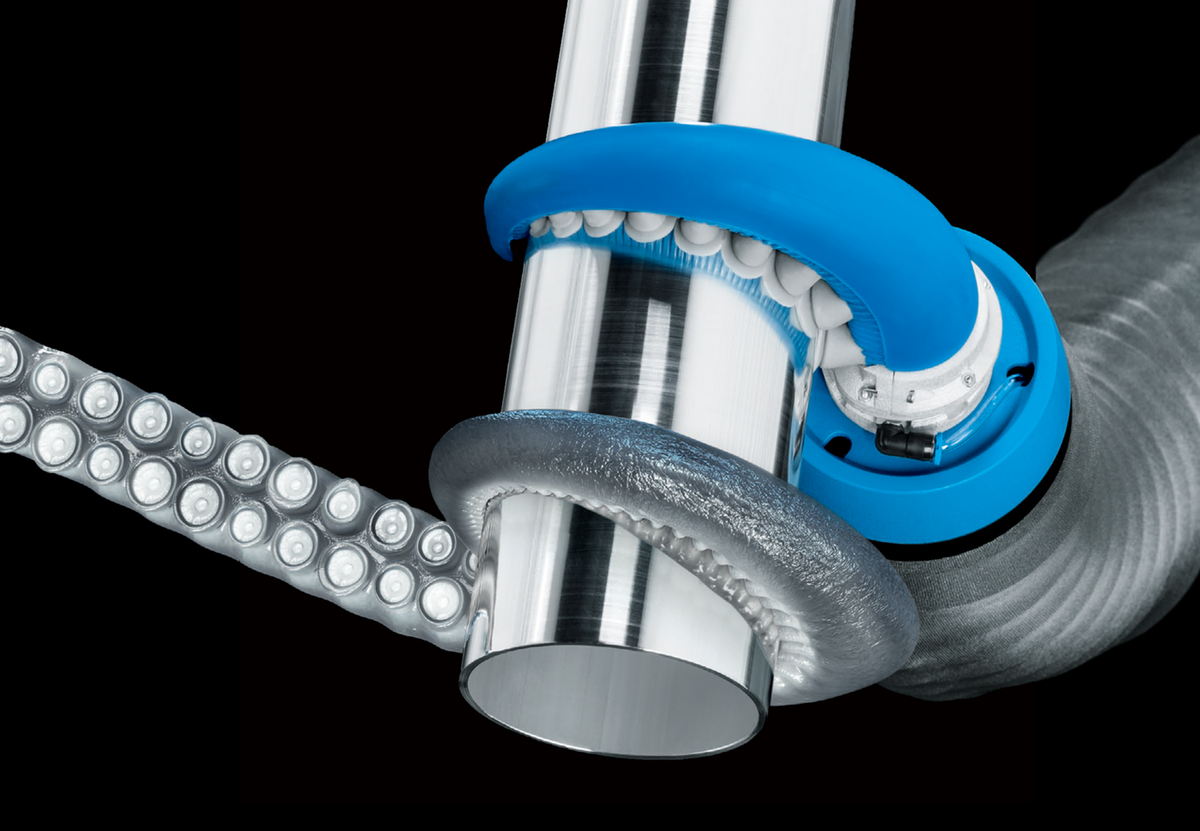
Researchers at Harvard’s Wyss Institute for Biologically Inspired Engineering and John A. Paulson School of Engineering and Applied Sciences (SEAS) and colleagues from Beihang University have developed an octopus-inspired soft robotic arm that can grip, move, and manipulate a wide range of objects. Its flexible, tapered design, complete with suction cups, gives the gripper a firm grasp on objects of all shapes, sizes and textures — from eggs to smartphones to large exercise balls.
“Most previous research on octopus-inspired robots focused either on mimicking the suction or the movement of the arm, but not both,” said co-first author August Domel, Ph.D., a Postdoctoral Scholar at Stanford University and former graduate student at the Wyss Institute and Harvard. “Our research is the first to quantify the tapering angles of the arms and the combined functions of bending and suction, which allows for a single small gripper to be used for a wide range of objects that would otherwise require the use of multiple grippers.”
