When Cars Think for Us: Autonomous Vehicles and the Role of Energy Storage
Talking PACK EXPO with OMRON
Talking PACK EXPO with SCHMALZ
Drywall installer Robot
Japan’s new Humanoid Robot built by the Japanese Japan’s Advanced Industrial Science and Technology Institute is able to install drywall:
Source : https://www.aist.go.jp/index_en.html
The post Drywall installer Robot appeared first on Roboticmagazine.
#270: A Mathematical Approach To Robot Ethics, with Robert Williamson
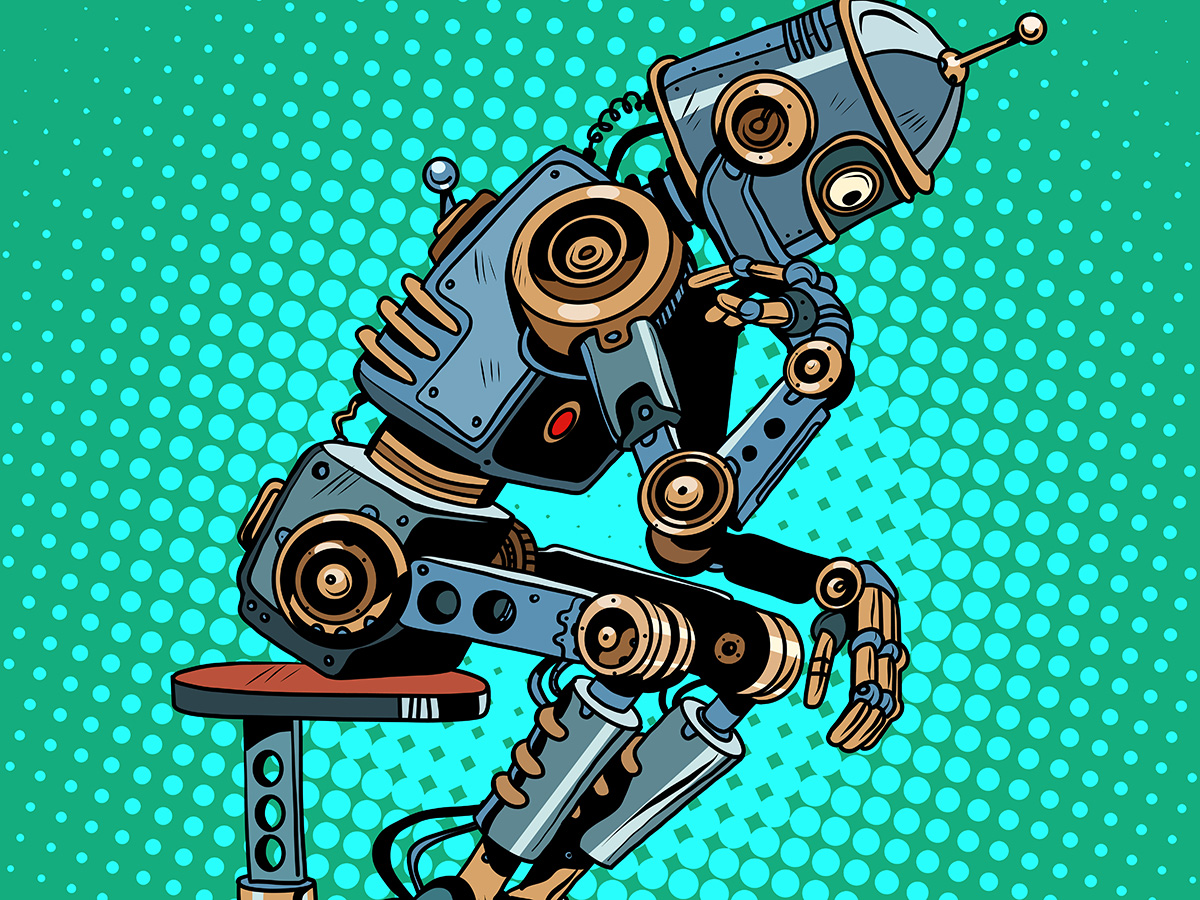
In this episode, Audrow Nash interviews Robert Williamson, a Professor at the Australian National University, who speaks about a mathematical approach to ethics. This approach can get us started implementing robots that behave ethically. Williamson goes through his logical derivation of a mathematical formulation of ethics and then talks about the cost of fairness. In making his derivation, he relates bureaucracy to an algorithm. He wraps up by talking about how to work ethically.
