Building The Next Generation of Commercial Mowers
Amazon develops algorithm to improve collaboration between robots and humans
Radar navigation for autonomous cars can ‘see’ through smoke, dust and fog
Fulfil Solutions Emerges From Stealth With Revolutionary Robotic Automation to Make Online Grocery Profitable
A tiny new climbing robot inspired by geckos and inchworms
Robot Talk Episode 38 – Jonathan Aitken
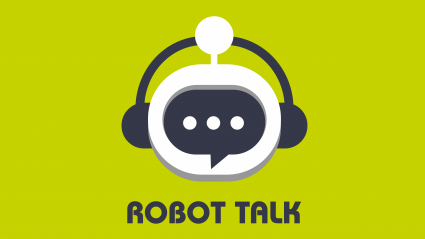
Claire chatted to Dr Jonathan Aitken from the University of Sheffield all about manufacturing, sewer inspection, and robots in the real world.
Jonathan Aitken is a Senior University Teacher in Robotics at the University of Sheffield. His research is focused on building useful, useable, and expandable architectures for future robotics systems. Most recently this has involved building complex digital twins for collaborative robots in manufacturing processes and investigating localisation for robots operating in sewer pipes. His teaching focuses on providing students with the tools to bring distributed computing to complex robotic processes.
Reaching like an octopus: A biology-inspired model opens the door to soft robot control
Flexible e-skin to spur rise of soft machines that feel
Why the conventional deep learning model is broken
Drones over Ukraine: What the war means for the future of remotely piloted aircraft in combat
When Will the Mobile Robot Vendor Base Consolidate?
Custom, 3D-printed heart replicas look and pump just like the real thing
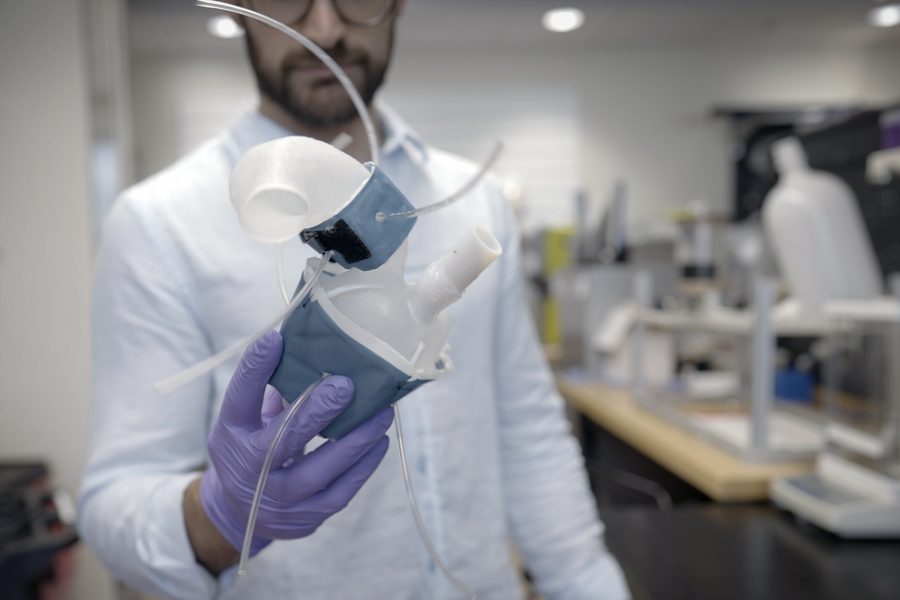
MIT engineers are hoping to help doctors tailor treatments to patients’ specific heart form and function, with a custom robotic heart. The team has developed a procedure to 3D print a soft and flexible replica of a patient’s heart. Image: Melanie Gonick, MIT
By Jennifer Chu | MIT News Office
No two hearts beat alike. The size and shape of the the heart can vary from one person to the next. These differences can be particularly pronounced for people living with heart disease, as their hearts and major vessels work harder to overcome any compromised function.
MIT engineers are hoping to help doctors tailor treatments to patients’ specific heart form and function, with a custom robotic heart. The team has developed a procedure to 3D print a soft and flexible replica of a patient’s heart. They can then control the replica’s action to mimic that patient’s blood-pumping ability.
The procedure involves first converting medical images of a patient’s heart into a three-dimensional computer model, which the researchers can then 3D print using a polymer-based ink. The result is a soft, flexible shell in the exact shape of the patient’s own heart. The team can also use this approach to print a patient’s aorta — the major artery that carries blood out of the heart to the rest of the body.
