Researchers introduce a new generation of tiny, agile drones
Envisioning the 6G Future
Researchers introduce a new generation of tiny, agile drones
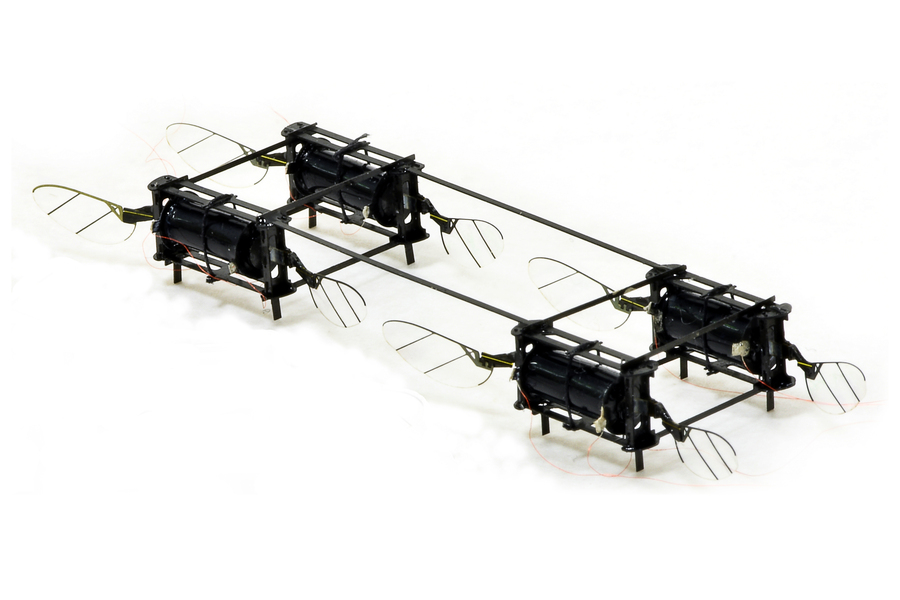
By Daniel Ackerman
If you’ve ever swatted a mosquito away from your face, only to have it return again (and again and again), you know that insects can be remarkably acrobatic and resilient in flight. Those traits help them navigate the aerial world, with all of its wind gusts, obstacles, and general uncertainty. Such traits are also hard to build into flying robots, but MIT Assistant Professor Kevin Yufeng Chen has built a system that approaches insects’ agility.
Chen, a member of the Department of Electrical Engineering and Computer Science and the Research Laboratory of Electronics, has developed insect-sized drones with unprecedented dexterity and resilience. The aerial robots are powered by a new class of soft actuator, which allows them to withstand the physical travails of real-world flight. Chen hopes the robots could one day aid humans by pollinating crops or performing machinery inspections in cramped spaces.
Chen’s work appears this month in the journal IEEE Transactions on Robotics. His co-authors include MIT PhD student Zhijian Ren, Harvard University PhD student Siyi Xu, and City University of Hong Kong roboticist Pakpong Chirarattananon.
Typically, drones require wide open spaces because they’re neither nimble enough to navigate confined spaces nor robust enough to withstand collisions in a crowd. “If we look at most drones today, they’re usually quite big,” says Chen. “Most of their applications involve flying outdoors. The question is: Can you create insect-scale robots that can move around in very complex, cluttered spaces?”
According to Chen, “The challenge of building small aerial robots is immense.” Pint-sized drones require a fundamentally different construction from larger ones. Large drones are usually powered by motors, but motors lose efficiency as you shrink them. So, Chen says, for insect-like robots “you need to look for alternatives.”
The principal alternative until now has been employing a small, rigid actuator built from piezoelectric ceramic materials. While piezoelectric ceramics allowed the first generation of tiny robots to take flight, they’re quite fragile. And that’s a problem when you’re building a robot to mimic an insect — foraging bumblebees endure a collision about once every second.
Chen designed a more resilient tiny drone using soft actuators instead of hard, fragile ones. The soft actuators are made of thin rubber cylinders coated in carbon nanotubes. When voltage is applied to the carbon nanotubes, they produce an electrostatic force that squeezes and elongates the rubber cylinder. Repeated elongation and contraction causes the drone’s wings to beat — fast.
Chen’s actuators can flap nearly 500 times per second, giving the drone insect-like resilience. “You can hit it when it’s flying, and it can recover,” says Chen. “It can also do aggressive maneuvers like somersaults in the air.” And it weighs in at just 0.6 grams, approximately the mass of a large bumble bee. The drone looks a bit like a tiny cassette tape with wings, though Chen is working on a new prototype shaped like a dragonfly.
