Sensitive robots feel the strain
#275: Presented work at IROS 2018 (Part 2 of 3), with Robert Lösch, Ali Marjovi and Sophia Sakr
In this episode, Audrow Nash interviews Robert Lösch, Ali Marjovi, and Sophia Sakr about the work they presented at the 2018 International Conference on Intelligent Robots and Systems (IROS) in Madrid, Spain.
Robert Lösch is a PhD Student at Technische Universität Bergakademie Freiberg (TU Freiberg) in Germany, and he speaks on an approach to have robots navigate mining environments. Lösch discusses the challenges of operating in mines, such as humidity and wireless communication, his current platform, as well as future work.
Ali Marjovi is a Post doc at the École Polytechnique Fédérale de Lausanne (EPFL) in Switzerland, and he speaks about on how robots could be used to localize odors, which could be useful for finding explosives or for search-and-rescue. Marjovi discusses how odor localization works, his experimental setup, the challenges of odor localization, and on giving robots a sense of smell.
Sophia Sakr, from Institut des Systèmes Intelligents et de Robotique (ISIR) in France, speaks about a haptic pair of tweezers (designed by Thomas Daunizeau). She discusses how it works, how this can be used to control other devices, and the future direction of her work.
Links
Elowan: A plant-robot hybrid
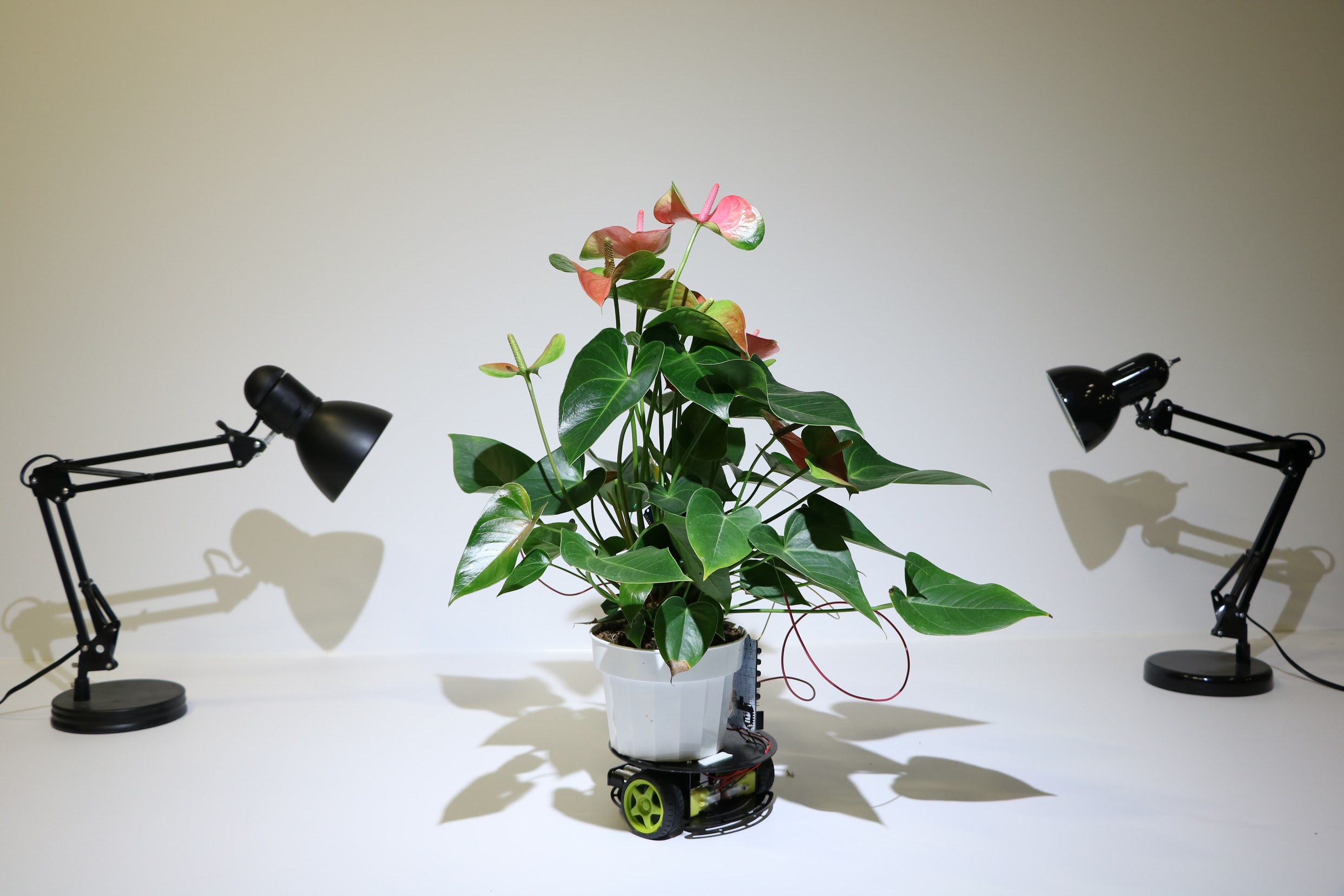
Elowan is a cybernetic lifeform, a plant in direct dialogue with a machine. Using its own internal electrical signals, the plant is interfaced with a robotic extension that drives it toward light.
