Robotic arms and temporary motorisation – the next generation of wheelchairs
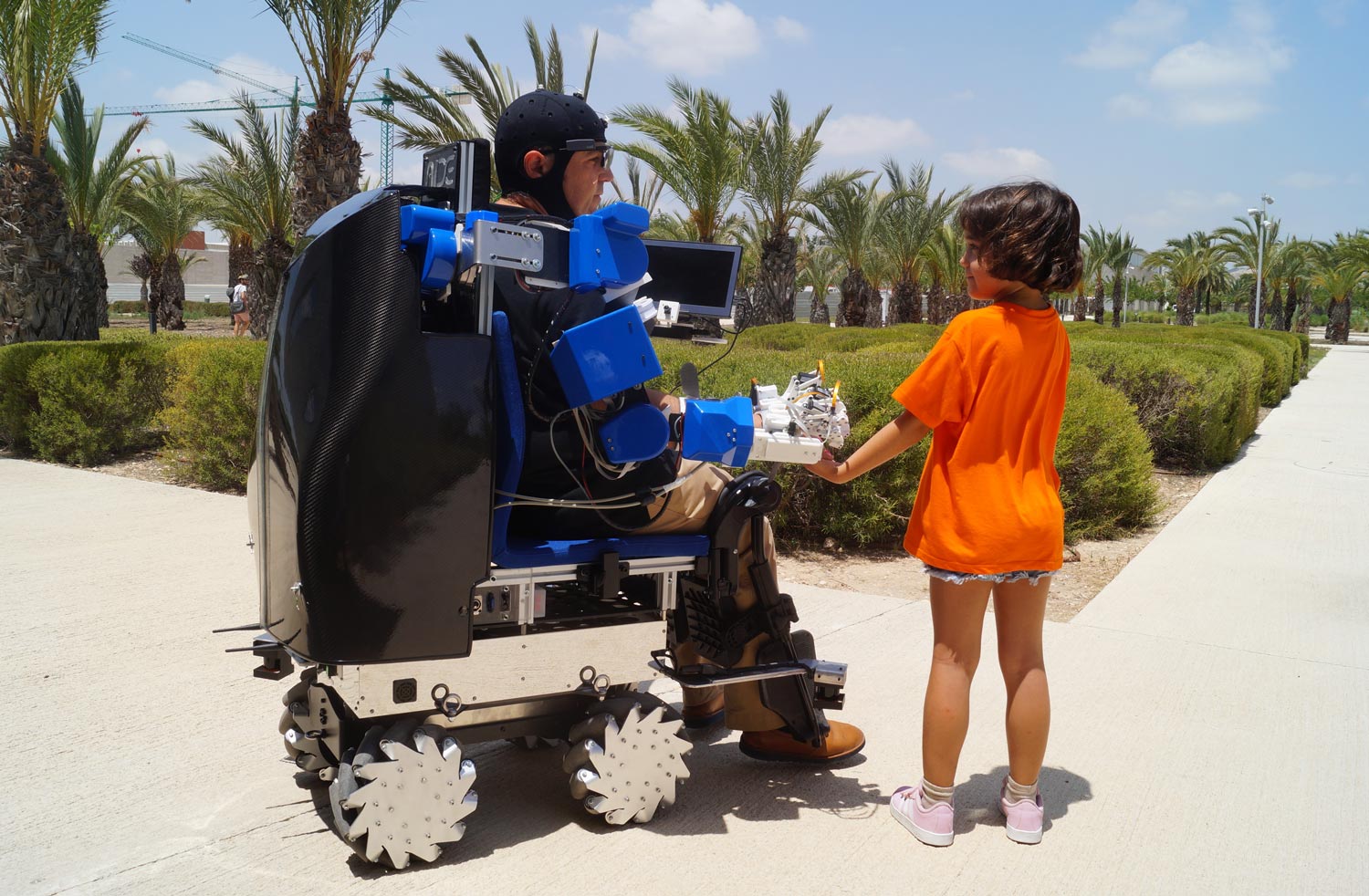
by Julianna Photopoulos
Next-generation wheelchairs could incorporate brain-controlled robotic arms and rentable add-on motors in order to help people with disabilities more easily carry out daily tasks or get around a city.
