Invata Rolls Out New Line of Mobile Robotics
RoboKrill: A crustacean-inspired swimming robot for marine exploration
Harnessing AI and robotics to treat spinal cord injuries
The Reddit Robotics Showcase 2022
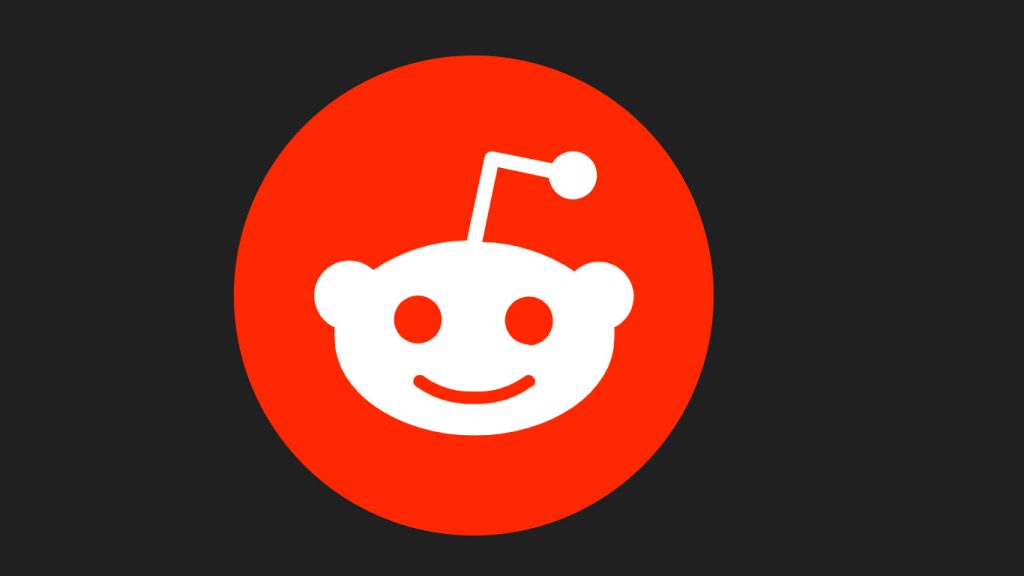
During the pandemic, members of the reddit & discord r/robotics community rallied to organize an online showcase for members of our community. What was originally envisioned as a half-day event with one mildly interesting guest speaker turned out to be a two day event with an incredible roster of participants from across the world. You can watch last year’s showcase here.
This year, we are planning an event which anticipates the enthusiasm we’ve had from our community, continuing to provide a unique opportunity for roboticists around the world to share and discuss their work, regardless of age or ability.
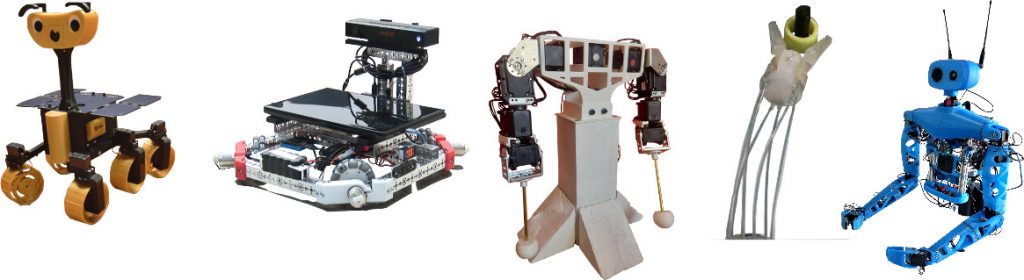
A small selection of last year’s robot’s.
We are delighted to receive support from Wevolver, Robohub, The National Robotarium, and the IEEE-RAS Soft Robotics Podcast, who are helping us to reach more people from academia industry, and ensure we are proactive with our representation of diversity.
“It’s great to see the reddit showcase is happening again! I presented last year, and it was a pleasure to see hobbyists, professionals, academics and students all together in one place sharing their passion for robotics. It’s important to see a diverse community, so I really encourage everyone to apply!
– Sabine Hauert, President of Robohub –
The showcase is free and online, and is to be held on July 30th & 31st 2022, livestreamed via the Reddit Robotics Showcase YouTube channel.