Plants are electrically active systems. They get bio-electrochemically excited and conduct these signals between tissues and organs. Such electrical signals are produced in response to changes in light, gravity, mechanical stimulation, temperature, wounding, and other environmental conditions.
The enduring evolutionary processes change the traits of an organism based on its fitness in the environment. In recent history, humans domesticated certain plants, selecting the desired species based on specific traits. A few became house plants, while others were made fit for agricultural practice. From natural habitats to micro-climates, the environments for these plants have significantly altered. As humans, we rely on technological augmentations to tune our fitness to the environment. However, the acceleration of evolution through technology needs to move from a human-centric to a holistic, nature-centric view.
Elowan is an attempt to demonstrate what augmentation of nature could mean. Elowan’s robotic base is a new symbiotic association with a plant. The agency of movement rests with the plant based on its own bio-electrochemical signals, the language interfaced here with the artificial world.
These in turn trigger physiological variations such as elongation growth, respiration, and moisture absorption. In this experimental setup, electrodes are inserted into the regions of interest (stems and ground, leaf and ground). The weak signals are then amplified and sent to the robot to trigger movements to respective directions.
Such symbiotic interplay with the artificial could be extended further with exogenous extensions that provide nutrition, growth frameworks, and new defense mechanisms.
About Cyborg Botany
Cyborg Botany is a new, convergent view of interaction design in nature. Our primary means of sensing and display interactions in the environment are through our artificial electronics. However, there are a plethora of such capabilities that already exist in nature. Plants, for example, are active signal networks that are self-powered, self-fabricating, and self-regenerating systems at scale. They have the best kind of capabilities that an electronic device could carry. Instead of building completely discrete systems, the new paradigm points toward using the capabilities that exist in plants (and nature at large) and creating hybrids with our digital world.
Elowan, the robot plant hybrid, is one in a series of such of plant-electronic hybrid experiments.
Project Members:
Harpreet Sareen, Pattie Maes
Credits: Elbert Tiao (Graphics/Video), California Academy of Sciences (Leaf travel video clip)
The Montréal Declaration: Why we must develop AI responsibly
Yoshua Bengio, Université de Montréal
I have been doing research on intelligence for 30 years. Like most of my colleagues, I did not get involved in the field with the aim of producing technological objects, but because I have an interest in the the abstract nature of the notion of intelligence. I wanted to understand intelligence. That’s what science is: Understanding.
However, when a group of researchers ends up understanding something new, that knowledge can be exploited for beneficial or harmful purposes.
That’s where we are — at a turning point where the science of artificial intelligence is emerging from university laboratories. For the past five or six years, large companies such as Facebook and Google have become so interested in the field that they are putting hundreds of millions of dollars on the table to buy AI firms and then develop this expertise internally.
The progression in AI has since been exponential. Businesses are very interested in using this knowledge to develop new markets and products and to improve their efficiency.
So, as AI spreads in society, there is an impact. It’s up to us to choose how things play out. The future is in our hands.
Killer robots, job losses
From the get-go, the issue that has concerned me is that of lethal autonomous weapons, also known as killer robots.
