Interview with Yuki Mitsufuji: Improving AI image generation
Yuki Mitsufuji is a Lead Research Scientist at Sony AI. Yuki and his team presented two papers at the recent Conference on Neural Information Processing Systems (NeurIPS 2024). These works tackle different aspects of image generation and are entitled: GenWarp: Single Image to Novel Views with Semantic-Preserving Generative Warping and PaGoDA: Progressive Growing of a One-Step Generator from a Low-Resolution Diffusion Teacher . We caught up with Yuki to find out more about this research.
There are two pieces of research we’d like to ask you about today. Could we start with the GenWarp paper? Could you outline the problem that you were focused on in this work?
The problem we aimed to solve is called single-shot novel view synthesis, which is where you have one image and want to create another image of the same scene from a different camera angle. There has been a lot of work in this space, but a major challenge remains: when an image angle changes substantially, the image quality degrades significantly. We wanted to be able to generate a new image based on a single given image, as well as improve the quality, even in very challenging angle change settings.
How did you go about solving this problem – what was your methodology?
The existing works in this space tend to take advantage of monocular depth estimation, which means only a single image is used to estimate depth. This depth information enables us to change the angle and change the image according to that angle – we call it “warp.” Of course, there will be some occluded parts in the image, and there will be information missing from the original image on how to create the image from a new angle. Therefore, there is always a second phase where another module can interpolate the occluded region. Because of these two phases, in the existing work in this area, geometrical errors introduced in warping cannot be compensated for in the interpolation phase.
We solve this problem by fusing everything together. We don’t go for a two-phase approach, but do it all at once in a single diffusion model. To preserve the semantic meaning of the image, we created another neural network that can extract the semantic information from a given image as well as monocular depth information. We inject it using a cross-attention mechanism, into the main base diffusion model. Since the warping and interpolation were done in one model, and the occluded part can be reconstructed very well together with the semantic information injected from outside, we saw the overall quality improved. We saw improvements in image quality both subjectively and objectively, using metrics such as FID and PSNR.
Can people see some of the images created using GenWarp?
Yes, we actually have a demo, which consists of two parts. One shows the original image and the other shows the warped images from different angles.
Moving on to the PaGoDA paper, here you were addressing the high computational cost of diffusion models? How did you go about addressing that problem?
Diffusion models are very popular, but it’s well-known that they are very costly for training and inference. We address this issue by proposing PaGoDA, our model which addresses both training efficiency and inference efficiency.
It’s easy to talk about inference efficiency, which directly connects to the speed of generation. Diffusion usually takes a lot of iterative steps towards the final generated output – our goal was to skip these steps so that we could quickly generate an image in just one step. People call it “one-step generation” or “one-step diffusion.” It doesn’t always have to be one step; it could be two or three steps, for example, “few-step diffusion”. Basically, the target is to solve the bottleneck of diffusion, which is a time-consuming, multi-step iterative generation method.
In diffusion models, generating an output is typically a slow process, requiring many iterative steps to produce the final result. A key trend in advancing these models is training a “student model” that distills knowledge from a pre-trained diffusion model. This allows for faster generation—sometimes producing an image in just one step. These are often referred to as distilled diffusion models. Distillation means that, given a teacher (a diffusion model), we use this information to train another one-step efficient model. We call it distillation because we can distill the information from the original model, which has vast knowledge about generating good images.
However, both classic diffusion models and their distilled counterparts are usually tied to a fixed image resolution. This means that if we want a higher-resolution distilled diffusion model capable of one-step generation, we would need to retrain the diffusion model and then distill it again at the desired resolution.
This makes the entire pipeline of training and generation quite tedious. Each time a higher resolution is needed, we have to retrain the diffusion model from scratch and go through the distillation process again, adding significant complexity and time to the workflow.
The uniqueness of PaGoDA is that we train across different resolution models in one system, which allows it to achieve one-step generation, making the workflow much more efficient.
For example, if we want to distill a model for images of 128×128, we can do that. But if we want to do it for another scale, 256×256 let’s say, then we should have the teacher train on 256×256. If we want to extend it even more for higher resolutions, then we need to do this multiple times. This can be very costly, so to avoid this, we use the idea of progressive growing training, which has already been studied in the area of generative adversarial networks (GANs), but not so much in the diffusion space. The idea is, given the teacher diffusion model trained on 64×64, we can distill information and train a one-step model for any resolution. For many resolution cases we can get a state-of-the-art performance using PaGoDA.
Could you give a rough idea of the difference in computational cost between your method and standard diffusion models. What kind of saving do you make?
The idea is very simple – we just skip the iterative steps. It is highly dependent on the diffusion model you use, but a typical standard diffusion model in the past historically used about 1000 steps. And now, modern, well-optimized diffusion models require 79 steps. With our model that goes down to one step, we are looking at it about 80 times faster, in theory. Of course, it all depends on how you implement the system, and if there’s a parallelization mechanism on chips, people can exploit it.