Robert Williamson
Robert (Bob) Williamson is a professor in the research school of computer science at Australian National University. Until recently he was the chief scientist of DATA61, where he continues as a distinguished researcher. He served as scientific director and (briefly) CEO of NICTA, and lead its machine learning research group. His research is focussed on machine learning. He is the lead author of the ACOLA report Technology and Australia’s Future. He obtained his PhD in electrical engineering from the university of Queensland in 1990. He is a fellow of the Australian Academy of Science.
Links
- Download mp3 (14.4 MB)
- Robert Williamson’s homepage
- Subscribe to Robots using iTunes
- Subscribe to Robots using RSS
- Support us on Patreon
#IROS2018 live coverage
The 2018 IEEE/RSJ International Conference on Intelligent Robots and Systems (#IROS2018) will be held for the first time in Spain in the lively capital city of Madrid from 1 to 5 October. This year’s motto is “Towards a Robotic Society”.
Check here over the week for videos and tweets.
And if you have an article published at IROS that you would like share with the world, just send an image and a short summary (a couple paragraphs) to sabine.hauert@robohub.org Read More
The robot eye with an all-round field of view
The impact of AI on work: implications for individuals, communities, and societies
By Jessica Montgomery, Senior Policy Adviser
Advances in AI technologies are contributing to new products and services across industries – from robotic surgery to debt collection – and offer many potential benefits for economies, societies, and individuals.
With this potential, come questions about the impact of AI technologies on work and working life, and renewed public and policy debates about automation and the future of work.
Building on the insights from the Royal Society’s Machine Learning study, a new evidence synthesis by the Royal Society and the British Academy draws on research across disciplines to consider how AI might affect work. It brings together key insights from current research and policy debates – from economists, historians, sociologists, data scientists, law and management specialists, and others – about the impact of AI on work, with the aim of helping policymakers to prepare for these.
Current understandings about the impact of AI on work
While much of the public and policy debate about AI and work has tended to oscillate between fears of the ‘end of work’ and reassurances that little will change in terms of overall employment, evidence suggests that neither of these extremes is likely. However, there is consensus that AI will have a disruptive effect on work, with some jobs being lost, others being created, and others changing.
Over the last five years, there have been many projections of the numbers of jobs likely to be lost, gained, or changed by AI technologies, with varying outcomes and using various timescales for analysis.
Most recently, a consensus has begun to emerge from such studies that 10-30% of jobs in the UK are highly automatable. Many new jobs will also be created. However, there remain large uncertainties about the likely new technologies and their precise relationship to tasks. Consequently, it is difficult to make predictions about which jobs will see a fall in demand and the scale of new job creation.
Implications for individuals, communities, and societies
Despite this uncertainty, evidence from previous waves of technological change – including the Industrial Revolution and the advent of computing – can provide evidence and insights to inform policy debates today.
Studies of the history of technological change demonstrate that, in the longer term, technologies contribute to increases in population-level productivity, employment, and economic wealth. However, such studies also show that these population-level benefits take time to emerge, and there can be periods in the interim where parts of the population experience significant disbenefits. In the context of the British Industrial Revolution, for example, studies show that wages stagnated for a period despite output per worker increasing. In the same period, technological changes enabled or interacted with large population movements from land to cities, ways of working at home and in factories changed, and there were changes to the distribution of income and wealth across demographics.
Evidence from historical and contemporary studies indicates that technology-enabled changes to work tend to affect lower-paid and lower-qualified workers more than others. For example, in recent years, technology has contributed to a form of job polarisation that has favoured higher-educated workers, while reducing the number of middle-income jobs, and increasing competition for non-routine manual labour.