While there is a moral question because machines have no understanding of the human, psychological and moral context, there is also a security question because these weapons could destabilize the world order.
Another issue that quickly surfaced is that of job losses caused by automation. We asked the question: Why? Who are we trying to bring relief to and from what? The trucker isn’t happy on the road? He should be replaced by… nobody?
We scientists seemingly can’t do much. Market forces determine which jobs will be eliminated or those where the workload will be lessened, according to the economic efficiency of the automated replacements. But we are also citizens who can participate in a unique way in the social and political debate on these issues precisely because of our expertise.
Computer scientists are concerned with the issue of jobs. That is not because they will suffer personally. In fact, the opposite is true. But they feel they have a responsibility and they don’t want their work to potentially put millions of people on the street.
Revising the social safety net
So strong support exists, therefore, among computer scientists — especially those in AI — for a revision of the social safety net to allow for a sort of guaranteed wage, or what I would call a form of guaranteed human dignity.
The objective of technological innovation is to reduce human misery, not increase it.
It is also not meant to increase discrimination and injustice. And yet, AI can contribute to both.
Discrimination is not so much due, as we sometimes hear, to the fact AI was conceived by men because of the alarming lack of women in the technology sector. It is mostly due to AI leading on data that reflects people’s behaviour. And that behaviour is unfortunately biased.
In other words, a system that relies on data that comes from people’s behaviour will have the same biases and discrimination as the people in question. It will not be “politically correct.” It will not act according to the moral notions of society, but rather according to common denominators.
Society is discriminatory and these systems, if we’re not careful, could perpetuate or increase that discrimination.
There could also be what is called a feedback loop. For example, police forces use this kind of system to identify neighbourhoods or areas that are more at-risk. They will send in more officers… who will report more crimes. So the statistics will strengthen the biases of the system.
The good news is that research is currently being done to develop algorithms that will minimize discrimination. Governments, however, will have to bring in rules to force businesses to use these techniques.
Saving lives
There is also good news on the horizon. The medical field will be one of those most affected by AI — and it’s not just a matter of saving money.
Doctors are human and therefore make mistakes. So the more we develop systems with more data, fewer mistakes will occur. Such systems are more precise than the best doctors. They are already using these tools so they don’t miss important elements such as cancerous cells that are difficult to detect in a medical image.
There is also the development of new medications. AI can do a better job of analyzing the vast amount of data (more than what a human would have time to digest) that has been accumulated on drugs and other molecules. We’re not there yet, but the potential is there, as is more efficient analysis of a patient’s medical file.
We are headed toward tools that will allow doctors to make links that otherwise would have been very difficult to make and will enable physicians to suggest treatments that could save lives.
The chances of the medical system being completely transformed within 10 years are very high and, obviously, the importance of this progress for everyone is enormous.
I am not concerned about job losses in the medical sector. We will always need the competence and judgment of health professionals. However, we need to strengthen social norms (laws and regulations) to allow for the protection of privacy (patients’ data should not be used against them) as well as to aggregate that data to enable AI to be used to heal more people and in better ways.
The solutions are political
Because of all these issues and others to come, the Montréal Declaration for Responsible Development of Artificial Intelligence is important. It was signed Dec. 4 at the Society for Arts and Technology in the presence of about 500 people.
It was forged on the basis of vast consensus. We consulted people on the internet and in bookstores and gathered opinion in all kinds of disciplines. Philosophers, sociologists, jurists and AI researchers took part in the process of creation, so all forms of expertise were included.
There were several versions of this declaration. The first draft was at a forum on the socially responsible development of AI organized by the Université de Montréal on Nov. 2, 2017.
That was the birthplace of the declaration.
Its goal is to establish a certain number of principles that would form the basis of the adoption of new rules and laws to ensure AI is developed in a socially responsible manner. Current laws are not always well adapted to these new situations.
And that’s where we get to politics.
The abuse of technology
Matters related to ethics or abuse of technology ultimately become political and therefore belong in the sphere of collective decisions.
How is society to be organized? That is political.
What is to be done with knowledge? That is political.
I sense a strong willingness on the part of provincial governments as well as the federal government to commit to socially responsible development.
Because Canada is a scientific leader in AI, it was one of the first countries to see all its potential and to develop a national plan. It also has the will to play the role of social leader.
