Robots may care for you in old age—and your children will teach them
iRobot Introduces the Root Coding Robot Through Acquisition of Root Robotics
#289: On Design in Human-Robot Interaction, with Bilge Mutlu
In this episode, Audrow Nash interviews Bilge Mutlu, Associate Professor at the University of Wisconsin–Madison, about design-thinking in human-robot interaction. Professor Mutlu discusses design-thinking at a high-level, how design relates to science, and he speaks about the main areas of his work: the design space, the evaluation space, and how features are used within a context. He also gives advice on how to apply a design-oriented mindset.
Bilge Mutlu
Bilge Mutlu is an Associate Professor of Computer Science, Psychology, and Industrial Engineering at the University of Wisconsin–Madison. He directs the Wisconsin HCI Laboratory and organizes the WHCI+D Group. He received his PhD degree from Carnegie Mellon University‘s Human-Computer Interaction Institute.
Links
The world’s smallest autonomous racing drone
Racing team 2018-2019: Christophe De Wagter, Guido de Croon, Shuo Li, Phillipp Dürnay, Jiahao Lin, Simon Spronk
Autonomous drone racing
Drone racing is becoming a major e-sports. Enthusiasts – and now also professionals – transform drones into seriously fast racing platforms. Expert drone racers can reach speeds up to 190 km/h. They fly by looking at a first-person view (FPV) of their drone, which has a camera transmitting images mounted on the front.
In recent years, the advance in areas such as artificial intelligence, computer vision, and control has raised the question whether drones would not be able to fly faster than humans. The advantage for the drone could be that it can sense much more than the human pilot (like accelerations and rotation rates with its inertial sensors) and process all image data quicker on board of the drone. Moreover, its intelligence could be shaped purely for only one goal: racing as fast as possible.
In the quest for a fast-flying, autonomous racing drone, multiple autonomous racing drone competitions have been organized in the academic community. These “IROS” drone races (where IROS stands for one of the most well-known world-wide robotics conferences) have been held from 2016 on. Over these years, the speed of the drones has been gradually improving, with the faster drones in the competition now moving at ~2 m/s.
Smaller
Most of the autonomous racing drones are equipped with high-performance processors, with multiple, high-quality cameras and sometimes even with laser scanners. This allows these drones to use state-of-the-art solutions to visual perception, like building maps of the environment or tracking accurately how the drone is moving over time. However, it also makes the drones relatively heavy and expensive.
At the Micro Air Vehicle laboratory (MAVLab) of TU Delft, we have as aim to make light-weight and cheap autonomous racing drones. Such drones could be used by many drone racing enthusiasts to train with or fly against. If the drone becomes small enough, it could even be used for racing at home. Aiming for “small” means serious limitations to the sensors and processing that can be carried onboard. This is why in the IROS drone races we have always focused on monocular vision (a single camera) and on software algorithms for vision, state estimation, and control that are computationally highly efficient.
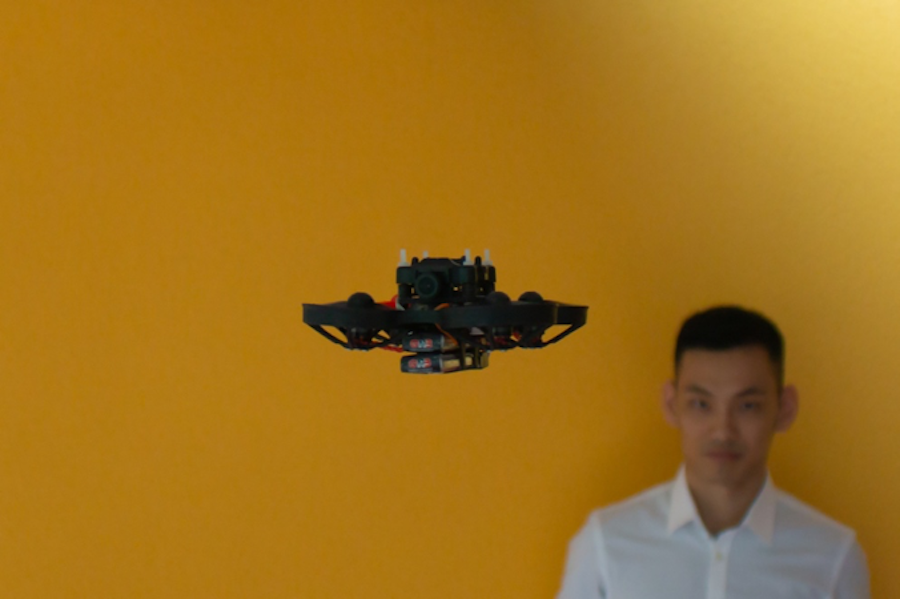
With its 72 grams and 10 cm diameter, the modified “Trashcan” drone is currently the smallest autonomous racing drone in the world. In the background, Shuo Li, PhD student at working on autonomous drone racing at the MAVLab.
A 72-gram autonomous racing drone
Here, we report on how we made a tiny autonomous racing drone fly through a racing track with on average 2 m/s, which is competitive with other, larger state-of-the-art autonomous racing drones.
The drone, which is a modified Eachine “Trashcan”, is 10 cm in diameter and weighs 72 grams. This weight includes a 17-gram JeVois smart-camera, which consists of a single, rolling shutter CMOS camera, a 4-core ARM v7 1.34 GHz processor with 256 MB RAM, and a 2-core Mali GPU. Although limited compared to the processors used on other drones, we consider it as more than powerful enough: With the algorithms we explain below, the drone actually only uses a single CPU core. The JeVois camera communicates with a 4.2gram Crazybee F4 Pro Flight Controller running Paparazzi autopilot, via the MAVLink communication protocol. Both the JeVois code and Paparazzi code is open source and available to the community.
An important characteristic of our approach to drone racing is that we do not rely on accurate, but computationally expensive methods for visual Simultaneous Localization And Mapping (SLAM) or Visual Inertial Odometry (VIO). Instead, we focus on having the drone predict its motion as good as possible with an efficient prediction model and correct any drift of the model with vision-based gate detections.
Prediction
A typical prediction model would involve the integration of the accelerometer readings. However, on small drones the Inertial Measurement Unit (IMU) is subject to a lot of vibration, leading to noisier accelerometer readings. Integrating such noisy measurements quickly leads to an enormous drift in both the velocity and position estimates of the drone. Therefore, we have opted for a simpler solution, in which the IMU is only used to determine the attitude of the drone. This attitude can then be used to predict the forward acceleration, as illustrated in the figure below. If one assumes the drone to fly at a constant height, the force in the z-direction has to equal the gravity force. Given a specific pitch angle, this relation leads to a specific forward force due to the thrust. The prediction model then updates the velocity based on this predicted forward force and the expected drag force given the estimated velocity.
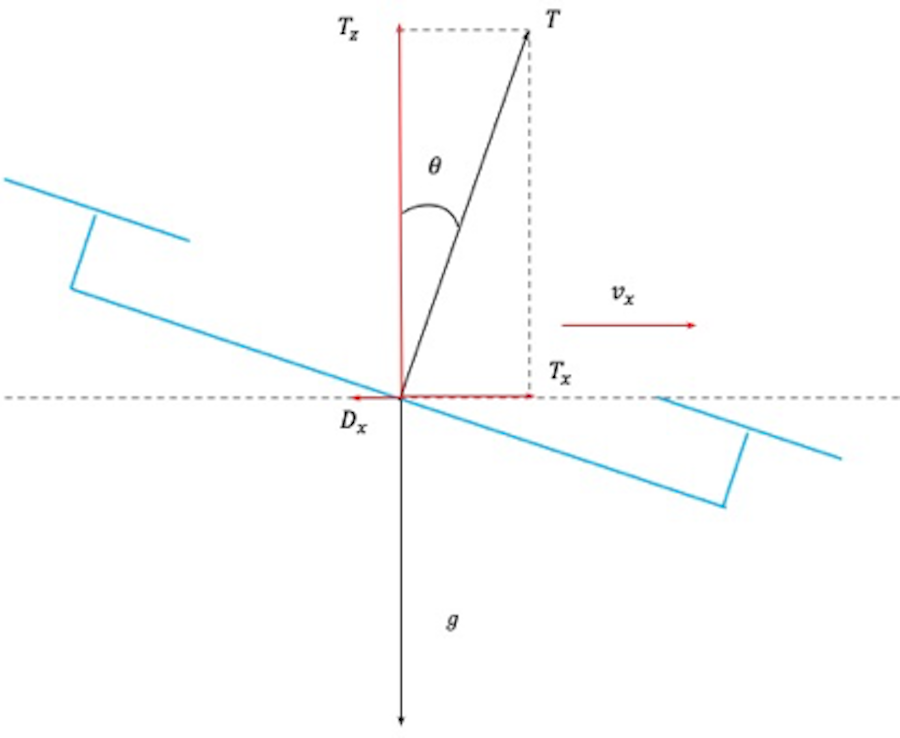
Vision-based corrections
The prediction model is corrected with the help of vision-based position measurements. First, a snake-gate algorithm is used to detect the colored gate in the image. This algorithm is extremely efficient, as it only processes a small portion of the image’s pixels. It samples random image locations and when it finds the right color, it starts following it around to determine the shape. After a detection, the known size of the gate is used to determine the drone’s relative position to the gate (see the figure below). This is a standard perspective-N-point problem. The output of this process is a relative position to a gate. Subsequently, we figure out which gate on the racing track is most likely in our view, and transform the relative position to the gate to a global position measurement. Since our vision process often outputs quite precise position estimates but sometimes also produces significant outliers, we do not use a Kalman filter but a Moving Horizon Estimator for the state estimation. This leads to much more robust position and velocity estimates in the presence of outliers.