Professor Nicolás García-Aracil from the Universidad Miguel Hernández (UMH) in Elche, Spain, has developed an automated wheelchair with an exoskeleton robotic arm to use at home, as part of a project called AIDE.
It uses artificial intelligence to extract relevant information from the user, such as their behaviour, intentions and emotional state, and also analyses its environmental surroundings, he says.
The system, which is based on an arm exoskeleton attached to a robotised wheelchair, is designed to help people living with various degrees and forms of disabilities carry out daily functions such as eating, drinking, and washing up, on their own and at home. While the user sits in the wheelchair, they wear the robotised arm to help them grasp objects and bring them close — or as the whole system is connected to the home automation system they can ask the wheelchair to move in a specific direction or go into a particular room.
Its mechanical wheels are made to move in narrow spaces, ideal for home-use, and the system can control the environment remotely – for example, switching lights on and off, using the television or making and answering phone calls. What’s more, it can anticipate the person’s needs.
‘We can train artificially intelligent algorithms to predict what the user wants to do,’ said Prof. García-Aracil. ‘Maybe the user is in the kitchen and wants a drink. The system provides their options (on a monitor) so they can control the exoskeleton to raise the glass and drink.’
Multimodal system
The technology isn’t simple. As well as the exoskeleton robotic arm attached to the robotic wheelchair, the chair has a small monitor and uses various sensors, including two cameras to recognise the environment, voice control, eye-tracking glasses to recognise objects, and sensors that capture brain activity, eye movements and signals from muscles.
Depending on each person’s needs and disabilities, the multiple devices are used accordingly. For example, someone with a severe disability such as a cervical spinal cord injury, who wouldn’t otherwise be able to use voice control, could use the brain activity and eye movement sensors combined.
The user wears a cap on their head, filled with electrodes, to record the brain’s activity which controls the exoskeleton hand’s movement, explains Prof. García-Aracil. So when the user sees themself closing their hand onto an object for example, the exoskeleton arm actually does it for them. This technology is called brain-neural-computer interaction (BNCI), where brain — as well as muscle — activity can be recorded and used to interact with an electronic device.
But the system can sometimes make mistakes so there is an abort signal, says Prof. García-Aracil. ‘We use the horizontal movement of the eye, so when you move your eyes to the right you trigger an action, but when you move your eyes to the left you abort that action,’ he explains.
The AIDE prototype was successfully tested last year by 17 people with disabilities including acquired brain injury (ABI), multiple sclerosis (MS), and spinal cord injury (SCI), at the Cedar Foundation in Belfast, Northern Ireland. Its use was also demonstrated at UMH in Elche, with the user asking to be taken to the cafeteria, then asking for a drink, and drinking it with the help of the exoskeletal arm.
Now more work needs to be carried out to make the system easier to use, cheaper and ready for the market, says Prof. García-Aracil.
But it’s not just new high-tech wheelchairs that can increase the functionality for users. Researchers on the FreeWheel project are developing a way of adding motorised units to existing wheelchairs to improve their utility in urban areas.
‘Different settings have different challenges,’ said project coordinator Ilaria Schiavi at IRIS SRL in Torino, Italy. For example, someone with a wheelchair may struggle to go uphill or downhill without any physical assistance whilst outdoors. But this system could allow people using wheelchairs to have an automated wheelchair experience regardless of whether they are indoors or outdoors, she says.
Rentable
The motorised units would attach to manual wheelchairs people already have in order to help them move around more easily and independently, Schiavi explains. These could either be rented for short periods of time and tailored according to the location — an indoor or outdoor environment — or bought, in which case would be completely personalised to the individual.
The researchers are also developing an app for the user which would include services such as ordering a bespoke device to connect the wheelchair and the unit, booking the unit, controlling it, and planning a journey within urban areas for shopping or sightseeing.
‘You have mobility apps that allow you to book cars, for example. Our app will allow the owner of a wheelchair to firstly subscribe to the service, which would include buying a customised interface to use between their own wheelchair and the motorising unit they have booked,’ said Schiavi.
‘A simple customised interface will allow wheelchair users to motorise their exact device, as it is used by them, at a reasonable cost.’