Montréal has been at the forefront of this sense of awareness for the past two years. I also sense the same will in Europe, including France and Germany.
Generally speaking, scientists tend to avoid getting too involved in politics. But when there are issues that concern them and that will have a major impact on society, they must assume their responsibility and become part of the debate.
And in this debate, I have come to realize that society has given me a voice — that governments and the media were interested in what I had to say on these topics because of my role as a pioneer in the scientific development of AI.
So, for me, it is now more than a responsibility. It is my duty. I have no choice.
Yoshua Bengio, Professeur titulaire, Département d’informatique et de recherche opérationnelle, Université de Montréal
This article is republished from The Conversation under a Creative Commons license. Read the original article.
Inside “The Laughing Room”
Still photos courtesy of metaLAB (at) Harvard.
By Brigham Fay
“The Laughing Room,” an interactive art installation by author, illustrator, and MIT graduate student Jonathan “Jonny” Sun, looks like a typical living room: couches, armchairs, coffee table, soft lighting. This cozy scene, however, sits in a glass-enclosed space, flanked by bright lights and a microphone, with a bank of laptops and a video camera positioned across the room. People wander in, take a seat, begin chatting. After a pause in the conversation, a riot of canned laughter rings out, prompting genuine giggles from the group.
Presented at the Cambridge Public Library in Cambridge, Massachusetts, Nov. 16-18, “The Laughing Room” was an artificially intelligent room programmed to play an audio laugh track whenever participants said something that its algorithm deemed funny. Sun, who is currently on leave from his PhD program within the MIT Department of Urban Studies and Planning, is an affiliate at the Berkman Klein Center for Internet and Society at Harvard University, and creative researcher at the metaLAB at Harvard, created the project to explore the increasingly social and cultural roles of technology in public and private spaces, users’ agency within and dependence on such technology, and the issues of privacy raised by these systems. The installations were presented as part of ARTificial Intelligence, an ongoing program led by MIT associate professor of literature Stephanie Frampton that fosters public dialogue about the emerging ethical and social implications of artificial intelligence (AI) through art and design.
Setting the scene
“Cambridge is the birthplace of artificial intelligence, and this installation gives us an opportunity to think about the new roles that AI is playing in our lives every day,” said Frampton. “It was important to us to set the installations in the Cambridge Public Library and MIT Libraries, where they could spark an open conversation at the intersections of art and science.”
“I wanted the installation to resemble a sitcom set from the 1980s–a private, familial space,” said Sun. “I wanted to explore how AI is changing our conception of private space, with things like the Amazon Echo or Google Home, where you’re aware of this third party listening.”
“The Control Room,” a companion installation located in Hayden Library at MIT, displayed a live stream of the action in “The Laughing Room,” while another monitor showed the algorithm evaluating people’s speech in real time. Live streams were also shared online via YouTube and Periscope. “It’s an extension of the sitcom metaphor, the idea that people are watching,” said Sun. The artist was interested to see how people would act, knowing they had an audience. Would they perform for the algorithm? Sun likened it to Twitter users trying to craft the perfect tweet so it will go viral.
Programming funny
“Almost all machine learning starts from a dataset,” said Hannah Davis, an artist, musician, and programmer who collaborated with Sun to create the installation’s algorithm. She described the process at an “Artists Talk Back” event held Saturday, Nov. 17, at Hayden Library. The panel discussion included Davis; Sun; Frampton; collaborator Christopher Sun, research assistant Nikhil Dharmaraj, Reinhard Engels, manager of technology and innovation at Cambridge Public Library, Mark Szarko, librarian at MIT Libraries, and Sarah Newman, creative researcher at the metaLAB. The panel was moderated by metaLAB founder and director Jeffrey Schnapp.
Davis explained how, to train the algorithm, she scraped stand-up comedy routines from YouTube, selecting performances by women and people of color to avoid programming misogyny and racism into how the AI identified humor. “It determines what is the setup to the joke and what shouldn’t be laughed at, and what is the punchline and what should be laughed at,” said Davis. Depending on how likely something is to be a punchline, the laugh track plays at different intensities.
Fake laughs, real connections
Sun acknowledged that the reactions from “The Laughing Room” participants have been mixed: “Half of the people came out saying ‘that was really fun,’” he said. “The other half said ‘that was really creepy.’”