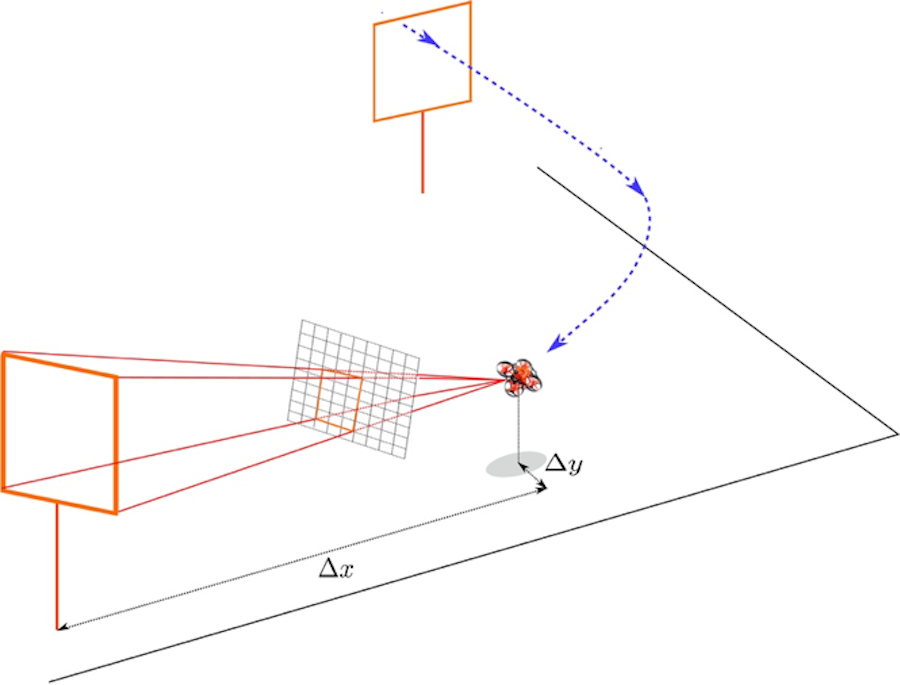
Racing performance and future steps
The drone used the newly developed algorithms to race along a 4-gate race track in TU Delft’s Cyberzoo. It can fly multiple laps at an average speed of 2 m/s, which is competitive with larger, state-of-the-art autonomous racing drones (see the video at the top). Thanks to the central role of gate detections in the drone’s algorithms, the drone can cope with moderate displacements of the gates.
Possible future directions of research are to make the drone smaller and fly faster. In principle, being small is an advantage, since the gates are relatively bigger. This allows the drone to choose its trajectory more freely than a big drone, which may allow for faster trajectories. In order to better exploit this characteristic, we would have to fit optimal control algorithms into the onboard processing. Moreover, we want to make the vision algorithms more robust – as the current color-based snake gate algorithm is quite dependent on lighting conditions. An obvious option here is to start using deep neural networks, which would have to fit within the dual-core Mali GPU on the JeVois.
Arxiv article: Visual Model-predictive Localization for Computationally Efficient Autonomous Racing of a 72-gram Drone, Shuo Li, Erik van der Horst, Philipp Duernay, Christophe De Wagter, Guido C.H.E. de Croon.
The little robot that could
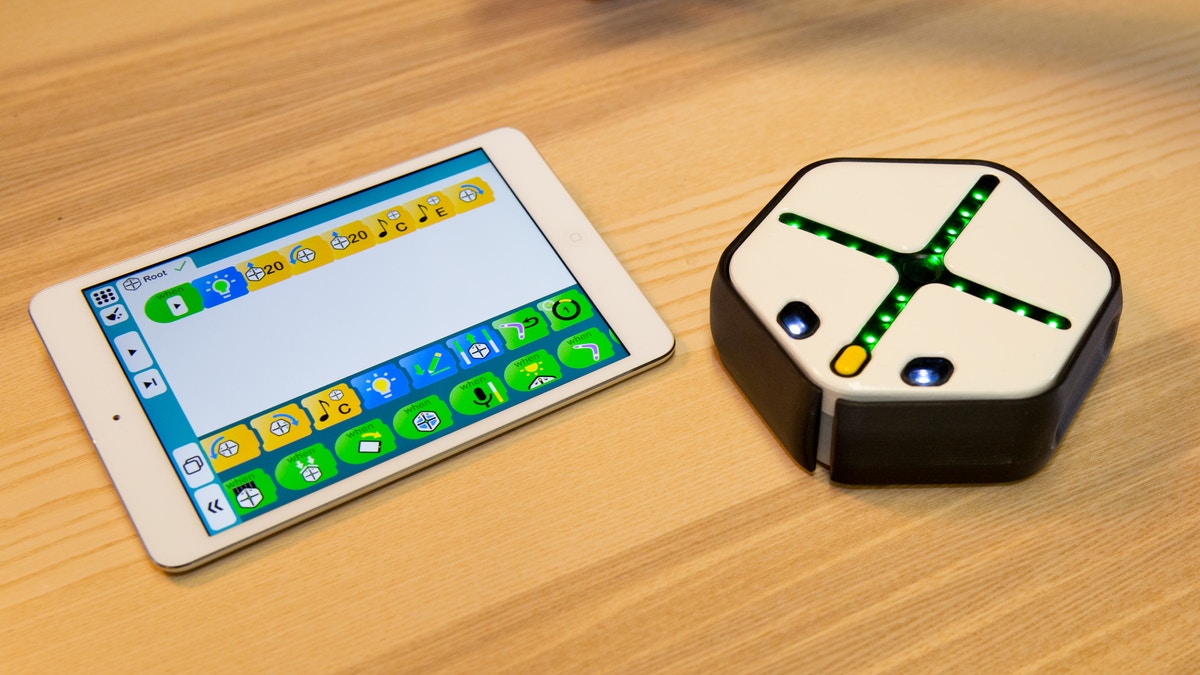
iRobot Corp. announced its acquisition of Root Robotics, Inc., whose educational Root coding robot got its start as a summer research project at the Wyss Institute for Biologically Inspired Engineering in 2011 and subsequently developed into a robust learning tool that is being used in over 500 schools to teach children between the ages of four and twelve how to code in an engaging, intuitive way. iRobot plans to incorporate the Root robot into its growing portfolio of educational robot products, and continue the work of scaling up production and expanding Root’s programming content that began when Root Robotics was founded by former Wyss Institute members in 2017.
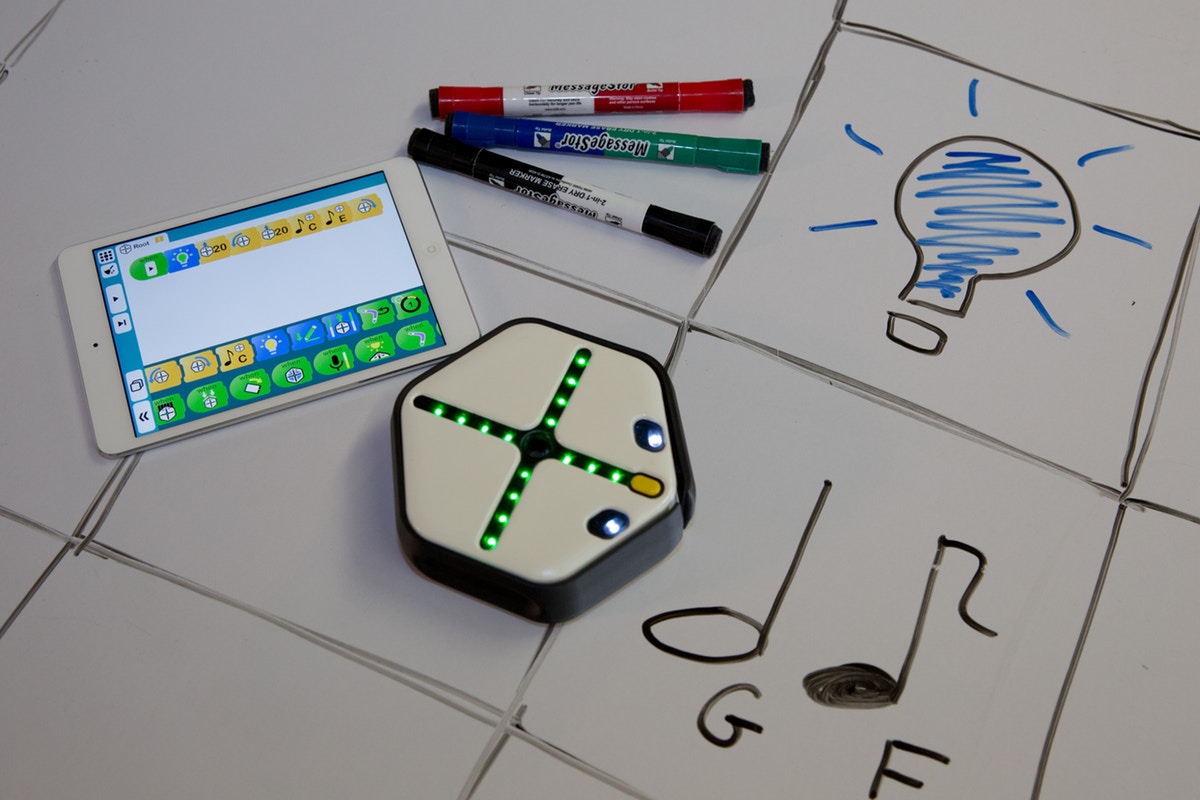
“We’re honored that we got to see a Wyss Institute technology go from its earliest stages to where we are today, with the opportunity to make a gigantic impact on the world,” said Zivthan Dubrovsky, former Bioinspired Robotics Platform Lead at the Wyss Institute and co-founder of Root Robotics who is now the General Manager of Educational Robots at iRobot. “We’re excited to see how this new chapter in Root’s story can further amplify our mission of making STEM education accessible to students of any age in any classroom around the world.”