Customisation is made possible through additive manufacturing (AM) technologies, she says. AM technologies build 3D objects by adding materials, such as metal or plastic, layer-by-layer.
Schiavi and her colleagues are exploring various uses for the motorised units and next year, the team plans to test this system with mobility-impaired people in both Greece and Italy. They hope that, once developed, they will be made available like city bicycles in public spaces such as tourist attractions or shopping centres.
The research in this article was funded by the EU.
Giving robots a better feel for object manipulation
A new “particle simulator” developed by MIT researchers improves robots’ abilities to mold materials into simulated target shapes and interact with solid objects and liquids. This could give robots a refined touch for industrial applications or for personal robotics— such as shaping clay or rolling sticky sushi rice.
Courtesy of the researchers
By Rob Matheson
A new learning system developed by MIT researchers improves robots’ abilities to mold materials into target shapes and make predictions about interacting with solid objects and liquids. The system, known as a learning-based particle simulator, could give industrial robots a more refined touch — and it may have fun applications in personal robotics, such as modelling clay shapes or rolling sticky rice for sushi.
In robotic planning, physical simulators are models that capture how different materials respond to force. Robots are “trained” using the models, to predict the outcomes of their interactions with objects, such as pushing a solid box or poking deformable clay. But traditional learning-based simulators mainly focus on rigid objects and are unable to handle fluids or softer objects. Some more accurate physics-based simulators can handle diverse materials, but rely heavily on approximation techniques that introduce errors when robots interact with objects in the real world.
In a paper being presented at the International Conference on Learning Representations in May, the researchers describe a new model that learns to capture how small portions of different materials — “particles” — interact when they’re poked and prodded. The model directly learns from data in cases where the underlying physics of the movements are uncertain or unknown. Robots can then use the model as a guide to predict how liquids, as well as rigid and deformable materials, will react to the force of its touch. As the robot handles the objects, the model also helps to further refine the robot’s control.
In experiments, a robotic hand with two fingers, called “RiceGrip,” accurately shaped a deformable foam to a desired configuration — such as a “T” shape — that serves as a proxy for sushi rice. In short, the researchers’ model serves as a type of “intuitive physics” brain that robots can leverage to reconstruct three-dimensional objects somewhat similarly to how humans do.
“Humans have an intuitive physics model in our heads, where we can imagine how an object will behave if we push or squeeze it. Based on this intuitive model, humans can accomplish amazing manipulation tasks that are far beyond the reach of current robots,” says first author Yunzhu Li, a graduate student in the Computer Science and Artificial Intelligence Laboratory (CSAIL). “We want to build this type of intuitive model for robots to enable them to do what humans can do.”
“When children are 5 months old, they already have different expectations for solids and liquids,” adds co-author Jiajun Wu, a CSAIL graduate student. “That’s something we know at an early age, so maybe that’s something we should try to model for robots.”
Joining Li and Wu on the paper are: Russ Tedrake, a CSAIL researcher and a professor in the Department of Electrical Engineering and Computer Science (EECS); Joshua Tenenbaum, a professor in the Department of Brain and Cognitive Sciences; and Antonio Torralba, a professor in EECS and director of the MIT-IBM Watson AI Lab.
Dynamic graphs
A key innovation behind the model, called the “particle interaction network” (DPI-Nets), was creating dynamic interaction graphs, which consist of thousands of nodes and edges that can capture complex behaviors of so-called particles. In the graphs, each node represents a particle. Neighboring nodes are connected with each other using directed edges, which represent the interaction passing from one particle to the other. In the simulator, particles are hundreds of small spheres combined to make up some liquid or a deformable object.
The graphs are constructed as the basis for a machine-learning system called a graph neural network. In training, the model over time learns how particles in different materials react and reshape. It does so by implicitly calculating various properties for each particle — such as its mass and elasticity — to predict if and where the particle will move in the graph when perturbed.
The model then leverages a “propagation” technique, which instantaneously spreads a signal throughout the graph. The researchers customized the technique for each type of material — rigid, deformable, and liquid — to shoot a signal that predicts particles positions at certain incremental time steps. At each step, it moves and reconnects particles, if needed.