That was the impression shared by Colin Murphy, a student at Tufts University who heard about the project from following Sun on Twitter: “This idea that you are the spectacle of an art piece, that was really weird.”
“It didn’t seem like it was following any kind of structure,” added Henry Scott, who was visiting from Georgia. “I felt like it wasn’t laughing at jokes, but that it was laughing at us. The AI seems mean.”
While many found the experience of “The Laughing Room” uncanny, for others it was intimate, joyous, even magical.
“There’s a laughter that comes naturally after the laugh track that was interesting to me, how it can bring out the humanness,” said Newman at the panel discussion. “The work does that more than I expected it to.”
Frampton noted how the installation’s setup also prompted unexpected connections: “It enabled strangers to have conversations with each other that wouldn’t have happened without someone listening.”
Continuing his sitcom metaphor, Sun described these first installations as a “pilot,” and is looking forward to presenting future versions of “The Laughing Room.” He and his collaborators will keep tweaking the algorithm, using different data sources, and building on what they’ve learned through these installations. “The Laughing Room” will be on display in the MIT Wiesner Student Art Gallery in May 2019, and the team is planning further events at MIT, Harvard, and Cambridge Public Library throughout the coming year.
“This has been an extraordinary collaboration and shown us how much interest there is in this kind of programming and how much energy can come from using the libraries in new ways,” said Frampton.
“The Laughing Room” and “The Control Room” were funded by the metaLAB (at) Harvard, the MIT De Florez Fund for Humor, the Council of the Arts at MIT, and the MIT Center For Art, Science and Technology and presented in partnership with the Cambridge Public Library and the MIT Libraries.
Waymo soft launches in Phoenix, but…
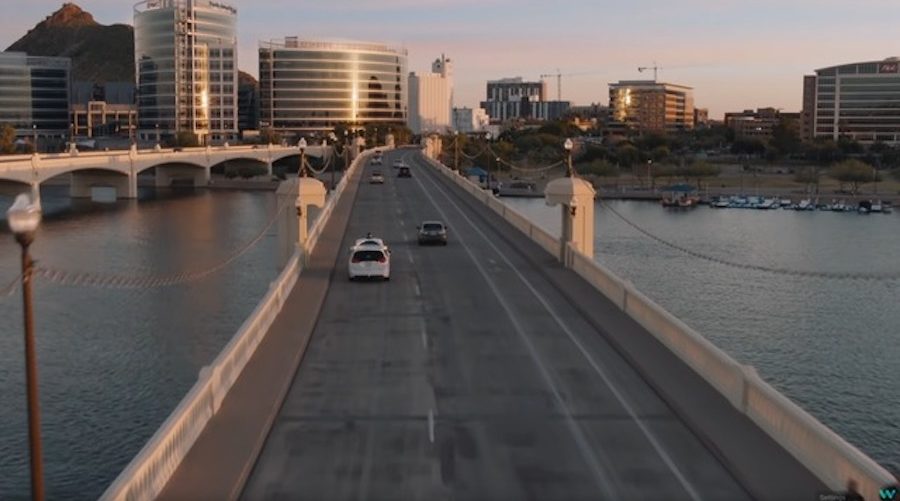
Waymo announced today they will begin commercial operations in the Phoenix area under the name “Waymo One.” Waymo has promised that it would happen this year, and it is a huge milestone, but I can’t avoid a small bit of disappointment.
Regular readers will know I am a huge booster of Waymo, not simply because I worked on that team in its early years, but because it is clearly the best by every metric we know. However, this pilot rollout is also quite a step down from what was anticipated, though for sensible reasons.
- At first, it is only available to the early rider program members. In fact, it’s not clear that this is any different from what they had before, other than it is more polished and there is a commercial charging structure (not yet published.)
- Vehicles will continue to operate with safety drivers.
Other companies — including Waymo, Uber, Lyft and several others — have offered limited taxi services with safety drivers. This service is mainly different in its polish and level of development — or at least that’s all we have been told. They only say they “hope” to expand it to people outside the early rider program soon.
In other words, Waymo has missed the target it set of a real service in 2018. It was a big, hairy audacious target, so there is no shame or surprise in missing it, and it may not be missed by much.