Root began in the lab of Wyss Core Faculty Member and Bioinspired Robotics Platform co-lead Radhika Nagpal, Ph.D., who was investigating the idea of robots that could climb metal structures using magnetic wheels. “Most whiteboards in classrooms are backed with metal, so I thought it would be wonderful if a robot could automatically erase the whiteboard as I was teaching – ironically, we referred to it as a ‘Roomba® for whiteboards,’ because many aspects were directly inspired by iRobot’s Roomba at the time,” said Nagpal, who is also the Fred Kavli Professor of Computer Science at Harvard’s John A. Paulson School of Engineering and Applied Sciences (SEAS). “Once we had a working prototype, the educational potential of this robot was immediately obvious. If it could be programmed to detect ink, navigate to it, and erase it, then it could be used to teach students about coding algorithms of increasing complexity.”
That prototype was largely built by Raphael Cherney, first as a Research Engineer in Nagpal’s group at Harvard in 2011, and then beginning in 2013 when he was hired to work on developing Root full-time along with Dubrovsky and other members of the Wyss Institute. “When Raphael and Radhika pitched me the idea of Root, I fell in love with it immediately,” said Dubrovsky. “My three daughters were all very young at the time and I wanted them to have exposure to STEM concepts like coding and engineering, but I was frustrated by the lack of educational systems that were designed for children their age. The idea of being able to create that for them was really what motivated me to throw all my weight behind the project.”
Under Cherney and Dubrovsky’s leadership, Root’s repertoire expanded to include drawing shapes on the whiteboard as it wheeled around, navigating through obstacles drawn on the whiteboard, playing music, and more. The team also developed Root’s coding interface, which has three levels of increasing complexity that are designed to help students from preschool to high school easily grasp the concepts of programming and use them to create their own projects. “The tangible nature of a robot really brings the code to life, because the robot is ‘real’ in a way that code isn’t – you can watch it physically carrying out the instructions that you’ve programmed into it,” said Cherney, who co-founded Root Robotics and is now a Principal Systems Engineer at iRobot. “It helps turn coding into a social activity, especially for kids, as they learn to work in teams and see coding as a fun and natural thing to do.”
Over the next three years the team iterated on Root’s prototype and began testing it in classrooms in and around Boston, getting feedback from students and teachers to get the robot closer to its production-ready form. “Robots are very hard to build, and the support we had from the Wyss Institute let us do it right, instead of just fast,” said Cherney. “We were able to develop Root from a prototype to a product that worked in schools and was doing what we envisioned, and the whole process was much smoother than it would have been if we had just been a team working in a garage.”
By 2016, they felt ready for commercialization. They ran a Kickstarter® campaign as a market test to see if they had a viable consumer business, and raised nearly $400,000 from almost 2,000 backers, far exceeding their target of $250,000. Buoyed by this vote of confidence from potential customers, Dubrovsky and Cherney left the Wyss Institute in the summer of 2017 to co-found Root Robotics with Nagpal serving as Scientific Advisor and $2.5 million in seed funds, and a license from Harvard’s Office of Technology Development. While most of their time at the Wyss Institute was spent getting the robot right, the company focused on getting the content of Root’s programming app up to par, setting up a classroom in their office and inviting students to come try out the robot, then updating their content with insights learned from those experiences.
Once they achieved their vision for three different levels of programming targeting students of different ages, they shipped Root robots to their Kickstarter backers and made it available for purchase on their website in September 2018. Since then, over a million coding projects have been run on the Root app. “What’s been most rewarding for me personally is seeing my kids take Root to their classrooms and show their teachers and their peers what they’ve been able to make a robot do. Getting to see them problem-solve and iterate and then achieve something they’re proud of is priceless,” said Dubrovsky. “I’ve been pleasantly surprised by seeing people come up with new things to do with the robot that we never thought of,” added Cherney. “The way it seems to immediately unlock creativity is beautiful and inspiring.”
The Root robot has tremendous value as a tool for teaching students not only coding, but also concepts of AI, engineering and autonomous robots, all of which are very important for our future.
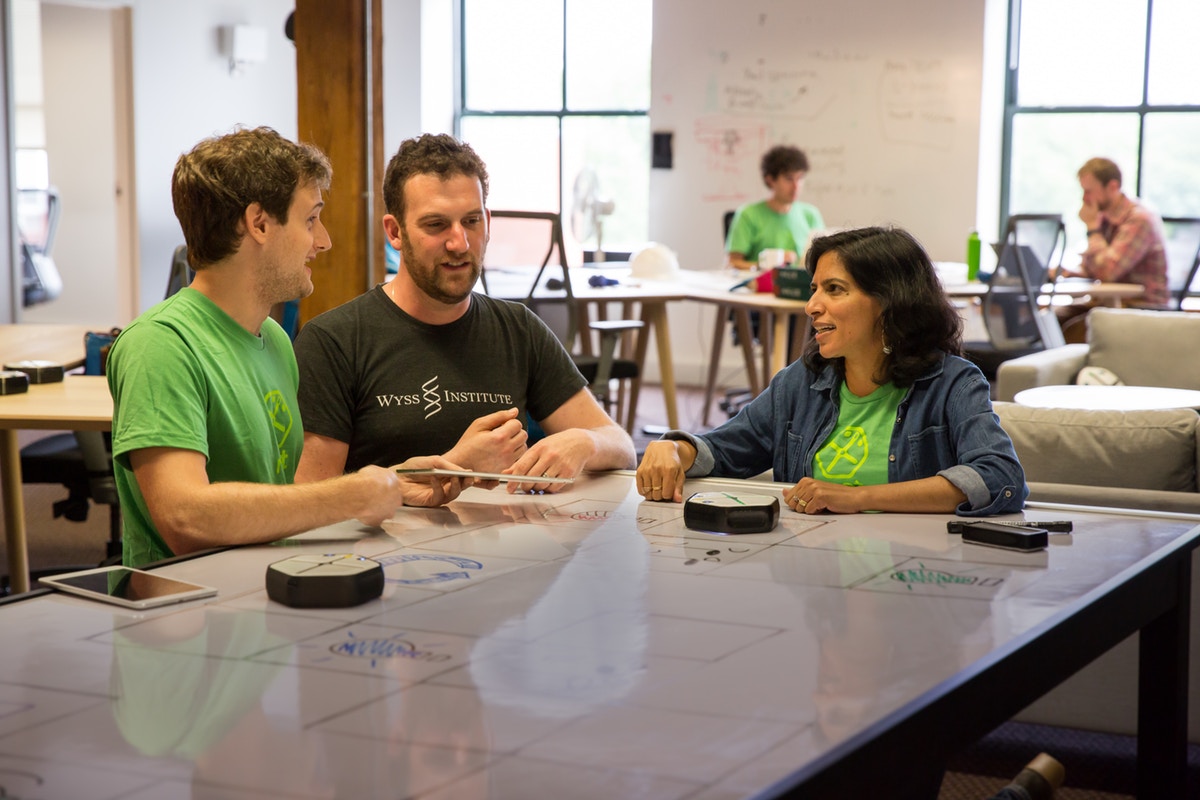
“One of the things that really attracted us to Root was that it was designed as an education product from the ground up, which fits perfectly with our own deep passion for using robots as a way of turbo charging STEM education,” said Colin Angle, chairman and CEO of iRobot. “The Root robot has tremendous value as a tool for teaching students not only coding, but also concepts of AI, engineering and autonomous robots, all of which are very important for our future.”
Nagpal is still sometimes floored by the fact that what started as an idea for a simple whiteboard-erasing robot ended up developing into such a robust teaching tool. “Without the Wyss Institute, I would not have even thought to try and commercialize this idea,” she said. “It supported an amazing team of engineers in creating and testing Root over several years, which allowed us to be able to raise the funds to launch the company with a product that was so well-developed that it now has the potential to really scale up and make a big difference in the world.”
“Root Robotics is one of the great success stories to come out of the Wyss Institute, partially because of how quickly the team recognized its potential impact and focused on de-risking it both technically and commercially,” said Wyss Founding Director Donald Ingber, M.D., Ph.D. “It was fantastic to see Root take root at the Institute, and we are immensely proud of them and their ability to develop a technology that can truly bring about positive change in our world by targeting children who are the creators and visionaries of tomorrow.” Ingber is also the Judah Folkman Professor of Vascular Biology at Harvard Medical School and the Vascular Biology Program at Boston Children’s Hospital, as well as Professor of Bioengineering at SEAS.
Spotting objects amid clutter
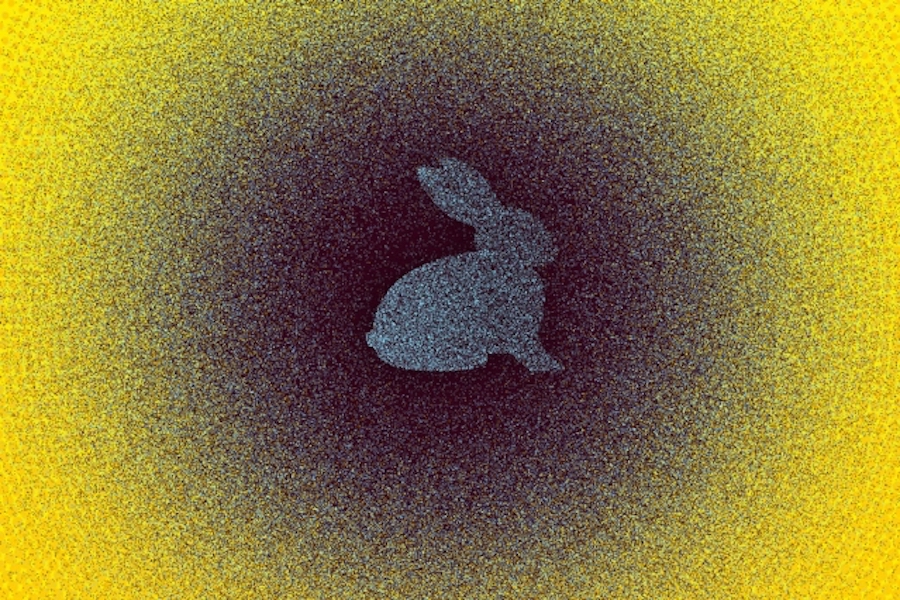
Image: Christine Daniloff, MIT
A new MIT-developed technique enables robots to quickly identify objects hidden in a three-dimensional cloud of data, reminiscent of how some people can make sense of a densely patterned “Magic Eye” image if they observe it in just the right way.