“Achieving flight with a centimeter-scale robot is always an impressive feat,” says Farrell Helbling, an assistant professor of electrical and computer engineering at Cornell University, who was not involved in the research. “Because of the soft actuators’ inherent compliance, the robot can safely run into obstacles without greatly inhibiting flight. This feature is well-suited for flight in cluttered, dynamic environments and could be very useful for any number of real-world applications.”
Helbling adds that a key step toward those applications will be untethering the robots from a wired power source, which is currently required by the actuators’ high operating voltage. “I’m excited to see how the authors will reduce operating voltage so that they may one day be able to achieve untethered flight in real-world environments.”
Building insect-like robots can provide a window into the biology and physics of insect flight, a longstanding avenue of inquiry for researchers. Chen’s work addresses these questions through a kind of reverse engineering. “If you want to learn how insects fly, it is very instructive to build a scale robot model,” he says. “You can perturb a few things and see how it affects the kinematics or how the fluid forces change. That will help you understand how those things fly.” But Chen aims to do more than add to entomology textbooks. His drones can also be useful in industry and agriculture.
Chen says his mini-aerialists could navigate complex machinery to ensure safety and functionality. “Think about the inspection of a turbine engine. You’d want a drone to move around [an enclosed space] with a small camera to check for cracks on the turbine plates.”
Other potential applications include artificial pollination of crops or completing search-and-rescue missions following a disaster. “All those things can be very challenging for existing large-scale robots,” says Chen. Sometimes, bigger isn’t better.
An autonomous underwater robot saves people from drowning
Novel soft tactile sensor with skin-comparable characteristics for robots
Rapid robotics for operator safety: what a bottle picker can do
Dutch brewing company Heineken is one of the largest beer producers in the world with more than 70 production facilities globally. From small breweries to mega-plants, its logistics and production processes are increasingly complex and its machinery ever more advanced. The global beer giant therefore began looking for robotics solutions to make its breweries safer and more attractive for employees while enabling a more flexible organisation.
The environment is constantly changing and the robot has to be able to respond immediately.
Shobhit Yadav, mechatronics engineer smart industries and robotics at TNO
Automatically adapting to the situation
Dennis van der Plas, senior global lead packaging lines at Heineken, says, “We are becoming a high-tech company and attracting more and more technically trained staff. Repetitive tasks – like picking up fallen bottles from the conveyor belt will not provide them job satisfaction.” As part of the SMITZH innovation programme, Heineken and RoboHouse fieldlab, with support from the Netherlands Organisation for Applied Scientific Research (TNO), have developed a solution on the basis of flexible manufacturing: automated handling of unexpected situations.
According to Shobhit Yadav of TNO, flexible manufacturing is one of the most important developments in smart industry. “Today, manufacturing companies mainly produce small series on demand. It means that manufacturers have to be able to make many different products. This can be achieved either with a large number of production lines or with a small number that are flexible enough to adapt.” The Heineken project fell into the second category and involved developing a robot that could recognise different kinds of beer bottles that had fallen over on the conveyor. The robot had to pick them up while the belt was still moving. “The environment is constantly changing and the robot has to be able to respond immediately”, explains Shobhit. “This is a typical example of a flexible production line that automatically adapts to the situation.”
Robotics for a safe and enjoyable working environment
Industrial robots have obviously been around for a while. “The automotive industry deploys robots for welding car parts, whereas our sector uses them for automatically palletising products”, says Dennis. “But with this project we took a different approach. Our starting point was not a question of which robots exist and how they could be used. Instead, we focused on the needs and wishes of the people in the breweries, the operators who control and maintain the machines and how robots could support them in their work.”
The solution, in other words, had to lead not only to process optimisation but also – especially – to improved safety and greater job satisfaction. In addition, it would result in Heineken becoming a better employer. It is why Dennis and his colleague Wessel Reurslag, global lead packaging engineering & robotics, asked the operators what they would need to make their work safer and more interesting. One of the use cases that emerged was picking up bottles that had fallen over on the conveyor belt: repetitive but also unsafe as the glass bottles could break.
The lab is the place to meet for anyone involved in robotics.