There is a good reason for missing the target. The Uber fatality, right in that very operation area, has everybody skittish. The public. Developers. Governments. It used up the tolerance the public would normally have for mistakes. Waymo can’t take the risk of a mistake, especially in Phoenix, especially now, and especially if it is seen it came about because they tried to go too fast, or took new risks like dropping safety drivers.
I suspect at Waymo they had serious talks about not launching in Phoenix, in spite of the huge investment there. But in the end, changing towns may help, but not enough. Everybody is slowed down by this. Even an injury-free accident that could have had an injury will be problematic — and the truth is, as the volume of service increases, that’s coming.
It was terribly jarring for me to watch Waymo’s introduction video. I set it to play at one minute, where they do the big reveal and declare they are “Introducing the self driving service.”
The problem? The car is driving down N. Mill Avenue in Tempe, the road on which Uber killed Elaine Herzberg, about 1,100 feet from the site of her death. Waymo assures me that this was entirely unintentional — and those who live outside the area or who did not study the accident may not recognize it — but it soured the whole launch for me.
What Caused Rethink Robotics to Shut Down?
Fauna Robotica
Universal Robots Celebrates 10 Year Anniversary of Selling the World’s First Commercially Viable Collaborative Robot
LiDAR Solution for Mass Production and Adoption
Brain Corp to Provide AI Services to Walmart
Visual model-based reinforcement learning as a path towards generalist robots
By Chelsea Finn∗, Frederik Ebert∗, Sudeep Dasari, Annie Xie, Alex Lee, and Sergey Levine
With very little explicit supervision and feedback, humans are able to learn a wide range of motor skills by simply interacting with and observing the world through their senses. While there has been significant progress towards building machines that can learn complex skills and learn based on raw sensory information such as image pixels, acquiring large and diverse repertoires of general skills remains an open challenge. Our goal is to build a generalist: a robot that can perform many different tasks, like arranging objects, picking up toys, and folding towels, and can do so with many different objects in the real world without re-learning for each object or task.
While these basic motor skills are much simpler and less impressive than mastering Chess or even using a spatula, we think that being able to achieve such generality with a single model is a fundamental aspect of intelligence.
The key to acquiring generality is diversity. If you deploy a learning algorithm in a narrow, closed-world environment, the agent will recover skills that are successful only in a narrow range of settings. That’s why an algorithm trained to play Breakout will struggle when anything about the images or the game changes. Indeed, the success of image classifiers relies on large, diverse datasets like ImageNet. However, having a robot autonomously learn from large and diverse datasets is quite challenging. While collecting diverse sensory data is relatively straightforward, it is simply not practical for a person to annotate all of the robot’s experiences. It is more scalable to collect completely unlabeled experiences. Then, given only sensory data, akin to what humans have, what can you learn? With raw sensory data there is no notion of progress, reward, or success. Unlike games like Breakout, the real world doesn’t give us a score or extra lives.
We have developed an algorithm that can learn a general-purpose predictive model using unlabeled sensory experiences, and then use this single model to perform a wide range of tasks.
With a single model, our approach can perform a wide range of tasks, including lifting objects, folding shorts, placing an apple onto a plate, rearranging objects, and covering a fork with a towel.
In this post, we will describe how this works. We will discuss how we can learn based on only raw sensory interaction data (i.e. image pixels, without requiring object detectors or hand-engineered perception components). We will show how we can use what was learned to accomplish many different user-specified tasks. And, we will demonstrate how this approach can control a real robot from raw pixels, performing tasks and interacting with objects that the robot has never seen before.
Reproducing paintings that make an impression
Image courtesy of the researchers
By Rachel Gordon
The empty frames hanging inside the Isabella Stewart Gardner Museum serve as a tangible reminder of the world’s biggest unsolved art heist. While the original masterpieces may never be recovered, a team from MIT’s Computer Science and Artificial Intelligence Laboratory (CSAIL) might be able to help, with a new system aimed at designing reproductions of paintings.
RePaint uses a combination of 3-D printing and deep learning to authentically recreate favorite paintings — regardless of different lighting conditions or placement. RePaint could be used to remake artwork for a home, protect originals from wear and tear in museums, or even help companies create prints and postcards of historical pieces.