This type of evidence suggests there are likely to be significant transitional effects as AI technologies begin to play a bigger role in the workplace, which cause disruption for some people or places. One of the greatest challenges raised by AI is therefore a potential widening of inequality.
The role of technology in changing patterns of work and employment
The extent to which technological advances are – overall – a substitute for human workers depends on a balance of forces. Productivity growth, the number of jobs created as a result of growing demand, movement of workers to different roles, and emergence of new jobs linked to the new technological landscape all influence the overall economic impact of automation by AI technologies. Concentration of market power can also play a role in shaping labour’s income share, competition, and productivity.
So, while technology is often the catalyst for revisiting concerns about automation and work, and may play a leading role in framing public and policy debates, it is not a unique or overwhelming force. Non-technological factors – including political, economic, and cultural elements – will contribute to shaping the impact of AI on work and working life.
Policy responses and ‘no regrets’ steps
In the face of significant uncertainties about the future of work, what role can policymakers play in contributing to the careful stewardship of AI technologies?
At workshops held by the Royal Society and British Academy, participants offered various suggestions for policy responses to explore, focused around:
- Ensuring that the workers of the future are equipped with the education and skills they will need to be ‘digital citizens’ (for example, through teaching key concepts in AI at primary school-level, as recommended in the Society’s Machine Learning report);
- Addressing concerns over the changing nature of working life, for example with respect to income security and the gig economy, and in tackling potential biases from algorithmic systems at work;
- Meeting the likely demand for re-training for displaced workers through new approaches to training and development; and
- Introducing measures to share the benefits of AI across communities, including by supporting local economic growth.
While it is not yet clear how potential changes to the world of work might look, active consideration is needed now about how society can ensure that the increased use of AI is not accompanied by increased inequality. At this stage, it will be important to take ‘no regrets’ steps, which allow policy responses to adapt as new implications emerge, and which offer benefits in a range of future scenarios. One example of such a measure would be in building a skills-base that is prepared to make use of new AI technologies.
Through the varying estimates of jobs lost or created, tasks automated, or productivity increases, there remains a clear message: AI technologies will have a significant impact on work, and their effects will be felt across the economy. Who benefits from AI-enabled changes to the world of work will be influenced by the policies, structures, and institutions in place. Understanding who will be most affected, how the benefits are likely to be distributed, and where the opportunities for growth lie will be key to designing the most effective interventions to ensure that the benefits of this technology are broadly shared.
Simulations are the key to intelligent robots
I read an article entitled Games Hold the Key to Teaching Artificial Intelligent Systems, by Danny Vena, in which the author states that computer games like Minecraft, Civilization, and Grand Theft Auto have been used to train intelligent systems to perform better in visual learning, understand language, and collaborate with humans. The author concludes that games are going to be a key element in the field of artificial intelligence in the near future. And he is almost right.
In my opinion, the article only touches the surface of artificial intelligence by talking about games. Games have been a good starting point for the generation of intelligent systems that outperform humans, but going deeper into the realm of robots that are useful in human environments will require something more complex than games. And I’m talking about simulations.
Using games to bootstrap intelligence
The idea behind beating humans at games has been in artificial intelligence since its birth. Initially, researchers created programs to beat humans at Tic Tac Toe and Chess (like, for example, IBM’s DeepBlue). However, those games’ intelligence was programmed from scratch by human minds. There were people writing the code that decided which move should be the next one. However, that manual approach to generate intelligence reached a limit: intelligence is so complex that we realized that it may be too difficult to manually write a program that emulates it.
Then, a new idea was born: what if we create a system that learns by itself? In that case, the engineers will only have to program the learning structures and set the proper environment to allow intelligence to bootstrap by itself.

AlphaGo beats Lee Sedol
(photo credit: intheshortestrun)
The results of that idea are programs that learn to play the games better than anyone in the world, even if nobody explains to the program how to play in the first place. For example, Google’s DeepMind company created AlphaGo Zero program uses that approach. The program was able to beat the best players of Go in the world. The company used the same approach to create programs that learnt to play Atari games, starting from zero knowledge. Recently, OpenAI used this approach for their bot program that beats pro players of the Dota 2 game. By the way, if you want to reproduce the results of the Atari games, OpenAI released the OpenAI Gym, containing all the code to start training your system with Atari games, and compare the performance against other people.