Wessel Reurslag, global lead packaging engineering & robotics at Heineken
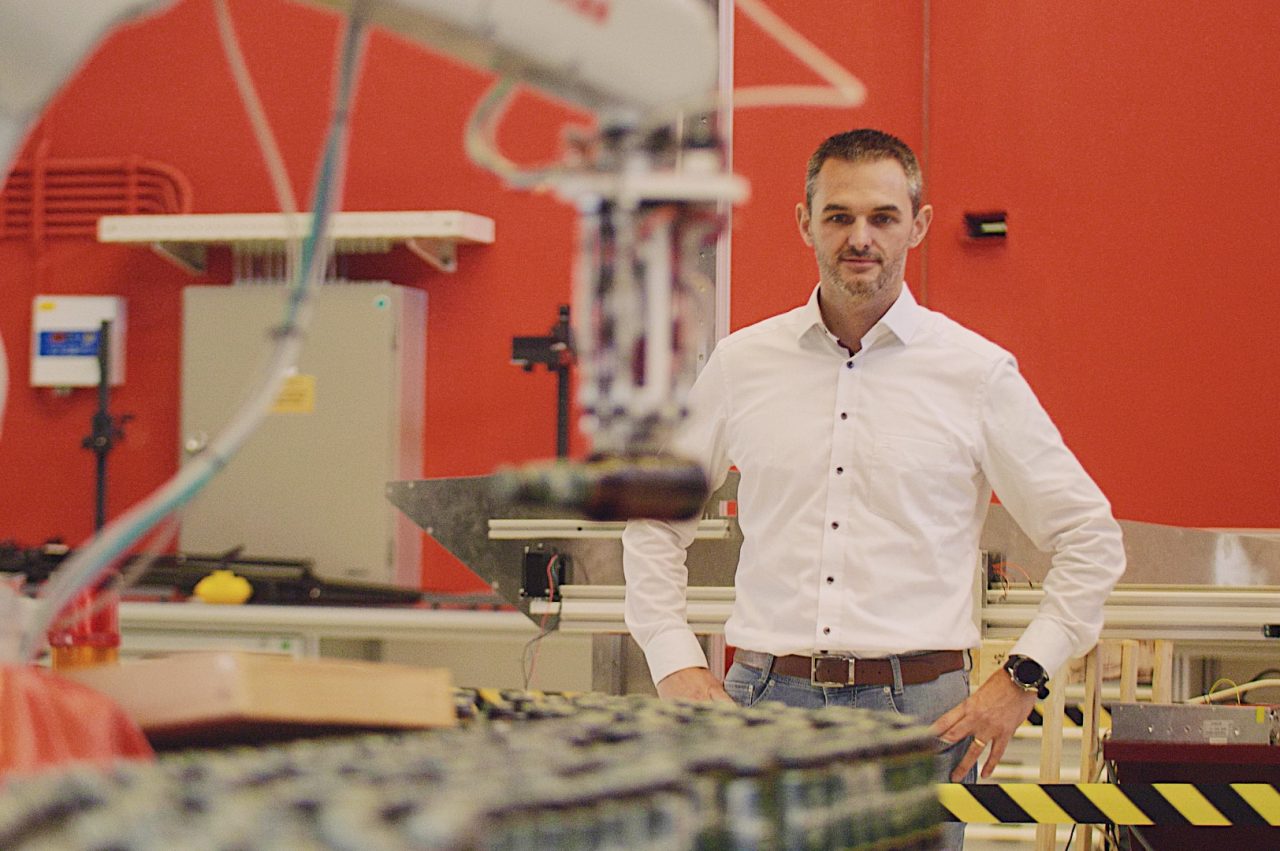
Experimenting without a business case
Heineken initially made contact with RoboHouse field lab through a sponsorship project with X!Delft, an initiative that strengthens corporate innovation and closes the gap between industry and Delft University of Technology. “The lab is the place to meet for anyone involved in robotics”, says Wessel. “It is also linked to SMITZH and thus connected to TNO.”
The parties soon realised that their ambitions overlapped. Heineken was seeking independent advice and both TNO and RoboHouse were looking for an applied research project that focused on flexible manufacturing. “This kind of partnership is very valuable to all involved”, says Shobhit. “SMITZH allows us at TNO to work with current issues in the industry and establish valuable contacts, which makes our research more relevant. In turn, manufacturers have somewhere to go with their questions and problems regarding smart technologies.”
Accessible way to do research and experiment
The guys at Heineken have nothing but praise for the innovative collaboration with TNO and RoboHouse. Dennis says, “The great thing is that all project partners were in it to learn something. At RoboHouse, we had access to the expertise of robotics engineers and state-of-the-art technologies like robotic arms. We supplied the bottle conveyor ourselves and TNO also added knowledge to the mix. It is an accessible way to do research and experiment. This would be a lot more difficult with a business case, which must involve an operational advantage from the start.”
Through close cooperation, we really developed a joint product.
Bas van Mil, mechanical engineer and robot gripper expert at RoboHouse
A joint product by TNO and RoboHouse
TNO and RoboHouse distilled two research goals from the use cases presented by Heineken: enabling real-time robot control and using vision technology to direct the robots with cameras. The main challenge involved devising a solution that could be applied to Heineken’s high-speed packaging lines. TNO worked on the control and movements of the robot, while RoboHouse took on the vision technology aspect. This entailed recognising the fallen bottles, developing the system’s software-based control and building the ‘gripper’ to pick up the bottles.