To mimic the heart’s pumping action, the team has fabricated sleeves similar to blood pressure cuffs that wrap around a printed heart and aorta. The underside of each sleeve resembles precisely patterned bubble wrap. When the sleeve is connected to a pneumatic system, researchers can tune the outflowing air to rhythmically inflate the sleeve’s bubbles and contract the heart, mimicking its pumping action.
The researchers can also inflate a separate sleeve surrounding a printed aorta to constrict the vessel. This constriction, they say, can be tuned to mimic aortic stenosis — a condition in which the aortic valve narrows, causing the heart to work harder to force blood through the body.
Doctors commonly treat aortic stenosis by surgically implanting a synthetic valve designed to widen the aorta’s natural valve. In the future, the team says that doctors could potentially use their new procedure to first print a patient’s heart and aorta, then implant a variety of valves into the printed model to see which design results in the best function and fit for that particular patient. The heart replicas could also be used by research labs and the medical device industry as realistic platforms for testing therapies for various types of heart disease.
“All hearts are different,” says Luca Rosalia, a graduate student in the MIT-Harvard Program in Health Sciences and Technology. “There are massive variations, especially when patients are sick. The advantage of our system is that we can recreate not just the form of a patient’s heart, but also its function in both physiology and disease.”
Rosalia and his colleagues report their results in a study appearing in Science Robotics. MIT co-authors include Caglar Ozturk, Debkalpa Goswami, Jean Bonnemain, Sophie Wang, and Ellen Roche, along with Benjamin Bonner of Massachusetts General Hospital, James Weaver of Harvard University, and Christopher Nguyen, Rishi Puri, and Samir Kapadia at the Cleveland Clinic in Ohio.
Print and pump
In January 2020, team members, led by mechanical engineering professor Ellen Roche, developed a “biorobotic hybrid heart” — a general replica of a heart, made from synthetic muscle containing small, inflatable cylinders, which they could control to mimic the contractions of a real beating heart.
Shortly after those efforts, the Covid-19 pandemic forced Roche’s lab, along with most others on campus, to temporarily close. Undeterred, Rosalia continued tweaking the heart-pumping design at home.
“I recreated the whole system in my dorm room that March,” Rosalia recalls.
Months later, the lab reopened, and the team continued where it left off, working to improve the control of the heart-pumping sleeve, which they tested in animal and computational models. They then expanded their approach to develop sleeves and heart replicas that are specific to individual patients. For this, they turned to 3D printing.
“There is a lot of interest in the medical field in using 3D printing technology to accurately recreate patient anatomy for use in preprocedural planning and training,” notes Wang, who is a vascular surgery resident at Beth Israel Deaconess Medical Center in Boston.
An inclusive design
In the new study, the team took advantage of 3D printing to produce custom replicas of actual patients’ hearts. They used a polymer-based ink that, once printed and cured, can squeeze and stretch, similarly to a real beating heart.
As their source material, the researchers used medical scans of 15 patients diagnosed with aortic stenosis. The team converted each patient’s images into a three-dimensional computer model of the patient’s left ventricle (the main pumping chamber of the heart) and aorta. They fed this model into a 3D printer to generate a soft, anatomically accurate shell of both the ventricle and vessel.