For example, if a solid box is pushed, perturbed particles will be moved forward. Because all particles inside the box are rigidly connected with each other, every other particle in the object moves the same calculated distance, rotation, and any other dimension. Particle connections remain intact and the box moves as a single unit. But if an area of deformable foam is indented, the effect will be different. Perturbed particles move forward a lot, surrounding particles move forward only slightly, and particles farther away won’t move at all. With liquids being sloshed around in a cup, particles may completely jump from one end of the graph to the other. The graph must learn to predict where and how much all affected particles move, which is computationally complex.
Shaping and adapting
In their paper, the researchers demonstrate the model by tasking the two-fingered RiceGrip robot with clamping target shapes out of deformable foam. The robot first uses a depth-sensing camera and object-recognition techniques to identify the foam. The researchers randomly select particles inside the perceived shape to initialize the position of the particles. Then, the model adds edges between particles and reconstructs the foam into a dynamic graph customized for deformable materials.
Because of the learned simulations, the robot already has a good idea of how each touch, given a certain amount of force, will affect each of the particles in the graph. As the robot starts indenting the foam, it iteratively matches the real-world position of the particles to the targeted position of the particles. Whenever the particles don’t align, it sends an error signal to the model. That signal tweaks the model to better match the real-world physics of the material.
Next, the researchers aim to improve the model to help robots better predict interactions with partially observable scenarios, such as knowing how a pile of boxes will move when pushed, even if only the boxes at the surface are visible and most of the other boxes are hidden.
The researchers are also exploring ways to combine the model with an end-to-end perception module by operating directly on images. This will be a joint project with Dan Yamins’s group; Yamin recently completed his postdoc at MIT and is now an assistant professor at Stanford University. “You’re dealing with these cases all the time where there’s only partial information,” Wu says. “We’re extending our model to learn the dynamics of all particles, while only seeing a small portion.”
Robots that can sort recycling
Photo: Jason Dorfman
By Adam Conner-Simons
Every year trash companies sift through an estimated 68 million tons of recycling, which is the weight equivalent of more than 30 million cars.
A key step in the process happens on fast-moving conveyor belts, where workers have to sort items into categories like paper, plastic and glass. Such jobs are dull, dirty, and often unsafe, especially in facilities where workers also have to remove normal trash from the mix.
With that in mind, a team led by researchers at MIT’s Computer Science and Artificial Intelligence Laboratory (CSAIL) has developed a robotic system that can detect if an object is paper, metal, or plastic.
The team’s “RoCycle” system includes a soft Teflon hand that uses tactile sensors on its fingertips to detect an object’s size and stiffness. Compatible with any robotic arm, RoCycle was found to be 85 percent accurate at detecting materials when stationary, and 63 percent accurate on an actual simulated conveyer belt. (Its most common error was identifying paper-covered metal tins as paper, which the team says would be improved by adding more sensors along the contact surface.)
“Our robot’s sensorized skin provides haptic feedback that allows it to differentiate between a wide range of objects, from the rigid to the squishy,” says MIT Professor Daniela Rus, senior author on a related paper that will be presented in April at the IEEE International Conference on Soft Robotics (RoboSoft) in Seoul, South Korea. “Computer vision alone will not be able to solve the problem of giving machines human-like perception, so being able to use tactile input is of vital importance.”
A collaboration with Yale University, RoCycle directly demonstrates the limits of sight-based sorting: It can reliably distinguish between two visually similar Starbucks cups, one made of paper and one made of plastic, that would give vision systems trouble.
Incentivizing recycling
Rus says that the project is part of her larger goal to reduce the back-end cost of recycling, in order to incentivize more cities and countries to create their own programs. Today recycling centers aren’t particularly automated; their main kinds of machinery include optical sorters that use different wavelength light to distinguish between plastics, magnetic sorters that separate out iron and steel products, and aluminum sorters that use eddy currents to remove non-magnetic metals.
This is a problem for one very big reason: just last month China raised its standards for the cleanliness of recycled goods it accepts from the United States, meaning that some of the country’s single-stream recycling is now sent to landfills.
“If a system like RoCycle could be deployed on a wide scale, we’d potentially be able to have the convenience of single-stream recycling with the lower contamination rates of multi-stream recycling,” says PhD student Lillian Chin, lead author on the new paper.
