Brain Corp. raises another $36M for mobile robots that clean during pandemic
A highly performing and efficient e-skin for robotic applications
Smarter, lighter exoskeletons to provide better mobility therapy
What Are the Costs of Implementing Asset Tracking? A Guide for SMBs
#308: Seeing like a Rover, with Janet Vertessi
In this episode, Audrow Nash speaks with Janet Vertessi, Assistant Professor of Sociology at Princeton, on her book Seeing Like a Rover: How Robots, Teams, and Images Craft Knowledge of Mars. The book is written about her experience living and working with NASA’s Mars Rover team, and includes her observations about the team’s leadership and their relationship with their robot millions of miles away on Mars. She also gives some advice from her findings for teams.
Janet Vertessi
Janet Vertesi specializes in the sociology of science, knowledge, and technology. She has spent the past 7 years studying several NASA spacecraft teams as an ethnographer. Her book, Seeing like a Rover: Images and Interaction on the Mars Exploration Rover Mission (Chicago, 2014) draws on over two years of ethnographic immersion with the Mars Exploration Rover mission to show how scientists and engineers use digital images to conduct scientific research on another planet. She is currently working on followup study of the NASA-ESA Cassini mission to Saturn focusing on the role of sociotechnical organization in research, data-sharing, and decision-making on robotic spacecraft teams. Vertesi is also interested in the digital sociology: whether studying computational systems in social life, shifting sociological methods online, or applying sociological insights to build new technologies. She holds a Master’s degree from Cambridge and a PhD from Cornell, has received several grants from the National Science Foundation, and was awarded the Hacker-Mullins prize for best graduate student paper from the American Sociological Association, Science, Knowledge and Technology section in 2007.
Links
Challenges for the Packaging Industry
Conduct-A-Bot system uses muscle signals to enable more natural human-robot communication
Where are the robots when you need them!
Looking at the Open Source COVID-19 Medical Supplies production tally of handcrafted masks and faceshields, we’re trying to answer that question in our weekly discussions about ‘COVID-19, robots and us’. We talked to Rachel ‘McCrafty’ Sadd has been building systems and automation for COVID mask making, as the founder of Project Mask Making and #distillmyheart projects in the SF Bay Area, an artist and also as Executive Director of Ace Monster Toys makerspace/studio. Rachel has been organizing volunteers and automating workflows to get 1700 cloth masks hand sewn and distributed to people at risk before the end of April. “Where’s my f*king robot!” was the theme of her short presentation.
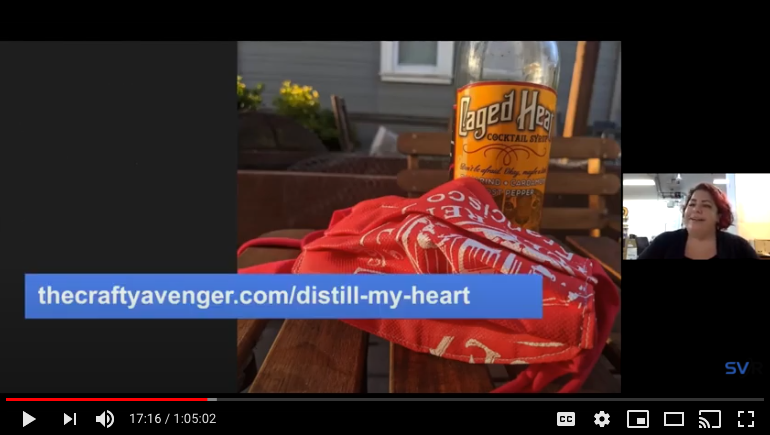
If you think that volunteer efforts aren’t able to make a dent in the problems, here’s the most recent (4/20/20) production tally for the group Open Source COVID-19 Medical Supplies, who speak regularly on this web series. One volunteer group has tallied efforts by volunteers across 45 countries who have so far produced 2,315,559 pieces of PPE. And that’s not counting the #distillmyheart masks. Here’s Rachel’s recent interview on KTVU. Those masks aren’t going to make themselves, people!