The research is published in Soft Robotics.
The researchers began by studying the tapering angle of real octopus arms and quantifying which design for bending and grabbing objects would work best for a soft robot. Next, the team looked at the layout and structure of the suckers (yes, that is the scientific term) and incorporated them into the design.
“We mimicked the general structure and distribution of these suckers for our soft actuators,” said co-first author Zhexin Xie, Ph.D., a graduate student at Beihang University. “Although our design is much simpler than its biological counterpart, these vacuum-based biomimetic suckers can attach to almost any object.”
Xie is the co-inventor of the Festo Tentacle Gripper, which is the first fully integrated implementation of this technology in a commercial prototype.
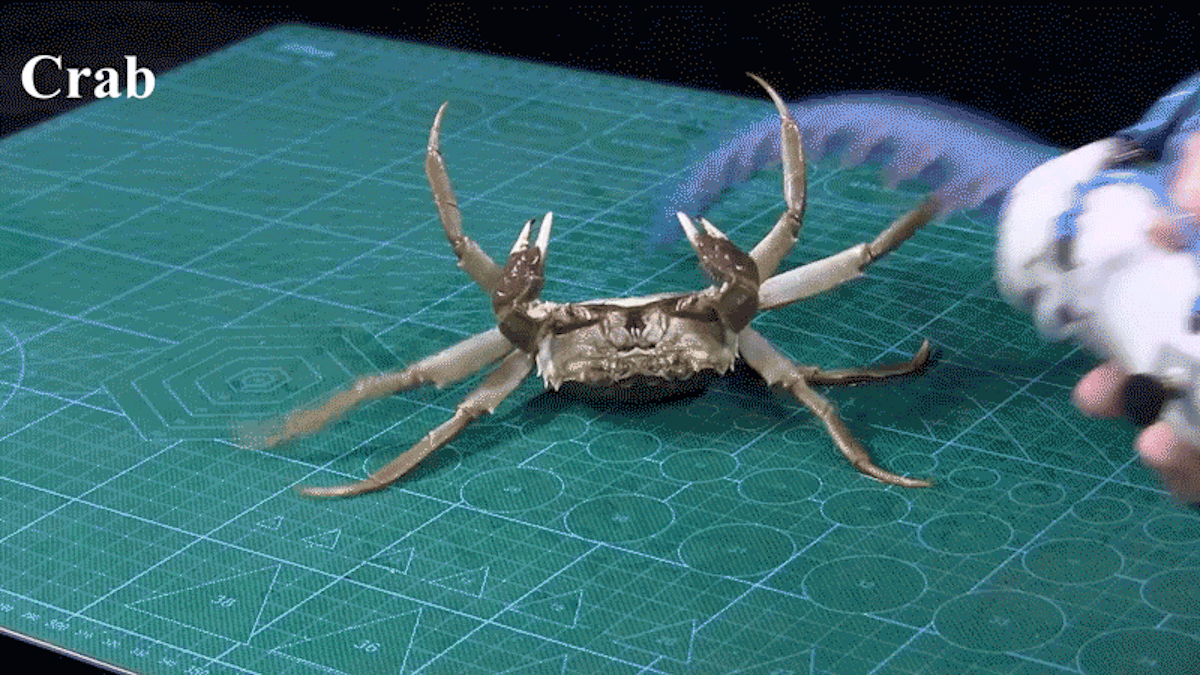
Researchers control the arm with two valves, one to apply pressure for bending the arm and one as a vacuum that engages the suckers. By changing the pressure and vacuum, the arm can attach to an object, wrap around it, carry it, and release it.
The researchers successfully tested the device on many different objects, including thin sheets of plastic, coffee mugs, test tubes, eggs, and even live crabs. The tapered design also allowed the arm to squeeze into confined spaces and retrieve objects.