Robots typically “see” their environment through sensors that collect and translate a visual scene into a matrix of dots. Think of the world of, well, “The Matrix,” except that the 1s and 0s seen by the fictional character Neo are replaced by dots — lots of dots — whose patterns and densities outline the objects in a particular scene.
Conventional techniques that try to pick out objects from such clouds of dots, or point clouds, can do so with either speed or accuracy, but not both.
With their new technique, the researchers say a robot can accurately pick out an object, such as a small animal, that is otherwise obscured within a dense cloud of dots, within seconds of receiving the visual data. The team says the technique can be used to improve a host of situations in which machine perception must be both speedy and accurate, including driverless cars and robotic assistants in the factory and the home.
“The surprising thing about this work is, if I ask you to find a bunny in this cloud of thousands of points, there’s no way you could do that,” says Luca Carlone, assistant professor of aeronautics and astronautics and a member of MIT’s Laboratory for Information and Decision Systems (LIDS). “But our algorithm is able to see the object through all this clutter. So we’re getting to a level of superhuman performance in localizing objects.”
Carlone and graduate student Heng Yang will present details of the technique later this month at the Robotics: Science and Systems conference in Germany.
“Failing without knowing”
Robots currently attempt to identify objects in a point cloud by comparing a template object — a 3-D dot representation of an object, such as a rabbit — with a point cloud representation of the real world that may contain that object. The template image includes “features,” or collections of dots that indicate characteristic curvatures or angles of that object, such the bunny’s ear or tail. Existing algorithms first extract similar features from the real-life point cloud, then attempt to match those features and the template’s features, and ultimately rotate and align the features to the template to determine if the point cloud contains the object in question.
But the point cloud data that streams into a robot’s sensor invariably includes errors, in the form of dots that are in the wrong position or incorrectly spaced, which can significantly confuse the process of feature extraction and matching. As a consequence, robots can make a huge number of wrong associations, or what researchers call “outliers” between point clouds, and ultimately misidentify objects or miss them entirely.
Carlone says state-of-the-art algorithms are able to sift the bad associations from the good once features have been matched, but they do so in “exponential time,” meaning that even a cluster of processing-heavy computers, sifting through dense point cloud data with existing algorithms, would not be able to solve the problem in a reasonable time. Such techniques, while accurate, are impractical for analyzing larger, real-life datasets containing dense point clouds.
Other algorithms that can quickly identify features and associations do so hastily, creating a huge number of outliers or misdetections in the process, without being aware of these errors.
“That’s terrible if this is running on a self-driving car, or any safety-critical application,” Carlone says. “Failing without knowing you’re failing is the worst thing an algorithm can do.”
A relaxed view
Yang and Carlone instead devised a technique that prunes away outliers in “polynomial time,” meaning that it can do so quickly, even for increasingly dense clouds of dots. The technique can thus quickly and accurately identify objects hidden in cluttered scenes.
The MIT-developed technique quickly and smoothly matches objects to those hidden in dense point clouds (left), versus existing techniques (right) that produce incorrect, disjointed matches. Gif: Courtesy of the researchers
The researchers first used conventional techniques to extract features of a template object from a point cloud. They then developed a three-step process to match the size, position, and orientation of the object in a point cloud with the template object, while simultaneously identifying good from bad feature associations.
The team developed an “adaptive voting scheme” algorithm to prune outliers and match an object’s size and position. For size, the algorithm makes associations between template and point cloud features, then compares the relative distance between features in a template and corresponding features in the point cloud. If, say, the distance between two features in the point cloud is five times that of the corresponding points in the template, the algorithm assigns a “vote” to the hypothesis that the object is five times larger than the template object.
The algorithm does this for every feature association. Then, the algorithm selects those associations that fall under the size hypothesis with the most votes, and identifies those as the correct associations, while pruning away the others. In this way, the technique simultaneously reveals the correct associations and the relative size of the object represented by those associations. The same process is used to determine the object’s position.
The researchers developed a separate algorithm for rotation, which finds the orientation of the template object in three-dimensional space.
To do this is an incredibly tricky computational task. Imagine holding a mug and trying to tilt it just so, to match a blurry image of something that might be that same mug. There are any number of angles you could tilt that mug, and each of those angles has a certain likelihood of matching the blurry image.
Existing techniques handle this problem by considering each possible tilt or rotation of the object as a “cost” — the lower the cost, the more likely that that rotation creates an accurate match between features. Each rotation and associated cost is represented in a topographic map of sorts, made up of multiple hills and valleys, with lower elevations associated with lower cost.
But Carlone says this can easily confuse an algorithm, especially if there are multiple valleys and no discernible lowest point representing the true, exact match between a particular rotation of an object and the object in a point cloud. Instead, the team developed a “convex relaxation” algorithm that simplifies the topographic map, with one single valley representing the optimal rotation. In this way, the algorithm is able to quickly identify the rotation that defines the orientation of the object in the point cloud.
With their approach, the team was able to quickly and accurately identify three different objects — a bunny, a dragon, and a Buddha — hidden in point clouds of increasing density. They were also able to identify objects in real-life scenes, including a living room, in which the algorithm quickly was able to spot a cereal box and a baseball hat.
Carlone says that because the approach is able to work in “polynomial time,” it can be easily scaled up to analyze even denser point clouds, resembling the complexity of sensor data for driverless cars, for example.
“Navigation, collaborative manufacturing, domestic robots, search and rescue, and self-driving cars is where we hope to make an impact,” Carlone says.
This research was supported in part by the Army Research Laboratory, the Office of Naval Research, and the Google Daydream Research Program.
Tackling sustainability and urbanization with AI-enabled furniture
At the turn of the twentieth century, the swelling populations of newly arrived immigrants in New York City’s Lower East Side reached a boiling point, forcing the City to pass the 1901 Tenement House Act. Recalling this legislation, New York City’s Mayor’s Office recently responded to its own modern housing crisis by enabling developers for the first time to build affordable micro-studio apartments of 400 square feet. One of the primary drivers of allocating tens of thousands of new micro-units is the adoption of innovative design and construction technologies that enable modular and flexible housing options. As Mayor de Blasio affirmed, “Housing New York 2.0 commits us to creating 25,000 affordable homes a year and 300,000 homes by 2026. Making New York a fairer city for today and for future generations depends on it.”
Urban space density is not just a New York City problem, but a world health concern. According to the United Nations, more than half of the Earth’s population currently resides in cities and this is projected to climb to close to three-quarters by 2050. In response to this alarming trend the UN drafted the 2030 Agenda for Sustainable Development. Stressing the importance of such an effort, UN Deputy Secretary Amina J. Mohammed declared, “It is clear that it is in cities where the battle for sustainability will be won or lost. Cities are the organizing mechanisms of the twenty-first century. They are where young people in all parts of the world flock to develop new skills, attain new jobs, and find opportunities in which to innovate and create their futuresThe 2030 Agenda for Sustainable Development is the most ambitious agenda ever set forth for humanity.”
Absent from the UN study is utilizing mechatronics to address the challenges of urbanization. For example, robots have been deployed on construction sites in China to rapidly print building materials. There are also a handful of companies utilizing machines to cost effectively produce modular homes with the goal of replacing mud-huts and sheet metal shanties. However, the progress of automating low-to-middle income housing has been slow going until this week. Ikea, the world’s largest furniture retailer which specializes in low cost decorating solutions, announced on Tuesday the launch of Rognan – a morphing robotic furniture system for the micro-home. Collaborating with the Swedish design powerhouse is hardware startup, Ori Living. The MIT spin-out first introduced its chameleon-changing furniture platform two years ago with an expandable wardrobe that quickly shifted from bookcase/home office to walk-in closet at the touch of a button. Today such systems can be bought through the company’s website for a price upwards of $5,000. It is expected that the partnership with IKEA will bring enormous economies of scale with the mass production of its products.
The first markets targeted by IKEA next year for Rognan are the cramped neighborhoods of Hong Kong and Japan, where the average citizen lives in 160 square feet. Seana Strawn, IKEA’s product developer for new innovations, explains “Instead of making the furniture smaller, we transform the furniture to the function that you need at that time When you sleep, you do not need your sofa. When you use your wardrobe, you do not need your bed etc.”
Ori founder, Hasier Larrea, elaborates on his use of machine learning to size the space to the occupants requirements. “Every floor is different, so you need a product that’s smart enough to know this, and make a map of the floor,” describes Larrea. By using sensors to create an image of the space, the robot seamlessly transforms from closet-to-bed-to-desk-to-media center. To better understand the marketability of such a system, I polled a close friend who analyzes such innovations for a large Wall Street bank. This potential customer remarked that he will wait to purchase his own Rognan until it can anticipate his living habits, automatically sense when it is time for work, play or bed.