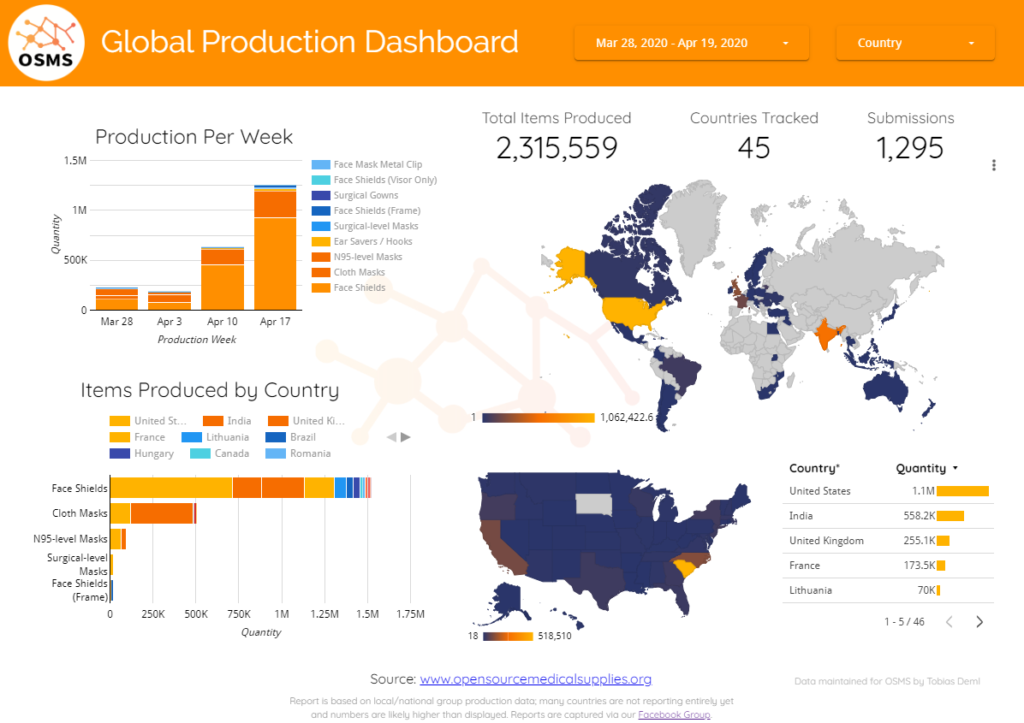
We also heard from Robin Murphy, Expert in Rescue Robotics & Raytheon Professor at Texas A&M University, who updated her slides on the way in which robots are being used in COVID-19 response. You can find more information on Robotics for Infectious Diseases, an organization formed in response to the Ebola outbreak and chaired by Dr Murphy. There is also a series of interviews answering any questions a roboticist might have about deploying robots with public health, public safety and emergency managers.