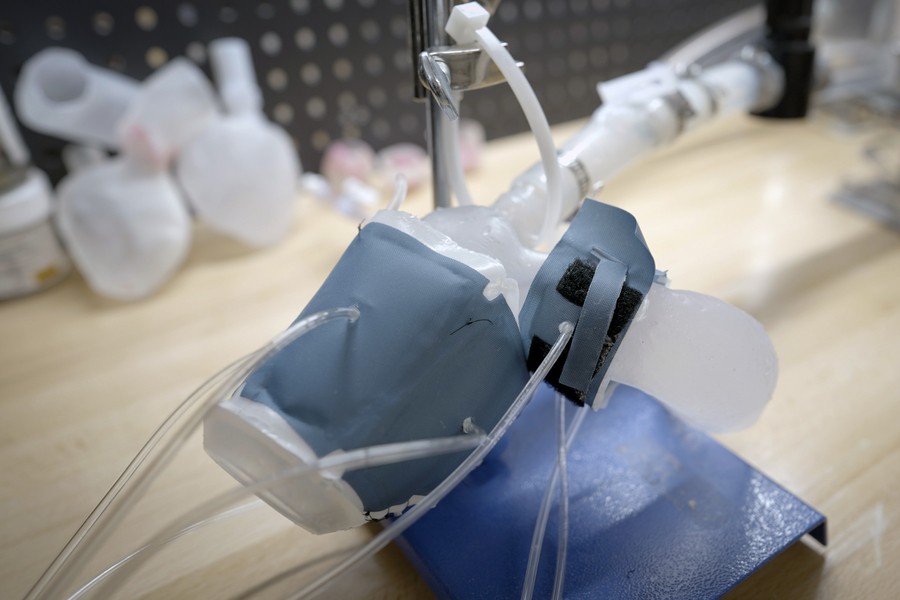
The action of the soft, robotic models can be controlled to mimic the patient’s blood-pumping ability. Image: Melanie Gonick, MIT
The team also fabricated sleeves to wrap around the printed forms. They tailored each sleeve’s pockets such that, when wrapped around their respective forms and connected to a small air pumping system, the sleeves could be tuned separately to realistically contract and constrict the printed models.
The researchers showed that for each model heart, they could accurately recreate the same heart-pumping pressures and flows that were previously measured in each respective patient.
“Being able to match the patients’ flows and pressures was very encouraging,” Roche says. “We’re not only printing the heart’s anatomy, but also replicating its mechanics and physiology. That’s the part that we get excited about.”
Going a step further, the team aimed to replicate some of the interventions that a handful of the patients underwent, to see whether the printed heart and vessel responded in the same way. Some patients had received valve implants designed to widen the aorta. Roche and her colleagues implanted similar valves in the printed aortas modeled after each patient. When they activated the printed heart to pump, they observed that the implanted valve produced similarly improved flows as in actual patients following their surgical implants.
Finally, the team used an actuated printed heart to compare implants of different sizes, to see which would result in the best fit and flow — something they envision clinicians could potentially do for their patients in the future.
“Patients would get their imaging done, which they do anyway, and we would use that to make this system, ideally within the day,” says co-author Nguyen. “Once it’s up and running, clinicians could test different valve types and sizes and see which works best, then use that to implant.”
Ultimately, Roche says the patient-specific replicas could help develop and identify ideal treatments for individuals with unique and challenging cardiac geometries.
“Designing inclusively for a large range of anatomies, and testing interventions across this range, may increase the addressable target population for minimally invasive procedures,” Roche says.
This research was supported, in part, by the National Science Foundation, the National Institutes of Health, and the National Heart Lung Blood Institute.
Intelligent Box Opening Device (IBOD)
Fully autonomous real-world reinforcement learning with applications to mobile manipulation
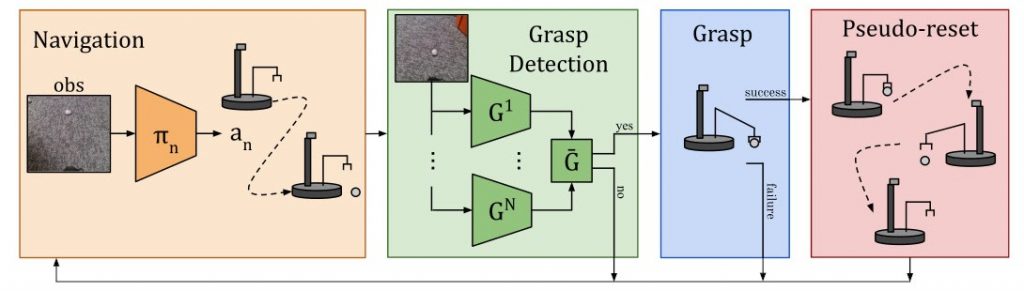
By Jędrzej Orbik, Charles Sun, Coline Devin, Glen Berseth
Reinforcement learning provides a conceptual framework for autonomous agents to learn from experience, analogously to how one might train a pet with treats. But practical applications of reinforcement learning are often far from natural: instead of using RL to learn through trial and error by actually attempting the desired task, typical RL applications use a separate (usually simulated) training phase. For example, AlphaGo did not learn to play Go by competing against thousands of humans, but rather by playing against itself in simulation. While this kind of simulated training is appealing for games where the rules are perfectly known, applying this to real world domains such as robotics can require a range of complex approaches, such as the use of simulated data, or instrumenting real-world environments in various ways to make training feasible under laboratory conditions. Can we instead devise reinforcement learning systems for robots that allow them to learn directly “on-the-job”, while performing the task that they are required to do? In this blog post, we will discuss ReLMM, a system that we developed that learns to clean up a room directly with a real robot via continual learning.