What I took from those results is that the idea of making an intelligent system generate intelligence by itself is a good approach, and that the algorithms used for teaching can be used for making robots learn about their space (I’m not so optimistic about the way to encode the knowledge and to set the learning environment and stages, but that is another discussion).
From games to simulations
OpenAI wanted to go further. Instead of using games to generate programs that can play a game, they applied the same idea to make a robot do something useful: learn to manipulate a cube on its hand. In this case, instead of using a game, they used a simulation of the robot. The simulation was used to emulate the robot and its environment as if it were a real one. Then, they allowed the algorithm to control the simulated robot and make the robot learn about the task to solve by using domain randomization. After many trials, the simulated robot was able to manipulate the block in the expected way. But that was not all! At the end of the article, the authors successfully transferred the learned control program of the simulated robot to a real robot, which performed in a way similar to the simulated one. Except it was real.

Simulated Hand OpenAI Manipulation Experiment (image credit: OpenAI)

Real Hand OpenAI Manipulation Experiment (photo credit:OpenAI)
A similar approach was applied by OpenAI to a Fetch robot trained to grasp a spam box off of a table filled with different objects. The robot was trained in simulation and it was successfully transferred to the real robot.

OpenAI teaches Fetch robot to grasp from table using simulations (photo credit: OpenAI)
We are getting close to the holy grail in robotics, a complete self-learning system!
Training robots
However, in their experiments, engineers from OpenAI discovered that training for robots is a lot more complex than training algorithms for games. Meanwhile, in games, the intelligent system has a very limited list of actions and perceptions available; robots face a huge and continuous spectrum in both domains, actions and perceptions. We can say that the options are infinite.
That increase in the number of options diminishes the usefulness of the algorithms used for RL. Usually, the way to deal with the problem is with some artificial tricks, like discarding some of the information completely or discretizing the data values artificially, reducing the options to only a few.
OpenAI engineers found that even if the robots were trained in simulations, their approach could not scale to more complex tasks.
Massive data vs. complex learning algorithms
As Andrew Ng indicated, and as an engineer from OpenAI personally indicated to me based on his results, massive data with simple learning algorithms wins over complicated algorithms with a small amount of data. This means that it is not a good idea to try to focus on getting more complex learning algorithms. Instead, the best approach for reaching intelligent robotics would be to use simple learning algorithms trained with massive amounts of data (which makes sense if we observe our own brain: a massive amount of neural networks trained over many years).
Google has always known that. Hence, in order to obtain massive amounts of data to train their robots, Google created a real life system with real robots, training all day long in a large space. Even if it is a clever idea, we can all see that this is not practical in any sense for any kind of robot and application (breaking robots, limited to execution in real time, a limited amount of robots, a limited amount of environments, and so on…).

Google robots training for grasping
That leads us to the same solution again: to use simulations. By using simulations, we can put any robot in any situation and train them there. Also, we can have virtually an infinite number of them training in parallel, and generate massive amounts of data in record time.
Even if that approach looks very clear right now, it was not three years ago when we created our company, The Construct, around robot simulations in the cloud. I remember exhibiting at the Innorobo 2015 exhibition and finding, after extensive interviews among all the other exhibitors, that only two among them were using simulations for their work. Furthermore, roboticists considered simulations to be something nasty to be avoided at all cost, since nothing can compare with the real robot (check here for a post I wrote about it at the time).
Thankfully, the situation has changed since then. Now, using simulations for training real robots is starting to become the way.
Transferring to real robots
We all know that it is one thing to get a solution with the simulation and another for that solution to work on the real robot. Having something done by the robot in the simulation doesn’t imply that it will work the same way on the real robot. Why is that?