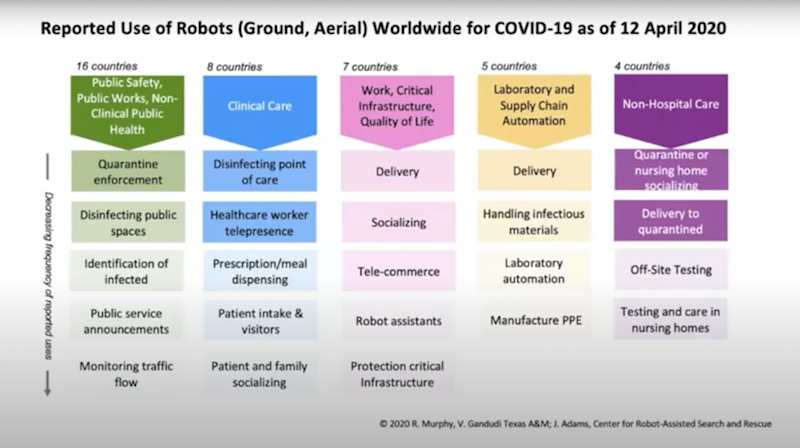
Next we heard from Missy Cummings, Expert in Robotics Safety & Professor at Duke University. “I’ve been doing robotics, certification testing and certification for almost 10 years now. I started out in drones. And then kind of did a segue over into driverless cars and I also work on medical systems. So I work in this field of safety critical systems, where the operation of the robot in terms of the drone or the car or the medical robot, it can actually do damage to people if not designed correctly. Here’s a link to a paper that I’ve written for AI Magazine that’s really looking at the maturity of driverless cars.“
“I spent a ridiculous amount of time on Capitol Hill trying to to be a middle ground between, yes, these are good technologies. We want to do research and investment and keep keep building a capacity. But no, we’re not ready to have widespread deployment yet. And I don’t care what Elon Musk says you’re not getting full self driving anytime soon.”
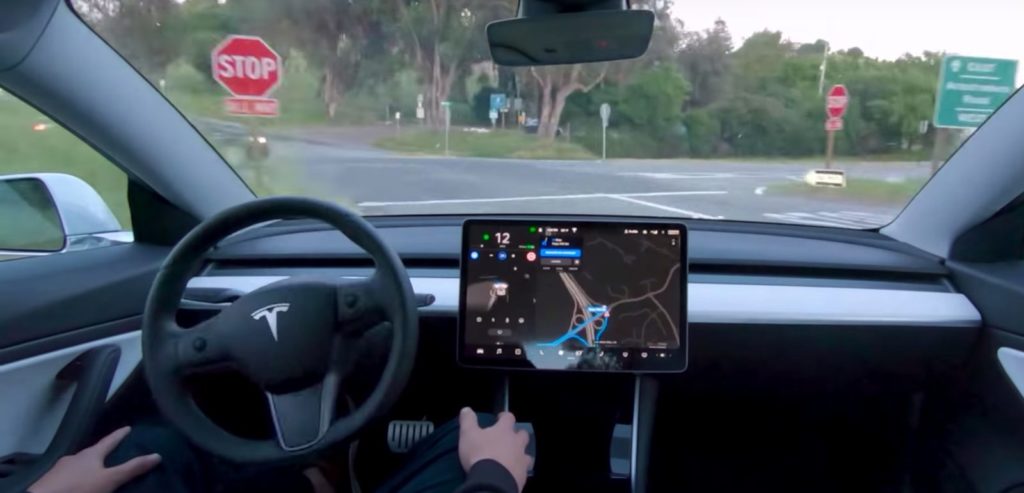
“Any reasoning system has to go through four levels of reasoning, you start at the basics, what we call skill based reasoning, then you go up to rule knowledge and expert based reasoning. And so where do we see that in cars? When you learn to drive you had to learn skill based reasoning, which was learning for example, how to track light lines on the road. Once you did that, maybe 20 minutes to learn that then you never actually have a problem with that again.”