We extend an open invitation to those keen to share their robotics with the world. Please apply via this online form link. Successful applicants will be offered a timeslot of 10, 15, or 30 minutes, which includes both presentation and Q&A time. The committee will form presentation categories based on the applications. If you wish to register your interest as a keynote speaker, please email RRS chair Olly Smith directly.
This is not a formal academic conference, and alongside academic and industry professionals we encourage hobbyists, undergraduates, early career academics etc to apply, even if their work is still in progress. To that end, speakers are recommended to present their work in a public friendly manner.
To apply, please submit an application via this link before the deadline of April 30th.
The Aim
The primary purpose of this event is to showcase the multitude of projects underway in the r/Robotics Reddit community. Topics range across all focuses of robotics, such as simulation, navigation, control, perception, and mechatronic design. We will use this showcase to present discussion pieces and foster conversation between active members in the robotics community around the world. The showcase will feature invited roboticists in research and industry to discuss what they see as technical challenges or interesting directions for robots. We will focus on the following topics and showcase some of the amazing work being done by amateurs and academics, students and industry professionals alike.
- Autonomous Ground Vehicle (AGV – Mobile Robots)
- Unmanned Aerial Vehicle (UAV – Drones)
- Autonomous Underwater Vehicle (AUV – Submarines, Sailboats)
- Legged Robots (Bipeds, Quadrupeds, Hexapods)
- Manipulation (Robot Arms, Grippers, Hands)
- Simulation (Physical, Virtual, AI)
- Multi-Agent & Swarm Robotics
- Navigation, Path Planning, & Motion Planning
- Localisation & Mapping
- Perception & Machine Vision
- Artificial Intelligence, Machine Learning, Deep Learning
- Social Robots & Human Robot Interaction
- Domestic & Consumer Robots
- Commercial & Industrial Robotics
- Search & Rescue
Interview with Axel Krieger and Justin Opfermann: autonomous robotic laparoscopic surgery for intestinal anastomosis
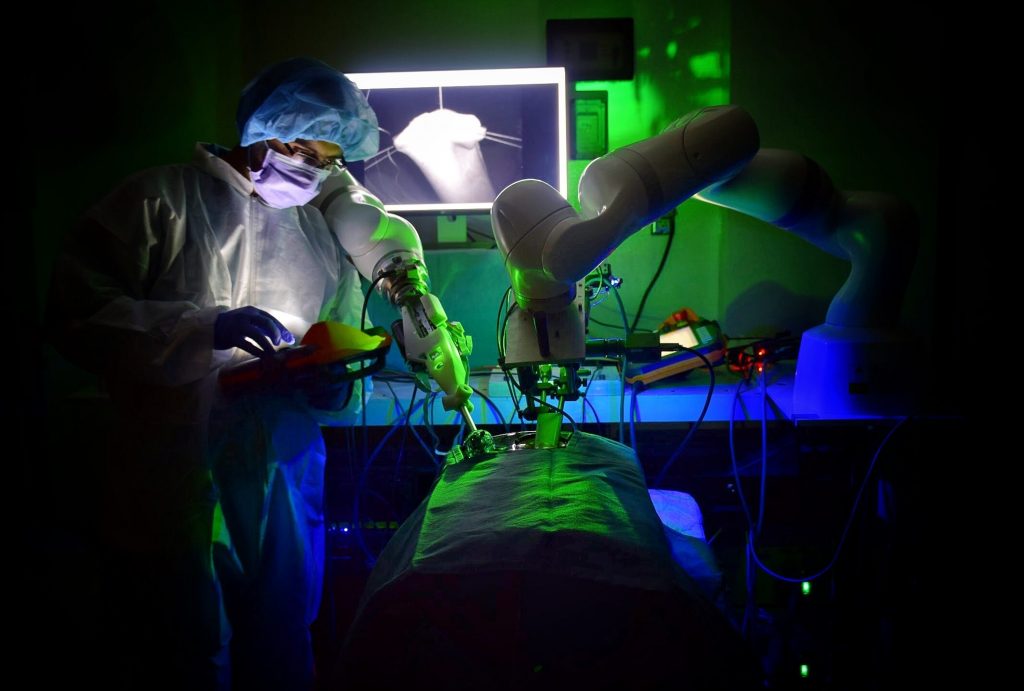
Axel Krieger is the Head of the Intelligent Medical Robotic Systems and Equipment (IMERSE) Lab at Johns Hopkins University, where Justin Opfermann is pursuing his PhD degree. Together with H. Saeidi, M. Kam, S. Wei, S. Leonard , M. H. Hsieh and J. U. Kang, they recently published the paper ‘Autonomous robotic laparoscopic surgery for intestinal anastomosis‘ in Science Robotics. Below, Axel and Justin tell us more about their work, the methodology, and what they are planning next.