In many existing robotic systems, […] the robot will do a ‘blind pick.’
Bas van Mil, mechanical engineer and robot gripper expert at RoboHouse
“Communication between the robot and the computer is very important”, explains Bas van Mil, mechanical engineer at RoboHouse. “Our input and TNO’s work were complementary. For example, RoboHouse did not possess Shobhit’s knowledge of control technology, which is indispensable for controlling the robot. Through close cooperation, we really developed a joint product.”
Every millisecond counts
The biggest challenge in detecting and tracking fallen beer bottles is that they never stop moving. As Bas explains, “They do not just move in the direction of the bottle conveyor but can also roll around on the belt itself. In many existing robotic systems, the camera takes a single photo that informs the movements of the robot. The robot will do a ‘blind pick’ with no way of knowing whether anything has changed since the photo was taken. This only works if the environment stays the same – but in this case, it doesn’t.”
The solution involves a system in which the camera and the movements of the robot are constantly connected to each other. Bas says, “Every millisecond counts as the bottle will disappear from view and the robot will still try to pick it up from the spot where it was half a second ago.” A RoboHouse programmer developed the camera software to be as fast and efficient as possible. The field lab even purchased a powerful computer running an advanced AI system especially for the project.
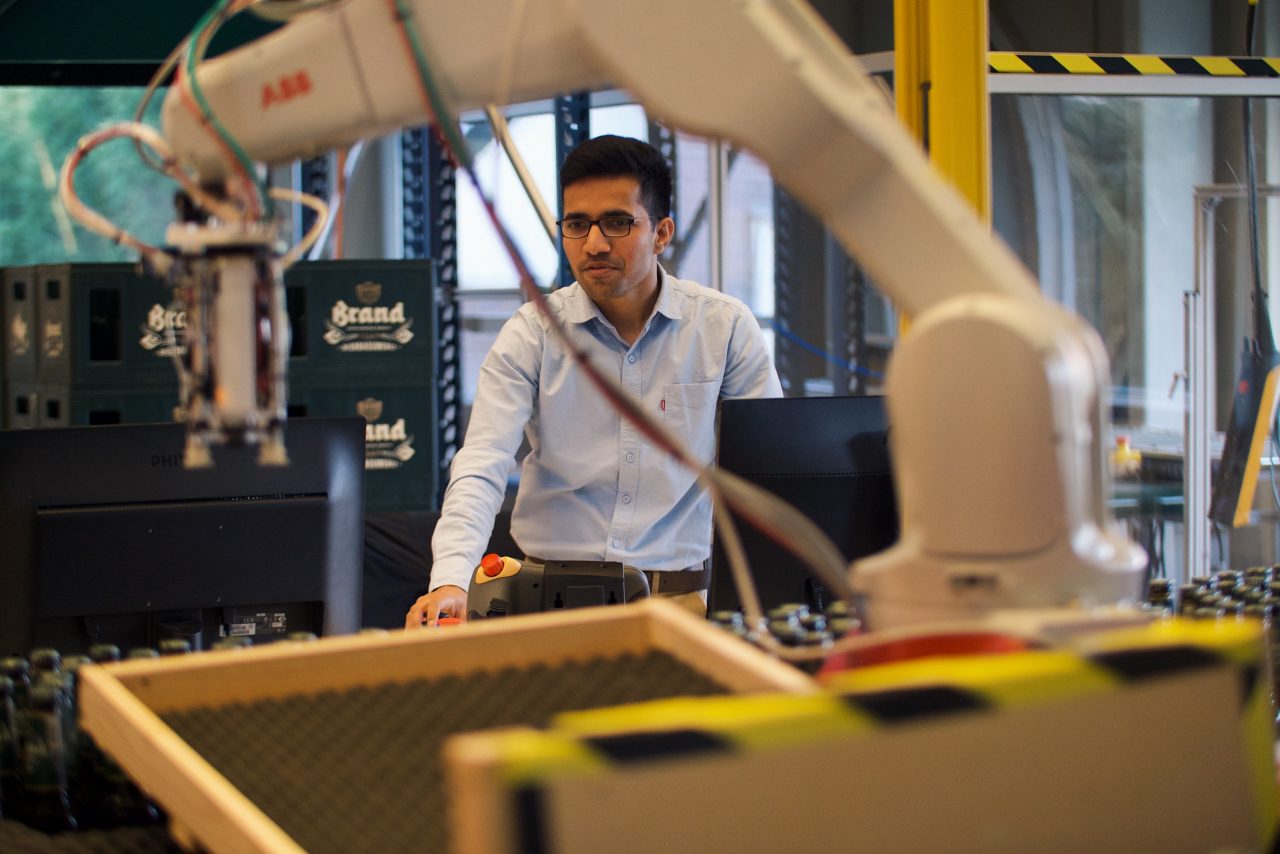
We are receiving feedback as well as requests for the new robotic systems from breweries all over the globe.