“If you just reproduce the color of a painting as it looks in the gallery, it might look different in your home,” says Changil Kim, one of the authors on a new paper about the system, which will be presented at ACM SIGGRAPH Asia in December. “Our system works under any lighting condition, which shows a far greater color reproduction capability than almost any other previous work.”
To test RePaint, the team reproduced a number of oil paintings created by an artist collaborator. The team found that RePaint was more than four times more accurate than state-of-the-art physical models at creating the exact color shades for different artworks.
At this time the reproductions are only about the size of a business card, due to the time-costly nature of printing. In the future the team expects that more advanced, commercial 3-D printers could help with making larger paintings more efficiently.
While 2-D printers are most commonly used for reproducing paintings, they have a fixed set of just four inks (cyan, magenta, yellow, and black). The researchers, however, found a better way to capture a fuller spectrum of Degas and Dali. They used a special technique they call “color-contoning,” which involves using a 3-D printer and 10 different transparent inks stacked in very thin layers, much like the wafers and chocolate in a Kit-Kat bar. They combined their method with a decades-old technique called half-toning, where an image is created by lots of little colored dots rather than continuous tones. Combining these, the team says, better captured the nuances of the colors.
With a larger color scope to work with, the question of what inks to use for which paintings still remained. Instead of using more laborious physical approaches, the team trained a deep-learning model to predict the optimal stack of different inks. Once the system had a handle on that, they fed in images of paintings and used the model to determine what colors should be used in what particular areas for specific paintings.
Despite the progress so far, the team says they have a few improvements to make before they can whip up a dazzling duplicate of “Starry Night.” For example, mechanical engineer Mike Foshey said they couldn’t completely reproduce certain colors like cobalt blue due to a limited ink library. In the future they plan to expand this library, as well as create a painting-specific algorithm for selecting inks, he says. They also can hope to achieve better detail to account for aspects like surface texture and reflection, so that they can achieve specific effects such as glossy and matte finishes.
“The value of fine art has rapidly increased in recent years, so there’s an increased tendency for it to be locked up in warehouses away from the public eye,” says Foshey. “We’re building the technology to reverse this trend, and to create inexpensive and accurate reproductions that can be enjoyed by all.”
Kim and Foshey worked on the system alongside lead author Liang Shi; MIT professor Wojciech Matusik; former MIT postdoc Vahid Babaei, now Group Leader at Max Planck Institute of Informatics; Princeton University computer science professor Szymon Rusinkiewicz; and former MIT postdoc Pitchaya Sitthi-Amorn, who is now a lecturer at Chulalongkorn University in Bangkok, Thailand.
This work is supported in part by the National Science Foundation.
The end of parking as we know it
A day before snow hindered New York commuters, researchers at the University of Iowa and Princeton identified the growth of urbanization as the leading cause for catastrophic storm damage. Wednesday’s report stated that the $128 billion wake of Hurricane Harvey was 21 times greater due to the population density of Houston, one of America’s fastest growing cities. This startling statistic is even more alarming in light of a recent UN study which reported that 70% of the projected 9.7 billion people in the world will live in urban centers by 2050. Superior urban management is one of the major promises of autonomous systems and smart cities.
Today, one of the biggest headaches for civil planners is the growth of traffic congestion and demand for parking, especially considering cars are one of the most inefficient and expensive assets owned by Americans. According to the Governors Highway Safety Association the average private car is parked 95% of the time. Billions of dollars of real-estate in America is dedicated to parking. For example, in a city like Seattle 40% of the land is consumed by parking. Furthermore, INRIX analytics estimates that more than $70 billion is spent by Americans looking for parking. The average driver wastes $345 a year in time, fuel and emissions. “Parking pain costs much moreNew Yorkers spend 107 hours a year looking for parking spots at a cost of $2,243 per driver,” states INRIX.This month I spoke with Dr. Anuja Sonalker about her plan to save Americans billions of dollars from parking. Dr. Sonalker is the founder of STEER Technologies, a full-service auto-valet platform that is providing autonomous solutions to America’s parking pain. The STEER value proposition uses a sensor array that easily connects to a number of popular automobile models, seamlessly controlled by one’s smart phone. As Dr. Sonalker explains, “Simply put STEER allows a vehicle user to pull over at a curb (at certain destinations), and with a press of a button let the vehicle go find a parking spot and park for you. When it’s time to go, simply summon the vehicle and it comes back to get you.” An added advantage of STEER is its ability to conserve space, as cars can be parked very close together since computers don’t use doors.