Well, there is something called the reality gap. We can define the reality gap as the difference between the simulation of a situation and the real-life situation. Since it is impossible for us to simulate something to a perfect degree, there will always be differences between simulation and reality. If the difference is big enough, it may happen that the results obtained in the simulator are not relevant at all. That is, you have a big reality gap, and what applies in the simulation does not apply to the real world.
That problem of the reality gap is one of the main arguments used to discard simulators for robotics. And in my opinion, the path to follow is not to discard the simulators and find something else, but instead to find solutions to cross that reality gap. As for solutions, I believe we have two options:
1. Create more accurate simulators. That is on its way. We can see efforts in this direction. Some simulators concentrate on better physics (Mujoco); others on a closer look at reality (Unreal or Unity-based simulators, like Carla or AirSim). We can expect that as computer power continues to increase, and cloud systems become more accessible, the accuracy of simulations is going to keep increasing in both senses, physics and looks.
2. Build better ways to cross the reality gap. In its original work, Noise and the reality gap, Jakobi (the person who identified the problem of the reality gap) indicated that one of the first solutions is to make a simulation independent of the reality gap. His idea was to introduce noise in those variables that are not relevant to the task. The modern version of that noise introduction is the concept of domain randomization, as described in the paper Domain Randomization for Transferring Deep Neural Networks from Simulation to the Real World.
Domain randomization basically consists of performing the training of the robot in a simulated environment where its non-relevant-to-the-task features are changed randomly, like the colors of the elements, the light conditions, the relative position to other elements, etc.
The goal is to make the trained algorithm be unaffected by those elements in the scene that provide no information to the task at hand, but which may confuse it (because the algorithm doesn’t know which parts are the relevant ones to the task). I can see domain randomization as a way to tell the algorithm where to focus its attention, in terms of the huge flow of data that it is receiving.
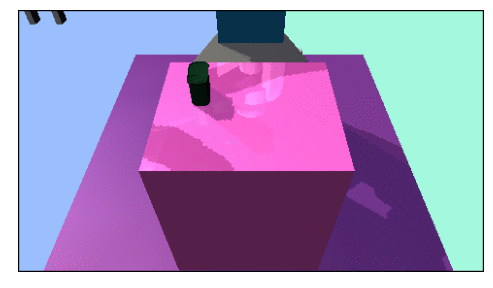
Domain randomization applied by OpenAI to train a Fetch robot in simulation (photo credit: OpenAI)
In more recent works, the OpenAI team has released a very interesting paper that improves domain randomization. They introduce the concept of dynamics randomization. In this case, it is not the environment that is changing in the simulation, but the properties of the robot (like its mass, distance between grippers, etc.). The paper is Sim-to-real transfer of robotic control with dynamics randomization. That is the approach that OpenAI engineers took to successfully achieve the manipulation robot.
Some software that goes on the line
What follows is a list of software that allows the training of robots in simulations. I’m not including just robotics simulators (like Gazebo, Webots, and V-Rep) because they are just that, robot simulators in the general sense. The software listed here goes one step beyond that and provides a more complete solutions for doing the training in simulations. Of course, I have discarded the system used by OpenAI (which is Mujoco) because it requires the building of your own development environment.
Carla
Carla is an open source simulator for self-driving cars based on Unreal Engine. It has recently included a ROS bridge.
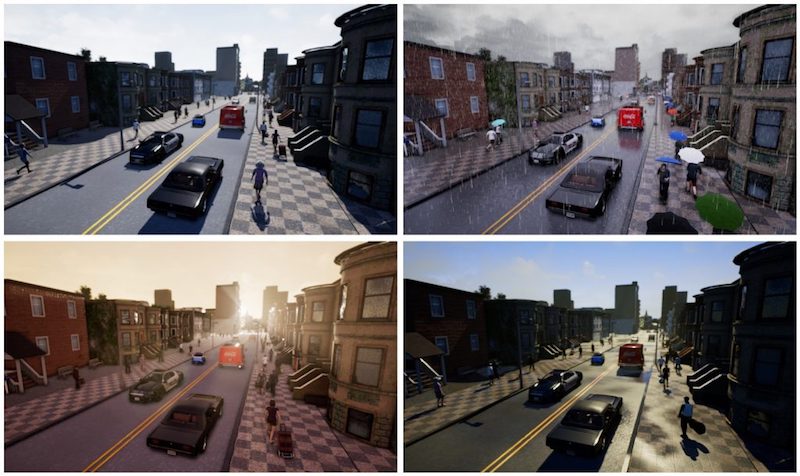
Carla simulator (photo credit Carla)
Microsoft Airsim
Microsoft Airsim drones simulator follows a similar approach to Carla, but for drones. Recently, they updated the simulator to also include self-driving cars.