Dennis van der Plas, senior global lead packaging lines at Heineken
TNO and RoboHouse then wrote a programme together that determines the speed of the robot from the moment a fallen bottle is detected. This enables the robot to move with the bottle based on its calculated speed. It is what makes this robot so different from existing ones. “The robot responds immediately to changes”, says Shobhit. “In fact, it is 30 per cent faster than the current top speed of Heineken’s bottle conveyors. As a result, it has a wide range of applications and can be used in a variety of environments with different production speeds.”
Smarter thanks to independent partners
Heineken valued not just the successful innovation but also the independent character of TNO and RoboHouse during the development process. Dennis says, “We now have a much better idea of what is technically feasible, what the challenges are and what we can realistically ask of our technical suppliers. Thanks to this project, we can act much more like a smart buyer and make smarter demands of our suppliers. This information is relevant to have, especially as we operate in such an innovative field where we do not just buy parts off the shelf. After all, if I ask too little, I will not get the best out of my project. But if I ask too much, it affects our relationship with the supplier.”
The project has also served as a source of inspiration for Dennis’s colleagues worldwide. “We have been sharing videos and reports from SMITZH on the intranet, building a kind of community within Heineken. We are receiving feedback as well as requests for the new robotic systems from breweries all over the globe.” To meet the demand, Dennis and Wessel want to supply breweries with a ready-to-use version. “We are currently looking for parties that can make the technology available and provide support services.”
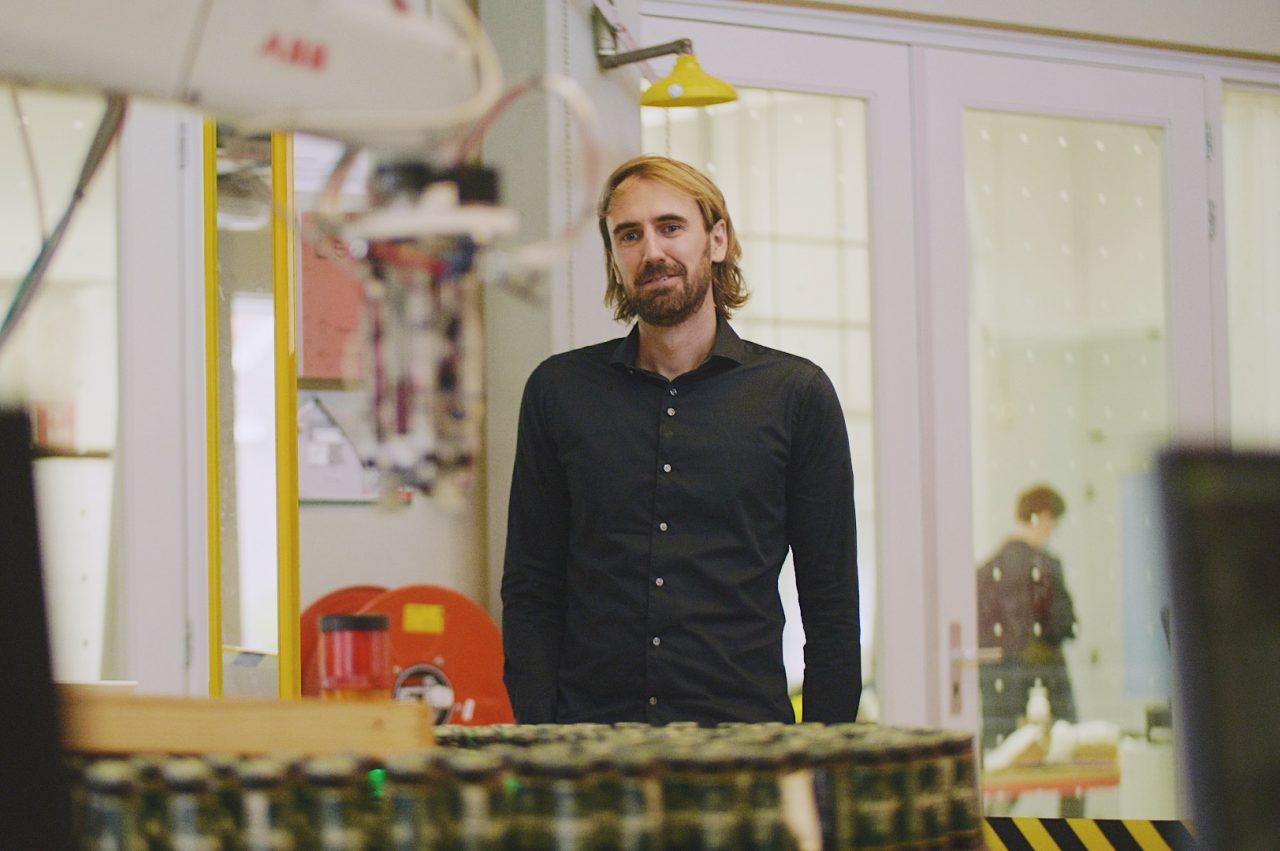
Meanwhile, RoboHouse and TNO aim to continue optimising the robot. “This is only the pilot version”, says Bas. “We can still improve its flexibility, for example by installing a different vision module, thereby making the technology even more widely applicable.” Both organisations are therefore looking for use cases in which they can use the same technology to solve other problems. “We are looking at the bigger picture”, explains Shobhit. “This project could serve as a model for similar challenges in other industries.”