Is there anything else you would like to add about either of the projects?
Ultimately, we want to achieve real-time generation, and not just have this generation be limited to images. Real-time sound generation is an area that we are looking at.
Also, as you can see in the animation demo of GenWarp, the images change rapidly, making it look like an animation. However, the demo was created with many images generated with costly diffusion models offline. If we could achieve high-speed generation, let’s say with PaGoDA, then theoretically, we could create images from any angle on the fly.
Find out more:
- GenWarp: Single Image to Novel Views with Semantic-Preserving Generative Warping, Junyoung Seo, Kazumi Fukuda, Takashi Shibuya, Takuya Narihira, Naoki Murata, Shoukang Hu, Chieh-Hsin Lai, Seungryong Kim, Yuki Mitsufuji.
- GenWarp demo
- PaGoDA: Progressive Growing of a One-Step Generator from a Low-Resolution Diffusion Teacher, Dongjun Kim, Chieh-Hsin Lai, Wei-Hsiang Liao, Yuhta Takida, Naoki Murata, Toshimitsu Uesaka, Yuki Mitsufuji, Stefano Ermon.
About Yuki Mitsufuji
![]() |
Yuki Mitsufuji is a Lead Research Scientist at Sony AI. In addition to his role at Sony AI, he is a Distinguished Engineer for Sony Group Corporation and the Head of Creative AI Lab for Sony R&D. Yuki holds a PhD in Information Science & Technology from the University of Tokyo. His groundbreaking work has made him a pioneer in foundational music and sound work, such as sound separation and other generative models that can be applied to music, sound, and other modalities. |
Repurposed Roombas: Scientists program domestic robots for additional household tasks
High-wire act: Soft robot can carry cargo up and down steep aerial wires
Automate 2025 Q&A with igus
Google AI Offers Free Ride for College Students
In an extremely aggressive promotion, Google is offering U.S. college students a free, one-year ride on Google One AI Premium — a fierce competitor to ChatGPT.
The deal translates into $20/month savings for a year — and gives those students access to some of the most advanced AI on the planet, including the Gemini Advanced chatbot, Deep Research, text editor Canvas and auto-video generation.
Observes Josh Woodward, vice president, Google Labs & Google Gemini: “To top all of this off, you’ll get 2 TB of storage, providing plenty of space for school projects, research, high-resolution media and your personal photos or videos.”
Currently, students are the number one users of Google’s chief competitor, ChatGPT, according to ChatGPT-maker OpenAI.
In other AI news and analysis:
*New ChatGPT AI Engine Smarter than 98% of Humans: Stick a fork in it: Apparently, the battle of wits between humans AI is so yesterday — and we flesh-bags have lost.
New test results from Mensa — the global group of the rumoredly smartest people in the world — show that one of ChatGPT’s newest AI engines, o3, has an IQ of 136.
Observes writer Liam Wright: “The score, calculated from a seven-run rolling average, places the model above approximately 98% of the human population, according to a standardized bell-curve IQ distribution used in the benchmarking.”
Currently, ChatGPT runs on a number of specialized AI engines — including ChatGPT-40, which is rated best overall for writing.
ChatGPT-03 was designed to excel in reasoning, math and other hard sciences applications.
*Grok AI Chatbot Adds AI Writing Editor: Elon Musk’s answer to ChatGPT — the Grok AI Writer/Chatbot — has added an online editor for use when working with writing or code.
Dubbed Grok Studio, the editor is similar to the online editor ‘Canvas’ tool that ChatGPT added a few months back — which is also featured in a similar form on the Google AI chatbot Gemini.
Observes writer Eric Hal Schwartz: “One element that stands out, though, is that Grok Studio links with Google Drive and can pull in your files directly from Drive, including documents, spreadsheets and presentations.”
*ChatGPT Now Synthesizes Its Knowledge of You When Searching: ChatGPT will now synthesize analysis of how you use the chatbot when you do searches with the chatbot.
The result: Ideally, you should see more personalized results from your ChatGPT search, based on what ChatGPT thinks you’re looking for.
Observes writer Kyle Wiggers: “For example, for a user that ChatGPT ‘knows’ from memory is vegan and lives in San Francisco, ChatGPT may rewrite the prompt ‘what are some restaurants near me that I’d like’ as ‘good vegan restaurants, San Francisco.'”
*Quick Study: Update on ChatGPT’s Flurry of New Features: AI expert Kevin Stravert offers a great ‘How To’ overview on the flurry of new features that have popped-up in ChatGPT during the past few months in this video.
Click to this video for tips on how to get ChatGPT to do your research for you while you work on other tasks, AI-automate use of your writing and related apps — and much more.
Observes Stravert: “Whether you’re a student, creator, or professional, these updates are designed to supercharge your productivity and creativity.”