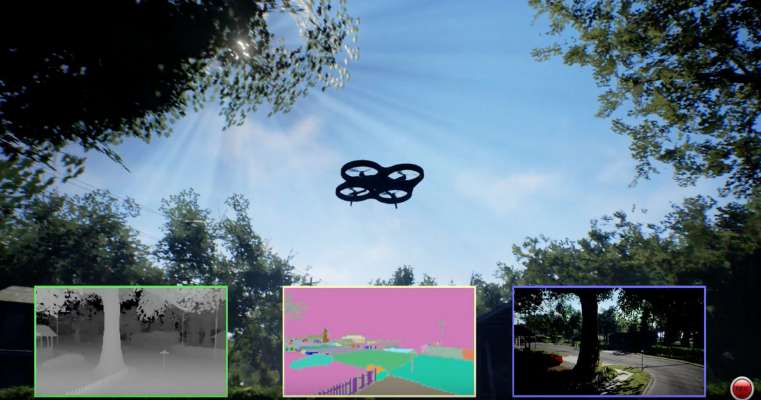
Airsim (photo credit: Microsoft)
Nvidia Isaac
Nvidia Isaac aims to be a complete solution for training robots on simulations and then transferring to real robots. There is still nothing available, but they are working on it.
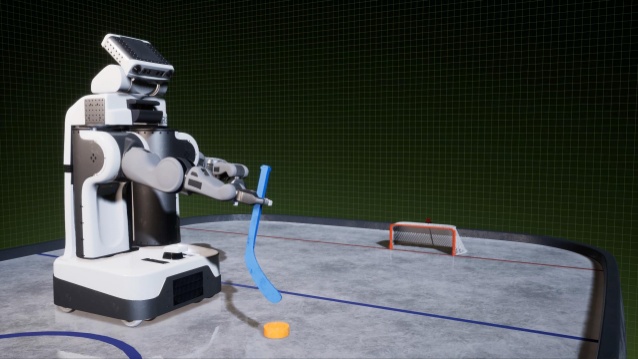
Isaac (photo credit: Nvidia)
ROS Development Studio
The ROS Development Studio is the development environment that our company created, and it has been conceived from the beginning to simulate and train any ROS-based robot, requiring nothing to be installed in your computer (cloud-based). Simulations for the robots are already provided with all the ROS controllers up and running, as well as the machine learning tools. It includes a system of Gym cloud computers for the parallel training of robots on an infinite number of computers.

ROS Development Studio showing an industrial environment
Here is a video showing a simple example of training two cartpoles in parallel using the Gym computers inside the ROS Development Studio:
(Readers, if you know other software like this that I can add, let me know.)
Conclusion
Making all those deep neural networks learn in a training simulation is the way to go, and as we may see in the future, this is just the tip of the iceberg. My personal opinion is that intelligence is yet more embodied than current AI approaches admit: you cannot have intelligence without a body. Hence, I believe that the use of simulated embodiments will be even higher in the future. We’ll see.
Memory-jogging robot to keep people sharp in ‘smart’ retirement homes
by Steve Gillman
Almost a fifth of the European population are over 65 years old, but while quality of life for this age bracket is better than ever before, many will at some point suffer from a decline in their mental abilities.
Without adequate care, cognitive abilities can decline quicker, yet with the right support people can live longer, healthier and more independent lives. Researchers working on a project called ENRICHME have attempted to address this by developing a robotic assistant to help improve mental acuity.