It’s surprisingly hard to develop machines that can distinguish between paper, plastic, and metal, which shows how impressive a feat it is for humans. When we pick up an object, we can immediately recognize many of its qualities even with our eyes closed, like whether it’s large and stiff or small and soft. By feeling the object and understanding how that relates to the softness of our own fingertips, we are able to learn how to handle a wide range of objects without dropping or breaking them.
This kind of intuition is tough to program into robots. Traditional hard (“rigid”) robot hands have to know an object’s exact location and size to be able to calculate a precise motion path. Soft hands made of materials like rubber are much more flexible, but have a different problem: Because they’re powered by fluidic forces, they have a balloon-like structure that can puncture quite easily.
How RoCycle works
Rus’ team used a motor-driven hand made of a relatively new material called “auxetics.” Most materials get narrower when pulled on, like a rubber band when you stretch it; auxetics, meanwhile, actually get wider. The MIT team took this concept and put a twist on it, quite literally: They created auxetics that, when cut, twist to either the left or right. Combining a “left-handed” and “right-handed” auxetic for each of the hand’s two large fingers makes them interlock and oppose each other’s rotation, enabling more dynamic movement. (The team calls this “handed-shearing auxetics”, or HSA.)
“In contrast to soft robots, whose fluid-driven approach requires air pumps and compressors, HSA combines twisting with extension, meaning that you’re able to use regular motors,” says Chin.
The team’s gripper first uses its “strain sensor” to estimate an object’s size, and then uses its two pressure sensors to measure the force needed to grasp an object. These metrics — along with calibration data on the size and stiffnesses of objects of different material types — are what gives the gripper a sense of what material the object is made. (Since the tactile sensors are also conductive, they can detect metal by how much it changes the electrical signal.)
“In other words, we estimate the size and measure the pressure difference between the current closed hand and what a normal open hand should look like,” says Chin. “We use this pressure difference and size to classify the specific object based on information about different objects that we’ve already measured.”
RoCycle builds on an set of sensors that detect the radius of an object to within 30 percent accuracy, and tell the difference between “hard” and “soft” objects with 78 percent accuracy. The team’s hand is also almost completely puncture resistant: It was able to be scraped by a sharp lid and punctured by a needle more than 20 times, with minimal structural damage.
As a next step, the researchers plan to build out the system so that it can combine tactile data with actual video data from a robot’s cameras. This would allow the team to further improve its accuracy and potentially allow for even more nuanced differentiation between different kinds of materials.
Chin and Rus co-wrote the RoCycle paper alongside MIT postdoc Jeffrey Lipton, as well as PhD student Michelle Yuen and Professor Rebecca Kramer-Bottiglio of Yale University.
This project was supported in part by Amazon, JD.com, the Toyota Research Institute, and the National Science Foundation.
2018 industrial robot sales barely eke out year-over-year gain
The International Federation of Robotics (IFR), at a press conference here last week, announced preliminary 2018 figures for the industrial sector of the robotics industry. Last year set another record — but just barely. It was only up 1% over 2017. No information was given about service and field robotics.
It’s true that 2017 was a banner year, with a 30% year-over-year gain. So what happened in 2018 to slow that progress?
- China’s auto sales were down for the first time in 28 years — down 6% — and U.S. sales were flat. Globally, car sales were down 3%. This caused the automotive sector of the robotics industry to be down by 15% in China and 26% in the US.
- Global smartphones were also down 5%, which caused the electronics sector of the robotics industry to decline by 8%.
- According to Henry Sun, director of strategy at MINO Automotive Equipment China, “Consumers appear to be taking a ‘wait and see’ approach, as there is some uncertainty with rumors of policies affecting auto purchases, as well as uncertainty surrounding the general economy.”
- On the bright side, he also said: “EV manufacturing is a big stimulus for automotive robots. Installation of new production lines and plants and re-tooling of existing ones — such as battery and e-motor assembly — and expanding the EV portfolio is fundamental to many OEMs’ long-term strategies. China is the global focal point for EVs, and significant investments will be made [over the next many years].”
Although robot sales were down in Asia, they were up 6% in the Americas and 7% in in the EU. In fact, the U.S. had a really good year, up 15% from 2017, while both Canada and Mexico were down 15% and 13%, respectively.