“The results from our study not only provide new insights into the creation of next-generation soft robotic actuators for gripping a wide range of morphologically diverse objects, but also contribute to our understanding of the functional significance of arm taper angle variability across octopus species,” said Katia Bertoldi, Ph.D., an Associate Faculty member of the Wyss Institute who is also the William and Ami Kuan Danoff Professor of Applied Mechanics at SEAS, and co-senior author of the study.
This research was also co-authored by James Weaver from the Wyss Institute, Ning An and Connor Green from Harvard SEAS, Zheyuan Gong, Tianmiao Wang, and Li Wen from Beihang University, and Elias M. Knubben from Festo SE & Co. It was supported in part by the National Science Foundation under grant DMREF-1533985 and Festo Corporate’s project division.
Driverless shuttles: the latest from two European projects
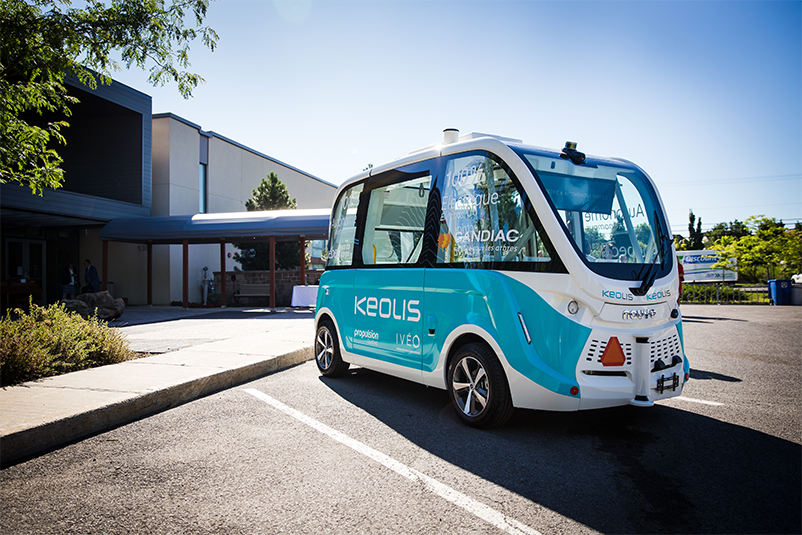
By Julianna Photopoulos
Jutting out into the sea, the industrial port area of Nordhavn in Denmark’s capital, Copenhagen, is currently being transformed into a futuristic waterfront city district made up of small islets. It’s billed as Scandinavia’s largest metropolitan development project and, when complete, will have living space for 40,000 people and workspace for another 40,000.
At the moment, Nordhavn is only served by a nearby S-train station and bus stops located near the station. There are no buses or trains running within the development area, although there are plans for an elevated metro line, and parking will be discouraged in the new neighbourhood. This is a great opportunity for autonomous vehicles (AVs) to operate as a new public transport solution, connecting this area more efficiently, says Professor Dimitri Konstantas at the University of Geneva in Switzerland.
‘We believe that AVs will become the new form of transport in Europe,’ he said. ‘We want to prove that autonomous vehicles are a sustainable, viable and environmental solution for urban and suburban public transportation.’
Prof. Konstantas is coordinating a project called AVENUE, which aims to do this in four European cities. In Nordhavn, the team plans to roll out autonomous shuttles on a loop with six stops around the seafront. They hope to have them up and running in two years. But once in place, the Nordhavn plan may provide a glimpse of how AV-based public transportation systems could work in the future.
Prof. Konstantas envisages these eventually becoming an on-demand, door-to-door service, where people can get picked up and go where they want rather than predetermined itineraries and bus stops.
In Nordhavn, AVENUE will test and implement an autonomous ‘mobility cloud’, currently under development, to link the shuttles with existing public transport, such as the nearby train station. An on-demand service will ultimately allow passengers to access the available transport with a single app, says Prof. Konstantas.
Integrating autonomous shuttles into the wider transport system is vital if they are to take off, says Guido Di Pasquale from the International Association of Public Transport (UITP) in Brussels, Belgium.