We evaluate our method on different tasks that range in difficulty. The top-left task has uniform white blobs to pickup with no obstacles, while other rooms have objects of diverse shapes and colors, obstacles that increase navigation difficulty and obscure the objects and patterned rugs that make it difficult to see the objects against the ground.
To enable “on-the-job” training in the real world, the difficulty of collecting more experience is prohibitive. If we can make training in the real world easier, by making the data gathering process more autonomous without requiring human monitoring or intervention, we can further benefit from the simplicity of agents that learn from experience. In this work, we design an “on-the-job” mobile robot training system for cleaning by learning to grasp objects throughout different rooms.
Lesson 1: The Benefits of Modular Policies for Robots.
People are not born one day and performing job interviews the next. There are many levels of tasks people learn before they apply for a job as we start with the easier ones and build on them. In ReLMM, we make use of this concept by allowing robots to train common-reusable skills, such as grasping, by first encouraging the robot to prioritize training these skills before learning later skills, such as navigation. Learning in this fashion has two advantages for robotics. The first advantage is that when an agent focuses on learning a skill, it is more efficient at collecting data around the local state distribution for that skill.
That is shown in the figure above, where we evaluated the amount of prioritized grasping experience needed to result in efficient mobile manipulation training. The second advantage to a multi-level learning approach is that we can inspect the models trained for different tasks and ask them questions, such as, “can you grasp anything right now” which is helpful for navigation training that we describe next.
Training this multi-level policy was not only more efficient than learning both skills at the same time but it allowed for the grasping controller to inform the navigation policy. Having a model that estimates the uncertainty in its grasp success (Ours above) can be used to improve navigation exploration by skipping areas without graspable objects, in contrast to No Uncertainty Bonus which does not use this information. The model can also be used to relabel data during training so that in the unlucky case when the grasping model was unsuccessful trying to grasp an object within its reach, the grasping policy can still provide some signal by indicating that an object was there but the grasping policy has not yet learned how to grasp it. Moreover, learning modular models has engineering benefits. Modular training allows for reusing skills that are easier to learn and can enable building intelligent systems one piece at a time. This is beneficial for many reasons, including safety evaluation and understanding.
Lesson 2: Learning systems beat hand-coded systems, given time
Many robotics tasks that we see today can be solved to varying levels of success using hand-engineered controllers. For our room cleaning task, we designed a hand-engineered controller that locates objects using image clustering and turns towards the nearest detected object at each step. This expertly designed controller performs very well on the visually salient balled socks and takes reasonable paths around the obstacles but it can not learn an optimal path to collect the objects quickly, and it struggles with visually diverse rooms. As shown in video 3 below, the scripted policy gets distracted by the white patterned carpet while trying to locate more white objects to grasp.
1)
2)
3)
4)
We show a comparison between (1) our policy at the beginning of training (2) our policy at the end of training (3) the scripted policy. In (4) we can see the robot’s performance improve over time, and eventually exceed the scripted policy at quickly collecting the objects in the room.
Given we can use experts to code this hand-engineered controller, what is the purpose of learning? An important limitation of hand-engineered controllers is that they are tuned for a particular task, for example, grasping white objects. When diverse objects are introduced, which differ in color and shape, the original tuning may no longer be optimal. Rather than requiring further hand-engineering, our learning-based method is able to adapt itself to various tasks by collecting its own experience.
However, the most important lesson is that even if the hand-engineered controller is capable, the learning agent eventually surpasses it given enough time. This learning process is itself autonomous and takes place while the robot is performing its job, making it comparatively inexpensive. This shows the capability of learning agents, which can also be thought of as working out a general way to perform an “expert manual tuning” process for any kind of task. Learning systems have the ability to create the entire control algorithm for the robot, and are not limited to tuning a few parameters in a script. The key step in this work allows these real-world learning systems to autonomously collect the data needed to enable the success of learning methods.
This post is based on the paper “Fully Autonomous Real-World Reinforcement Learning with Applications to Mobile Manipulation”, presented at CoRL 2021. You can find more details in our paper, on our website and the on the video. We provide code to reproduce our experiments. We thank Sergey Levine for his valuable feedback on this blog post.