‘One of the big problems of mild cognitive impairment is temporary memory loss,’ said Dr Nicola Bellotto from the School of Computer Science at the University of Lincoln in the UK and one of the principal investigators of ENRICHME.
‘The goal of the project was to assist and monitor people with cognitive impairments and offer basic interactions to help a person maintain their cognitive abilities for longer.’
The robot moves around a home providing reminders about medication as well as offering regular physical and mental exercises – it can even keep tabs on items that are easily misplaced.
A trial was conducted with the robot in three retirement homes in England, Greece and Poland. At each location the robot helped one or more residents, and was linked to sensors placed throughout the building to track the movements and activities of those taking part.
‘All this information was used by the robot,’ said Dr Bellotto. ‘If a person was in the bedroom or kitchen the robot could rely on the sensors to know where the person is.’
The robot was also kitted out with a thermal camera so it could measure the temperature of a person in real time, allowing it to estimate the levels of their respiration and heartbeat. This could reveal if someone was experiencing high levels of stress related to a particular activity and inform the robot to act accordingly.
This approach is based around a principle called ambient assisted living, which combines technology and human carers to improve support for older people. In ENRICHME’s case, Dr Bellotto said their robots could be operated by healthcare professionals to provide tailored care for elderly patients.
The users involved in the trials showed high level of engagement with the robot they were living with – even naming it Alfie in one case – and also provided good feedback, said Dr Bellotto. But he added that it is still a few years away from being rolled out to the wider public.
‘Some of the challenges we had were how to approach the right person in these different environments because sometimes a person lives with multiple people, or because the rooms are small and cluttered and it is simply not possible for the robot to move safely from one point to another,’ he said.
Dr Bellotto and his team are now applying for funding for new projects to solve the remaining technical problems, which hopefully one day will help them take the robot one step closer to commercialisation.
This type of solution would help increase people’s physical and psychological wellbeing, which could help to reduce public spending on care for older people. In 2016 the total cost of ageing in the EU was 25% of GDP, and this figure is expected to rise in the coming decades.
Ageing populations
‘One of the big challenges we have in Europe is the high number of elderly people that the public health system has to deal with,’ said Professor María Inés Torres, a computer scientist from the University of the Basque Country in Spain.
Older people can fall into bad habits for a variety of reasons, such as being unable to go for walks and cook healthy meals because of physical limitations. The loss of a loved one can also lead to reduced socialising, exercising or eating well. These unhealthy habits are all exacerbated by depression caused by loneliness, and with 32% of people aged over 65 living alone in Europe, this is a significant challenge to overcome.
‘If you are able to decrease the correlation between depression and age, so keeping people engaged with life in general and social activities, these people aren’t going to visit the doctor as much,’ said Prof. Torres, who is also the coordinator of EMPATHIC, a project that has developed a virtual coach to help assist elderly people to live independently.
The coach will be available on smart devices such as phones or tablets and is focused on engaging with someone to help them keep up the healthier habits they may have had in the past.
‘The main goal is that the user reflects a little bit and then they can agree to try something,’ said Prof. Torres.
For instance, the coach may ask users if they would like go to the local market to prepare their favourite dinner and then turn it into a social activity by inviting a friend to come along. This type of approach addresses the three key areas that cause older people’s health to deteriorate, said Prof. Torres, which are poor nutrition, physical activity and loneliness.
For the coach to be effective the researchers have to build a personal profile for each user, as every person is different and requires specific suggestions relevant to them. They do this by building a database for each person over time and combining it with dialogue designed around the user’s culture.
The researchers are testing the virtual coach on smart devices with 250 older people in three areas – Spain, France and Norway – who are already providing feedback on what works and what doesn’t, which will increase the chances of the virtual coach actually being used.
By the end of the project in 2020, the researchers hope to have a prototype ready for the market, but Prof. Torres insisted that it will not replace healthcare professionals. Instead she sees the smart coach as another tool to help older people live a more independent life – and in doing so reduce the pressure on public healthcare systems.
The research in this article was funded by the EU. If you liked this article, please consider sharing it on social media.