Platform for valuable connections
All parties emphasise the benefits of working together and sharing knowledge to achieve a successful and relevant innovation. “There are very few places like SMITZH where manufacturing companies can go with these kinds of questions”, says Shobhit. “SMITZH is quite unique in that sense and is vitally important because it offers a specific platform for appropriate collaborations.” Wessel agrees: “If there is one thing this project has made clear, it is that producers like Heineken, tech companies and knowledge institutions should collaborate more intensively to substantiate these kinds of projects. You won’t find a solution in a PDF or a presentation. There can be no genuine solution until it is made real and put into practice.”
Do you have a similar use case and are you, like Heineken, searching for a practical solution? Or would you first like to learn more about RoboHouse or this specific project? Feel free to contact our lead engineer Bas van Mil, or email SMITZH with questions about its collaboration programme, or contact Shobhit Yadav at TNO more information.
SMITZH and future of work fieldlab RoboHouse
SMITZH is an innovation programme focused on smart manufacturing solutions in West Holland. It brings together supply and demand to stimulate the industrial application of smart manufacturing technologies and help regional companies innovate. Each SMITZH project consists of at least one manufacturing company and a fieldlab. RoboHouse served as the fieldlab in this project.
The post Rapid Robotics for Operator Safety: What a Bottle Picker Can Do appeared first on RoboValley.
Digital Innovation Hubs: €1.5 billion network to support green and digital transformation starts to take shape
A two-day discussion on the future of European Digital Innovation Hubs (EDIH) took place last month, as over 2000 stakeholders and policy-makers gathered online for the first time to take stock of the upcoming hubs network.
European Digital Innovation Hubs are one-stop-shops where companies and public sector organisations can access and test digital innovations, gain the required digital skills, get advice on financing support and ultimately accomplish their digital transformation in the context of the twin green and digital transition which is at the core of European industrial policy.
The EDIHs will become a network spread across the entire EU, sharing best practices and specialist knowledge and ready to support companies and public administrations in any region and economic sector. EDIHs will also play a brokering role between public administrations and companies providing e-government technologies, and are an important tool in Europe’s industrial and SME policies as they are close to local companies and ‘speak their language’.
Member States have already been invited to designate potential hubs. These potential hubs met for the first time on Tuesday the 26th of January at the Digital Innovation Hubs annual conference, organised in collaboration with the Luxembourg Ministry of Economy. Keynote speakers included Commissioner for the Internal Market Thierry Breton and Luxembourg Prime Minister Xavier Bettel. The 2000+ participants were provided with a unique opportunity to better prepare their submissions for the upcoming call and connect for further collaboration in building up the network of EDIHs.
Commissioner for the Internal Market Thierry Breton said:
Our vision is a resilient EU which is at the forefront of digital, where every citizen is digitally literate and where every single company can use the technology they need. Digital technology will help to provide the best public services in the world, and even small companies will be able to do business everywhere in Europe. This is the Europe we want, and the work of the European Digital Innovation Hubs will contribute to its construction.
The event was a discussion forum for potential EDIH candidates, policy makers and regional managers where the important role of the hubs was put in the context of Europe’s economic recovery and practical questions regarding how to set up a hub were answered. The conference is a crucial step in kick-starting the network, and is critical to building EU capacity to accelerate the digital innovation of businesses.