“So once you have the cognitive capacity that you’ve learned skills, then you have enough spare mental capacity to think about rule based reasoning. And that’s when you start to understand, Okay, I see this octagon in front of me, it is a stop sign it’s read, I know that what it means there’s a set of procedures that go along with stopping and I’m going to follow those when I see it. Then once you have the rules of the environment that you’ve learned, then you have the spare capacity to start thinking about knowledge base reasoning, the big jump between rule and knowledge base reasoning is the ability to judge under uncertainty. So this is where you start to see the uncertainty arrow growing. So when you go up to knowledge base reasoning, you are starting to have to make guesses about the world with imperfect information. “
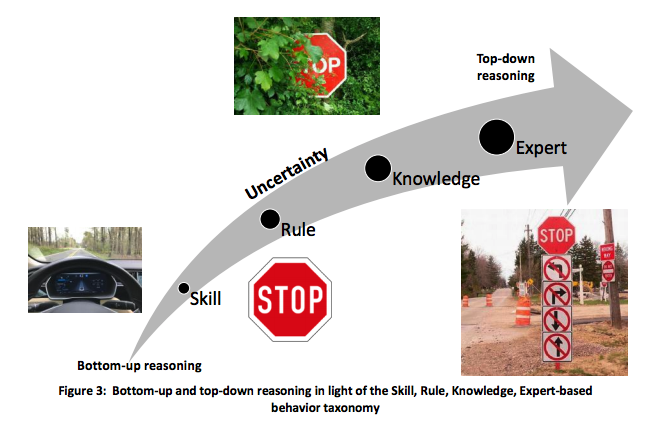
“So I like to show this picture of a stop sign partially obscured by vegetation. There are many many, many driverless car system computer vision systems right now that cannot figure this out that if they see some level of partially obscured stop sign, they just cannot see because they don’t see the way that we see. They don’t see the complete picture. And so they don’t judge that there’s a stop sign there. And you might have seen the recent case of the Tesla being tricked by a partially modified 35 mile per hour sign with a little bit of tape to make it see 85 miles per hour. It’s a really good illustration of just how brittle machine learning deep learning is when it comes to perceptual based reasoning. And then we get to the highest level of reasoning where you really have to make judgments under maximum uncertainty. “
“I love this illustration of this stop sign with these four different arrows. You cannot do this, you cannot turn left, you cannot go right, you cannot go straight and you cannot go back. So I’d be curious, I’d like to see what any driverless car would do in this situation. Because what do you do in the situation? You have to break one of these rules, you have to make a judgment, you have to figure out what is the best possible way to get yourself out of the system. And it means that you’re going to have to break rules inside the system. So the expert base reasoning knowledge base reasoning there is what we call top down reasoning, it’s you taking experience judgment in the world that you’ve had. As you’ve gotten older in life and had more experiences, you bring that to bear to make better guesses about what you should do in the future.”
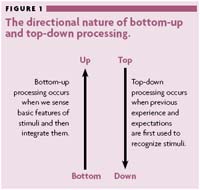
“Bottom up reasoning is, is essentially what is happening in machine learning. You’re taking all the bits and bytes of information from the world, and then processing that to then make some kind of action. So right now Computers are really good at skill based reasoning, some rule based reasoning, humans are really the best at knowledge and expert based reasoning. And this is something we call functional allocation. But the problem is there’s a big break between rule and knowledge. Driverless cars cannot do this right now, until we can make that jump into knowledge and expert base reasoning. What are we going to do have to do?” [Missy Cummings, Robot Safety Expert]
Michael Sayre, CEO Cognicept said “I’m working with a company called Cognizant as CEO. We’re essentially solving a lot of the problems that Missy highlighted, which is that, you know, when we look at autonomy, and moving robots into the real world, there are a lot of complexities about the real world that cause what we call edge case failure in these systems. And so what we’ve built is essentially a system that allows a confused robot to dial out for human operator.”
“Human in the loop is not a new idea. This is something that self driving cars have used. What we’ve built is essentially a system and a service that allows this confused robot to dialogue for help on real time basis. We essentially listen for intervention requests from robots. So that can be an error code, or, you know, some kind of failure of the system timeouts, whatever it is really, we can listen for that event. And then we cause a ticket to be registered in our system, which our operators will then see, that connects them to the robot, they can kind of get a sense of what’s happening in the robots environment, they can get sensor information, populated in a 3d canvas, we can see videos and so forth, that allow the operator to make judgments on the robots behalf. “
“Self driving vehicles is probably not the best example for us. But maybe you would be able to use our system in something like a last mile delivery vehicle, which will face a lot of the same problems. Maybe the robots uncertain about whether it can cross the road. We can have a look at the video feed from that robot, understand what the traffic signals are saying, or what the environment looks like. And then give the robotic command to essentially help it with getting past the scenario that caused that Case failure. So we see this as sort of a way to help get robots into more useful service.”