Currently, STEER is piloting its technology near its Maryland headquarters. In describing her early success, Dr. Sonalker boasts, “We have successfully completed testing various scenarios under different weather, and lighting conditions at malls, train stations, airports, parks, construction sites, downtown areas. We have also announced launch dates in late 2019 with the Howard Hughes Corporation to power the Merriweather district – a 4.9 Million square foot new smart development in Columbia, MD, and the BWI airport.” The early showing from STEER’s performance is the results of its proprietary product that is built for all seasons and topographies. “Last March, we demonstrated live in Detroit under a very fast snowstorm system. Within less than an hour the ground was covered in 2+ inches of snow,” describes Dr. Sonalker. “No lane markings were visible any more, and parking lines certainly were not visible. The STEER car successfully completed its mission to ‘go park’, driving around the parking lot, recognizing other oncoming vehicles, pacing itself accordingly and locating and manoeuvring itself into a parking spot among other parked vehicles in that weather.”
In breaking down the STEER solution, Dr. Sonalker expounds, “The technology is built with a lean sensor suite, its cost equation is very favorable to both after market and integrated solutions for consumer ownership.” She further clarifies, “From a technology stand point both solutions are identical in the feature they provide. The difference lies in how the technology is integrated into the vehicle. For after market, STEER’s technology runs on additional hardware that is retrofitted to the vehicle. In an integrated solution STEER’s technology would be housed on an appropriate ECU driven by vehicle economics and architecture, but with a tight coupling with STEER’s software. The coupling will be cross layered in order to maintain the security posture.” Unlike many self-driving applications that rely heavily on LIDAR (Light Detection And Ranging), STEER uses location mapping of predetermined parking structures along with computer vision. I pressed Dr. Sonalker about her unusual setup, “Yes, it’s true we don’t use LIDAR. You see, STEER started from the principle of security-led design which is where we start from a minimum design, minimum attack surface, maximum default security.”
I continued my interview of Dr. Sonalker to learn how she plans to roll out the platform, “In the long term, we expect to be a feature on new vehicles as they roll out of the assembly line. 2020-2021 seems to be happening based on our current OEM partner targets. Our big picture vision is that people no longer have to think about what to do with their cars when they get to their destination. The equivalent effect of ride sharing – your ride ends when you get off. There will be a network of service points that your vehicle will recognize and go park there until you summon it for use again.” STEER’s solution is part of a growing fleet of new smart city initiatives cropping up across the automation landscape. At last year’s Consumer Electronic Show, German auto supplier Robert Bosch GmbH unveiled its new crowd-sourcing parking program called, “community-based parking.” Using a network of cameras and sensors to identify available parking spots Bosch’s cloud network automatically directs cars to the closest spot. This is part of Bosch’s larger urban initiative, as the company’s president Mike Mansuetti says, “You could say that our sensors are the eyes and ears of the connected city. In this case, its brain is our software. Of Bosch’s nearly 400,000 associates worldwide, more than 20,000 are software engineers, nearly 20% of whom are working exclusively on the IoT. We supply an open software platform called the Bosch IoT Suite, which offers all the functions necessary to connect devices, users, and companies.”
As the world grabbles with population explosion exacerbated by cars strangling city centers, civil engineers are challenging technologists to reimagine urban communities. Today, most cars are dormant, and when used run at one-fifth capacity with typical trips less than a mile from one’s home (easily accessible on foot or bike). In the words Dr. Sonalker, “All autonomous technologies will lead to societal change. AV Parking will result in more efficient utilization of existing spaces, fitting more in the same spaces, better use of underutilized remote lots, and frankly, even shunting parking off to further remote locations and using prime space for more enjoyable activities.”
Join the next RobotLab forum discussing “Cybersecurity & Machines” to learn how hackers are attacking the the ecosystem of smart cities and autonomous vehicles with John Frankel of ffVC and Guy Franklin of SOSA on February 12th in New York City, RSVP Today!