Ori’s philosophy is enabling people to “live large in a small footprint.” Larrea professes that the only way to combat urbanization is thinking differently. As the founder exclaims, “We cannot keep designing spaces the same way we’ve been designing spaces 20 years ago, or keep using all the same furniture we were using in homes that were twice the size, or three times the size. We need to start thinking about furniture that adapts to us, and not the other way around.” Larrea’s credo can be heard in the words of former Tesla technologist Sankarshan Murthy who aims to revolutionize interior design with robotic ceiling dropping storage furniture. Murthy’s startup, Bubblebee Spaces, made news last April with the announcement of a $4.3 million funding round led by Loup Ventures. Similar to a Broadway set change, Bubblebee lowers and hoists up wooden cases on an as needed basis, complete with an iPhone or iPad controller. “Instead of square feet you start looking at real estate in volume. You are already paying for all this air and ceiling space you are not using. We unlock that for you,” brags Murthy. Ori is also working on a modern Murphy Bed that lowers from ceiling, as the company’s press release stated last November its newest product, “a bed that seamlessly lowers from the ceiling, or lifts to the ceiling to reveal a stylish sofa” all at the beckon of one’s Alexa device.
In 1912, William Murphy received his patent for the “Disappearing Bed. Today’s robotic furniture, now validated by the likes of Ikea, could be the continuation of his vision. Several years ago, MIT student Daniel Leithinger first unveiled a shape shifting table. As Leithinger reminisces, “We were inspired by those pinscreen toys where you press your hand on one end, and it shows on the other side.” While it was never intended to be commercialized, the inventor was blown away by the emails he received. “One person said we should apply it to musical interfaces and another person said it would be great to use to help blind children understand art and other things. These are things we didn’t even think about,” shares Leithinger. As Ori and Bubblebee are working diligently to replace old couch springs for new gears and actuators, the benefits of such technology are sure to go beyond just better storage as we enter the new age of the AI Home.
1000x faster data augmentation
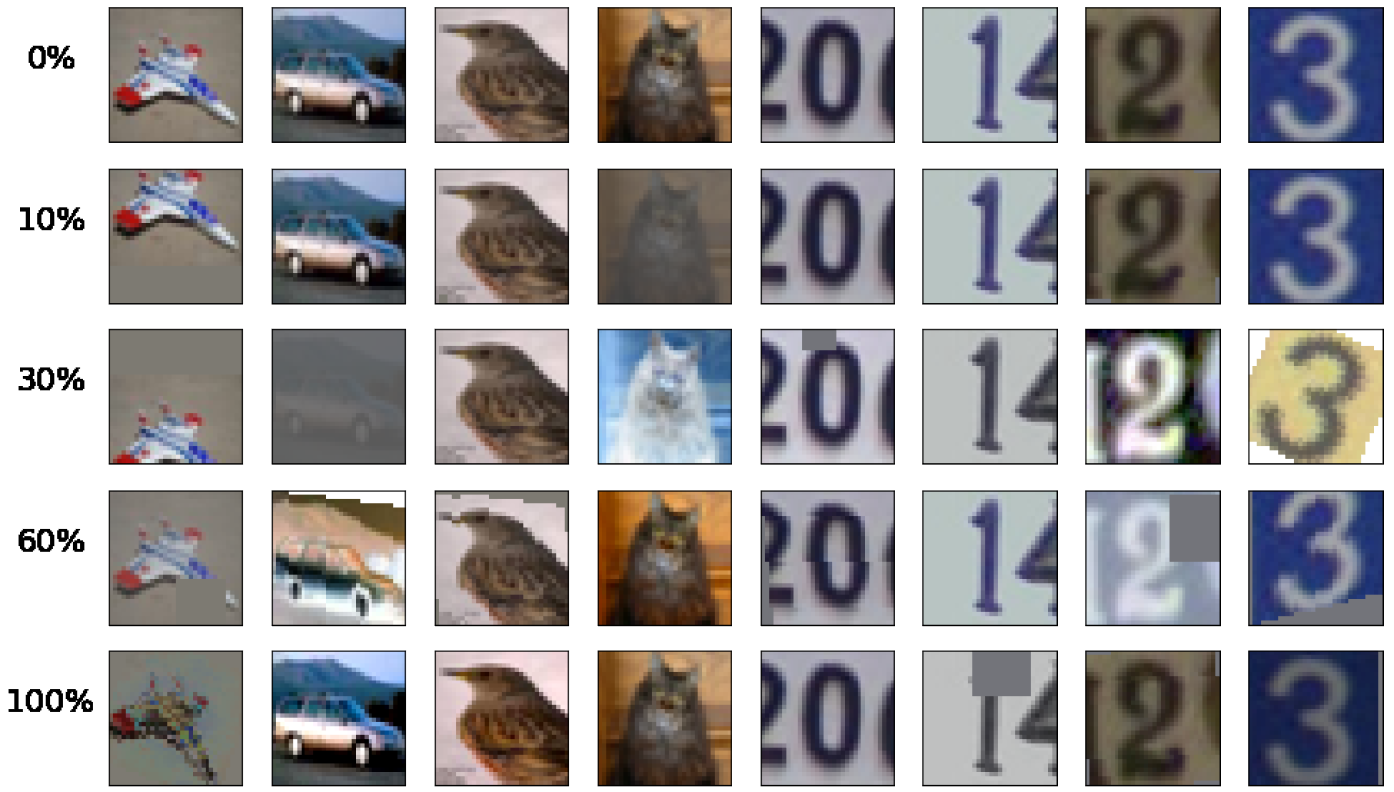
In this blog post we introduce Population Based Augmentation (PBA), an algorithm that quickly and efficiently learns a state-of-the-art approach to augmenting data for neural network training. PBA matches the previous best result on CIFAR and SVHN but uses one thousand times less compute, enabling researchers and practitioners to effectively learn new augmentation policies using a single workstation GPU. You can use PBA broadly to improve deep learning performance on image recognition tasks.
We discuss the PBA results from our recent paper and then show how to easily run PBA for yourself on a new data set in the Tune framework.
Why should you care about data augmentation?
Recent advances in deep learning models have been largely attributed to the quantity and diversity of data gathered in recent years. Data augmentation is a strategy that enables practitioners to significantly increase the diversity of data available for training models, without actually collecting new data. Data augmentation techniques such as cropping, padding, and horizontal flipping are commonly used to train large neural networks. However, most approaches used in training neural networks only use basic types of augmentation. While neural network architectures have been investigated in depth, less focus has been put into discovering strong types of data augmentation and data augmentation policies that capture data invariances.
An image of the number “3” in original form and with basic augmentations applied.
Recently, Google has been able to push the state-of-the-art accuracy on datasets such as CIFAR-10 with AutoAugment, a new automated data augmentation technique. AutoAugment has shown that prior work using just applying a fixed set of transformations like horizontal flipping or padding and cropping leaves potential performance on the table. AutoAugment introduces 16 geometric and color-based transformations, and formulates an augmentation policy that selects up to two transformations at certain magnitude levels to apply to each batch of data. These higher performing augmentation policies are learned by training models directly on the data using reinforcement learning.
What’s the catch?
AutoAugment is a very expensive algorithm which requires training 15,000 models to convergence to generate enough samples for a reinforcement learning based policy. No computation is shared between samples, and it costs 15,000 NVIDIA Tesla P100 GPU hours to learn an ImageNet augmentation policy and 5,000 GPU hours to learn an CIFAR-10 one. For example, if using Google Cloud on-demand P100 GPUs, it would cost about \$7,500 to discover a CIFAR policy, and \$37,500 to discover an ImageNet one! Therefore, a more common use case when training on a new dataset would be to transfer a pre-existing published policy, which the authors show works relatively well.
Population Based Augmentation
Our formulation of data augmentation policy search, Population Based Augmentation (PBA), reaches similar levels of test accuracy on a variety of neural network models while utilizing three orders of magnitude less compute. We learn an augmentation policy by training several copies of a small model on CIFAR-10 data, which takes five hours using a NVIDIA Titan XP GPU. This policy exhibits strong performance when used for training from scratch on larger model architectures and with CIFAR-100 data.
Relative to the several days it takes to train large CIFAR-10 networks to convergence, the cost of running PBA beforehand is marginal and significantly enhances results. For example, training a PyramidNet model on CIFAR-10 takes over 7 days on a NVIDIA V100 GPU, so learning a PBA policy adds only 2% precompute training time overhead. This overhead would be even lower, under 1%, for SVHN.
CIFAR-10 test set error between PBA, AutoAugment, and the baseline which only uses horizontal flipping, padding, and cropping, on WideResNet, Shake-Shake, and PyramidNet+ShakeDrop models. PBA is significantly better than the baseline and on-par with AutoAugment.
PBA leverages the Population Based Training algorithm to generate an augmentation policy schedule which can adapt based on the current epoch of training. This is in contrast to a fixed augmentation policy that applies the same transformations independent of the current epoch number.
This allows an ordinary workstation user to easily experiment with the search algorithm and augmentation operations. One interesting use case would be to introduce new augmentation operations, perhaps targeted towards a particular dataset or image modality, and be able to quickly produce a tailored, high performing augmentation schedule. Through ablation studies, we have found that the learned hyperparameters and schedule order are important for good results.
How is the augmentation schedule learned?
We use Population Based Training with a population of 16 small WideResNet models. Each worker in the population will learn a different candidate hyperparameter schedule. We transfer the best performing schedule to train larger models from scratch, from which we derive our test error metrics.
Overview of Population Based Training, which discovers hyperparameter schedules by training a population of neural networks. It combines random search (explore) with the copying of model weights from high performing workers (exploit). Source
The population models are trained on the target dataset of interest starting with all augmentation hyperparameters set to 0 (no augmentations applied). At frequent intervals, an “exploit-and-explore” process “exploits” high performing workers by copying their model weights to low performing workers, and then “explores” by perturbing the hyperparameters of the worker. Through this process, we are able to share compute heavily between the workers and target different augmentation hyperparameters at different regions of training. Thus, PBA is able to avoid the cost of training thousands of models to convergence in order to reach high performance.