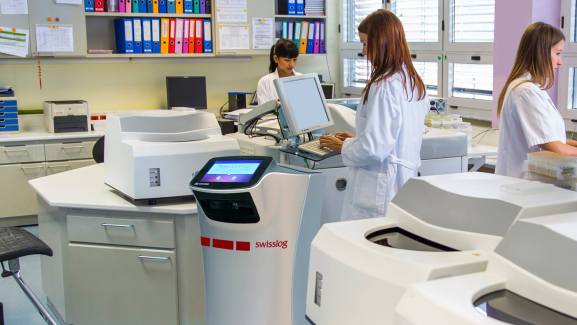
“You know, right now, even at 1% failure rate for a lot of these applications can be a deal breaker. You know, we, especially for self driving cars, as everybody mentioned, you know, the cost of failure is really high. But even in other sort of less critical cases, like in building delivery, you see, you know, if something is spinning around in a circle or not performing its job, it causes people to lose confidence in the system stopped using it. And it’s also you know, during the time that it’s confused, not performing its function. So we essentially built this system as a way to bring robots into a broader range of applications and improve the sort of uptime of the system so that it doesn’t get into these positions where it’s stuck during its operation.”
“Similarly, we have robots that get lost in spaces that are widely variable. So you know, a warehouse that has boxes or pallets that move in and out of the space very frequently. That’s going to confuse the robot because its internal map is static in most cases. And when you have a very large change, the robots going to be confused about its location, and then not be able to proceed with its its normal operation. That’s something that we can help with we essentially will be able to look at the robot’s environment and understand where it is in its space and then update its location. Again, you know, we look at different types of obstacles. You know, a plastic bag is not really an obstacle, we can, you can run through that. But on a LIDAR, it shows up the same way as a pile of bricks.”
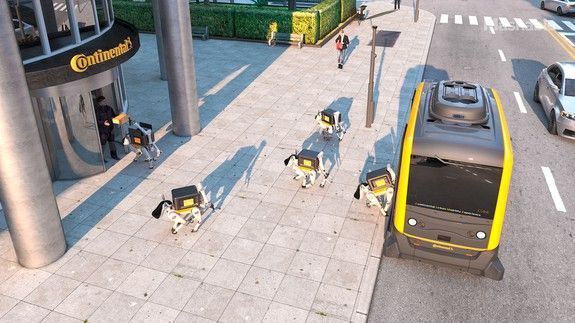
“So by having a human in the loop element, we are able to sort of handle these edge case failures and get robots to perform functions that they wouldn’t otherwise be able to perform and be useful in applications that were maybe too challenging for full autonomy. I think a lot of it has to do with sort of how dangerous is the robot in question. So, you know, for a self driving vehicle, very dangerous, right, we’ve got a half ton of steel, you know, moving at, you know, relatively rapid speeds. This is a dangerous system. “
“On the other hand, in building delivery robots, we’re doing some work in quarantine zones, making deliveries in buildings that allow social distancing to be maintained. We can put needed supplies inside of this delivery robot and send it in a building to the delivery room. So worst case scenario, we might bump into somebody. It’s just inconvenient and might sort of ruin the either the economics of the usefulness of the robot. That would be a good case for these less critical systems. So things like in-building delivery, material handling and logistics spaces. Maybe like a picking arm like a robot arm pulling things out of a box and putting it into a shipping container, or into another robot for in-building delivery.”