Half of the €1.5 billion investment in the EDIH network will come from the Digital Europe Programme and the rest through national and regional funds, financing the approximately 200 hubs from 2021 to 2027. They will act as a multiplier and widely diffuse the use of all the digital capacities built up under the different objectives of the Digital Europe Programme. Member States have worked in close collaboration with the European Commission to prepare the call.
Next steps
The potential hubs designated by Member States will be invited to submit their proposals for the call, which will select the hubs participating in the initial network of European Digital Innovation Hubs. Projects will be launched after summer 2021, and will immediately start working towards further digitalisation of all the sectors of the European economy, giving a strong contribution to the recovery after the COVID-19 crisis.
Researchers develop all-round grippers for contact-free society
Can a robot operate effectively underwater?
How Commercial Robotics Will Power the Logistics Industry in 2021
Self-supervised policy adaptation during deployment
Our method learns a task in a fixed, simulated environment and quickly adapts
to new environments (e.g. the real world) solely from online interaction during
deployment.
The ability for humans to generalize their knowledge and experiences to new situations is remarkable, yet poorly understood. For example, imagine a human driver that has only ever driven around their city in clear weather. Even though they never encountered true diversity in driving conditions, they have acquired the fundamental skill of driving, and can adapt reasonably fast to driving in neighboring cities, in rainy or windy weather, or even driving a different car, without much practice nor additional driver’s lessons. While humans excel at adaptation, building intelligent systems with common-sense knowledge and the ability to quickly adapt to new situations is a long-standing problem in artificial intelligence.
A robot trained to perform a given task in a lab environment may not generalize
to other environments, e.g. an environment with moving disco lights, even
though the task itself remains the same.
In recent years, learning both perception and behavioral policies in an end-to-end framework by deep Reinforcement Learning (RL) has been widely successful, and has achieved impressive results such as superhuman performance on Atari games played directly from screen pixels. Although impressive, it has become commonly understood that such policies fail to generalize to even subtle changes in the environment – changes that humans are easily able to adapt to. For this reason, RL has shown limited success beyond the game or environment in which it was originally trained, which presents a significant challenge in deployment of policies trained by RL in our diverse and unstructured real world.
Generalization by Randomization
In applications of RL, practitioners have sought to improve the generalization ability of policies by introducing randomization into the training environment (e.g. a simulation), also known as domain randomization. By randomizing elements of the training environment that are also expected to vary at test-time, it is possible to learn policies that are invariant to certain factors of variation. For autonomous driving, we may for example want our policy to be robust to changes in lighting, weather, and road conditions, as well as car models, nearby buildings, different city layouts, and so forth. While the randomization quickly evolves into an elaborate engineering challenge as more and more factors of variation are considered, the learning problem itself also becomes harder, greatly decreasing the sample efficiency of learning algorithms. It is therefore natural to ask: rather than learning a policy robust to all conceivable environmental changes, can we instead adapt a pre-trained policy to the new environment through interaction?
Left: training in a fixed environment. Right: training with
domain randomization.
Policy Adaptation
A naïve way to adapt a policy to new environments is by fine-tuning parameters using a reward signal. In real-world deployments, however, obtaining a reward signal often requires human feedback or careful engineering, neither of which are scalable solutions.
In recent work from our lab, we show that it is possible to adapt a pre-trained policy to unseen environments, without any reward signal or human supervision. A key insight is that, in the context of many deployments of RL, the fundamental goal of the task remains the same, even though there may be a mismatch in both visuals and underlying dynamics compared to the training environment, e.g. a simulation. When training a policy in simulation and deploying it in the real world (sim2real), there are often differences in dynamics due to imperfections in the simulation, and visual inputs captured by a camera are likely to differ from renderings of the simulation. Hence, the source of these errors often lie in an imperfect world understanding rather than misspecification of the task itself, and an agent’s interactions with a new environment can therefore provide us with valuable information about the disparity between its world understanding and reality.
Illustration of our framework for adaptation. Left: training before
deployment. The RL objective is optimized together with a self-supervised
objective. Right: adaptation during deployment. We optimize only the
self-supervised objective, using observations collected through interaction
with the environment.