Example and Code
We leverage Tune’s built-in implementation of PBT to make it straightforward to use PBA.
import ray
def explore(config):
"""Custom PBA function to perturb augmentation hyperparameters."""
...
ray.init()
pbt = ray.tune.schedulers.PopulationBasedTraining(
time_attr="training_iteration",
reward_attr="val_acc",
perturbation_interval=3,
custom_explore_fn=explore)
train_spec = {...} # Things like file paths, model func, compute.
ray.tune.run_experiments({"PBA": train_spec}, scheduler=pbt)
We call Tune’s implementation of PBT with our custom exploration function. This will create 16 copies of our WideResNet model and train them time-multiplexed. The policy schedule used by each copy is saved to disk and can be retrieved after termination to use for training new models.
You can run PBA by following the README at: https://github.com/arcelien/pba. On a Titan XP, it only requires one hour to learn a high performing augmentation policy schedule on the SVHN dataset. It is also easy to use PBA on a custom dataset as well: simply define a new dataloader and everything else falls into place.
Big thanks to Daniel Rothchild, Ashwinee Panda, Aniruddha Nrusimha, Daniel Seita, Joseph Gonzalez, and Ion Stoica for helpful feedback while writing this post. Feel free to get in touch with us on Github!
This post is based on the following paper to appear in ICML 2019 as an oral presentation:
Chip design drastically reduces energy needed to compute with light
A new photonic chip design drastically reduces energy needed to compute with light, with simulations suggesting it could run optical neural networks 10 million times more efficiently than its electrical counterparts.
Image: courtesy of the researchers, edited by MIT News
By Rob Matheson
MIT researchers have developed a novel “photonic” chip that uses light instead of electricity — and consumes relatively little power in the process. The chip could be used to process massive neural networks millions of times more efficiently than today’s classical computers do.
Neural networks are machine-learning models that are widely used for such tasks as robotic object identification, natural language processing, drug development, medical imaging, and powering driverless cars. Novel optical neural networks, which use optical phenomena to accelerate computation, can run much faster and more efficiently than their electrical counterparts.
But as traditional and optical neural networks grow more complex, they eat up tons of power. To tackle that issue, researchers and major tech companies — including Google, IBM, and Tesla — have developed “AI accelerators,” specialized chips that improve the speed and efficiency of training and testing neural networks.
For electrical chips, including most AI accelerators, there is a theoretical minimum limit for energy consumption. Recently, MIT researchers have started developing photonic accelerators for optical neural networks. These chips perform orders of magnitude more efficiently, but they rely on some bulky optical components that limit their use to relatively small neural networks.
In a paper published in Physical Review X, MIT researchers describe a new photonic accelerator that uses more compact optical components and optical signal-processing techniques, to drastically reduce both power consumption and chip area. That allows the chip to scale to neural networks several orders of magnitude larger than its counterparts.
Simulated training of neural networks on the MNIST image-classification dataset suggest the accelerator can theoretically process neural networks more than 10 million times below the energy-consumption limit of traditional electrical-based accelerators and about 1,000 times below the limit of photonic accelerators. The researchers are now working on a prototype chip to experimentally prove the results.
“People are looking for technology that can compute beyond the fundamental limits of energy consumption,” says Ryan Hamerly, a postdoc in the Research Laboratory of Electronics. “Photonic accelerators are promising … but our motivation is to build a [photonic accelerator] that can scale up to large neural networks.”
Practical applications for such technologies include reducing energy consumption in data centers. “There’s a growing demand for data centers for running large neural networks, and it’s becoming increasingly computationally intractable as the demand grows,” says co-author Alexander Sludds, a graduate student in the Research Laboratory of Electronics. The aim is “to meet computational demand with neural network hardware … to address the bottleneck of energy consumption and latency.”
Joining Sludds and Hamerly on the paper are: co-author Liane Bernstein, an RLE graduate student; Marin Soljacic, an MIT professor of physics; and Dirk Englund, an MIT associate professor of electrical engineering and computer science, a researcher in RLE, and head of the Quantum Photonics Laboratory.
Compact design
Neural networks process data through many computational layers containing interconnected nodes, called “neurons,” to find patterns in the data. Neurons receive input from their upstream neighbors and compute an output signal that is sent to neurons further downstream. Each input is also assigned a “weight,” a value based on its relative importance to all other inputs. As the data propagate “deeper” through layers, the network learns progressively more complex information. In the end, an output layer generates a prediction based on the calculations throughout the layers.
All AI accelerators aim to reduce the energy needed to process and move around data during a specific linear algebra step in neural networks, called “matrix multiplication.” There, neurons and weights are encoded into separate tables of rows and columns and then combined to calculate the outputs.
In traditional photonic accelerators, pulsed lasers encoded with information about each neuron in a layer flow into waveguides and through beam splitters. The resulting optical signals are fed into a grid of square optical components, called “Mach-Zehnder interferometers,” which are programmed to perform matrix multiplication. The interferometers, which are encoded with information about each weight, use signal-interference techniques that process the optical signals and weight values to compute an output for each neuron. But there’s a scaling issue: For each neuron there must be one waveguide and, for each weight, there must be one interferometer. Because the number of weights squares with the number of neurons, those interferometers take up a lot of real estate.
“You quickly realize the number of input neurons can never be larger than 100 or so, because you can’t fit that many components on the chip,” Hamerly says. “If your photonic accelerator can’t process more than 100 neurons per layer, then it makes it difficult to implement large neural networks into that architecture.”
The researchers’ chip relies on a more compact, energy efficient “optoelectronic” scheme that encodes data with optical signals, but uses “balanced homodyne detection” for matrix multiplication. That’s a technique that produces a measurable electrical signal after calculating the product of the amplitudes (wave heights) of two optical signals.
Pulses of light encoded with information about the input and output neurons for each neural network layer — which are needed to train the network — flow through a single channel. Separate pulses encoded with information of entire rows of weights in the matrix multiplication table flow through separate channels. Optical signals carrying the neuron and weight data fan out to grid of homodyne photodetectors. The photodetectors use the amplitude of the signals to compute an output value for each neuron. Each detector feeds an electrical output signal for each neuron into a modulator, which converts the signal back into a light pulse. That optical signal becomes the input for the next layer, and so on.
The design requires only one channel per input and output neuron, and only as many homodyne photodetectors as there are neurons, not weights. Because there are always far fewer neurons than weights, this saves significant space, so the chip is able to scale to neural networks with more than a million neurons per layer.
Finding the sweet spot
With photonic accelerators, there’s an unavoidable noise in the signal. The more light that’s fed into the chip, the less noise and greater the accuracy — but that gets to be pretty inefficient. Less input light increases efficiency but negatively impacts the neural network’s performance. But there’s a “sweet spot,” Bernstein says, that uses minimum optical power while maintaining accuracy.
That sweet spot for AI accelerators is measured in how many joules it takes to perform a single operation of multiplying two numbers — such as during matrix multiplication. Right now, traditional accelerators are measured in picojoules, or one-trillionth of a joule. Photonic accelerators measure in attojoules, which is a million times more efficient.
In their simulations, the researchers found their photonic accelerator could operate with sub-attojoule efficiency. “There’s some minimum optical power you can send in, before losing accuracy. The fundamental limit of our chip is a lot lower than traditional accelerators … and lower than other photonic accelerators,” Bernstein says.
My top three policy and governance issues in AI/ML
In preparation for a recent meeting of the WEF global AI council, we were asked the question:
What do you think are the top three policy and governance issues that face AI/ML currently?
Here are my answers.
1. For me the biggest governance issue facing AI/ML ethics is the gap between principles and practice. The hard problem the industry faces is turning good intentions into demonstrably good behaviour. In the last 2.5 years there has been a gold rush of new ethical principles in AI. Since Jan 2017 at least 22 sets of ethical principles have been published, including principles from Google, IBM, Microsoft and Intel. Yet any evidence that these principles are making a difference within those companies is hard to find – leading to a justifiable accusation of ethics-washing – and if anything the reputations of some leading AI companies are looking increasingly tarnished.
2. Like others I am deeply concerned by the acute gender imbalance in AI (estimates of the proportion of women in AI vary between ~12% and ~22%). This is not just unfair, I believe it too be positively dangerous, since it is resulting in AI products and services that reflect the values and ambitions of (young, predominantly white) men. This makes it a governance issue. I cannot help wondering if the deeply troubling rise of surveillance capitalism is not, at least in part, a consequence of male values.
3. A major policy concern is the apparently very poor quality of many of the jobs created by the large AI/ML companies. Of course the AI/ML engineers are paid exceptionally well, but it seems that there is a very large number of very poorly paid workers who, in effect, compensate for the fact that AI is not (yet) capable of identifying offensive content, nor is it able to learn without training data generated from large quantities of manually tagged objects in images, nor can conversational AI manage all queries that might be presented to it. This hidden army of piece workers, employed in developing countries by third party sub contractors and paid very poorly, are undertaking work that is at best extremely tedious (you might say robotic) and at worst psychologically very harmful; this has been called AI’s dirty little secret and should not – in my view – go unaddressed.
Autonomous boats can target and latch onto each other
MIT researchers have given their fleet of autonomous “roboats” the ability to automatically target and clasp onto each other — and keep trying if they fail. The roboats are being designed to transport people, collect trash, and self-assemble into floating structures in the canals of Amsterdam.
Courtesy of the researchers
By Rob Matheson
The city of Amsterdam envisions a future where fleets of autonomous boats cruise its many canals to transport goods and people, collect trash, or self-assemble into floating stages and bridges. To further that vision, MIT researchers have given new capabilities to their fleet of robotic boats — which are being developed as part of an ongoing project — that lets them target and clasp onto each other, and keep trying if they fail.