“While we try to get as fast as we can, you’re still talking about 30 seconds, maybe before you can really respond to the problem in a meaningful way. So, you know, 30 seconds is an eternity for a self driving vehicle, whereas for an in-building delivery robot, it’s not a big deal. So I think you know, the answer to that it’s pretty application dependent and also system dependent, you know, how dangerous is the system inherently?” [Michael Sayre, CEO of Cognicept]
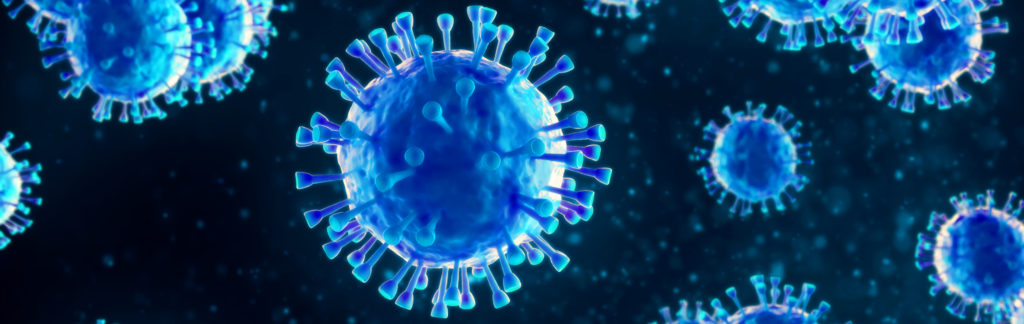
Rex St John, ARM IOT Ecosystem Evangelist presented an unusual COVID-19 response topic. “This isn’t quite a robotics topic. But a few weeks ago, I began working on a project called Rosetta@home. So if you’re not familiar, there’s a lot of researchers that are studying protein folding, and other aspects of biological research. And they don’t have the funding to pay for supercomputer time. So what they do is they they distribute the research workloads to volunteer networks all around the world through this program called boink. So there’s a lot of these programs, there’s SETI@home, and Rosetta@home and Fold@home. And there’s all these people that volunteer their extra compute cycles by downloading this client. And then researchers upload work, jobs to the cloud, and then those jobs are distributed to these home computers.”
“So because I work at arm, we realize that Fold@home and Rosetta@home. are two projects which are used specifically to study protein folding. They did not have arm 64 bit clients available, which means you can’t run them on a Raspberry Pi four, you can’t run them on some of the newer arm hardware. So there are a lot of people in the community that were wanting to help out with Fold@home and Rosetta@home, which are now being used extensively by researchers specifically to study COVID-19. So we put together this community project. And it came together very, very quickly. Because