*United Arab Emirates Now Writing Laws With AI: While some industries fret over the implications of implementing AI, the UAE law community has gone full throttle instead.
Observes TechInAsia: “This initiative represents a significant change in the UAE’s legislative processes.
“The newly established Regulatory Intelligence Office will oversee this initiative, which aims to expedite law creation.”
*For Many, An Outrage: Some California Bar Exam Questions Were Written by AI: More than a few members of the California legal community are incensed that AI was used to help write some questions for the state’s Bar Exam.
Observes writer Benj Edwards: “The State Bar disclosed that its psychometrician — a person or organization skilled in administrating psychological tests, ACS Ventures — created 23 of the 171 scored multiple-choice questions with AI assistance.
Adds Mary Basick, assistant dean of academic skills, University of California: “The debacle that was the February 2025 bar exam is worse than we imagined.
“I’m almost speechless. Having the questions drafted by non-lawyers using artificial intelligence is just unbelievable.”
*Australians Duped: Radio DJ Presented as Human Is Really an AI: Listeners to ‘Australia’s Home of Hip Hop and R&B’ have been gas-lighted: The DJ for the show — presented as human — is really just AI-generated.
Essentially, the DJ has been on the air for about six months “without any disclosure that it’s an AI-generated presenter,” according to writer Simon Thomsen.
Adds Teresa Lim, vice president, Australian Association of Voice Actors: “Listeners deserve honesty and upfront disclosure — instead of a lack of transparency.”
*Chinese Competitor to ChatGPT ‘Profound Threat’ to U.S. Security: DeepSeek, the AI writer/chatbot that roiled the stock market in early 2025 after it was revealed that it only cost $6 million to create, is a profound security threat to the U.S., according to a U.S. Congressional Committee.
According to the committee’s report on DeepSeek, “the app siphons data back to the People’s Republic of China (PRC), creates security vulnerabilities for its users — and relies on a model that covertly censors and manipulates information pursuant to Chinese law.
“For these reasons, it is evident that the DeepSeek Web site and app act as a direct channel for foreign intelligence gathering on Americans’ private data.”
AI BIG PICTURE: AI ‘Pulse Check’ from the ‘Godfather of AI:’ Nobel laureate and key developer of AI Geoffrey Hinton is out with a new interview — and a new dose of potential gloom and doom.
Hinton, a former AI researcher at Google who left so he could more freely talk about AI’s dangers now says in this April 2025 interview that the emergence of AI agents — which enable AI to work independently from humans — has increased the chance that humanity could lose control of AI.
While Hinton freely admits that the ultimate trajectory of AI — either as an overall catalyst of good or evil in the world — is anyone’s guess, he adds that humanity needs to work much harder to prevent a dystopian outcome.
One of the key threats of AI’s breakneck development, according to Hinton: Bad actors who harness the tech for malicious — and potentially massively destructive ends.
Observes Hinton: “We’re at this very, very special point in history where in a relatively short time, everything might totally change — a change of scale we’ve never seen before.”
Bottom line: If you’re looking for an extremely in-depth, extremely informed and extremely insightful overarching look at the current — and short-term future — of AI, this 51-minute video is your ticket.
The video is presented by ‘CBS Mornings’ and squired by extremely talented and AI-knowledgeable interviewer, Brook Silva-Braga.

Share a Link: Please consider sharing a link to https://RobotWritersAI.com from your blog, social media post, publication or emails. More links leading to RobotWritersAI.com helps everyone interested in AI-generated writing.
–Joe Dysart is editor of RobotWritersAI.com and a tech journalist with 20+ years experience. His work has appeared in 150+ publications, including The New York Times and the Financial Times of London.
The post Google AI Offers Free Ride for College Students appeared first on Robot Writers AI.
Microrobots powered by thin-film actuator can morph, lock shapes and operate untethered
Cutting the complexity from digital carpentry
Robot Talk Episode 118 – Soft robotics and electronic skin, with Miranda Lowther
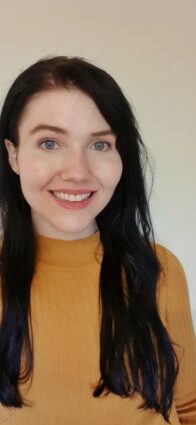
Claire chatted to Miranda Lowther from the University of Bristol about soft, sensitive electronic skin for prosthetic limbs.
Miranda Lowther is a PhD researcher at the FARSCOPE-TU Centre for Doctoral Training, a joint venture between University of Bristol, University of West of England, and Bristol Robotics Laboratory, where she is pursuing her passion for using soft robotics and morphological computation to help people in healthcare. For her PhD, she is investigating how soft e-skins and morphological computation concepts can be used to improve prosthetic user health, comfort, and quality of life, through sensing and adaptation.