What is the topic of the research in your paper?
Our research is focused on the design and evaluation of medical robots for autonomous soft tissue surgeries. In particular, this paper describes a surgical robot and workflow to perform autonomous anastomosis of the small bowel. Performance of the robot is conducted in synthetic tissues against expert surgeons, followed by experiments in pig studies to demonstrate preclinical feasibility of the system and approach.
Could you tell us about the implications of your research and why it is an interesting area for study?
Anastomosis is an essential step to the reconstructive phase of surgery and is performed over a million times each year in the United States alone. Surgical outcomes for patients are highly dependent on the surgeon’s skill, as even a single missed stitch can lead to anastomotic leak and infection in the patient. In laparoscopic surgeries these challenges are even more difficult due to space constraints, tissue motion, and deformations. Robotic anastomosis is one way to ensure that surgical tasks that require high precision and repeatability can be performed with more accuracy and precision in every patient independent of surgeon skill. Already there are autonomous surgical robots for hard tissue surgeries such as bone drilling for hip and knee implants. The Smart Tissue Autonomous Robot (STAR) takes the autonomous robotic skill one step further by performing surgical tasks on soft tissues. This enables a robot to work with a human to complete more complicated surgical tasks where preoperative planning is not possible. We hypothesize that this will result in a democratized surgical approach to patient care with more predictable and consistent patient outcomes.
Could you explain your methodology?
Until this paper, autonomous laparoscopic surgery was not possible in soft tissue due to the unpredictable motions of the tissue and limitations on the size of surgical tools. Performing autonomous surgery required the development of novel suturing tools, imaging systems, and robotic controls to visualize a surgical scene, generate an optimized surgical plan, and then execute that surgical plan with the highest precision. Combining all of these features into a single system is challenging. To accomplish these goals we integrated a robotic suturing tool that simplifies wristed suturing motions to the press of a button, developed a three dimensional endoscopic imaging system based on structured light that was small enough for laparoscopic surgery, and implemented a conditional autonomy control scheme that enables autonomous laparoscopic anastomosis. We evaluated the system against expert surgeons performing end to end anastomosis using either laparoscopic or da Vinci tele-operative techniques on synthetic small bowel across metrics such as consistency of suture spacing and suture bite, stitch hesitancy, and overall surgical time. These experiments were followed by preclinical feasibility tests in porcine small bowel. Limited necropsy was performed after one week to evaluate the quality of the anastomosis and immune response.
What were your main findings?
Comparison studies in synthetic tissues indicated that sutures placed by the STAR system had more consistent spacing and bite depth than those applied by surgeons using either a manual laparoscopic technique or robotic assistance with the da Vinci surgical system. The improved precision afforded by the autonomous approach led to a higher quality anastomosis for the STAR system which was qualitatively verified by laminar four dimension MRI flow fields across the anastomosis. The STAR system completed the anastomosis with a first stitch success rate of 83% which was better than surgeons in either group. Following the ex-vivo tests, STAR performed laparoscopic small bowel anastomosis in four pigs. All animals survived the procedure and had an average weight gain over the 1 week survival period. STAR’s anastomoses had similar burst strength, lumen area reduction, and healing as manually sewn samples, indicating the feasibility of autonomous soft tissue surgeries.
What further work are you planning in this area?