once everybody learned about this opportunity, they jumped on board very quickly. So what happened was these guys from Neocortex, it’s a startup out of San Jose. They jumped on this and their CTO ported all the key libraries from Rosetta@home to arm 64 and then within a couple weeks a week or two actually, we’re now up to 793 arm 64 bit devices that are supporting researchers studying COVID-19 so anybody that wants to help out if you’ve got a Raspberry Pi four or an arm 64 bit device you can install Rosetta@home on your Raspberry Pi four and begin crunching on proteins to help researchers fight back COVID-19. https://www.neocortix.com/coronavirus
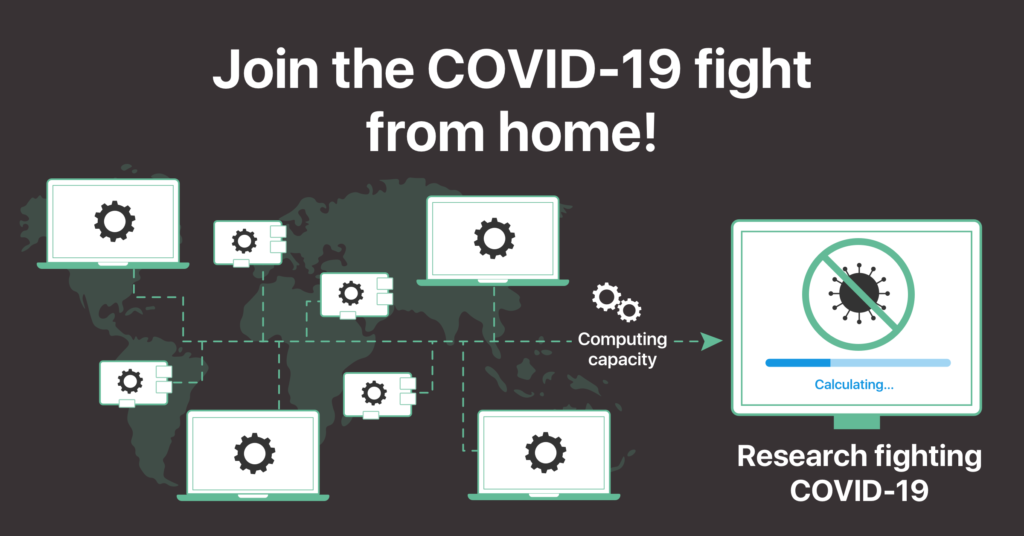
“You can see this is the spike protein right there of COVID-19. COVID-19 uses the spike protein to sort of latch on to the the receptors of human cells and that’s how it kind of invades your body. So they’re doing a lot of work to understand the structure and behavior that spike protein on Rosetta and Fold@home.” [Rex St John ARM IoT Evangelist]
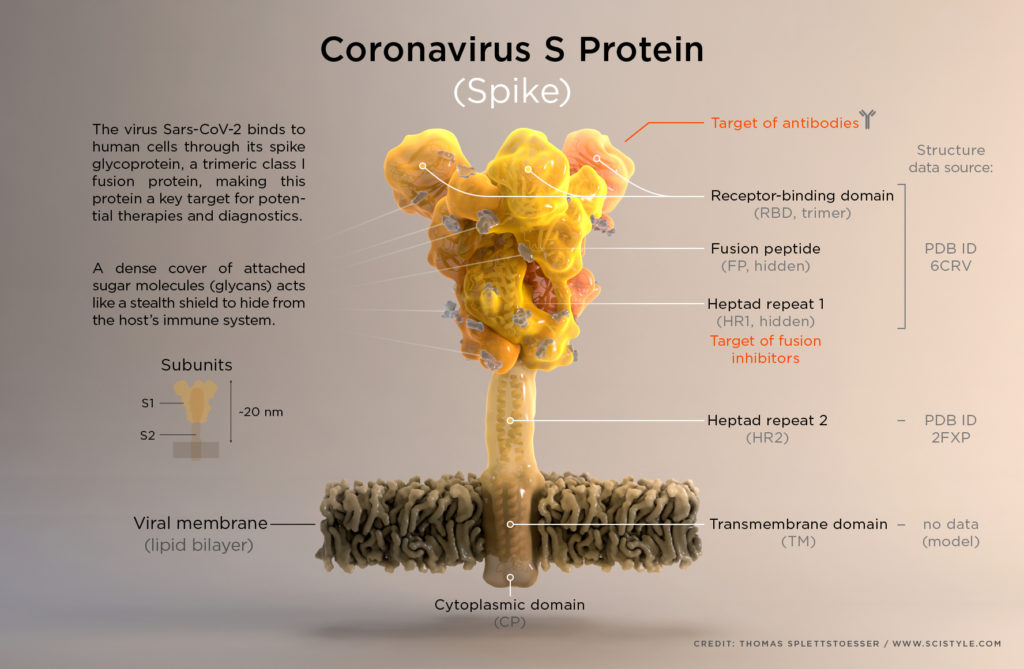
Ken Goldberg, Director of CITRIS People and Robots Initiative said “I do have one thought that I’d like to share that occurred to me this week, which is that I wonder if we’re shifting from what used to be called the ‘High Tech High Touch’ concept from John Naisbitt. He wrote ‘Mega Trends’ about how we were moving as we got toward more high tech, we’d also just as much crave that touch. And I wonder if we’re moving toward a low touch future where we actually will see new value in things that are don’t involve touch.” “It’s been so interesting for me to be you know, to be in the house. I’ve gotten a whole new appreciation for things like washing machines and even vacuum cleaners. They’re incredible these mechanisms that help us do things, that rather than us reaching down and touching everything they basically do it for us.”