Double-digit growth was seen in other types of manufacturing, including the food and beverage, pharmaceuticals, plastics, and metals sectors, reported the IFR.
IFR spokesman Steven Wyatt recaps 2018
Challenges on the horizon
Looking forward, the auto industry is likely to be more volatile, particularly as it transitions from the combustion engine to EVs (electric vehicles) and self-driving vehicles begin to come to market.
Speakers in the IFR CEO Roundtable, held at Automate in conjunction with the IFR’s announcement of its 2018 preliminary figures, stressed that finding skilled workers continues to be a primary concern.
Another challenge is that robot makers need to provide operating software for their products that is easy to learn, doesn’t require a legacy programmer, and is intuitive to use.
“The U.S. government is not doing a lot to strengthen U.S. competitiveness in robotics,” said Robert Atkinson, president of ITIF (Information Technology and Innovation Foundation). “The National Science Foundation does have a national robotics initiative to support research, but it is largely under-funded, not tied enough to industry needs, and is focused only on robots that complement, rather than replace workers.”
Byron Clayton, CEO of ARM (the Advanced Robotics for Manufacturing Institute), said: “The shortage of skilled workers is driving changes in how potential and existing employees are recruited and trained. Unskilled workers must be taught to operate, program, and maintain robots and related technologies.”
Introduction of machine learning
Junji Tsuda, IFR president and director chairman of the board of Yaskawa, discussed the role AI will play in the next few years in the robotics industry.
“AI is the great accelerator to enhance the capability of sensors and analysis of data,” he said. “AI technology application has already started, [but] we need system integrators‘ involvement to accelerate the process. There are two aspects of AI application: one for engineering, and the other for stable operations.”
- For engineering, the digital twin will be the key method, and machine learning with a simulator will be the biggest contributor.
- For stable operations, sensors will be the key factors to control quality of manufacturing and to keep machines running without unpredictable failures.
About the IFR
The IFR is composed of all of the national robot associations around the world, major R&D institutes, and big robot suppliers and integrators.
It is the primary resource for worldwide data on the use of robotics and produces two annual reports covering sales for the previous calendar year: World Robotics Industrial Robots and World Robotics Service Robots. The 2019 reports covering 2018 activity will be available in late September or October at a cost of around $2,250 for the set.
The IFR also sponsors the annual International Symposium on Robotics held this year in conjunction with Automate. It also co-sponsors the IERA Awards, which recognize the entrepreneurial commercialization of ideas into actual products.
Automate 2019 startup showdown recap
It’s been two years since the last time I judged the Automate Startup Competition. More than any other trade show contest, this event has been an oracle of future success. In following up with the last vintage of participants, all of the previous entrees are still operating and many are completing multi-million dollar financing rounds. As an indication of the importance of the venue, and quite possibly the growth of the industry, The Robot Report announced last week that 2017 finalist, Kinema Systems was acquired by SoftBank’s Boston Dynamics.
Traditionally, autonomous machines at the ProMat Show have been relegated to a subsection of the exhibit floor under the Automate brand. A couple of years ago there were a handful of self-driving rovers with twice as many robotic arms, today almost one third of the entire McCormick Center was promoting unmanned solutions. As e-commerce sales continue to explode, pressuring fulfilment centers nationwide, the logistics industry now demands two separate conventions with Automate 2021 being held in the Motor City for the first time. This palpable buzz formed the backdrop to the packed startup theater that represented the burgeoning mechatronic ecosystem. In the words of Jeff Burnstein, president of A3 (Automate’s organizers), “Automation is among the most dynamic emerging markets, with venture funding increasing robustly each year. The finalists in the Automate Launch Pad Startup Competition represent the many types of innovation that will transform the manufacturing and services sectors over the next decade.”