About a quarter of Amsterdam’s surface area is water, with 165 canals winding alongside busy city streets. Several years ago, MIT and the Amsterdam Institute for Advanced Metropolitan Solutions (AMS Institute) teamed up on the “Roboat” project. The idea is to build a fleet of autonomous robotic boats — rectangular hulls equipped with sensors, thrusters, microcontrollers, GPS modules, cameras, and other hardware — that provides intelligent mobility on water to relieve congestion in the city’s busy streets.
One of project’s objectives is to create roboat units that provide on-demand transporation on waterways. Another objective is using the roboat units to automatically form “pop-up” structures, such as foot bridges, performance stages, or even food markets. The structures could then automatically disassemble at set times and reform into target structures for different activities. Additionally, the roboat units could be used as agile sensors to gather data on the city’s infrastructure, and air and water quality, among other things.
In 2016, MIT researchers tested a roboat prototype that cruised around Amsterdam’s canals, moving forward, backward, and laterally along a preprogrammed path. Last year, researchers designed low-cost, 3-D-printed, one-quarter scale versions of the boats, which were more efficient and agile, and came equipped with advanced trajectory-tracking algorithms.
In a paper presented at the International Conference on Robotics and Automation, the researchers describe roboat units that can now identify and connect to docking stations. Control algorithms guide the roboats to the target, where they automatically connect to a customized latching mechanism with millimeter precision. Moreover, the roboat notices if it has missed the connection, backs up, and tries again.
The researchers tested the latching technique in a swimming pool at MIT and in the Charles River, where waters are rougher. In both instances, the roboat units were usually able to successfully connect in about 10 seconds, starting from around 1 meter away, or they succeeded after a few failed attempts. In Amsterdam, the system could be especially useful for overnight garbage collection. Roboat units could sail around a canal, locate and latch onto platforms holding trash containers, and haul them back to collection facilities.
“In Amsterdam, canals were once used for transportation and other things the roads are now used for. Roads near canals are now very congested — and have noise and pollution — so the city wants to add more functionality back to the canals,” says first author Luis Mateos, a graduate student in the Department of Urban Studies and Planning (DUSP) and a researcher in the MIT Senseable City Lab. “Self-driving technologies can save time, costs and energy, and improve the city moving forward.”
“The aim is to use roboat units to bring new capabilities to life on the water,” adds co-author Daniela Rus, director of the Computer Science and Artificial Intelligence Laboratory (CSAIL) and the Andrew and Erna Viterbi Professor of Electrical Engineering and Computer Science. “The new latching mechanism is very important for creating pop-up structures. Roboat does not need latching for autonomous transporation on water, but you need the latching to create any structure, whether it’s mobile or fixed.”
Joining Mateos on the paper are: Wei Wang, a joint postdoc in CSAIL and the Senseable City Lab; Banti Gheneti, a graduate student in the Department of Electrical Engineering and Computer Science; Fabio Duarte, a DUSP and Senseable City Lab research scientist; and Carlo Ratti, director of the Senseable City Lab and a principal investigator and professor of the practice in DUSP.
Making the connection
Each roboat is equipped with latching mechanisms, including ball and socket components, on its front, back, and sides. The ball component resembles a badminton shuttlecock — a cone-shaped, rubber body with a metal ball at the end. The socket component is a wide funnel that guides the ball component into a receptor. Inside the funnel, a laser beam acts like a security system that detects when the ball crosses into the receptor. That activates a mechanism with three arms that closes around and captures the ball, while also sending a feedback signal to both roboats that the connection is complete.
On the software side, the roboats run on custom computer vision and control techniques. Each roboat has a LIDAR system and camera, so they can autonomously move from point to point around the canals. Each docking station — typically an unmoving roboat — has a sheet of paper imprinted with an augmented reality tag, called an AprilTag, which resembles a simplified QR code. Commonly used for robotic applications, AprilTags enable robots to detect and compute their precise 3-D position and orientation relative to the tag.
Both the AprilTags and cameras are located in the same locations in center of the roboats. When a traveling roboat is roughly one or two meters away from the stationary AprilTag, the roboat calculates its position and orientation to the tag. Typically, this would generate a 3-D map for boat motion, including roll, pitch, and yaw (left and right). But an algorithm strips away everything except yaw. This produces an easy-to-compute 2-D plane that measures the roboat camera’s distance away and distance left and right of the tag. Using that information, the roboat steers itself toward the tag. By keeping the camera and tag perfectly aligned, the roboat is able to precisely connect.
The funnel compensates for any misalignment in the roboat’s pitch (rocking up and down) and heave (vertical up and down), as canal waves are relatively small. If, however, the roboat goes beyond its calculated distance, and doesn’t receive a feedback signal from the laser beam, it knows it has missed. “In challenging waters, sometimes roboat units at the current one-quarter scale, are not strong enough to overcome wind gusts or heavy water currents,” Mateos says. “A logic component on the roboat says, ‘You missed, so back up, recalculate your position, and try again.’”
Future iterations
The researchers are now designing roboat units roughly four times the size of the current iterations, so they’ll be more stable on water. Mateos is also working on an update to the funnel that includes tentacle-like rubber grippers that tighten around the pin — like a squid grasping its prey. That could help give the roboat units more control when, say, they’re towing platforms or other roboats through narrow canals.
In the works is also a system that displays the AprilTags on an LCD monitor that changes codes to signal multiple roboat units to assemble in a given order. At first, all roboat units will be given a code to stay exactly a meter apart. Then, the code changes to direct the first roboat to latch. After, the screen switches codes to order the next roboat to latch, and so on. “It’s like the telephone game. The changing code passes a message to one roboat at a time, and that message tells them what to do,” Mateos says.
Darwin Caldwell, the research director of Advanced Robotics at the Italian Institute of Technology, envisions even more possible applications for the autonomous latching capability. “I can certainly see this type of autonomous docking being of use in many areas of robotic ‘refuelling’ and docking … beyond aquatic/naval systems,” he says, “including inflight refuelling, space docking, cargo container handling, [and] robot in-house recharging.”
The research was funded by the AMS Institute and the City of Amsterdam.
Autonomous vehicles for social good: Learning to solve congestion
By Eugene Vinitsky
We are in the midst of an unprecedented convergence of two rapidly growing trends on our roadways: sharply increasing congestion and the deployment of autonomous vehicles. Year after year, highways get slower and slower: famously, China’s roadways were paralyzed by a two-week long traffic jam in 2010. At the same time as congestion worsens, hundreds of thousands of semi-autonomous vehicles (AVs), which are vehicles with automated distance and lane-keeping capabilities, are being deployed on highways worldwide. The second trend offers a perfect opportunity to alleviate the first. The current generation of AVs, while very far from full autonomy, already hold a multitude of advantages over human drivers that make them perfectly poised to tackle this congestion. Humans are imperfect drivers: accelerating when we shouldn’t, braking aggressively, and make short-sighted decisions, all of which creates and amplifies patterns of congestion.
On the other hand, AVs are free of these constraints: they have low reaction times, can potentially coordinate over long distances, and most importantly, companies can simply modify their braking and acceleration patterns in ways that are congestion reducing. Even though only a small percentage of vehicles are currently semi-autonomous, existing research indicates that even a small penetration rate, 3-4%, is sufficient to begin easing congestion. The essential question is: will we capture the potential gains, or will AVs simply reproduce and further the growing gridlock?
Given the unique capabilities of AVs, we want to ensure that their driving patterns are designed for maximum impact on roadways. The proper deployment of AVs should minimize gridlock, decrease total energy consumption, and maximize the capacity of our roadways. While there have been decades of research on these questions, there isn’t an existing consensus on the optimal driving strategies to employ, nor easy metrics by which a self-driving car company could assess a driving strategy and then choose to implement it in their own vehicles. We postulate that a partial reason for this gap is the absence of benchmarks: standardized problems which we can use to compare progress across research groups and methods. With properly designed benchmarks we can examine an AV’s driving behavior and quickly assign it a score, ensuring that the best AV designs are the ones to make it out onto the roadways. Furthermore, benchmarks should facilitate research, by making it easy for researchers to rapidly try out new techniques and algorithms and see how they do at resolving congestion.
In an attempt to fill this gap, our CORL paper proposes 11 new benchmarks in centralized mixed-autonomy traffic control: traffic control where a small fraction of the vehicles and traffic lights are controlled by a single computer. We’ve released these benchmarks as a part of Flow, a tool we’ve developed for applying control and reinforcement learning (via using RLlib and rllab as the reinforcement learning libraries) to autonomous vehicles and traffic lights in the traffic simulators SUMO and AIMSUN. A high score in these benchmarks means an improvement in real-world congestion metrics such as average speed, total system delay, and roadway throughput. By making progress on these benchmarks, we hope to answer fundamental questions about AV usage and provide a roadmap for deploying congestion improving AVs in the real world.
The benchmark scenarios, depicted at the top of this post, cover the following settings:
-
A simple figure eight, representing a toy intersection, in which the optimal solution is either a snaking behavior or learning to alternate which direction is moving without conflict.
-
A resizable grid of traffic lights where the goal is to optimize the light patterns to minimize the average travel time.
-
An on-ramp merge in which a vehicle aggressive merging onto the main highway causes a shockwave that lowers the average speed of the system.
-
A toy model of the San-Francisco to Oakland Bay Bridge where four lanes merge to two and then to one. The goal is to prevent congestion from forming so to maximize the number of exiting vehicles.