To take advantage of this information we turn to the literature of self-supervised learning. We propose PAD, a general framework for adaptation of policies during deployment, by using self-supervision as a proxy for the absent reward signal. A given policy network $\pi$ parameterized by a collection of parameters $\theta$ is split sequentially into an encoder $\pi_{e}$ and a policy head $\pi_{a}$ such that $a_{t} = \pi(s_{t}; \theta) = \pi_{a} (\pi_{e}(s_{t}; \theta_{e}) ;\theta_{a})$ for a state $s_{t}$ and action $a_{t}$ at time $t$. We then let $\pi_{s}$ be a self-supervised task head and similarly let $\pi_{s}$ share the encoder $\pi_{e}$ with the policy head. During training, we optimize a self-supervised objective jointly together with the RL task, where the two tasks share part of a neural network. During deployment, we can no longer assume access to a reward signal and are unable to optimize the RL objective. However, we can still continue to optimize the self-supervised objective using observations collected through interaction with the new environment. At every step in the new environment, we update the policy through self-supervision, using only the most recently collected observation:
$$s_t \sim p(s_t | a_{t-1}, s_{t-1}) \\
\theta_{e}(t) = \theta_{e}(t-1) – \nabla_{\theta_{e}}L(s_{t}; \theta_{s}(t-1), \theta_{e}(t-1))$$
where L is a self-supervised objective. Assuming that gradients of the self-supervised objective are sufficiently correlated with those of the RL objective, any adaptation in the self-supervised task may also influence and correct errors in the perception and decision-making of the policy.
In practice, we use an inverse dynamics model $a_{t} = \pi_{s}( \pi_e(s_{t}), \pi_e(s_{t+1}))$, predicting the action taken in between two consecutive observations. Because an inverse dynamics model connects observations directly to actions, the policy can be adjusted for disparities both in visuals and dynamics (e.g. lighting conditions or friction) between training and test environments, solely through interaction with the new environment.
Adapting policies to the real world
We demonstrate the effectiveness of self-supervised policy adaptation (PAD) by training policies for robotic manipulation tasks in simulation and adapting them to the real world during deployment on a physical robot, taking observations directly from an uncalibrated camera. We evaluate generalization to a real robot environment that resembles the simulation, as well as two more challenging settings: a table cloth with increased friction, and continuously moving disco lights. In the demonstration below, we consider a Soft Actor-Critic (SAC) agent trained with an Inverse Dynamics Model (IDM), with and without the PAD adaptation mechanism.
Transferring a policy from simulation to the real world. SAC+IDM is a
Soft Actor-Critic (SAC) policy trained with an Inverse Dynamics Model (IDM),
and SAC+IDM (PAD) is the same policy but with the addition of policy
adaptation during deployment on the robot.
PAD adapts to changes in both visuals and dynamics, and nearly recovers the original success rate of the simulated environment. Policy adaptation is especially effective when the test environment differs from the training environment in multiple ways, e.g. where both visuals and physical properties such as object dimensionality and friction differ. Because it is often difficult to formally specify the elements that vary between a simulation and the real world, policy adaptation may be a promising alternative to domain randomization techniques in such settings.
Benchmarking generalization
Simulations provide a good platform for more comprehensive evaluation of RL algorithms. Together with PAD, we release DMControl Generalization Benchmark, a new benchmark for generalization in RL based on the DeepMind Control Suite, a popular benchmark for continuous control from images. In the DMControl Generalization Benchmark, agents are trained in a fixed environment and deployed in new environments with e.g. randomized colors or continuously changing video backgrounds. We consider an SAC agent trained with an IDM, with and without adaptation, and compare to CURL, a contrastive method discussed in a previous post. We compare the generalization ability of methods in the visualization below, and generally find that PAD can adapt even in non-stationary environments, a challenging problem setting where non-adaptive methods tend to fail. While CURL is found to generalize no better than the non-adaptive SAC trained with an IDM, agents can still benefit from the training signal that CURL provides during the training phase. Algorithms that learn both during training and deployment, and from multiple training signals, may therefore be preferred.
Generalization to an environment with video background. CURL is a
contrastive method, SAC+IDM is a Soft Actor-Critic (SAC) policy trained
with an Inverse Dynamics Model (IDM), and SAC+IDM (PAD) is the same
policy but with the addition of policy adaptation during deployment.
Summary
Previous work addresses the problem of generalization in RL by randomization, which requires anticipation of environmental changes and is known to not scale well. We formulate an alternative problem setting in vision-based RL: can we instead adapt a pre-trained policy to unseen environments, without any rewards or human feedback? We find that adapting policies through a self-supervised objective – solely from interactions in the new environment – is a promising alternative to domain randomization when the target environment is truly unknown. In the future, we ultimately envision agents that continuously learn and adapt to their surroundings, and are capable of learning both from explicit human feedback and through unsupervised interaction with the environment.
This post is based on the following paper:
- Self-Supervised Policy Adaptation during Deployment
Nicklas Hansen, Rishabh Jangir, Yu Sun, Guillem Alenyá, Pieter Abbeel, Alexei A. Efros, Lerrel Pinto, Xiaolong Wang
Ninth International Conference on Learning Representations (ICLR), 2021
arXiv, Project Website, Code