Freeing The Supplychain From Bottlenecks
The first company that presented, IM Systems (IMS) traveled from the Netherlands to Chicago to unveil its invention that strikes at the core of the robo-universe – actuation. Today, most of the co-bots deployed are utilizing rotary actuators from Japanese-owned Harmonic Drive (HD). HD’s speed reducers are the industry’s standard for gearing technology, offering the greatest level of movement control, force and precision of any commercially available mechanical system. This has translated to more than $500 million in annual revenue for HD and is projected to grow to more than $3 billion by 2024. HD’s grip on the industry is citied by many as the main reason why collaborative robot companies have failed to achieve unicorn-level growth. Currently unchallenged by competitors, HD has been free to artificially inflate prices by stiffly controlling the number of units it ships a year, maintaining a careful balance of low supply and high demand. As Thibaud Verschoor, founder of IM Systems, walked on stage I eagerly awaited to hear how his company was planning to disrupt HD’s virtual monopoly. Verschoor introduced a toothless gearbox called the Archimedes Drive that relies on friction instead of gear teeth to transmit torque, promising greater precision and lower cost thus hitting directly at Harmonic Drive. Originating from the Delft University of Technology, IMS is already boasting of its growing list of pre-orders with roboticists lining up for a long-awaited alternative. The startup founder quipped that today it is ‘now quicker to gestate a baby than get an actuator from Harmonic Drive, but not anymore’ as its business child will be shipping next year.
Extending Uptime Performance
In addition to actuation, energy efficiency has been a hurdle for robots in completing unplugged missions. WiBotic, an innovation spun out of the University of Washington, promises continuous charging availability with its patented wireless inductive power transfer system. Dr. Ben Waters took to the stage to demonstrate how WiBotic is able to charge unmanned vehicles and drones within a ten centimeter proximity of his transfer coils. In addition, WiBotic’s platform is also able to monitor a facility’s entire fleet of autonomous systems to provide managers with better power consumption and machine utilization data, enabling greater productivity and cost efficiency. When I asked Dr. Waters what is next for his company, he exclaimed that they have conquered the air with drones, land with robots, now they are aiming at the sea.
Maximizing Human Labor
Labor more than any other theme was the big discussion on the floor of ProMat and Automate. While many pundits decry automation for taking jobs, Daniel Theobald of Vecna Robotics shared with me, at our fireside chat, that no one has been fired because of a robot. In fact, today there are not enough humans to fulfill the growing demand of a global economy. One of the most grueling tasks still performed by humans is riveting, often performed in stiff contortions for hours at a time. Wilder Systems is a new collaborative robot system for the aerospace industry to relieve humans from the repetitive dangerous occupation of vertical drilling and fastening performed during fuselage manufacturing. Wilder offers a modular mobile system that is affordable for factories in providing their customers with greater speed, consistency, and accuracy. Until recently robotic systems like Wilder were only available to large corporations but now with the startups “robot-as-a-service” business model, smaller aircraft plants are able to automate.
Providing A Gentle Touch
When moderating a session the day before about the definition of success in deploying robots, a large grocery store operator asked the panel what is available for his needs. While hard goods is challenging, picking up the wide variety of fragile organic materials of food is almost impossible for traditional metal grippers. While there are a small number of soft robot solutions available on the market, most require air to be pumped into their elastomeric end effectors, adding cost and complexity to the installation. Ubiros claims to be the “first fully electrically operated soft gripper” without any expensive peripheral equipment, such as pressurized air. To illustrate the tenderness of their solution the startup showcased FlowerBot, a robot arm with their gripper that is capable of picking up roses to create attractive (pre-programmed) bouquets. Dr. Cagdas D. Onal, the company’s founder, proudly introduced the judges to their new customer that just purchased 250 units to begin automating his floral fulfillment center. To Dr Onal’s credit, the Automate pavilion literally smelled like roses as throngs of people sat in the theater with vases on their laps.
Visualizing Installations Before Production
An old proverb teaches that “seeing is believing,” unfortunately machines are often plagued more by Murphy’s Law during the on-boarding process. Firefly Dimension, an augmented reality startup for industrial applications, is focusing on speeding up manufacturing and product development with its unique perspective. Firefly’s headset is the only one in development promoting a 100% field of view equal to one’s eyes. This translates to lower down time, costs and potentially better outcomes. The company shared with the judges its early successes with manufacturers in China that are already testing their prototypes in their production facilities. As many industry analysts project the market for augmented reality solutions to exceed $60 billion by 2024, the Silicon Valley team of entrepreneurs is well positioned to take advantage of the next stage of automation technologies.