Our group is researching marker-less strategies to track tissue position, motion, and plan surgical tasks without the need for fiducial markers on tissues. The ability to three dimensionally reconstruct the surgical field on a computer and plan surgical tasks without the need for artificial landmarks would simplify autonomous surgical planning and enable collaborative surgery between an autonomous robot and human. Using machine learning and neural networks, we have demonstrated the robot’s ability to identify tissue edges and track natural landmarks. We are planning to implement fail-safe techniques and hope to perform first in human studies in the next few years.
About the interviewees
![]() |
Axel Krieger (PhD), an Assistant Professor in mechanical engineering, focuses on the development of novel tools, image guidance, and robot-control techniques for medical robotics. He is a member of the Laboratory for Computational Sensing and Robotics. He is also the Head of the Intelligent Medical Robotic Systems and Equipment (IMERSE) Lab at Johns Hopkins University. |
![]() |
Justin Opfermann (MS) is a PhD robotics student in the Department of Mechanical Engineering at Johns Hopkins University. Justin has ten years of experience in the design of autonomous robots and tools for laparoscopic surgery, and is also affiliated with the Laboratory for Computational Sensing and Robotics. Before joining JHU, Justin was a Project Manager and Senior Research and Design Engineer at the Sheikh Zayed Institute for Pediatric Surgical Innovation at Children’s National Hospital. |
Bendy robotic arm twisted into shape with help of augmented reality
Turing AI Debuts Mobilized Security Platform: The Turing Robot
How to help humans understand robots
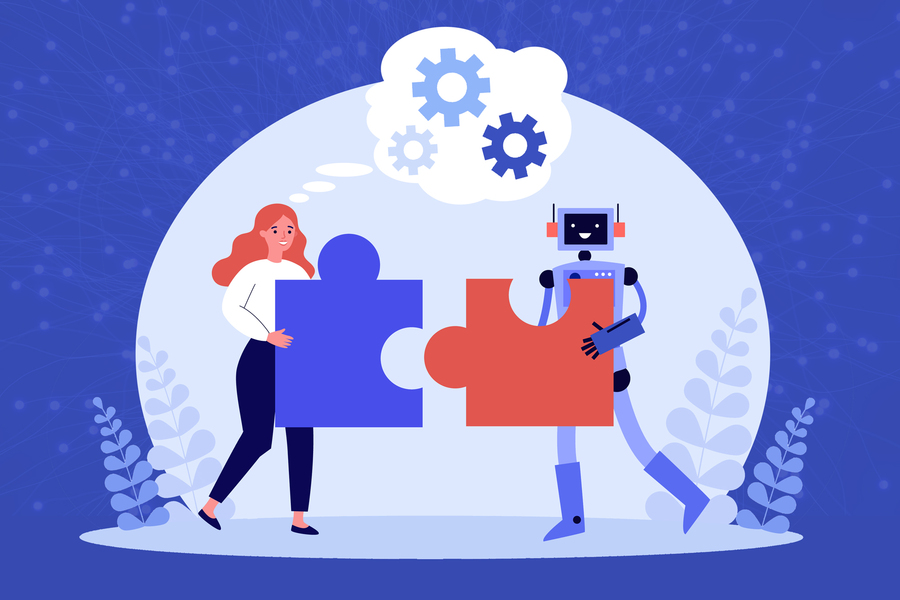
Researchers from MIT and Harvard suggest that applying theories from cognitive science and educational psychology to the area of human-robot interaction can help humans build more accurate mental models of their robot collaborators, which could boost performance and improve safety in cooperative workspaces. Image: MIT News, iStockphoto
By Adam Zewe | MIT News Office
Scientists who study human-robot interaction often focus on understanding human intentions from a robot’s perspective, so the robot learns to cooperate with people more effectively. But human-robot interaction is a two-way street, and the human also needs to learn how the robot behaves.
Thanks to decades of cognitive science and educational psychology research, scientists have a pretty good handle on how humans learn new concepts. So, researchers at MIT and Harvard University collaborated to apply well-established theories of human concept learning to challenges in human-robot interaction.
They examined past studies that focused on humans trying to teach robots new behaviors. The researchers identified opportunities where these studies could have incorporated elements from two complementary cognitive science theories into their methodologies. They used examples from these works to show how the theories can help humans form conceptual models of robots more quickly, accurately, and flexibly, which could improve their understanding of a robot’s behavior.