“I’ve been thinking about you before this pandemic. There are a lot of things out there like robot vending machines that I was a little skeptical about. And I thought, well, I don’t really see what’s the big advantage, given a choice I’d rather have a human making a hamburger or coffee. But now I’m starting to really think that equation has changed. And I wonder if that’s going to change permanently. In other words, are we actually going to see this a real trend toward things like these robot coffee making baristas and robot burgers like Creator, the company in San Francisco. or Miso robotics is developing fast food making robots. I think it’s time to really reevaluate those trends because I think there is going to be an actual visceral appeal for this kind of low touch future.” [Ken Goldberg CITRIS People and Robots]
There’ll be more next week on Tuesday April 28 so sign up for COVID-19, robots and us with guest speakers focusing on regulations, risks and opportunities:
- Chelsey Colbert, Policy Council at The Future of Privacy Forum
- Michael Froomkin, Laurie Silvers and Mitchell Rubenstein Distinguished Professor of Law
- Ryan Calo, Lane Powell & D. Wayne Gittinger Endowed Professorship of Law
- Sue Keay, Research Director at CSIRO Data 61 and Aust National COVID response
- Robin Murphy, Rescue Robotics Expert and Raytheon Professor at Texas A&M University
- Thomas Low, Director of Robotics SRI International
- Ken Goldberg, Director of CITRIS People and Robots Initiative
- Andra Keay, Director of Silicon Valley Robotics and founder of Women in Robotics
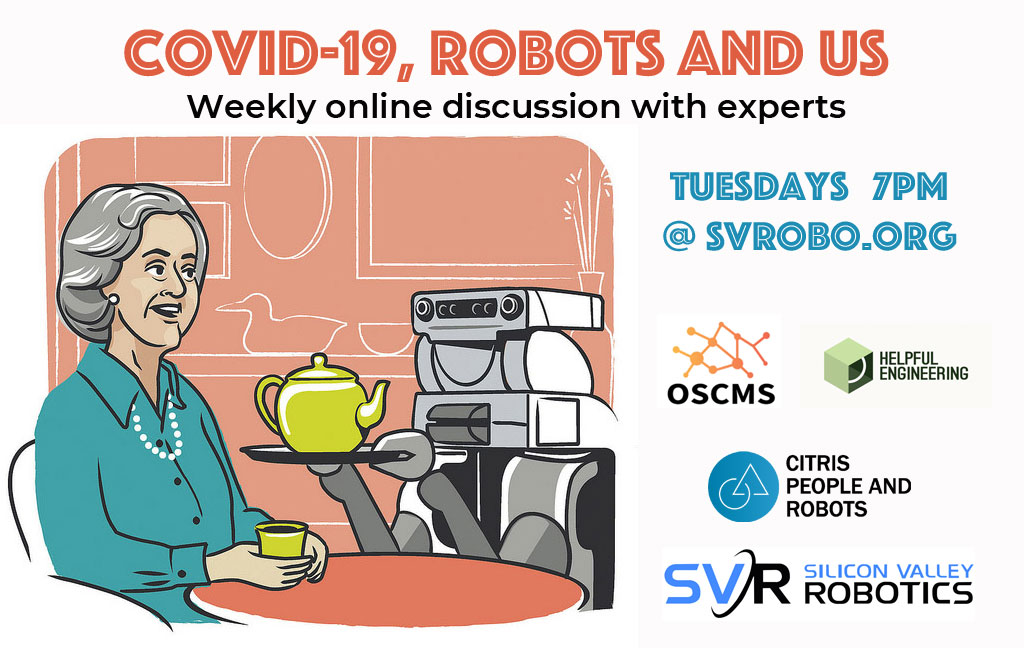