Making Robots Accessible For The Masses
According to Dr. Rahul Chipalkatty, CEO of Southie Autonomy, most of the tasks in the warehouse and factory are not automated because they change too quickly for professional integrators and on-staff engineers to respond. Dr. Chipalkatty asserts that this market is actually the lowest hanging fruit for automation, but has remained untapped as technologists have failed to target non-technical workers. To counter this trend, Southie literally developed a magic wand that utilizes artificial intelligence, gesture control and augmented reality projections to train robots on the fly to respond to spontaneous jobs. The Boston-based startup is marketing its technology for “ANY industrial robot to be re-purposed and re-deployed by ANY person, without robotics expertise or even computer skills.” Companies like Southie that focus on ease-of-use interfaces will only further the overall adoption of robotics in the coming years for millions of employees globally.
Building A Collision-Free World
On the day of the startup competition, Realtime Robotics announced the launch of its proprietary computer board and software that enables “collision-free” motion planning within milliseconds for collaborative robots and autonomous vehicles to work together. Marketed as RapidPlan and RapidSense the startup contends that its solution is the only one available to enable machines to safely operate within workcells with humans and other robots simultaneously. According to the press release (which was later explained on stage) RapidPlan enables users to load up to “20 million motions” into the system that analyzes “800,000 motions at 30 frames-per-second” which is automatically integrated with the machines onboard sensors via RapidSense. Realtime Robotics’ CEO, Peter Howard, bragged, “Our collision-free motion planning solutions allow robots to perform safely in dynamic, unstructured, and collaborative workspaces, while instantaneously reacting to changes as they occur.” Realtime, backed by Toyota AI, is definitely on my startup watch list for 2019/2020.
And The Winner Is
Deliberating in the judges room, we were challenged to pick a winner from so many qualified diverse startups. Balancing the presentations against the immediate needs of automation industry, one company universally stood out for its important contribution. IM Systems’ Archimedes Drive has the potential to generate a billion-dollar valuation with its promise of bringing down the cost of adoption and quickening the speed of deployment. Following the show, I caught up with IMS founder Jack Schorsch and asked him how he plans to compete against a multinational conglomerate like Harmonic Drive. Schorsch responded, “I do think that HD & Nabtesco have to some degree deliberately throttled their supply, in order to keep unit profits high on sales to everyone but Fanuc/ABB/Yaskawa. On the flip side, I know in my bones that if you can come to market with a technically comparable or better drive you can sell every unit that you can make.”
Join RobotLab on May 16th when we host a discussion with Alexis Block, inventor of HuggieBot, and Andrew Flett, partner at Mobility Impact Partners, on “Society 2.0: Understanding The Human-Robot Connection In Improving The World,” RSVP Today!
Robots that learn to use improvised tools
By Annie Xie
In many animals, tool-use skills emerge from a combination of observational learning and experimentation. For example, by watching one another, chimpanzees can learn how to use twigs to “fish” for insects. Similarly, capuchin monkeys demonstrate the ability to wield sticks as sweeping tools to pull food closer to themselves. While one might wonder whether these are just illustrations of “monkey see, monkey do,” we believe these tool-use abilities indicate a greater level of intelligence.
Left: A chimpanzee fishing for termites. Right: A gorilla using a stick to gather herbs. (source)
The question our new work explores is: can we enable robots to use tools in the same way — through observation and experimentation?
A requisite for performing complex multi-object manipulation tasks, such as those involved in tool use, is an understanding of physical cause-and-effect relationships. Therefore, the ability to predict how one object might interact with another is crucial. Our prior work has investigated how visual predictive models of cause-and-effect can be learned from unsupervised robot interaction with the world. After learning such a model, the robot can plan to accomplish a diverse set of simple tasks, including cloth folding and object arrangement. However, if we consider the more complex interactions that occur in tool-use tasks, such as how a broom can sweep dirt into a dustpan, undirected experimentation isn’t enough.
Hence, taking inspiration from how animals learn, we designed an algorithm that allows robots to learn tool-use skills through a similar paradigm of imitation and interaction. In particular, we show that, with a mix of demonstration data and unsupervised experience, a robot can use novel objects as tools and even improvise tools in the absence of traditional ones. Further, depending on the demands of the task, our method demonstrates the ability to decide whether to use the provided tools. In this post, we will describe how this works.