‘Autonomous vehicles have to be deployed as fleets of shared vehicles, fully integrated and complementing public transport,’ he said. ‘This is the only way we can ensure a sustainable usage of AVs in terms of space occupancy, traffic congestion and the environment.’
Single service
Di Pasquale points to a concept known as Mobility-as-a-Service (MaaS) as a possible model for future transport systems. This model combines both public and private transport. It allows users to create, manage and pay trips as a single service with an online account. For example, Uber, UbiGo in Sweden and Transport for Greater Manchester in the UK are exploring MaaS to enable users to get from one destination to another by combining transport and booking it as one trip, depending on their preferred option based on cost, time and convenience.
Di Pasquale coordinates a project called SHOW, which aims to deploy more than 70 automated vehicles in 21 European cities to assess how they can best be integrated with different wider transport systems and diverse users’ needs. They are testing combinations of AV types, from shuttles to cars and buses, in real-life conditions over the next four years. During this time, he expects the project’s AVs to transport more than 1,500,000 people and 350,000 containers of goods. ‘SHOW will be the biggest ever showcase and living lab for AV fleets,’ he said.
He says that most of the cities involved have tested autonomous last-mile vehicles in the past and are keen to include them in their future sustainable urban mobility plans.
However, rolling out AVs requires overcoming city-specific challenges, such as demonstrating safety.
‘Safety and security risks have restricted the urban use of AVs to dedicated lanes and low speed — typically below 20km/h,’ explained Di Pasquale. ‘This strongly diminishes their usefulness and efficiency, as in most city environments there is a lack of space and a high cost to keep or build such dedicated lanes.’
It could also deter users. ‘For most people, a speed barely faster than walking is not an attractive solution,’ he said.
We want to prove that autonomous vehicles are a sustainable, viable and environmental solution for urban and suburban public transportation.
Prof. Dimitri Konstantas, University of Geneva, Switzerland
Di Pasquale hopes novel technology will make higher speed and mixed traffic more secure, and guarantee fleets operating safely by monitoring and controlling them remotely.
Each city participating in SHOW will use autonomous vehicles in various settings, including mixed and dedicated lanes, at various speeds and types of weather. For safety and regulation reasons, all of them will have a driver present.
The objective is to make the vehicle fully autonomous without the need for a driver as well as optimise the service to encourage people to make the shift from ownership of cars to shared services, according to Di Pasquale. ‘This would also make on-demand and last-mile services sustainable in less densely populated areas or rural areas,’ he said.
Authorisation
But the technical issues of making the vehicle autonomous are only a part of the challenge.
There’s also the issue of who pays for it, says Di Pasquale. ‘AVs require sensors onboard, as well as adaptations to the physical and digital infrastructure to be deployed,’ he explained. ‘Their market deployment would require cities to drastically renew their fleets and infrastructures.’
SHOW’s pilots are scheduled to start in two years from now, as each city has to prepare by obtaining the necessary permits and getting the vehicles and technology ready, says Di Pasquale.
Getting authorisation to operate in cities is one of the biggest hurdles. City laws and regulations differ everywhere, says Prof. Konstantas.
AVENUE is still awaiting city licences to test in Nordhavn, despite a national law being passed on 1 July 2017 allowing for AVs to be tested in public areas. Currently, they have pilots taking place in Lyon, France and Luxembourg. In Geneva, the team has managed to get the required licences and the first worldwide on-demand, AV public transportation service will be rolled out on a 69-bus-stop circuit this summer.
AVENUE’s initial results show that cities need to make substantial investments to deploy AVs and to benefit from this technology. The legal and regulatory framework in Europe will also need to be adapted for smooth deployment of services, says Prof. Konstantas.
Both he and Di Pasquale hope their work can pave the way to convince operators and authorities to invest in fleets across Europe’s cities.
‘Depending on the willingness of public authorities, this can take up to four years until we see real, commercially sustainable AV-based public transportation services on a large scale in Europe,’ said Prof. Konstantas.
The research in this article was funded by the EU.
This post Driverless shuttles: what are we waiting for? was originally published on Horizon: the EU Research & Innovation magazine | European Commission.