As an example of an exciting and helpful emergent behavior that was discovered in these benchmarks, the following GIF shows a segment of the bottleneck scenario in which the four lanes merge down to two, with a two-to-one bottleneck further downstream that is not shown. In the top, we have the fully human case in orange. The human drivers enter the four-to-two bottleneck at an unrestricted rate, which leads to congestion at the two-to-one bottleneck and subsequent congestion that slows down the whole system. In the bottom video, there is a mix of human drivers (orange) and autonomous vehicles (red). We find that the autonomous vehicles learn to control the rate at which vehicles are entering the two-to-one bottleneck and they accelerate to help the vehicles behind them merge smoothly. Despite only one in ten vehicles being autonomous, the system is able to remain uncongested and there is a 35% improvement in the throughput of the system.
Once we formulated and coded up the benchmarks, we wanted to make sure that researchers had a baseline set of values to check their algorithms against. We performed a small hyperparameter sweep and then ran the best hyperparameters for the following RL algorithms: Augmented Random Search, Proximal Policy Optimization, Evolution Strategies, and Trust Region Policy Optimization. The top graphs indicate baseline scores against a set of proxy rewards that are used during training time. Each graph corresponds to a scenario and the scores the algorithms achieved as a function of training time. These should make working with the benchmarks easier as you’ll know immediately if you’re on the right track based on whether your score is above or below these values.
From an impact on congestion perspective however, the graph that really matters is the one at the bottom, where we score the algorithms according to the metrics that genuinely affect congestion. These metrics are: average speed for the Figure Eight and Merge, average delay per vehicle for the Grid, and total outflow in vehicles per hour for the bottleneck. The first four columns are the algorithms graded according to these metrics and in the last column we list the results of a fully human baseline. Note that all of these benchmarks are at relatively low AV penetration rates, ranging from 7% at the lowest to 25% at the highest (i.e. ranging from 1 AV in every 14 vehicles to 1 AV in every 4). The congestion metrics in the fully human column are all sharply worse, suggesting that even at very low penetration rates, AVs can have an incredible impact on congestion.
So how do the AVs actually work to ease congestion? As an example of one possible mechanism, the video below compares an on-ramp merge for a fully human case (top) and the case where one in every ten drivers is autonomous (red) and nine in ten are human (white). In both cases, a human driver is attempting to aggressively merge onto the ramp with little concern for the vehicles on the main road. In the fully human case, the vehicles are packed closely together, and when a human driver sharply merges on, the cars behind need to brake quickly, leading to “bunching”. However, in the case with AVs, the autonomous vehicle accelerates with the intent of opening up larger gaps between the vehicles as they approach the on-ramp. The larger spaces create a buffer zone, so that when the on-ramp vehicle merges, the vehicles on the main portion of the highway can brake more gently.
There is still a lot of work to be done; while we’re unable to prove it mathematically, we’re fairly certain that none of our results achieve the optimal top scores and the full paper provides some arguments suggesting that we’ve just found local minima.
There’s a large set of totally untackled questions as well. For one, these benchmarks are for the fully centralized case, when all the cars are controlled by one central computer. Any real road driving policy would likely have to be decentralized: can we decentralize the system without decreasing performance? There are also notions of fairness that aren’t discussed. As the video below shows, bottleneck outflow can be significantly improved by fully blocking a lane; while this driving pattern is efficient, it severely penalizes some drivers while rewarding others, invariably leading to road rage. Finally, there is the fascinating question of generalization. It seems difficult to deploy a separate driving behavior for every unique driving scenario; is it possible to find one single controller that works across different types of transportation networks? We aim to address all of these questions in a future set of benchmarks.
If you’re interested in contributing to these new benchmarks, trying to beat our old benchmarks, or working towards improving the mixed-autonomy future, get in touch via our GitHub page or our website!
Thanks to Jonathan Liu, Prastuti Singh, Yashar Farid, and Richard Liaw for edits and discussions. Thanks to Aboudy Kriedieh for helping prepare some of the videos. This article was initially published on the BAIR blog, and appears here with the authors’ permission.
Joining forces to boost AI adoption in Europe
Europe is gearing up to launch an Artificial Intelligence Public Private Partnership (AI PPP) that brings together AI, data, and robotics. At its core is a drive to lead the world in the development and deployment of trustworthy AI based on EU fundamental rights, principles and values.
The effort is being led by two well-established associations representing over 400 European organisations from Industry and Research: the Big Data Value Association and euRobotics. A first step in this process saw the launch of a consultation document in Brussels last week entitled “Strategic Research, Innovation and Deployment Agenda for an AI PPP”.
The opportunity for Europe
The strategy document comes on the backdrop of international competition, with every country vying to take the lead in AI.
Roberto Viola, Director-General of the European Commission, DG CONNECT, linked it to the recently disputed European Champions League match between Tottenham and Liverpool, which saw an unexpected roster of teams make the final. “Are you sure that China and the US will lead AI? Watch our planes, watch our industrial robots, watch our cars – why are we so shy about our successes? I’ve been around the world, and I’m always asked how Europe can work with other countries in AI. I don’t think we’ve lost the race, in fact I don’t think it’s a race. If it’s a race, it’s about delivering good services in AI to Europeans. We can be in the final of the Champions league.”
Viola says harmonious cross-fertilisation across three dimensions is needed to make AI a success in Europe. First, the EU needs to mainstream AI in society and the economy. Companies have to find their place, and there is a big role for the public to support AI and its introduction in real scenarios. This is the part missing in the EU, compared to US and China. There is a need for more big public procurement. Second, there is a need to push for research and development in AI through European funding programmes, and third, policy is needed to accompany the development of AI in society.
Photo: Roberto Viola, Director-General of the European Commission, DG CONNECT; Credits: Octavian Carare
Trustworthy AI
Viola says “Europe was one of the first to develop ethical codes in AI, and now everyone is doing it. It shows that we know what is important and the impact and relevance of AI for society.” Responsible AI was the leitmotif of the day, with everyone highlighting it as Europe’s unique selling point. The message was clear, Europe can do excellent AI, but is leading in terms of deploying it with society in mind. Juha Heikkilä, Head of the Robotics and Artificial Intelligence Unit at the European Commission says “Europe is doing things in a different way – that takes the citizen into account.”
AI Market Opportunity
The combination of big data, advances in algorithms, computing power, and advanced robotics is opening up new market opportunities for AI enabled systems.
Photo: David Bisset, Director – euRobotics, Sonja Zillner, Siemens AG; Credits: Octavian Carare
Sonja Zillner from Siemens, Co-Chief Editor for the strategy, says “the vision is to boost EU industrial competitiveness and lead the world in development and deployment value-driven trustworthy AI”. When asked for their preferred applications for the development of AI, the public requested improved healthcare services, energy efficiency, and availability of trains, as well as increased productivity in digital factories. This led to the realisation, Zillner adds, that “AI is across all sectors. All the sectors are investing. This is an important take-home – working across sectors is really central. We want to leverage AI driven market opportunity, across all sectors.”
A European AI Framework
David Bisset, Executive Director of euRobotics and Co-Chief Editor of the strategy presented an AI Framework that builds on the legal and societal fabric that underpins the impact of AI in Europe. Central to the success of this framework will be an ecosystem that brings together skills, data, and environments to experiment and deploy the technology. Bisset says “we need data stores, regulatory sandboxes that allow us to test robots in cities without breaking the rules, we need EU regulation that creates a level playing field”. New technologies that work across sectors are needed for sensing, measurement and perception, continuous and integrated knowledge, trustworthy, hybrid decision making, physical and human action and interaction, systems, methodologies, and hardware.
Boosting the adoption of AI in Europe faces several challenges however, including the lack of skills, technology problems, lack of private investment, complexity of deploying products, and the policy and regulation landscape. Bisset says “We need a collective action from all stakeholders to address these challenges – we need an AI Innovation ecosystem”. Stakeholders include researchers, innovators, technology creators, regulators, users, citizens, investors, data suppliers, and application providers.
The implementation of the AI PPP will address the following Working Areas.
WA1: Mobilising the EU AI ecosystem
WA2: Skills and acceptance
WA3: Innovation and market enablers
WA4: Guiding standards and regulation
WA5: Promoting Research Excellence
Words of Wisdom
The event closed with a panel discussion, here are some nuggets of wisdom.
Photo: Panel; Credits: Octavian Carare
“We will not have enough people to treat patients. Without AI, without robotics, it will be a disaster for patients. Whatever the cost, patients will demand their treatments. Treatments will be better, more precise with AI.” Rolf Roussaint, Director of Anaesthesiology at University Hospital Aachen.
“We need to measure that AI is bringing benefits – measurable AI is important, maybe more important than explainable AI” Henk-Jan Vink, TNO Managing Director Unit ICT.
“We should not be too shy about what the EU is doing in AI – we should be proud.”
“We need to be inclusive, it’s not robotics vs AI. We see robotics and AI as two sides of the same coin. One is a physical instantiation of AI. We need to join forces.” Juha Heikkilä, Head of Unit, Robotics and Artificial Intelligence, DG CONNECT, European Commission.
“Don’t fall in love with the technology – researchers are in love with the technology – and industry with profit. Instead we need use cases proving the new benefits for services and impacts on quality of life that AI can bring.” Gabriella Cattaneo, Associate Vice President of IDC4EU European Government Consulting Unit.
“There will be no progress for AI if we can’t find a way for researchers and startups to have access to data.” Hubert Tardieu, ATOS, Advisor to the CEO.
“In China and the US data is not an issue. The EU doesn’t have that data however – or it’s not shared due to concern. Focussing on the citizen is really important, but we also need to push for access to data.” Federico Milani, Deputy Head of Unit, Data Policies and Innovation, DG CONNECT, European Commission.
Call for collaboration
To conclude the event, Thomas Hahn, President of BDVA and Bernd Liepert, President of euRobotics, moderators of this event and the panel, launched a call for participation and collaboration to all European players active in this domain and committed to boost AI adoption in Europe!
Photo: Bernd Liepert, President of euRobotics, Thomas Hahn, President of BDVA, Credits: Octavian Carare