Humans who build more accurate mental models of a robot are often better collaborators, which is especially important when humans and robots work together in high-stakes situations like manufacturing and health care, says Serena Booth, a graduate student in the Interactive Robotics Group of the Computer Science and Artificial Intelligence Laboratory (CSAIL), and lead author of the paper.
“Whether or not we try to help people build conceptual models of robots, they will build them anyway. And those conceptual models could be wrong. This can put people in serious danger. It is important that we use everything we can to give that person the best mental model they can build,” says Booth.
Booth and her advisor, Julie Shah, an MIT professor of aeronautics and astronautics and the director of the Interactive Robotics Group, co-authored this paper in collaboration with researchers from Harvard. Elena Glassman ’08, MNG ’11, PhD ’16, an assistant professor of computer science at Harvard’s John A. Paulson School of Engineering and Applied Sciences, with expertise in theories of learning and human-computer interaction, was the primary advisor on the project. Harvard co-authors also include graduate student Sanjana Sharma and research assistant Sarah Chung. The research will be presented at the IEEE Conference on Human-Robot Interaction.
A theoretical approach
The researchers analyzed 35 research papers on human-robot teaching using two key theories. The “analogical transfer theory” suggests that humans learn by analogy. When a human interacts with a new domain or concept, they implicitly look for something familiar they can use to understand the new entity.
The “variation theory of learning” argues that strategic variation can reveal concepts that might be difficult for a person to discern otherwise. It suggests that humans go through a four-step process when they interact with a new concept: repetition, contrast, generalization, and variation.
While many research papers incorporated partial elements of one theory, this was most likely due to happenstance, Booth says. Had the researchers consulted these theories at the outset of their work, they may have been able to design more effective experiments.
For instance, when teaching humans to interact with a robot, researchers often show people many examples of the robot performing the same task. But for people to build an accurate mental model of that robot, variation theory suggests that they need to see an array of examples of the robot performing the task in different environments, and they also need to see it make mistakes.
“It is very rare in the human-robot interaction literature because it is counterintuitive, but people also need to see negative examples to understand what the robot is not,” Booth says.
These cognitive science theories could also improve physical robot design. If a robotic arm resembles a human arm but moves in ways that are different from human motion, people will struggle to build accurate mental models of the robot, Booth explains. As suggested by analogical transfer theory, because people map what they know — a human arm — to the robotic arm, if the movement doesn’t match, people can be confused and have difficulty learning to interact with the robot.
Enhancing explanations
Booth and her collaborators also studied how theories of human-concept learning could improve the explanations that seek to help people build trust in unfamiliar, new robots.
“In explainability, we have a really big problem of confirmation bias. There are not usually standards around what an explanation is and how a person should use it. As researchers, we often design an explanation method, it looks good to us, and we ship it,” she says.
Instead, they suggest that researchers use theories from human concept learning to think about how people will use explanations, which are often generated by robots to clearly communicate the policies they use to make decisions. By providing a curriculum that helps the user understand what an explanation method means and when to use it, but also where it does not apply, they will develop a stronger understanding of a robot’s behavior, Booth says.
Based on their analysis, they make a number recommendations about how research on human-robot teaching can be improved. For one, they suggest that researchers incorporate analogical transfer theory by guiding people to make appropriate comparisons when they learn to work with a new robot. Providing guidance can ensure that people use fitting analogies so they aren’t surprised or confused by the robot’s actions, Booth says.
They also suggest that including positive and negative examples of robot behavior, and exposing users to how strategic variations of parameters in a robot’s “policy” affect its behavior, eventually across strategically varied environments, can help humans learn better and faster. The robot’s policy is a mathematical function that assigns probabilities to each action the robot can take.
“We’ve been running user studies for years, but we’ve been shooting from the hip in terms of our own intuition as far as what would or would not be helpful to show the human. The next step would be to be more rigorous about grounding this work in theories of human cognition,” Glassman says.
Now that this initial literature review using cognitive science theories is complete, Booth plans to test their recommendations by rebuilding some of the experiments she studied and seeing if the theories actually improve human learning.
This work is supported, in part, by the National Science Foundation.